Create AI-powered tutorials effortlessly: Learn, teach, and share knowledge with our intuitive platform. (Get started for free)
7 Critical Success Metrics for Technology Career Growth in 2025 Data-Driven Analysis
7 Critical Success Metrics for Technology Career Growth in 2025 Data-Driven Analysis - Quantifiable Skill Impact Through GitHub Contributions and Open Source Engagement Stats
In the evolving technology landscape, actively participating in open source projects, particularly through platforms like GitHub, provides a clear path to demonstrating tangible skill development and influence. GitHub's meticulous tracking of contributions, including both coding and non-code elements like documentation and support, offers a quantifiable way to evaluate individual impact within a project. This is increasingly important as open source communities become more diverse, requiring insights into how different groups and individuals contribute.
Tools designed to analyze GitHub activity provide a window into how collaborative environments function, revealing valuable information about contributor behavior and the overall health of a project. This level of analysis not only helps managers and project leads optimize open source initiatives but also allows developers to showcase their collaborative abilities, a skill becoming increasingly valued. The combination of quantifiable contributions, insights into collaboration dynamics, and practical problem-solving experience gained through open source participation has evolved into a crucial indicator for career advancement in the tech sector. This is because direct engagement within these ecosystems showcases both practical skillsets and a dedication to fostering a shared knowledge base.
GitHub's extensive logging capabilities offer a detailed picture of project activity and individual developer involvement. This includes tracking commits and other contributions, which could be useful in understanding how individuals contribute. However, the open-source landscape is evolving and encompasses far more than just coding. We're seeing a broader range of individuals contributing, particularly from educational, data science, and research backgrounds. It's worth noting that activities like documenting or providing support are equally important, even though they might not be directly related to writing code. Tools like the GitHub Contributors Action are being used by project maintainers to better grasp contributor activity and build stronger, more inclusive communities. This highlights the need for acknowledging the varied ways individuals can contribute. From a researcher's point of view, tools like GitHub Organization Analysis are intriguing, as they can help us better understand how various contributors interact and behave within a project. This information can be helpful for open source project management and development.
Beyond GitHub, we can track contributions across different platforms, like GitLab and Bitbucket. This gives us a more comprehensive picture of the contribution landscape. It seems encouraging to see initiatives like "good first issue" and "help wanted" labels, as they can help guide newcomers who want to get involved with a project. Understanding the dynamic nature of developer contributions over time is a crucial part of the research puzzle. By leveraging techniques like time-series analysis, we can uncover trends and patterns in these contributions. This can provide valuable insights into the challenges and opportunities within various open-source ecosystems.
The real-world problems tackled within open source offer developers the opportunity to hone their technical skills and learn how to collaborate effectively. While the concept of "rewarding" contributions is interesting, and we see initiatives like platforms using Open Collective to distribute funds, it's important to also explore the broader implications and effects of such efforts in shaping contributor motivations and behavior.
7 Critical Success Metrics for Technology Career Growth in 2025 Data-Driven Analysis - Cloud Platform Certifications ROI Analysis AWS Azure and GCP Career Advancement Data
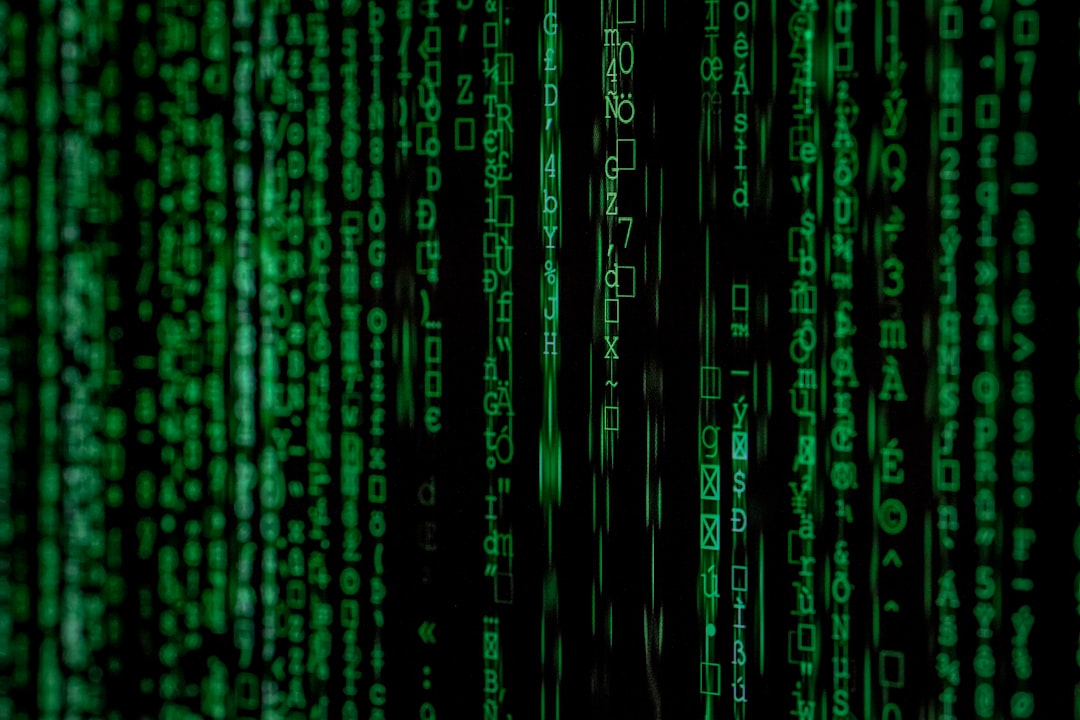
In the competitive landscape of cloud computing, certifications offered by AWS, Azure, and GCP have become significant markers for career progression. These certifications act as a demonstration of expertise in a rapidly evolving sector, potentially opening doors to better job opportunities. AWS currently leads the market with a substantial share, closely followed by Azure and GCP, and each platform has a unique set of certifications tailored to specific skillsets. For example, Azure certifications can be beneficial for individuals already working within Microsoft's technology ecosystem, potentially giving them a competitive advantage. On the other hand, GCP's certifications tend to lean towards specialized areas like data engineering and machine learning, capitalizing on Google's strengths in those domains.
However, achieving these certifications often requires significant time and effort, including hands-on experience and potentially extensive study. This substantial investment raises questions about the actual return on investment in terms of career advancement. Therefore, carefully evaluating one's career trajectory and interests is crucial before committing to a particular certification path. While certifications can help validate skills, the cloud computing world is consistently changing, requiring continuous learning and adaptability to stay relevant in the long run. The value of these certifications might diminish over time if one does not continue to acquire new skills and knowledge. Ultimately, the decision of which platform to pursue certification in should be guided by an individual's specific career goals and aspirations within the broader field of cloud computing.
Cloud certifications, particularly those from AWS, Azure, and GCP, are increasingly seen as valuable assets in the tech job market. While the industry is dominated by AWS (holding a 33% market share in 2023), Azure and GCP are also making significant strides, especially in specific areas. Azure seems to have an advantage for those already embedded in the Microsoft ecosystem, likely due to the integration points and familiarity with their tools. GCP, on the other hand, leans towards data engineering and machine learning, drawing strength from Google's work in these areas.
The path to certification isn't necessarily straightforward. For instance, the requirements for AWS are quite substantial, often requiring candidates to demonstrate years of experience in related fields. This suggests a level of maturity and complexity in the certification programs, with different platforms offering various tiers of certification. It's interesting how different platforms structure their certifications – AWS has "Specialty" certifications, while Azure emphasizes "Expert" levels, and GCP goes with "Professional" certifications. Each platform appears to be trying to communicate a specific level of competency.
In a constantly evolving field like cloud computing, continuous learning is critical for maintaining a competitive edge. However, the cost of entry can be a barrier for some, with AWS exams, for instance, costing around $150 and requiring several hours to complete. The sheer volume of questions can be daunting. Ultimately, the decision of which cloud certification to pursue should be aligned with individual goals and interests. Each platform has its own unique strengths and offers opportunities that might suit certain careers or skill sets more than others. The landscape is evolving rapidly, making it crucial to choose a path that aligns with long-term aspirations and the industry's trajectory.
7 Critical Success Metrics for Technology Career Growth in 2025 Data-Driven Analysis - Remote Work Project Management KPIs Meeting Deadlines and Team Collaboration Metrics
In the context of remote work, project management hinges on effectively managing deadlines and nurturing strong team collaboration. Metrics like consistently hitting deadlines, producing high-quality work, and adeptly using project management tools are crucial for understanding how remote teams function and how individual team members contribute. Remote environments can introduce unique challenges, but regular check-ins and robust communication can help bridge the distance and ensure that progress stays on track, allowing managers to promptly address roadblocks. A successful strategy blends both the results a team achieves and how their actions impact project outcomes, creating a well-rounded picture of success and keeping the entire team focused on the bigger goals. As the tech world changes, mastering these remote work project management skills will become even more essential for career success in 2025. While some might initially find remote team management difficult, trust and clear communication are crucial to building a high-performing, productive team.
Managing projects remotely presents a unique set of challenges, particularly when it comes to keeping teams aligned and on track. It's become clear that simply shifting to remote work doesn't automatically translate into success. We need a more systematic approach, and using key performance indicators (KPIs) seems like a good starting point. These KPIs should be focused on areas like hitting deadlines, the quality of work, and how well the team uses project management tools.
It's important to be realistic when setting KPIs. We need to make sure they're aligned with each team member's capabilities and the workload they have. A good mix of outcomes (what's being accomplished) and the impact (how it affects the project) helps us gauge success in a remote environment.
Tools like Asana, Trello, and Jira help teams stay organized, especially on larger projects. They provide a clear way to assign tasks, track deadlines, and provide updates. But simply having these tools isn't enough. The success of a remote team hinges on tangible results and everyone being on the same page with project progress.
Honestly, managers might find the transition to remote work a bit challenging initially. There are shifts in team dynamics to navigate. Effective communication and fostering trust become crucial to overcome these hurdles.
To truly manage a remote project team, it's essential to bring clarity to the goals, provide support, and incorporate data analysis into the process. We also need to prioritize effective time management. It appears that a good approach is to focus on metrics related to tech career growth in 2025, which aligns with the industry's demand for data-driven decision-making. This emphasizes the importance of adapting to remote work best practices.
It's interesting to note the increasing prominence of data analysis in project management. We can learn a lot about team dynamics and efficiency from analyzing the data we gather. Understanding how individuals work remotely and the patterns in their contributions can help us improve team management and project outcomes. In essence, we're trying to leverage data and insights to make remote work not just feasible, but also beneficial and effective.
7 Critical Success Metrics for Technology Career Growth in 2025 Data-Driven Analysis - Technical Leadership Impact Measured Through Team Performance and Project Delivery
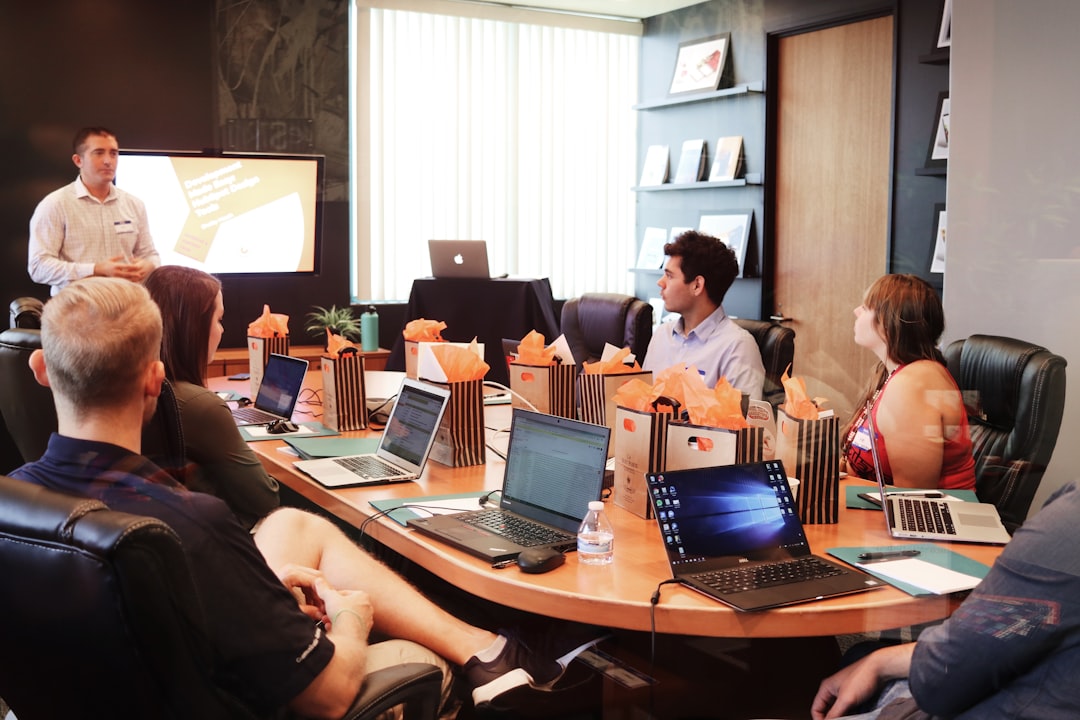
Technical leadership significantly impacts a team's ability to perform well and deliver projects successfully in the tech industry. Beyond simply achieving project goals, skilled technical leaders are focused on long-term value and making sure project objectives align with the overall business strategy. This includes creating a collaborative environment that encourages team members to leverage their diverse knowledge and expertise. Fostering trust and motivation within the team is a critical part of leadership, and leaders need to understand how individual team members contribute to the larger goals.
Using metrics like team velocity and the amount of work in progress (WIP) can give a much clearer picture of team dynamics and overall productivity. These insights are essential to improving team performance and driving project outcomes. As the tech world evolves rapidly, the best technical leaders are able to adapt and make adjustments. They are also likely to embrace new tools and data-driven approaches to enhance collaboration and refine project delivery processes over time.
Examining the impact of technical leadership through the lens of team performance and project delivery is a fascinating area of study. It's intuitive that strong leadership can influence team outcomes, but quantifying that impact is essential for understanding how to cultivate effective leaders within the tech industry, especially as the industry continues to evolve.
One area of interest is how leadership affects the team's overall velocity over time. It seems reasonable to assume that a cohesive and motivated team, fostered through strong technical leadership, would likely deliver work at a higher pace. However, merely looking at short-term output might not be sufficient. We need to consider the long-term implications of projects and how a team's work contributes to the larger goals of the organization. This requires a more holistic view of impact, evaluating both immediate and future consequences of decisions.
The makeup of a team plays a significant role in project success. A diverse group of individuals, with a blend of skills and experiences, can potentially bring more creativity and novel solutions to the table. But without strong leadership, this diversity might lead to disjointed efforts. Leadership qualities like communication, problem-solving, and decision-making are crucial for harnessing the potential of a diverse team. An effective technical leader can foster a collaborative environment where everyone feels heard and valued. This can improve team performance by building trust and ensuring open communication, leading to better decision-making.
It's insightful to consider how the technical leader prioritizes tasks. A good leader will likely base these decisions on the business value each task brings. Story points, often used in Agile environments, are a common way to gauge task complexity and estimate time. But we must be wary of using such metrics in isolation. Factors like risk and dependencies can significantly impact the prioritization process.
It's becoming increasingly common for organizations to leverage big data analytics for improved project performance. This ability to gather, analyze, and interpret data can give valuable insights into team dynamics and task management. For example, analyzing Work in Progress (WIP) can highlight potential bottlenecks or areas where the team might be spreading themselves too thin, leading to reduced focus.
Ultimately, project success starts with clear goals. A project leader must translate overarching business objectives into actionable steps. Defining metrics like task completion rates, customer satisfaction, and return on investment (ROI) can provide objective measures of project success. It's also important to remember that the definition of success can change over time, particularly as the goals of an organization evolve.
In conclusion, effective technical leadership plays a vital role in driving team performance and successful project delivery. While quantifying this impact is challenging, researchers and organizations are increasingly using data to understand the connection between leadership styles, team dynamics, and project outcomes. The ability to leverage data, combined with a strong understanding of leadership qualities, is crucial for maximizing the potential of both individuals and teams within the dynamic landscape of the technology industry.
7 Critical Success Metrics for Technology Career Growth in 2025 Data-Driven Analysis - Data Engineering and AI Implementation Success Rate in Production Environments
The success of integrating data engineering and AI into live systems heavily depends on how well data is managed and its quality. For AI models to perform accurately and minimize mistakes, data needs to be high quality and carefully prepared. If not, the accuracy of AI output takes a hit. Organizations must focus on finding dependable data sources and making sure the data they use is complete, in the right format, and representative of the people they want the AI to work with. Getting these data engineering and AI initiatives ready for actual use involves a lot of detailed work: testing, checking how they are doing, and keeping them running. This transition from initial development to full production is complex. As tech keeps changing, setting up clear ways to measure how well these AI projects are working becomes more important. This not only helps you get good results but also allows data engineering and AI professionals to adapt and grow in this constantly changing field.
The increasing reliance on AI in various sectors has amplified the need for robust data engineering practices. However, a significant portion of AI projects stumble in the transition to production environments, often failing to meet their intended goals. Research suggests that over 70% of these projects face this challenge, indicating a critical link between successful AI implementations and well-structured data engineering pipelines.
One contributing factor to this low success rate is a lack of effective collaboration between the individuals building the AI models (often data scientists) and those responsible for preparing and managing the data (data engineers). When these roles aren't well-integrated, data silos can form, leading to inefficient workflows and ultimately hindering progress.
Furthermore, the quality of the data used to train AI models is a primary determinant of their success. Studies show that a substantial portion—perhaps 60%—of AI project failures stem from poor data quality. Issues like inconsistencies, inaccuracies, and missing data can significantly degrade AI performance, highlighting the importance of robust data cleaning and validation processes.
Another hurdle is the time it takes to deploy AI models into production environments. In many cases, this process can extend beyond six months, emphasizing the need for efficient data engineering practices and approaches like agile development to streamline the journey from model development to deployment.
Beyond these practical challenges, there's a growing concern about the skills gap within the tech industry. Many data engineers, perhaps as many as half, are lacking essential cloud-based skills that are crucial for deploying and scaling AI models in modern cloud-native architectures.
The importance of continuous monitoring of AI model performance post-deployment is also frequently overlooked. Research indicates that a large majority of companies (around 80%) don't implement effective monitoring systems. This lack of monitoring can lead to declining performance over time and a decrease in the value of the data engineering efforts. Implementing a system of feedback and monitoring allows for better adjustments to be made over time.
AI model performance can also be affected by the underlying data architecture. Integrating data systems with AI models often leads to complex and sometimes inefficient architectures. Well-designed data workflows can significantly reduce these latencies, enhancing the responsiveness of AI solutions.
Moreover, data isn't static. It changes over time, and AI models need to adapt. The effectiveness of an AI model often degrades without periodic retraining. Companies that have successfully implemented scheduled retraining cycles have reported noticeable improvements in AI performance.
Finally, a significant issue is the presence of biases in the training data used to develop AI models. Data engineers need to develop rigorous checks and sourcing strategies to mitigate the risks of skewed AI outcomes caused by biases that might be present in the original data sets. It is estimated that almost 70% of AI algorithms face this challenge.
These challenges and their impact on AI project success highlight the ongoing need for ongoing research into data engineering best practices and strategies that support robust AI implementation in production environments. As we see the growing application of AI in various sectors, the role of the data engineer in ensuring a successful transition to a production environment will continue to grow in importance.
7 Critical Success Metrics for Technology Career Growth in 2025 Data-Driven Analysis - Continuous Learning Progress Tracking via Online Course Completion and Knowledge Application
In the rapidly changing tech world, continuous learning is essential for staying relevant. Online courses are increasingly common, making it crucial to track not just completion but also how well learners apply what they've learned. By tracking course completion rates, we can gain a sense of learner commitment and how effectively courses are designed and delivered. However, simply finishing a course isn't enough. We need to see if the knowledge gained translates to practical use. This is where real-time data analysis becomes valuable, providing insights into how effectively individuals are applying their newly acquired skills. Cultivating a culture that values continuous learning and emphasizes knowledge application is likely beneficial for both individual growth and overall organizational performance within the tech sector. While individual motivation plays a role, providing feedback loops and data to individuals to understand their progression is an important step. There are also concerns over gamification and whether pushing completion rates might influence the quality of understanding and application of the concepts.
Continuous learning is becoming increasingly important in the ever-evolving tech landscape, and tracking progress is key to understanding its impact on career growth. We can gain insights into the effectiveness of learning initiatives by tracking online course completion rates and observing how learners apply their new knowledge in real-world situations.
One interesting observation is that learners often report increased confidence in their abilities after finishing an online course. This confidence boost seems to translate to improved job performance, suggesting that continuous learning can have a direct impact on one's career. While it's based on self-reported data, it is a starting point for assessing the effectiveness of these programs.
Course completion rates serve as a valuable metric to gauge learner commitment and the efficacy of a course's design and delivery. It seems individuals who finish their courses are more likely to receive promotions within a year, which hints at a correlation between dedication to learning and career advancement. We need to further explore the causality here, as this kind of data is prone to bias and correlation is not causation.
Beyond completion rates, analyzing knowledge application is crucial. Evidence shows that putting newly acquired skills into practice on the job significantly impacts knowledge retention and adaptability. When learners use their skills in real-world scenarios, knowledge retention appears to improve considerably, emphasizing the importance of experiential learning and bridging the gap between classroom learning and application.
The rise of Learning Management Systems (LMSs) in corporations suggests a shift towards data-driven approaches to employee development. Companies are adopting these systems to monitor progress, track completion rates, and potentially make adjustments to their training programs. The gamification elements embedded in many of these courses also seem to be helping boost completion rates, indicating the positive impact of making learning more engaging.
Interestingly, organizations using progress tracking report an increase in employee retention. This is likely due to the fact that employees feel more invested in their own development when they can see evidence of their progress. The ability to personalize courses through real-time analytics further enhances the learning experience, resulting in improved relevance and faster skill acquisition.
Continuous learning appears to boost an employee's ability to adapt to change within the workplace. This adaptability is vital in a field where technology evolves quickly. It suggests that a culture of continuous learning can lead to a more adaptable and resilient workforce. Moreover, there's evidence that such initiatives can provide a significant return on investment for companies, particularly in the tech sector. This makes a strong argument for prioritization of such initiatives.
However, there appears to be a disconnect between employee expectations and what employers are providing. It seems that a considerable portion of tech professionals would like employers to provide clearer pathways for tracking skill development. This gap suggests a need for employers to rethink their approach to continuous learning and provide a more structured and transparent experience for their workforce.
In conclusion, continuous learning, tracked via online course completion and knowledge application, presents a powerful tool for career development in the tech industry. While the data and research show potential, a more nuanced understanding of the complexities is required. By tracking progress, understanding knowledge application, and fostering a learning culture, organizations can empower their workforce and improve their ability to compete in the rapidly evolving tech world.
7 Critical Success Metrics for Technology Career Growth in 2025 Data-Driven Analysis - Industry Networking Impact Analysis Through Conference Participation and Knowledge Sharing
Within the dynamic landscape of technology careers, building a strong professional network through conference participation and knowledge sharing is increasingly vital for career growth. Attending these events offers opportunities to connect with peers, exchange ideas, and collaborate on innovative projects. While the act of attending a conference might appear simple, a comprehensive evaluation of its impact requires considering both the readily measurable benefits like increased revenue and the less quantifiable advantages such as building new relationships. Furthermore, assessing the effectiveness of conferences necessitates the use of meaningful metrics, encompassing attendee feedback, levels of engagement, the depth of knowledge acquired, and the ability to apply learned information in real-world scenarios. Given the increasing interconnectedness of industries, like those focused on semiconductors and pharmaceutical advancements, the deliberate exchange of knowledge through collaboration emerges as a critical factor in maintaining a competitive edge and driving productivity across a range of fields. It's also important to note that evaluating knowledge sharing on its own is insufficient. Instead, it needs to be linked to broader outcomes and long-term impacts to truly understand its value.
The exchange of information is crucial for propelling technological progress in key industries by fostering resource sharing and collaborative invention. While the concept of knowledge sharing is well-established, there's a lack of thorough research into how it's actually applied and what effects it has on innovation in practice.
We can measure how effective attending events like conferences is by looking at the Return on Investment (ROI). ROI considers both things like increased revenue and harder-to-measure benefits like networking.
Sharing knowledge within strategic partnerships focused on innovation in industrial technology is essential for stimulating collaborative innovation and improving overall performance. The pharmaceutical field illustrates the importance of knowledge sharing as a way to accelerate innovation, particularly in dealing with global challenges such as the COVID-19 pandemic.
Looking at statistical data shows that virtual events have had a major effect on the event industry, changing how events are planned and run. We can gauge how much impact a conference has on attendees by using metrics like feedback from attendees, how engaged they are, what they learned, and whether it facilitated sharing of knowledge.
Industries like the semiconductor sector, which are becoming more globalized, show a trend toward companies working together more often to do complex research on technology. We can use event data to understand trends and how events are doing overall, looking at things like how satisfied and involved participants are.
Organizations that prioritize knowledge sharing strategically can improve their competitive position and overall performance across various industries. This shows us that organizations need to make knowledge sharing a priority. It's interesting to observe how conference participation helps with networking, leading to new professional contacts and learning from others. It would be valuable to delve deeper into what types of conferences and knowledge sharing patterns lead to better career outcomes. This would help us gain a better understanding of which conferences or networks would be most impactful for different individuals.
Create AI-powered tutorials effortlessly: Learn, teach, and share knowledge with our intuitive platform. (Get started for free)
More Posts from aitutorialmaker.com: