Create AI-powered tutorials effortlessly: Learn, teach, and share knowledge with our intuitive platform. (Get started for free)
7 Key AI-Driven Innovations Reshaping Financial Analysis Training in 2024
7 Key AI-Driven Innovations Reshaping Financial Analysis Training in 2024 - Natural Language Processing for Real-Time Market Sentiment Analysis
Natural Language Processing (NLP) has become increasingly important for understanding market sentiment in real-time. By analyzing the massive amounts of text data found in social media, news articles, and financial reports, NLP helps us grasp the overall mood and opinions surrounding financial markets. NLP algorithms can quickly categorize these opinions as positive, negative, or neutral, offering a valuable tool for financial professionals.
This ability to quickly assess public sentiment not only enhances decision-making but also guides strategic actions within financial markets. Recent improvements in NLP have dramatically improved the speed and accuracy of sentiment analysis. This enables organizations to process huge volumes of user-generated content and extract valuable insights more efficiently. Looking forward, deep learning techniques hold the promise of making sentiment analysis even more refined, leading to even deeper insights and potentially reshaping how financial analysis is conducted. However, the challenges of managing and interpreting vast amounts of textual data still persist, and careful consideration is needed to avoid misinterpretations or biases in the data.
Analyzing market sentiment in real-time using Natural Language Processing (NLP) allows us to sift through massive volumes of data – social media feeds, news articles, financial reports – at incredible speeds. This capability is crucial for traders who need to respond swiftly to market fluctuations.
While not perfect, sentiment analysis, when combined with carefully trained machine learning models, can achieve accuracy rates of 80-90% when gauging sentiment from financial data. This makes it a valuable tool for anticipating market direction.
NLP's ability to identify specific entities within text – like companies, sectors, or economic indicators – using techniques like entity recognition, provides detailed insights that weren't easily accessible before. This offers unprecedented granularity for financial analysis.
The use of sentiment analysis in finance helps refine data by separating out noise and highlighting statistically relevant signals for investment strategies. It's like cleaning up clutter to find the most important information.
Going beyond basic positive, negative, and neutral sentiment classifications, algorithms can also capture the intensity or strength of expressed opinions. This insight into the passion behind a sentiment is critical for predicting market volatility.
We're seeing an increasing adoption of sentiment analysis among high-frequency trading firms. They leverage it to exploit short-lived market opportunities that stem from quick shifts in public perception, turning those fluctuations into potential gains.
The recent development of transformer-based models has significantly improved sentiment analysis's understanding of subtle linguistic cues, such as sarcasm or ambiguous phrasing, which are quite common in financial conversations. This helps to make the analysis more nuanced and accurate.
Through NLP's integration, sentiment analysis generates sentiment scores, which can potentially serve as early warning signals, potentially forecasting market changes before they show up in standard financial metrics. This ability to potentially predict future trends is intriguing.
Platforms like Reddit and Twitter are now significant sources for sentiment analysis due to their direct reflection of retail investor discussions. These platforms can influence stock prices almost immediately, highlighting a shift in how market sentiment is formed and propagated.
While impressive, NLP still has limitations in correctly interpreting sentiment, especially when encountering industry-specific jargon or new terms. Consequently, frequent model retraining is needed to keep pace with evolving financial language and ensure the models remain accurate and effective.
7 Key AI-Driven Innovations Reshaping Financial Analysis Training in 2024 - Automated Risk Assessment Models Using Machine Learning
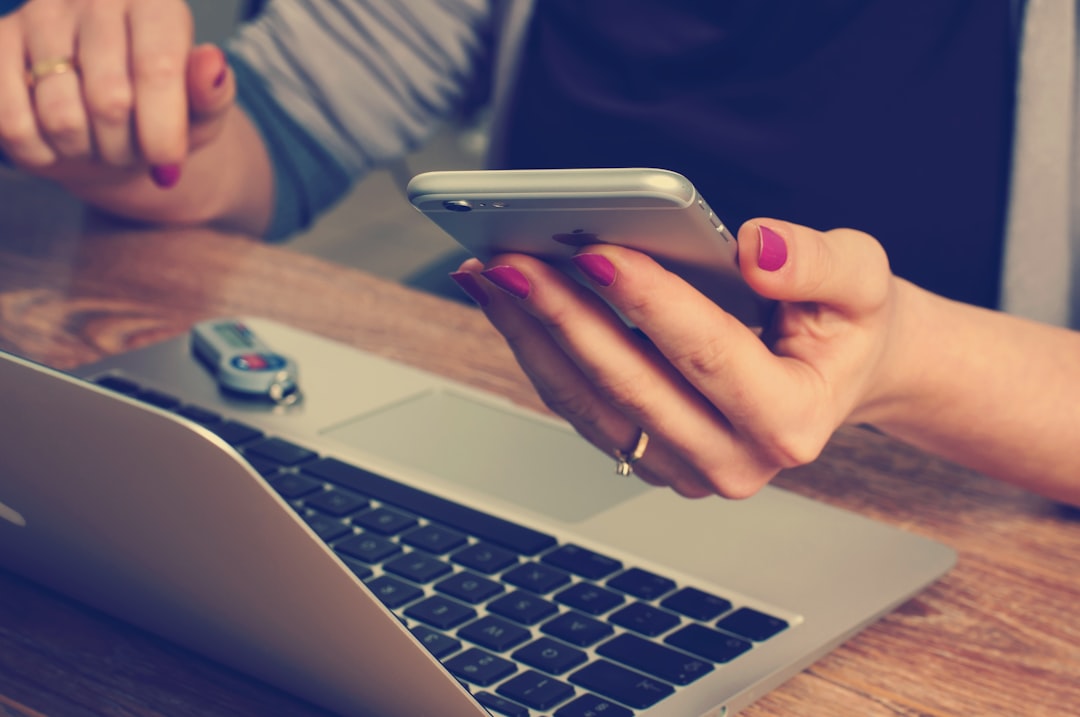
Automated risk assessment models, powered by machine learning, are rapidly changing how the financial industry manages risk. These models can sift through massive amounts of data, identifying subtle patterns that traditional methods often miss, leading to improved predictions and more informed decisions. By continuously learning from data, these models can adapt and become more accurate over time.
However, introducing AI into risk management isn't without its drawbacks. Data privacy concerns and the need for strong model governance frameworks are crucial considerations for organizations adopting these technologies. Furthermore, the complexity of the models themselves introduces potential risks that need to be carefully understood and managed. Financial organizations are increasingly embracing these advanced methods, making comprehensive training programs focused on AI-powered risk assessment a key aspect of professional development for financial analysts in 2024. They need to understand the nuances of these complex models and their potential implications.
Machine learning-powered automated risk assessment models are increasingly being used in finance, primarily due to their ability to process enormous datasets with thousands of variables in a fraction of the time it would take human analysts. This speed-up can significantly improve the pace of risk evaluation within financial institutions.
These models often use a technique called ensemble methods, which combine the outputs of multiple individual algorithms. This approach typically results in more accurate risk assessments, potentially helping organizations like hedge funds better manage risk in volatile market conditions.
One fascinating aspect of machine learning in risk assessment is its ability to discover hidden patterns and previously unknown risk correlations that traditional models often miss. This might include finding unexpected connections between seemingly unrelated financial instruments or market occurrences.
However, the quality of the input data directly impacts the accuracy of these models. Studies have shown that even slight inaccuracies in the dataset can lead to considerable errors in the risk predictions—up to 70% in some cases. This highlights the importance of meticulous data preprocessing before deploying machine learning models.
A crucial advantage of machine learning models is their ability to adapt and learn continuously from new data. This enables financial analysts to continually fine-tune their risk assessments as market circumstances evolve. This continuous learning capability is especially beneficial in dynamic and rapidly changing financial markets.
Some advanced models even incorporate concepts from game theory to simulate potential market behavior under various conditions. This provides financial organizations with valuable insights into the possible outcomes of different scenarios, leading to more informed decision-making processes.
The development of Explainable AI (XAI) is becoming increasingly important in this area. XAI aims to make the decision-making process of automated risk assessment models transparent, revealing how they arrive at specific risk evaluations. This enhanced transparency can build trust among stakeholders and improve compliance with financial regulations surrounding risk assessment practices.
Interestingly, many financial analysts still overlook the importance of training machine learning models on data from past financial crises. Models trained on historical crisis data can significantly enhance their predictive capabilities regarding the potential for future financial instability.
As the use of automated risk assessment models becomes more widespread, regulators are paying closer attention. They're requiring firms to demonstrate not only the accuracy of these models but also their fairness and impartiality, leading to increased complexity in compliance efforts.
While extremely powerful, automated risk assessment models aren't without their limitations. They can unintentionally inherit biases present in historical data, potentially leading to flawed risk assessments. This underscores the need for ongoing monitoring and adjustments by human analysts to ensure fairness and accuracy in the long run.
7 Key AI-Driven Innovations Reshaping Financial Analysis Training in 2024 - AI-Powered Predictive Analytics for Investment Strategies
AI-powered predictive analytics is transforming how investment strategies are developed by using massive amounts of data to make forecasts more precise and improve risk management. These tools combine historical data and machine learning algorithms to find patterns and anticipate market shifts, providing a sophisticated way to distribute assets and optimize investment portfolios. As the finance industry leans more on automated systems, this innovation allows analysts to dedicate their time to complex, strategic decisions instead of routine tasks. But, incorporating AI also presents difficulties like the need for high-quality data and the possibility of biases in the models, which means ongoing monitoring and updates are critical. In essence, while AI in predictive analytics holds great potential for streamlining finance, it needs a thoughtful approach to fully utilize its benefits without compromising ethical standards or sacrificing accuracy.
AI is rapidly changing how we approach investment strategies by enhancing the speed and depth of predictive analytics. These systems can sift through mountains of data – historical market trends, current indicators, even sometimes unconventional data like satellite imagery – far faster than any human could, potentially spotting investment opportunities or warning signs in mere seconds. The ability to quickly process terabytes of data gives AI a major advantage in finding patterns that might otherwise be missed.
One intriguing aspect is the use of complex algorithms to simulate a wide range of market scenarios. This allows investors to get a much more detailed picture of the potential risks and rewards of their decisions before they actually make a trade. It’s like running thousands of virtual market experiments to test a strategy. However, these sophisticated simulations are only as good as the data they are fed, highlighting the need for strong data quality standards.
Beyond traditional financial data, AI is now able to incorporate what is called "alternative data". Things like satellite images of retail parking lots or global shipping data can help paint a better picture of how companies are actually performing, going beyond just the usual financial metrics. This raises interesting questions about what other types of information might be useful in financial models going forward.
While some studies suggest that AI predictive models can be remarkably accurate in certain areas, achieving prediction rates of around 85%, it’s important to remember that this accuracy can change depending on the type of investment and how long we are looking out into the future. It's still not a guaranteed crystal ball.
It's not just about predicting markets; these tools can also analyze the behavior of traders themselves. By understanding how similar trades have played out in the past under similar market conditions, they can suggest better approaches, creating a dynamic feedback loop to improve future strategies. This is similar to how humans refine their approaches based on experience, but on a scale that is far larger and faster.
Traditional methods often rely on straightforward, linear relationships within data. AI’s capacity to detect non-linear connections provides analysts with a whole new level of understanding about how markets behave. This can lead to more innovative investment strategies that we might not have even thought about previously.
Unfortunately, the effectiveness of these tools is very much tied to the quality of the data they’re given. Garbage in, garbage out. Even small inaccuracies in the initial data can lead to significant errors in the predictions, potentially causing significant issues. This emphasizes the need for really robust data validation and cleaning before deploying AI-powered models.
Predictive models are helpful, but they’re not perfect. They can’t anticipate every market shock. This suggests a crucial role for human oversight and the ability to adjust to new information as it comes in. We can’t just blindly follow what the AI suggests.
Some advanced techniques incorporate reinforcement learning, allowing the models to learn and adjust from their own past investment decisions, continually optimizing themselves for changing market conditions. It’s like a continuous cycle of learning and refinement.
Despite all the impressive advances, many financial analysts still have some reservations about trusting these AI systems. Concerns over transparency and interpretability—understanding *why* the model came to a particular conclusion—are prominent, and will need to be addressed if these technologies are to achieve wider acceptance within the investment community. It's understandable that people want to be able to understand the reasoning behind major financial decisions.
7 Key AI-Driven Innovations Reshaping Financial Analysis Training in 2024 - Quantum Computing Applications in Complex Financial Modeling
Quantum computing has the potential to revolutionize complex financial modeling, offering significant improvements in accuracy and efficiency. This emerging technology can be applied to tasks such as pricing securities, optimizing investment portfolios, and developing sophisticated statistical models. These applications directly address a limitation of traditional approaches, namely, their struggle to predict infrequent yet impactful financial events, which was starkly illustrated during recent market downturns.
The unique computational power of quantum computing allows for the handling of exceptionally complex algorithms, yielding deeper insights that improve risk management strategies and support the creation of innovative financial products. As the field advances, it's clear that financial analysis training must incorporate quantum computing concepts to prepare future analysts for the implications of this technology within the industry. While promising, navigating the complex realm of quantum computing within the financial industry will necessitate careful consideration and ongoing adjustments to fully leverage its benefits in real-world situations. The potential for unexpected outcomes and the intricacies of this technology should not be overlooked as we move forward.
Quantum computing's potential to outperform traditional computers is generating a lot of excitement across many industries, finance included. The projected economic impact of this technology is substantial, with estimates suggesting it could add hundreds of billions of dollars in value by streamlining existing processes and generating new applications. Within finance, quantum computing is being explored for tasks like pricing securities, optimizing portfolios, and developing sophisticated statistical models to analyze potential future outcomes.
A core area where quantum computing excels is optimization problems, especially those that involve lots of combinations—which is very common in finance, like deciding how to best allocate assets in a portfolio to maximize returns. The ability of quantum computing to handle these complex problems is seen as a game-changer, potentially providing a huge leap in computing power for tackling algorithms that are currently difficult or impossible to solve effectively.
This new approach can potentially overcome some of the shortcomings of older financial modeling techniques. For instance, traditional methods often struggle to account for rare, low-probability events—as we've seen in some of the past financial crises. The superior computational ability of quantum computing could lead to better models that incorporate these events. This also impacts how we think about managing risk, which can become much more advanced and efficient.
Furthermore, the application of quantum methods in regression analysis, for instance, could significantly enhance our ability to make economic forecasts. This stems from the fact that they can fit mathematical functions to complex datasets with much greater accuracy than classical methods.
These advancements might help overcome previously intractable problems in finance, leading to the creation of entirely new financial products and services. There’s been some notable progress in developing quantum computing tools for financial applications, suggesting that this is increasingly feasible.
However, it's still early days for quantum computing in the finance world. Financial analysis training in 2024 will need to adapt, with educational programs evolving to incorporate elements of quantum computing to prepare professionals for this emerging landscape.
While promising, there's also a need to be cautious about the inherent limitations of quantum technology. The field is still maturing and faces challenges such as high error rates and the need for specialized hardware environments, which can make practical implementation difficult. There's also the potential for overconfidence in quantum-driven models if we are not careful. It's crucial that analysts continue to apply critical thinking to avoid relying too heavily on the models and losing sight of the intricate and nuanced details of real-world markets.
As the finance sector invests heavily in quantum computing research, it's not just about creating better algorithms but also about fostering the next generation of quantum finance experts. We see a clear need to bridge the current talent gap and ensure educational programs effectively prepare analysts for this future.
7 Key AI-Driven Innovations Reshaping Financial Analysis Training in 2024 - Blockchain Integration for Enhanced Data Security in Financial Training
Blockchain technology, with its decentralized structure, presents a compelling solution for strengthening data security in financial training environments. Its inherent features, such as immutability and cryptographic hashing, help create a more secure ecosystem compared to traditional centralized systems, which are susceptible to single points of failure and potential breaches. Applying blockchain to financial training can improve transparency and trust in data handling, especially with sensitive information like student records and course materials.
AI integration further enhances the capabilities of blockchain in this context. Combining their strengths allows for more accurate and dependable data processing, which is vital in financial analysis. This integration can also facilitate the development of sophisticated auditing techniques that enhance accountability and transparency within training programs.
However, realizing the full potential of blockchain integration in AI-driven financial training involves navigating challenges. One key issue is maintaining a balance between robust security protocols and computationally efficient data processing. Carefully designing the AI-blockchain framework is necessary to optimize the benefits while minimizing operational hurdles.
As the finance sector continues its embrace of both blockchain and AI, training programs need to adapt to ensure future financial analysts are equipped to understand and leverage these technologies effectively. This includes building curriculum that covers the nuances of blockchain and AI integration, alongside the unique security considerations and challenges associated with this evolution.
Blockchain's decentralized nature, where data is spread across multiple computers instead of a single central location, could potentially make it harder for hackers to compromise data used in financial training. If one node in the network gets compromised, the data is still safe on the other nodes. This resilience makes it a promising approach to improve security, particularly when compared to traditional systems that are more vulnerable to breaches due to being concentrated in one place.
In the context of training, blockchain's immutable record-keeping (meaning data once recorded can't be altered) could provide a tamper-proof environment for developing and validating complex financial models. If changes are made to a record, it's clearly marked, thus ensuring data integrity during the training process. This is helpful because trust in the training data is critical for building accurate and reliable models.
Blockchain can be applied to verify credentials in a way that's difficult to forge. As people complete financial training programs and earn certifications, those credentials can be recorded on the blockchain, providing a verifiable and permanent record of their accomplishments. Employers can have greater confidence in the qualifications of potential hires, since it's tough to fabricate a blockchain record.
Smart contracts, programs stored on the blockchain that automatically execute agreements when specific conditions are met, could streamline various administrative tasks in financial training. For example, smart contracts could automatically issue certificates of completion when learners finish a course, or even distribute payments for successful training programs. This automation could potentially reduce human error and speed up the administrative process.
Blockchain can help different organizations collaborate securely on financial analysis, which can be valuable in training. By using blockchain's cryptographic features, data can be encrypted and shared only with those authorized to see it, improving transparency while safeguarding sensitive data. This could be a useful tool to foster collaborative projects and learning experiences within the financial training space.
The near-instantaneous nature of data transfer on some blockchain systems could make them well-suited for real-time training simulations in the financial sector. Participants could get faster feedback and respond more quickly to changing market scenarios within a simulated environment, potentially making their decision-making skills more adaptable. However, it is important to remember that this speed can vary across different blockchain networks.
While it offers security improvements, blockchain's adoption also faces practical limitations. The process of validating transactions and maintaining data integrity in blockchain networks sometimes leads to delays. This needs to be considered when evaluating its use for financial training applications. Organizations will need to carefully assess whether the benefits of increased security outweigh potential transaction speed limitations.
The fusion of blockchain and Decentralized Finance (DeFi) offers a chance for financial training programs to provide hands-on experience in this evolving area of finance. Participants can be exposed to real-world DeFi concepts, like liquidity pools and decentralized lending protocols, and how they relate to risk management within a training context. However, the regulatory environment in this space is still under development and participants should approach it cautiously.
Blockchain allows tracking the origins of financial data, known as data provenance. This can be especially important in financial training as it helps determine the reliability and quality of the resources used in educational materials. Having clear records of how data was obtained, processed, and stored can enhance trust in the training process.
As blockchain integration becomes more mainstream in financial training, it presents a new set of regulatory considerations. Financial institutions must be aware of the changing regulatory environment and adapt training procedures to adhere to any new requirements for data privacy and security within this space. While promising, organizations must act responsibly and be ready to comply with evolving standards.
7 Key AI-Driven Innovations Reshaping Financial Analysis Training in 2024 - AI-Driven Personalized Learning Paths for Financial Analysts
The increasing integration of AI across financial analysis is pushing the need for more specialized and customized training. AI-driven personalized learning paths for financial analysts aim to deliver training that's specifically tailored to each individual's skill level, progress, and career aspirations. These paths use data analytics to track performance and adapt the learning content dynamically. This means training can be more engaging and effective, helping analysts absorb information better. However, relying on AI for personalized learning introduces challenges. Ensuring that these systems are designed and implemented ethically and with high-quality data is crucial to avoid potential bias or skewed learning outcomes. As financial analysis becomes more complex, personalized learning might become vital for ensuring that financial analysts have the right skills and understanding to navigate the ever-evolving landscape of the field.
Create AI-powered tutorials effortlessly: Learn, teach, and share knowledge with our intuitive platform. (Get started for free)
More Posts from aitutorialmaker.com: