7 Critical Responsibilities of Project Managers in Enterprise AI Implementations
7 Critical Responsibilities of Project Managers in Enterprise AI Implementations - Defining Strategic Alignment and Value Proposition for AI Projects
Successfully implementing AI projects hinges on a clear understanding of how they fit within the broader organizational landscape and deliver real value. This means aligning AI initiatives with the organization's core purpose, its current strengths and resources, and its competitive environment. A well-defined AI strategy should emerge from this alignment, providing a path forward.
Leaders must translate the transformative promise of AI into clear and measurable goals. Establishing key performance indicators (KPIs) is crucial, especially in light of the significant impact generative AI is having. However, simply aiming for AI adoption isn't enough. Project managers must grapple with the intricate process of integrating AI solutions into existing operational frameworks. This involves navigating the complexities of technical integration and addressing potential roadblocks to smooth functionality.
Ultimately, AI projects rely on a collaborative approach. Engaging stakeholders and ensuring everyone understands the goals and how their efforts contribute to achieving them is critical to project success. AI initiatives must demonstrate tangible value in relation to broader business aims to truly justify their implementation.
Within the realm of AI initiatives, a clear articulation of strategic alignment and the resultant value proposition is paramount. Recent studies suggest a strong correlation between achieving project goals and a well-defined alignment with broader organizational objectives. Interestingly, a lack of clarity regarding the expected value from an AI project is cited as a primary cause for a high failure rate among these endeavors.
It's intriguing how a strong strategic fit, encompassing both organizational mission and capabilities, can lead to tangible improvements in project efficiency and resource allocation. When AI initiatives are carefully connected to the core business strategy, project teams tend to stay focused, reducing extraneous features and, in turn, contributing to a more streamlined budget. This strategic alignment also plays a key role in fostering internal buy-in. Employees who understand how AI contributes to business goals are more likely to support and actively participate in these projects.
Beyond internal alignment, a clearly communicated value proposition can greatly impact how external stakeholders perceive the initiative. This, in turn, can improve their engagement and participation. The ability to adapt to the dynamic landscape of the market is another area where strategically aligned AI projects excel. Organizations that can adjust to changing market needs are more resilient, suggesting that careful foresight and alignment are crucial elements in building adaptable and future-proof AI strategies.
A related observation highlights how customer satisfaction can be greatly impacted by a clear understanding of the value proposition. When AI solutions are built around defined needs, rather than theoretical use cases, the end product is more likely to meet actual customer expectations. It's concerning, though, that a lack of upfront clarity frequently results in the "scope creep" phenomenon, leading to unforeseen expenses and delays. This underscores the importance of setting clear and measurable goals and sticking to them.
Finally, it's notable that businesses with cohesive AI strategies often garner a stronger perception in the industry, which can contribute to a substantial improvement in their competitive standing. Ultimately, organizations that weave AI initiatives into the fabric of their business strategy can achieve a powerful synergy, boosting both operational effectiveness and market positioning. These findings suggest that in the ever-evolving landscape of AI implementations, a well-defined strategic roadmap, alongside a compelling value proposition, might well be the cornerstone of project success.
7 Critical Responsibilities of Project Managers in Enterprise AI Implementations - Leveraging AI Tools for Task and Resource Management
Incorporating AI tools into task and resource management within enterprise AI projects offers significant potential for improvement, but it also requires careful consideration by project managers. AI can streamline many aspects of project management, automating repetitive tasks like scheduling and resource allocation, which frees up project managers to tackle more strategic challenges and complex decision-making. Furthermore, the real-time insights provided by AI improve project tracking, allowing for faster identification of potential risks and more proactive responses.
However, the integration of AI into established workflows needs careful navigation to avoid common pitfalls. Over-reliance on AI could lead to neglecting critical human oversight, potentially hindering the development of critical thinking and problem-solving skills within project teams. Additionally, it's crucial to ensure that AI-driven solutions are properly aligned with the overall project goals to prevent deviations from the original plan. The key to successful implementation lies in striking a balance between the benefits offered by AI's automation and optimization capabilities, and the need for human judgment, strategic thinking, and continuous monitoring to guarantee that projects remain on track and achieve their objectives. Ultimately, a measured and well-considered approach is essential to maximize the value derived from AI in task and resource management within enterprise AI implementations.
AI is increasingly being used in project management to automate routine tasks like scheduling and resource allocation, freeing up project managers to focus on more complex, strategic issues and problem-solving. This shift allows for a more proactive approach to project management, as AI tools can provide real-time insights and predict potential risks early on. This kind of foresight allows for quicker adjustments, preventing minor problems from escalating into major roadblocks.
A recent PMI survey indicates that the use of AI in project management is on the rise, with 21% of respondents using it frequently. Furthermore, a significant majority of senior leaders (82%) expect AI to have a considerable impact on project management in their organizations within the next five years. The same research also reveals that businesses utilizing AI in project management see tangible improvements. For example, they are 30% more likely to deliver projects on time and 23% more likely to meet their projected ROI.
One of the key advantages of incorporating AI into project management is the improved ability to identify potential issues before they disrupt a project's timeline. AI tools can analyze vast amounts of data and identify anomalies that might otherwise go unnoticed. This enhanced oversight strengthens the project manager's ability to manage risk and ensure smoother operations. The application of machine learning, data analytics, and automation through AI significantly streamlines workflows and improves decision-making.
AI's transformative potential extends to all phases of a project: planning, execution, and monitoring. By integrating AI tools into these phases, organizations can expect to see increased efficiency in project delivery and overall project outcomes. From the initial stages of gathering information to the final stages of resource allocation and risk assessment, AI-powered tools can manage every aspect of project workflows. While the potential benefits are exciting, it's important to remain aware of the potential challenges and risks of incorporating AI into project management workflows. There's always a need to ensure a clear understanding of AI's role in the larger organizational context to optimize implementation.
7 Critical Responsibilities of Project Managers in Enterprise AI Implementations - Building and Leading Effective AI Implementation Teams
Successfully implementing AI within an enterprise relies heavily on having strong, well-structured teams. These teams need a mix of skills and viewpoints to encourage creativity and ensure the ultimate goal is improving the business, not just showcasing technical abilities. Having these teams report directly to high-level executives helps keep the projects aligned with the organization's larger goals. This is especially important in building a company-wide culture where AI is seen as a key part of future operations.
Further, effective leadership is vital in fostering collaboration between different stakeholders—such as data specialists and subject matter experts—to find actual opportunities where AI can be used to enhance offerings or products. The process of creating these teams should be carefully planned out with clearly defined roles and responsibilities. Most importantly, there needs to be a shared sense of purpose and commitment to achieving specific outcomes that are in line with the larger aims of the organization.
Building and leading effective AI implementation teams is a complex endeavor, made even more challenging by the evolving nature of the field. Research increasingly points to the importance of team composition, highlighting the need for a diverse range of skills beyond just data science. Having domain experts, behavioral scientists, and even those with ethical considerations in the mix appears crucial for sound decision-making and innovative problem-solving.
However, a significant hurdle lies in the shortage of individuals with the necessary skills. Reports indicate that a large proportion of companies struggle to find people who are proficient in both AI and project management. This skills gap underscores the need for better training and education programs to address the demand.
Beyond technical skills, the soft skills of effective communication are proving to be a crucial factor in AI project success. Teams that foster open dialogue are more likely to achieve their objectives. This suggests that project managers must dedicate effort to creating and maintaining an environment where open communication is valued and actively encouraged.
It's interesting, though, that some of the obstacles are less obvious and perhaps a bit more human in nature. Research indicates that teams working on AI projects, much like other teams, are prone to biases. Confirmation bias, specifically, can lead to teams selecting information that reinforces existing beliefs, ignoring other evidence that might offer a more objective perspective. This can lead to less than optimal solutions, highlighting the need for project managers to encourage a more critical and objective assessment of available information.
Intriguingly, the effectiveness of a team is also influenced by how much time it dedicates to non-technical activities. Evidence suggests that successful AI project teams set aside a substantial chunk of their time—up to 25%—for team building, conflict resolution, and relationship-building activities. This seemingly counterintuitive practice points to the significance of the human element, fostering both collaboration and a culture of innovation.
Further research has explored the influence of emotional intelligence compared to just technical skills within the context of AI project teams. It is somewhat surprising that emotional intelligence appears to be a better predictor of project success than simply having technical expertise. This suggests that fostering a team environment where emotional intelligence is valued, and conflicts are handled with care, may be more beneficial than simply focusing on technical talent.
Similarly, the diversity of the team seems to be a key factor in success. Research consistently shows that more diverse teams are better at generating creative solutions. AI projects that lack a range of diverse backgrounds, perspectives, and ways of thinking, may well be missing out on vital insights and innovation. The leader’s approach also influences the team's performance, with transformational leaders inspiring and motivating team members, fostering greater commitment and enhancing project outcomes.
Implementing regular feedback loops is also a vital aspect of the process, with evidence demonstrating that performance can be improved by a significant amount by doing so. Such feedback mechanisms provide a space for ongoing learning and adaptation, helping the team respond to unforeseen challenges and adjust their strategies as needed.
Despite these insights, we face a sobering fact. The failure rate of AI projects remains worryingly high, with a significant number not achieving their intended goals. This serves as a powerful reminder that effectively managing and leading AI implementation teams requires a well-defined strategy and ongoing vigilance. It requires careful attention to team composition, effective communication, and the nurturing of emotional intelligence. If these elements are in place, there's a greater chance that these efforts will prove successful.
7 Critical Responsibilities of Project Managers in Enterprise AI Implementations - Identifying and Mitigating Risks in AI Project Execution
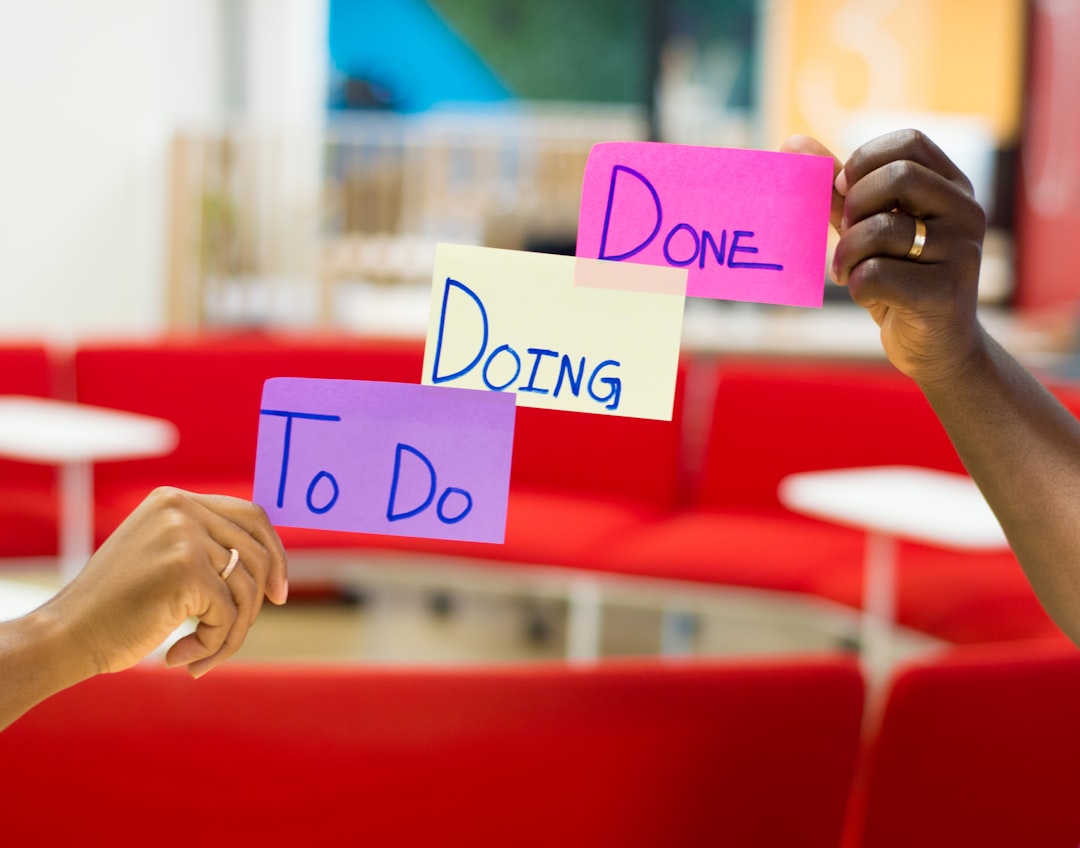
Successfully executing AI projects within an enterprise requires project managers to effectively identify and manage inherent risks. The complex nature of these implementations means that a failure to address risks proactively can easily lead to project failure. It's crucial for project managers to start by establishing clear project goals and then meticulously identify potential obstacles that might hinder progress. Furthermore, these risks need to be prioritized to ensure that the most impactful ones receive immediate attention. A robust risk management strategy should involve a dynamic and adaptive approach. This means continually monitoring and adjusting risk mitigation plans as the project unfolds and as external conditions shift. AI offers powerful tools for enhancing risk management. By utilizing AI, project managers can gain a deeper understanding of potential risks and develop more precise mitigation strategies, thereby improving the likelihood of successful project delivery. It's essential to note, however, that project managers should never lose sight of the importance of human judgment and critical thinking when leveraging AI for risk management. Over-reliance on AI-driven insights can potentially lead to neglecting crucial human aspects of problem-solving, hindering the ability to adapt to unforeseen challenges. A balanced approach that integrates the power of AI while retaining human oversight is critical for optimal project outcomes.
Successfully implementing AI projects requires a keen awareness of the potential hurdles that can derail even the most promising initiatives. One frequent misstep is overestimating what AI can realistically achieve. Research indicates a surprisingly high rate of AI project budget and timeline overruns, often stemming from inflated expectations about AI capabilities. This suggests that a more grounded perspective, recognizing the limitations of current AI technology, is crucial for realistic project planning.
Beyond technical concerns, navigating organizational culture can pose unexpected challenges. Resistance to change, both from fear of job displacement and from ingrained routines, can impede AI adoption and cause frustrating delays. It seems that humans, despite working on projects that seek to enhance our abilities, sometimes have a rather human and predictable resistance to new ideas.
The data underpinning AI systems is also a major point of vulnerability. AI's accuracy relies heavily on the quality of its input, and studies suggest a substantial amount of project time is dedicated to preparing and cleaning data. Poor data leads to unreliable outcomes, highlighting the vital role of data integrity in achieving intended project results.
Moreover, the potential for AI to inadvertently amplify existing biases in its training data is a serious concern. This isn't simply a technical concern but touches on ethical aspects of AI, potentially leading to unfair or discriminatory outcomes. It appears that ensuring AI systems are free from unintended biases requires careful consideration at all stages of development.
Adding to the complexity, the growing demand for AI expertise outpaces the current supply of skilled professionals. This skill gap leads to difficulties in finding individuals who can effectively manage AI project lifecycles, a challenge reported by a large proportion of organizations.
Closely linked to this is the risk of misalignment. When AI projects lack a clear connection to the overarching business strategy, the probability of failure rises dramatically. It appears that projects that lack strategic alignment are especially susceptible to failure, underscoring the importance of a well-defined strategic framework.
The costs of AI projects also require careful attention. Unexpected costs stemming from integration difficulties and ongoing maintenance can easily exceed initial estimates. This suggests that a comprehensive cost-benefit analysis and a detailed plan for handling unforeseen expenses is critical during the planning phase.
Working with diverse teams, comprised of individuals with varied expertise, is often associated with improved project success. Research suggests that these collaborative, multidisciplinary teams are more likely to deliver on time and within budget compared to teams with a more homogenous skillset. It would seem that encouraging collaborative, multidisciplinary teams is a wise investment in project success.
Effective communication across the entire project stakeholder group is another vital factor in project success. Studies show that projects with clear, consistent communication amongst involved parties are more likely to achieve their objectives. It appears that a well-defined communication strategy that fosters a shared understanding of the project goals, risks, and potential outcomes is essential.
Finally, continuous feedback loops are critical to adaptability and successful project outcomes. Teams that embrace continuous feedback are more flexible and responsive to challenges, improving their ability to adjust strategies effectively and leading to measurable improvements. It appears that fostering a culture of iterative feedback allows teams to continually learn and refine their approach, resulting in more robust and resilient outcomes.
It's clear that AI project management poses unique challenges that necessitate a proactive, informed approach. By understanding the potential pitfalls, organizations can mitigate risks, enhancing their chances of realizing the transformative potential of AI.
7 Critical Responsibilities of Project Managers in Enterprise AI Implementations - Ensuring Data Quality and Relevance for AI Model Training
The success of AI model training hinges on the quality and relevance of the data used. AI models are only as good as the information they're trained on, and poor data leads to unreliable and potentially harmful outcomes. Project managers need to carefully manage the complexities of data in AI projects, including the sheer volume of data that can be involved. Large datasets can introduce errors, inconsistencies, and gaps, impacting the accuracy of the trained models.
High-quality data ensures AI models generate useful and accurate insights, while poor-quality data can lead to incorrect predictions and decisions. Project managers must ensure data collection strategies are well-planned, using iterative processes to improve the quality and relevance of data over time. This might include focusing on reducing biases and improving data completeness. Dealing with the challenges of data quality effectively helps ensure AI projects deliver the intended value within the enterprise. Failing to address data quality issues can result in significant project setbacks and ultimately hinder the realization of expected benefits.
The effectiveness of AI models hinges on the quality and relevance of the data they're trained on. It's interesting to find that focusing on relevant data, even with smaller datasets, can yield better results than using vast amounts of irrelevant data. This highlights how important understanding the context of the data is when choosing it for training.
However, AI models can unintentionally inherit and amplify biases present in the data they learn from. To avoid skewed results, organizations must scrutinize their datasets for representation and balance. This is especially important when dealing with things like sensitive topics in areas like healthcare or finance.
Preparing data for use in AI models is a significant time investment, sometimes taking up to 80% of the project time. This underscores the need for establishing good data preparation processes that happen before model training. Ideally, this helps ensure data quality before it impacts the model.
Furthermore, even small changes in the data can noticeably alter a model's performance. So, it's important to carefully track those changes through a version control system for the datasets. This ensures we can pinpoint if and when the model's performance shifts.
Even sophisticated AI systems can make errors, often stemming from misinterpreting data patterns. This is why human oversight is crucial, especially when dealing with crucial applications where mistakes can be severe, like healthcare or finance. It can also help to understand why a model arrived at a certain decision.
While models might perform well in controlled settings, they can fail unexpectedly in the real world. This underlines the need for thorough testing in a variety of operational conditions before using a model in a real-world setting.
It's beneficial to regularly check the quality of the data to avoid long-term issues. Poor data quality is a strong predictor of model inaccuracy. Having regular quality checks can stop problems before they get worse.
As data changes, so should the data used for training. If this isn't done, models can become outdated quickly, making solutions that were initially great less effective.
The growing concern about data privacy means regulations like GDPR are becoming more important. Adhering to these rules isn't just a legal requirement, but it can also affect the model training process if access to certain data is restricted.
Collaboration is key when curating data. Bringing together people from different parts of the organization and from various fields has been shown to improve data quality and relevance. Having a variety of viewpoints makes it easier to get a thorough understanding of the data and how to use it.
7 Critical Responsibilities of Project Managers in Enterprise AI Implementations - Balancing Automation and Strategic Planning in Project Leadership
In the realm of project leadership, especially within the context of enterprise AI, the delicate balance between automation and strategic planning is paramount. While AI tools offer compelling opportunities to automate routine tasks and streamline operations, there's a risk of over-reliance, potentially diminishing the role of human judgment and strategic foresight. Successfully integrating AI requires project managers to skillfully harmonize the strengths of automation with the unique value of human insights. Maintaining a strong connection between AI-driven initiatives and the larger organizational goals is crucial. As AI continues to advance, project managers must navigate this evolving landscape, ensuring that automation enhances rather than overshadows strategic planning and stakeholder engagement. Ignoring this crucial balance can lead projects astray, potentially resulting in a disconnect from core objectives and, ultimately, project failure.
The increasing adoption of AI in project management, as seen in the 2023 PMI survey, is reshaping the landscape of project leadership. While 21% of respondents are already employing AI, and 82% of senior leaders anticipate its influence on project operations in the next five years, it's crucial to understand how to balance automation with strategic planning. AI can automate repetitive tasks, improving resource allocation and accelerating reporting, but this efficiency shouldn't come at the cost of strategic thinking and human judgment.
It's intriguing to observe how the introduction of AI can impact a project team's cognitive load. Too much automation might overwhelm team members, potentially hindering their ability to make informed decisions. Project managers must carefully curate the use of automation to avoid this pitfall, ensuring AI serves as a supportive tool rather than an overwhelming master. Furthermore, clear communication is vital, not just within the project team but also with other stakeholders. Research shows that effective communication can substantially improve outcomes by encouraging collaboration and a shared understanding of goals.
AI-powered insights are a powerful asset, but they shouldn't replace human critical thinking. Project teams need a space to analyze data generated by automated systems and engage in reflective discussions. This human touch is needed to catch biases in the data and identify blind spots that the AI might not recognize. Interestingly, emotional intelligence appears to be an increasingly important factor in team leadership. Research indicates that leaders who demonstrate high emotional intelligence are better at navigating the inevitable changes that occur during projects.
While AI can automate resource allocation, we need to be cautious about blindly relying on these automated processes. Research suggests that continuous human involvement in automated decision-making processes results in higher project success rates. Moreover, AI systems can sometimes perpetuate biases that are present in the data they are trained on. It's important to examine the algorithms and decision-making parameters in automated tools to mitigate this risk, ensuring human oversight guides the system towards strategic goals.
The dynamic nature of today's business environment requires project managers to strike a balance between automation's reliability and the ability to adapt. Organizations with strategic agility consistently outperform rigid organizations. Therefore, it's vital for project managers to incorporate human-led adjustments to project plans based on feedback and changes in the project's environment. Similarly, automated feedback mechanisms are valuable, but they need to be carefully integrated into a structure that promotes actionable insights. Otherwise, these insights can easily become simply another data point rather than a catalyst for adjustments in strategy.
It's surprising to discover that the very introduction of AI, while meant to streamline tasks and reduce burdens, can sometimes meet with resistance. Research indicates that the perception of automation as a job threat can lead to decreased engagement among team members. This emphasizes the critical role of transparent communication regarding how automation supports—not replaces—human roles. By managing the implementation of AI carefully and focusing on clear communication, project managers can leverage the strengths of automation without inadvertently creating negative impacts on team morale or hindering the progress of their projects.
In conclusion, AI's potential to optimize project management is clear, but it requires a nuanced approach from project leaders. Balancing automation with strategic planning, fostering effective communication, and integrating human judgment alongside algorithmic outputs are crucial. Successfully navigating this intricate interplay will be a defining characteristic of project management in the years to come, leading to more efficient, insightful, and ultimately, more successful projects.
7 Critical Responsibilities of Project Managers in Enterprise AI Implementations - Fostering Organizational Integration of AI Technologies
Integrating AI technologies into an organization requires a thoughtful and strategic plan that aligns AI projects with the overarching business goals. This integration is essential for driving innovation and future growth, but it often comes with obstacles, like resistance to change and mismatches with current workflows. A key ingredient for a successful integration of AI is the collaboration between different teams, each with its own specialized knowledge. Data scientists, subject matter experts, and project managers can work together, leveraging their unique skills to enhance the organization's abilities. Additionally, cultivating a workplace culture that embraces AI and ensuring everyone involved understands the project's purpose are crucial to making AI implementations a success. As businesses undergo digital transformation, it's important to find the right balance between the advanced technology and the human factors needed for effective decision-making and project completion.
Integrating AI technologies into organizations is proving to be more difficult than many initially thought. Research suggests a significant portion of organizations—over 60%—either delay or completely abandon AI projects because of the challenges in integrating them into existing systems and aligning them with internal operations. This finding reveals the complexities of smoothly incorporating new technologies into established workflows.
It's interesting to see that including a strong human element in AI projects significantly reduces failure rates. Organizations that prioritize human oversight in AI decision-making and processes experience a notable decrease in project failures (about 47% fewer failures). This implies that while AI excels at automating tasks, human judgment and intervention are crucial for ensuring optimal outcomes, especially in risk management and complex situations.
However, one of the biggest roadblocks seems to be a lack of expertise. Research has shown that only about 30% of project managers feel sufficiently equipped to handle the challenges of AI implementation. This substantial skills gap in managing AI projects suggests a critical need for increased training and development programs to bridge this divide and adequately prepare project management teams for the future of work.
Furthermore, the idea of implementing AI often meets resistance from employees. About 70% of employees are worried that AI will impact their jobs, leading to resistance that hinders successful implementation. This suggests that organizations need to implement robust change management programs that address anxieties and assure employees about the role of AI and the company's commitment to their well-being.
A concerning finding is that biases within the data used to train AI models can be amplified by the AI itself. In fact, a significant portion (up to 40%) of AI models don't sufficiently account for or address these biases in the data. This suggests a pressing need for stringent due diligence when selecting data sources for AI projects to prevent the propagation of unfair or discriminatory outcomes.
On the other hand, building a project team with people from diverse backgrounds and different disciplines appears to be a smart move. Organizations with interdisciplinary teams—teams comprised of various specialists—report a 25% increase in successful AI implementations. This finding highlights the value of diverse perspectives and problem-solving approaches in navigating the complexities of AI initiatives.
Keeping the project adaptable through regular feedback mechanisms seems to be important, too. Research suggests that ongoing feedback mechanisms increase project adaptability by about 50%. This adaptive ability enables teams to promptly adjust to changes in real-time data, highlighting the importance of building an environment that prioritizes continuous communication.
The organizational culture also appears to play a vital role in determining the success of AI initiatives. Organizations that have a culture focused on innovation and learning are able to adapt to the rapid changes of the AI landscape considerably faster than more rigid organizations (up to 60% faster). This implies that fostering a culture that encourages experimentation and learning can greatly enhance the adoption and effectiveness of AI projects.
One of the leading causes for AI project failure seems to be the lack of connection between short-term activities and the long-term goals of the organization. Roughly half of failed AI projects have this problem, highlighting the need for proper strategic planning and alignment with the overall objectives. Organizations that don't prioritize long-term strategic planning often struggle to achieve sustainable success with AI projects.
Finally, it's interesting that teams that spend roughly 30% of their time focusing on relationship-building activities and collaboration tend to be more successful than those that solely focus on technical aspects of the project. This points to the importance of nurturing soft skills like communication and collaboration to create a supportive and innovative project environment.
More Posts from aitutorialmaker.com: