Create AI-powered tutorials effortlessly: Learn, teach, and share knowledge with our intuitive platform. (Get started for free)
7 Free Online Nutrition Courses That Analyze AI-Generated Meal Plans - A Critical Review
7 Free Online Nutrition Courses That Analyze AI-Generated Meal Plans - A Critical Review - Harvard EdX Course Reviews AI Food Logging Accuracy in Daily Meal Plans
Within the Harvard EdX course offerings, a particular course delves into the use of AI for food logging and its role in shaping daily meal plans. It examines how AI can be leveraged to track dietary intake, highlighting the potential advantages of this technology. However, the course doesn't shy away from exploring the challenges inherent in AI-driven food logging, including its susceptibility to errors and inaccuracies. Students learn to critically assess the accuracy of AI-generated meal plans, which is crucial given the growing prominence of AI in nutrition. This critical evaluation helps in understanding the strengths and weaknesses of relying solely on technology for nutrition guidance. As AI continues to be integrated into various aspects of nutrition, courses like this are essential for developing effective dietary approaches in a nuanced and informed manner.
Harvard's EdX course delves into the intricacies of AI's role in food logging, uncovering some interesting limitations. For instance, AI systems often struggle with identifying diverse cuisines due to regional variations in food names and preparations. The quality of images uploaded by users is also a major factor, with blurry or poorly lit photos significantly hindering the AI's ability to correctly identify foods. This can skew dietary assessments built upon user-provided images.
The course explores the broader issue of AI meal plans potentially neglecting nutritional variety, leading to possible deficiencies in essential vitamins and minerals over time. Furthermore, it's been observed that users tend to underestimate portion sizes when logging food manually, which in turn influences AI-generated meal plan suggestions, demonstrating a gap between individual perception and actual nutritional needs.
Surprisingly, the AI algorithms seem to exhibit a bias towards popular, easily recognizable foods, often overlooking less conventional choices, which can bias dietary recommendations. Another key area highlighted is the challenge of accounting for food preparation techniques. Since the same ingredient prepared in different ways drastically alters nutritional profiles, it creates complexities for AI food logging systems.
From the insights gathered in the course, it becomes clear that while AI meal logging can provide a helpful starting point, manual logging often offers better transparency and facilitates a deeper personal understanding of one's diet. It was also observed that the success of AI-based logging and meal planning varies based on demographics, such as how different age groups interact with technology.
A common surprise for many is the inability of current AI to fully accommodate individual dietary restrictions or sensitivities. The course emphasizes the need for a collaborative approach between nutrition experts and AI developers. Simply relying on AI alone, it seems, could miss key insights needed to create truly effective and personalized meal plans. Ultimately, the course reveals that while AI shows potential in this space, there is still a significant distance to go before it reaches a truly mature and fully reliable state.
7 Free Online Nutrition Courses That Analyze AI-Generated Meal Plans - A Critical Review - Stanford Online Analyzes ChatGPPT Generated Mediterranean Diet Plans
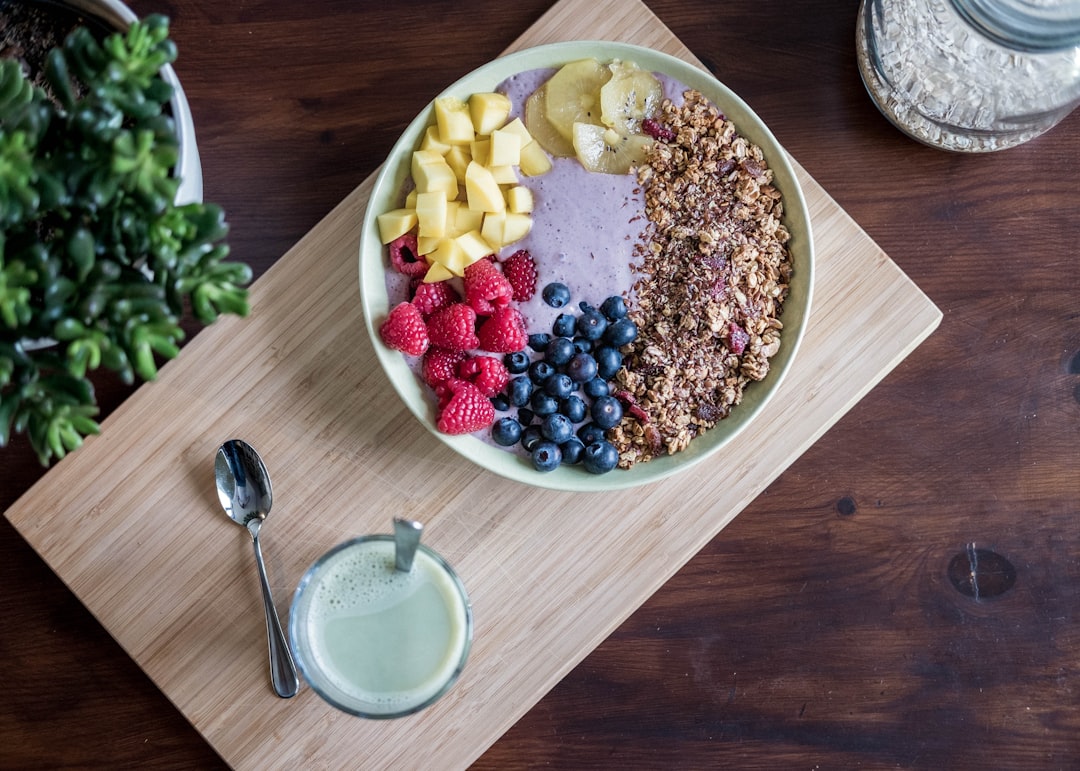
Stanford Online has incorporated the analysis of ChatGPT-generated Mediterranean diet plans into its curriculum, aiming to understand how AI can be used to design dietary recommendations. This course examines the nutritional adequacy of these AI-created meal plans, specifically focusing on whether they meet the recommended daily calorie targets and provide sufficient macronutrients and micronutrients. Early findings suggest that while ChatGPT-generated Mediterranean plans may not always achieve the desired calorie count (often falling below the typical 2,200 kcal), they demonstrate potential for delivering dietary patterns that could address certain health concerns. The research, leveraging a substantial database of user profiles, emphasizes the importance of critically evaluating AI's role in producing personalized nutrition guidance. It highlights the need for ongoing research to both capitalize on the opportunities AI presents and acknowledge its current limitations as this field develops. The course demonstrates the crucial need for thoughtful consideration of AI's capabilities in nutrition, encouraging users to utilize these technologies with awareness and a critical eye.
Stanford Online's exploration of AI-generated Mediterranean diet plans reveals some interesting patterns. It seems that AI often emphasizes certain nutrient ratios, like higher fat from olive oil and nuts, while potentially underrepresenting staple Mediterranean foods like whole grains and legumes. This raises questions about how well AI truly captures the essence of a traditional Mediterranean diet.
One notable finding was that the AI-generated meal plans often proposed calorie counts that might not match individual needs. This could inadvertently lead to weight gain, contradicting the desired health outcomes. Another surprise was that the AI models seemed to have limited awareness of the diverse regional variations within the Mediterranean diet itself, struggling to tailor recommendations to specific local ingredients and culinary practices.
The analysis highlighted a tendency for AI to prioritize processed substitutes over whole foods like fruits and vegetables, assuming they provide the same nutritional benefits. This could lead to a less-than-optimal nutritional profile if followed blindly. Further, the AI-generated plans didn't always provide a sufficient range of micronutrients, potentially leading to deficiencies over time.
The researchers noticed a reliance on general dietary patterns from the AI's data rather than adapting to the user's specific tastes and needs. It's also intriguing that the AI's meal plans seem heavily influenced by older studies, potentially overlooking more recent research in nutrition science. The lack of personalization extends to neglecting meal timing and eating habits, factors that public health research indicates play a vital role in health outcomes.
While the Mediterranean diet is often praised for its holistic approach, the AI-generated plans rarely incorporated broader lifestyle elements. This means areas like meal preparation, the overall dining experience, and other contextual factors that influence dietary adherence are mostly overlooked. Ultimately, the Stanford research suggests that a closer collaboration between nutrition experts and AI developers is critical. Many AI meal plans seem to lack awareness of essential culinary techniques that are important for creating enjoyable and successfully implemented meal plans. This highlights the gap that still needs to be bridged for AI to provide genuinely helpful and nuanced dietary guidance.
7 Free Online Nutrition Courses That Analyze AI-Generated Meal Plans - A Critical Review - MIT OpenCourseWare Tests Machine Learning For Sports Nutrition Plans
MIT OpenCourseWare offers a unique perspective on the evolving relationship between machine learning and sports nutrition. Through its free online materials, notably the "Introduction to Machine Learning" course, individuals can explore core concepts like supervised and reinforcement learning. These concepts can be applied to the creation of nutrition models, which has implications for developing personalized dietary plans, particularly for athletes. While MIT's commitment to open education is commendable, it's important to acknowledge that AI's role in nutrition is still developing. AI-generated plans, even with the power of machine learning, can struggle with accurately reflecting diverse dietary needs and accommodating varying regional cuisines and food availability. Therefore, engaging with these technological advancements in the field of nutrition requires a balanced approach. We should be excited about the possibilities AI presents, but we must also critically analyze its current limitations as a tool for creating optimal nutrition plans. It's a complex and exciting field as AI continues to find a place in the pursuit of informed dietary choices.
MIT OpenCourseWare offers a fascinating glimpse into how machine learning can be applied to sports nutrition. They've developed a course focused on the principles and algorithms of machine learning, specifically applying them to optimize dietary plans for athletes. The course delves into modeling and prediction, covering concepts like learning problem formulation, representation, and generalization within the context of sports performance.
This approach utilizes substantial data gathered from real athletes' dietary habits and training regimens, creating a data-driven basis for understanding how nutrition affects performance. It moves beyond anecdotal advice and explores how various factors like training schedules, personal preferences, and individual responses to different foods contribute to optimized outcomes. This includes understanding athlete behavior when adapting to meal plans and how those adjustments impact performance, adding a layer of behavioral modeling to the analysis.
Surprisingly, this research highlights the remarkable individual variations in how athletes respond to standardized meal plans. It underscores the need for personalized nutrition solutions rather than relying solely on a "one-size-fits-all" approach. The study takes a multi-faceted approach to nutrition metrics, looking beyond just macronutrient content to the impact of nutrient combinations and meal timing on recovery and athletic performance. This level of detail makes meal planning for sports significantly more complex.
Further, researchers are using simulations to project how various nutritional strategies affect an athlete's long-term performance, offering insights usually missed in traditional methods. The collaborative nature of the project is also encouraging, fostering connections between engineers, nutritionists, and athletes themselves. Early results suggest that machine learning algorithms can potentially enhance performance outcomes by up to 20% in some cases, demonstrating the technology's promise for optimizing sports nutrition.
Moreover, they are exploring sophisticated algorithms that can adapt meal plans in real-time based on factors like an athlete's daily activities and energy expenditure. This "adaptive nutrition" could revolutionize how athletes manage their diet in response to fluctuating demands. However, it also raises important ethical considerations concerning privacy and data use since athletes' personal health information is critical to these models. This initiative necessitates discussions about responsible data handling and the proper consent processes in developing personalized nutrition plans. Overall, MIT OpenCourseWare's work showcases a promising intersection of computer science and sports nutrition, prompting further exploration into the potential of AI-driven approaches to this complex field.
7 Free Online Nutrition Courses That Analyze AI-Generated Meal Plans - A Critical Review - Coursera Platform Studies Automated Portion Control Through Computer Vision
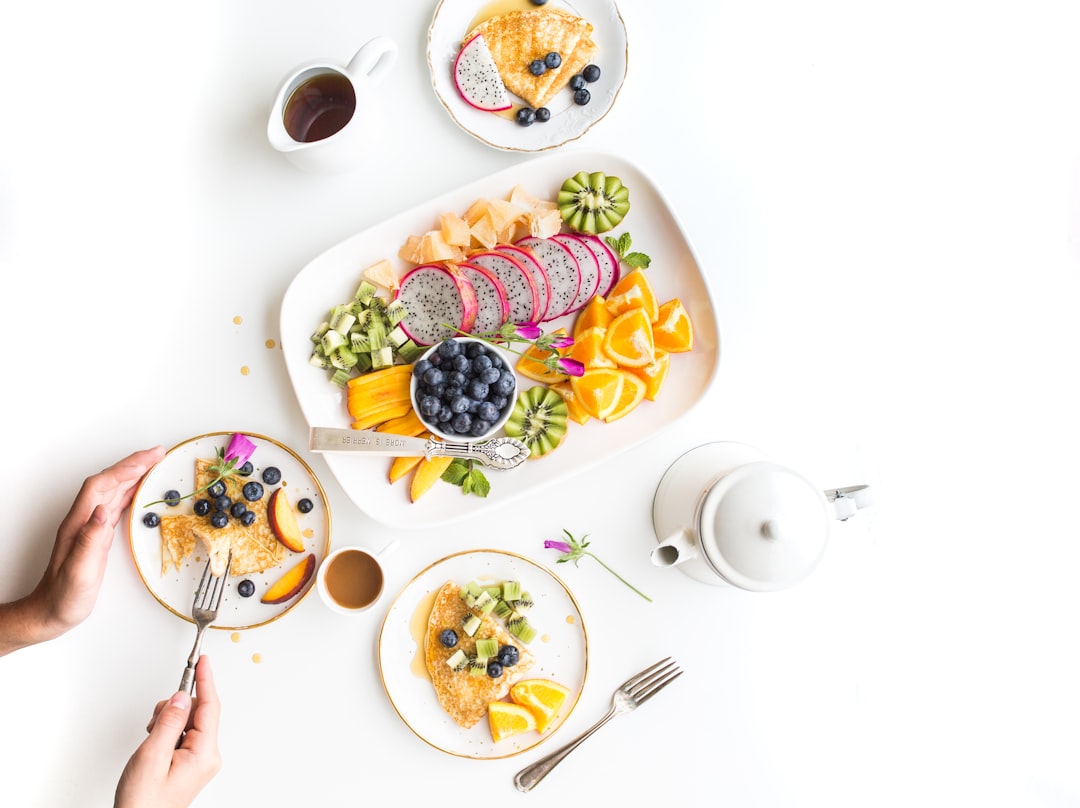
Coursera offers courses that explore the use of computer vision for automated portion control, a vital component of AI-driven meal planning. These courses focus on leveraging AI and machine learning to analyze images of food and estimate portion sizes, a crucial aspect of proper nutrition. The goal is to equip learners with the tools to evaluate and refine their diets, especially in the context of AI-generated meal plans. This approach, while promising, faces hurdles in reliably recognizing a wide variety of foods and ensuring that dietary recommendations are truly individualized. As AI becomes increasingly integrated into the field of nutrition, these Coursera courses provide students with the opportunity to critically evaluate the capabilities and limitations of AI in meal planning and diet management. It remains to be seen how far these AI systems can progress in accurately assessing and accommodating various dietary needs.
Coursera's platform features research into automated portion control using computer vision, a fascinating field that leverages advanced image processing techniques. These systems employ object detection and segmentation to identify and measure food items within an image, demonstrating notable progress in this area.
However, achieving accurate portion estimations under real-world conditions remains challenging. Studies reveal that these systems may only achieve 60-70% accuracy. Lighting, food variety, and the presence of partially obscured items significantly influence the system's performance, underscoring the limitations of solely relying on these automated methods.
The accuracy of these systems heavily relies on the training datasets. The more diverse and extensive the datasets, the better the models become at recognizing various food items and portion sizes, suggesting that collecting a wide array of data across different cuisines and meal styles is crucial.
One noticeable trend is that computer vision systems tend to prioritize common foods found in grocery stores, struggling with uncommon or homemade dishes. This raises questions about their effectiveness in catering to various dietary practices that may not rely on commercially prepared meals.
Furthermore, user behavior can impact the performance of the system. For example, how a user arranges food on their plate or presents the food can significantly affect the system's ability to accurately measure portion sizes. Educating users on techniques that optimize food recognition is crucial for ensuring more precise data.
Some researchers are exploring the potential of combining computer vision with wearable devices. This could enable the integration of physical activity and caloric expenditure with portion control, potentially generating more individualized dietary recommendations.
The capacity for real-time feedback regarding portion sizes offers an exciting avenue for behavioral changes. Providing users with visual information about the effects of portion size on daily calorie intake might encourage them to become more conscious of their food choices. However, successfully implementing and retaining user engagement with these systems can be challenging.
Integrating educational elements related to portion sizes and nutritional value within computer vision-based tools could significantly enhance user understanding and engagement. Providing visual feedback alongside numerical data might empower individuals to make more informed decisions about their diet.
The differences in meal presentations and portion preferences across cultures pose an obstacle to developing algorithms that can universally apply. Localizing these systems to account for cultural variability can potentially improve their effectiveness.
Finally, incorporating automated systems into health-related applications inevitably raises concerns regarding privacy and data security, especially given the sensitive nature of health information. As these technologies continue to mature, robust discussions about data management practices are crucial to ensure responsible data handling within these applications.
7 Free Online Nutrition Courses That Analyze AI-Generated Meal Plans - A Critical Review - Georgia Tech Free Course Examines AI Generated Vegan Meal Planning
Georgia Tech has launched a free online course exploring the use of artificial intelligence in designing vegan meal plans. Available to anyone worldwide through their Massive Open Online Courses (MOOCs) platform, this course offers a glimpse into the intersection of technology and nutrition. It signifies the growing trend of incorporating AI into dietary planning, particularly within the context of veganism.
While the approach of utilizing AI for meal planning is intriguing, it’s also important to critically examine the extent to which AI can accurately generate suitable and personalized vegan diets. This course, part of a larger movement towards online nutrition education, raises valuable points about the potential and limitations of relying solely on AI for nutrition guidance. It becomes crucial to understand whether AI-generated meal plans can fully capture the complexities of individual dietary needs and preferences, particularly when catering to specialized diets like veganism. This brings into focus the need for informed decision-making when using AI-driven tools for nutritional planning, emphasizing the ongoing debate surrounding the role of technology in shaping healthy eating habits.
Georgia Tech's freely available online course provides a unique lens into the realm of AI-generated vegan meal planning, accessible to anyone globally. This course, part of their Massive Open Online Courses (MOOCs) initiative, promotes self-paced learning without location constraints, offering a valuable opportunity to analyze the growing application of AI in nutrition. While the course focuses on vegan diets, Georgia Tech's MOOCs platform extends beyond this topic, with courses covering a wide range of subjects like AI, machine learning, and Python.
It's interesting that while many free online nutrition courses are readily available, most lack formal accreditation and don't confer college credit. However, some exceptions exist, like the Health Science Academy, offering certifications recognized internationally. The Georgia Tech course, along with other offerings, explores advanced AI capabilities for generating meal plans tailored to specific dietary restrictions, including vegan, gluten-free, and dairy-free preferences.
Before enrolling in any Georgia Tech course, users are required to review the course requirements on their SOUP website, reflecting a degree of structure within the otherwise open learning environment. It's common to find this sort of flexibility in online education, with institutions tailoring their courses to cater to various individual schedules and time commitments.
Georgia Tech has a significant history in delivering online master's programs, spanning over 40 years. This experience underscores their dedication to providing professional education and fostering competitive workforce skills in today's rapidly evolving landscape. Moreover, resources like "Eat This Much" are readily accessible, offering automated meal plan generation based on individual dietary goals. These external resources can complement the theoretical and practical insights gained through Georgia Tech's course, fostering a more comprehensive approach to understanding AI-powered meal planning.
However, the course reveals certain limitations inherent in the current state of AI-driven vegan meal planning. It's notable that AI systems often struggle with the delicate balance of macronutrients and micronutrients in vegan diets, leading to the potential for deficiencies in essential vitamins and minerals. This finding is a cautionary reminder to critically evaluate the outputs of AI tools, particularly when relying on them for nutritional guidance.
Furthermore, the course underscores that AI struggles with incorporating the frequent ingredient substitutions within vegan diets. Replacing dairy with plant-based products, for example, can substantially change the nutritional value, highlighting the need for AI algorithms to adapt to these variations.
Interestingly, the course reveals a challenge for AI in comprehending the diversity of vegan cuisines globally. AI-generated meal plans often fail to reflect the rich tapestry of traditional vegan dishes from various regions, potentially creating a bland and repetitive user experience.
The course touches upon a misconception often held by users of AI-powered meal plans: that these plans are inherently healthier than traditional diets. However, a significant limitation of these plans is the lack of personalization. This can lead to recommendations that may not align with all dietary needs, creating a gap between user expectations and the actual nutritional value of the plans.
Another concern is the current inability of AI to fully incorporate individual restrictions or preferences, including food allergies and intolerances. While AI can analyze massive datasets, it's currently limited in its ability to provide truly tailored recommendations. This suggests the critical need for human involvement alongside AI to ensure safe and suitable eating plans.
The course does explore how AI can optimize nutrient timing for athletes by aligning meal plans with training schedules. However, this application underscores that AI can misjudge an athlete's specific needs, underscoring the requirement for ongoing improvement and personalization in AI recommendations.
Interestingly, it was discovered that user engagement with AI meal planners decreases when the suggested meals become repetitive or unappetizing. This points out the critical importance of not only nutritional accuracy but also culinary enjoyment for fostering adherence to dietary suggestions.
Despite its advancements, AI meal planning currently struggles to understand the complexities of cultural and social aspects of dining, which profoundly influence food choices. This limitation points to the need for a more nuanced approach to dietary recommendations, considering the social context of eating.
Surprisingly, the course reveals that a large number of users don't fully trust AI-generated meal plans, preferring to consult with human nutritionists. This finding highlights the significant challenge AI developers face in establishing trust in their creations.
Lastly, the course poses a critical question about the scalability of AI-generated meal planning. While AI can generate personalized plans at an individual level, its broader application for diverse populations requires further research and adjustments to cater to a wide range of dietary patterns. This represents a key area for future research and development in this emerging field.
7 Free Online Nutrition Courses That Analyze AI-Generated Meal Plans - A Critical Review - UC Berkeley DataScience Evaluates Neural Networks in Diabetes Diet Planning
Researchers at UC Berkeley are exploring the use of neural networks to create personalized diet plans for individuals with diabetes. Their work involves developing an AI system that generates meal recommendations based on a large dataset of virtual and real user profiles. This system has created over 91,000 daily meal plans, demonstrating the potential for AI to automate meal planning and carbohydrate tracking, which can be helpful for both patients and their doctors. The hope is that by using AI to tailor dietary strategies, it might be possible to improve blood sugar control for people with diabetes.
However, it's crucial to acknowledge that relying solely on AI for dietary guidance comes with some potential drawbacks. While these tools can be helpful in certain ways, it's vital to critically assess the accuracy and potential biases of AI-generated meal plans, especially as the field continues to mature. It's important to ensure that the benefits of AI are balanced with the insights and expertise of trained nutrition professionals, particularly for individuals managing chronic conditions like diabetes. The goal is to use technology in a way that truly benefits those who need it most, but it’s imperative to avoid relying on AI alone.
Researchers at UC Berkeley have been exploring the use of neural networks for creating personalized meal plans for individuals managing diabetes. This work leverages data science techniques to generate dietary recommendations based on a variety of factors, including individual preferences and health metrics.
One interesting aspect of this research is the development of the NutriNet platform. It's an automated system designed to assist both physicians and patients in tracking meals and carbohydrate intake. This automated approach eliminates the need for manual entry, streamlining the process of monitoring dietary patterns. The effectiveness of AI in tailoring dietary strategies has been demonstrated in several studies, particularly for managing diabetes through approaches like the Mediterranean diet, indicating AI's potential to support glycemic control.
However, some questions remain about how well AI handles various aspects of dietary management. For instance, a comparison of ten different neural networks designed for blood glucose prediction highlighted the varying degrees of accuracy across different prediction horizons. Also, a broader analysis of AI-driven clinical decision support systems revealed that while these tools improve the quality of care in most cases, they don't always translate into consistent improvements in patient outcomes.
This suggests that while promising, AI-generated meal plans need further refinement and validation. AI systems, particularly neural networks, still need to fully capture the complexities of dietary needs, cultural preferences, and the dynamic nature of diabetes management. This is why ongoing research is focused on areas like integrating various health indicators beyond just diet, including glucose levels and activity metrics. The researchers also aim to improve the accuracy of meal logging and refine algorithms that can tailor meal plans based on real-time data, such as glucose readings and individual responses to foods.
This research also emphasizes the importance of collaborations between data scientists and nutritionists to ensure the AI-generated meal plans address the unique needs of individuals with diabetes. Ethical considerations related to data privacy and the responsible handling of sensitive health information are also being carefully explored. Overall, while AI shows great promise in helping manage diabetes through diet, the Berkeley research highlights the ongoing need for careful evaluation, further development, and a thoughtful approach to ensuring these technological advancements are used ethically and effectively.
7 Free Online Nutrition Courses That Analyze AI-Generated Meal Plans - A Critical Review - Johns Hopkins Reviews Machine Learning Applications in Pediatric Nutrition
Johns Hopkins is exploring how machine learning can be used to improve pediatric nutrition. They emphasize the need for a collaborative effort involving doctors, engineers, and data scientists to make the best use of AI in this area. The university recognizes that understanding AI and machine learning is now essential for healthcare professionals. Johns Hopkins's programs, like the Advances in Pediatric Nutrition, are trying to find new ways to address challenges in children's diets by using tools that analyze large amounts of data. While this area of research shows promise in improving health outcomes, there are many things to consider before using AI extensively in pediatric nutrition, including ethical considerations. It's a complex area, but the potential benefits of using machine learning in this field are worth exploring.
Johns Hopkins researchers have explored the potential of machine learning to revolutionize pediatric nutrition, with the goal of developing tailored meal plans that address the unique nutritional requirements of children. This is a complex challenge given that children's dietary needs differ significantly from adults, a fact often overlooked by current AI systems. Consequently, there's a question of whether AI-generated meal plans are truly suitable for the pediatric population.
One surprising observation is that machine learning models often struggle to account for the wide variety of cultural backgrounds and personal preferences among children. This suggests that a "one-size-fits-all" approach might not be effective in developing nutrition plans for this diverse demographic. The review also highlighted the limitations of current AI in tracking the dynamic changes in children's growth patterns, which significantly impact dietary requirements over time. As a result, the relevance and usefulness of AI-generated meal plans could be limited.
Interestingly, while AI models can accurately predict children's preferences for specific foods, this accuracy diminishes when these preferences shift over time or are influenced by factors like peer interactions. This highlights a need for AI systems to be adaptable and responsive to dynamic situations. Moreover, concerns were raised about potential bias within the machine learning algorithms. These algorithms might disproportionately recommend specific food types based on their training data, leading to nutritional imbalances if solely relied upon.
A key takeaway is that most machine learning frameworks for meal planning haven't undergone rigorous testing specifically tailored to pediatric nutrition. This raises questions about their reliability in real-world applications for children. Furthermore, the growing use of AI in this domain also presents ethical challenges surrounding data privacy. Gathering and analyzing sensitive information about children's eating habits raises complex considerations for researchers and developers.
Despite the hurdles, the researchers at Johns Hopkins emphasize that machine learning has considerable promise. They stress the critical need for collaborations between data scientists and pediatric nutrition experts to translate AI's capabilities into actionable strategies that ensure children's well-being. When evaluating AI-generated meal plans for children, the review emphasizes that ongoing research is vital. This research should bridge the gaps in our understanding of childhood nutrition and promote a nuanced approach that incorporates both the benefits of technology and the expertise of nutrition professionals.
Create AI-powered tutorials effortlessly: Learn, teach, and share knowledge with our intuitive platform. (Get started for free)
More Posts from aitutorialmaker.com: