7 Key Performance Metrics Every Business Analysis Course Should Cover in 2025
7 Key Performance Metrics Every Business Analysis Course Should Cover in 2025 - Customer Lifetime Value Analysis for Mid-Market Software Companies
For mid-market software firms, understanding Customer Lifetime Value (CLTV) is vital. CLTV represents the total income a customer is projected to generate over their entire engagement with the company. Balancing the cost of attracting new customers with the need to keep existing ones is a constant challenge. By understanding CLTV, these businesses can fine-tune their strategies. They can make well-informed decisions on resource allocation, leading to more efficient operations.
However, accurately calculating CLTV for each individual customer can be difficult. This is largely due to a lack of advanced analytics tools tailored for this segment. Without the ability to effectively measure CLTV at a detailed level, optimizing profits from both existing and new customers becomes more challenging.
To refine their approach to pricing and customer retention, mid-market software companies should compare their CLTV to industry benchmarks. This provides context for their performance and helps them see where improvements can be made. Improving CLTV analysis offers a valuable opportunity to identify strategies that promote ongoing business growth and success, particularly in a competitive environment.
In the realm of mid-market software, understanding how much revenue a customer brings in over their entire relationship – their Customer Lifetime Value (CLV) – is becoming increasingly important. We've found that tailoring marketing specifically to existing customers can boost CLV by as much as 30%. This isn't surprising, as the data consistently reveals that a relatively small portion of a company's customers (around 20%) generate a disproportionately large chunk (80%) of future revenue. This reinforces the idea that focusing on retention might be a more impactful approach than simply chasing new customers.
Furthermore, the link between retention and profitability is undeniable. Studies have demonstrated that a modest 5% improvement in customer retention can translate to a substantial profit increase ranging from 25% to 95% for mid-market software businesses. However, it's crucial to remember that CLV isn't a one-size-fits-all metric. Research suggests that the value of a high-tier customer can be up to 10 times greater than that of a low-tier customer, making segment-specific analysis vital. This variability emphasizes the need for a more nuanced understanding of customer segments when applying CLV principles.
One of the tangible benefits of CLV analysis is its ability to enhance sales forecasting. By implementing CLV prediction models, businesses can see an increase in the accuracy of their forecasts by around 20%, allowing for better resource allocation. Despite these potential gains, a concerningly large proportion (over 65%) of mid-market software companies either neglect CLV analysis or lack the proper tools and methods to calculate it effectively, leaving untapped revenue potential on the table.
Another facet of CLV is its ability to predict customer churn. Predictive models can be implemented to identify customers at risk of churning, allowing companies to proactively address these issues and reduce churn rates by up to 15%. This proactive approach is crucial in a competitive landscape where customer retention is paramount. Similarly, fostering a positive customer experience is directly correlated with a higher CLV. Organizations recognized for their exceptional customer service often see a 25% or greater increase in CLV.
Finally, the power of data analytics tools in understanding CLV cannot be overstated. These tools can reveal hidden patterns and correlations between customer behavior and profitability, enhancing overall business strategies. It's fascinating to see how CLV directly influences a company's valuation. Investors often interpret a high CLV as a sign of future financial stability and growth, making it a valuable metric in discussions about investment and the future of the company.
7 Key Performance Metrics Every Business Analysis Course Should Cover in 2025 - Sprint Completion Rate Tracking with Real Time Data Integration
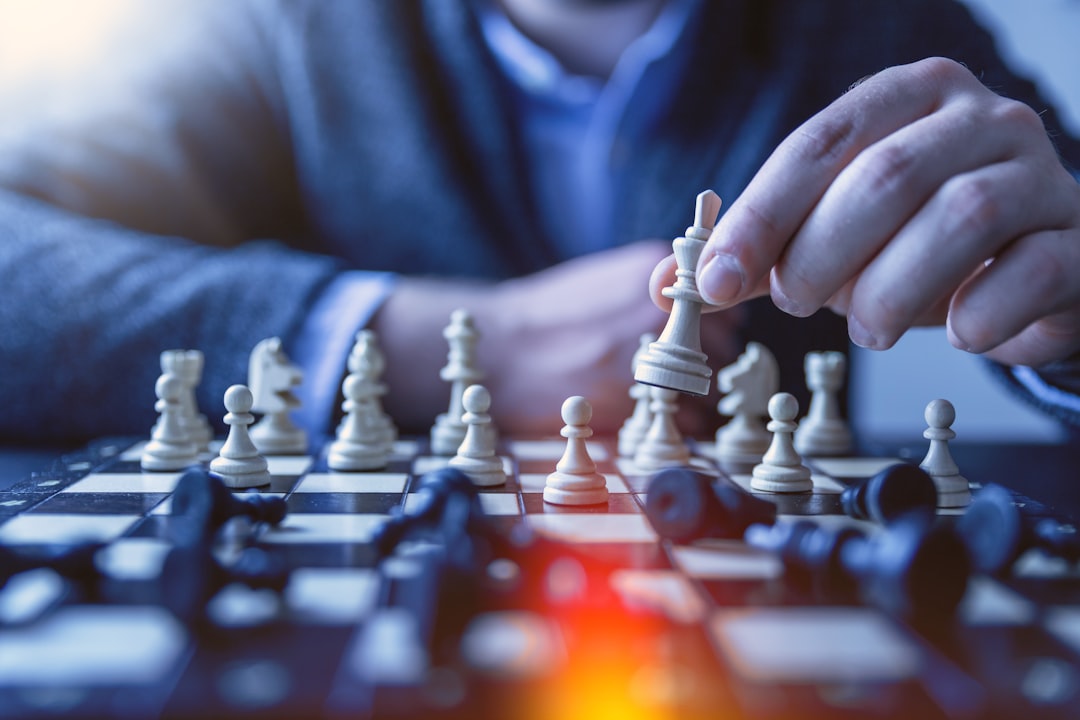
In today's fast-paced development environments, effectively tracking sprint completion rates has become crucial. Integrating real-time data into this process gives Agile teams a much clearer picture of their performance. By constantly monitoring sprint goal achievement, businesses gain a stronger grasp on team productivity and areas where things might be going off-track.
Real-time data gives teams a more accurate sense of their current velocity, enabling faster, more informed decision-making. When problems appear, the ability to react promptly is vital. Tools that visually present agile metrics, such as dashboards, are also beneficial, making it easier for everyone to understand the data and improving transparency.
Organizations are increasingly using this type of data-driven approach to optimize their sprint planning and execution, which ultimately improves the likelihood of successful project outcomes. While this sounds positive, we should be wary of overly simplistic solutions and remember that teams are composed of humans, and a purely numbers-based approach might not always be best. But, when thoughtfully implemented, these tracking mechanisms can provide valuable information to help everyone involved in a sprint improve their collaboration and productivity.
In the dynamic world of software development, especially within agile frameworks, understanding how effectively teams complete their sprints is crucial. Sprint completion rate, a core metric in this context, becomes significantly more valuable when combined with real-time data integration. This allows for a much deeper and more nuanced view of the development process.
While traditional approaches might rely on periodic reports, the integration of real-time data brings a level of immediacy that can greatly influence team dynamics and decision-making. It lets teams, and stakeholders, see exactly where a sprint stands in real-time. This real-time information offers a clearer picture of the project's health and allows for rapid adjustments to address challenges or capitalize on opportunities as they emerge.
For example, suppose a sprint is falling behind schedule. With real-time data, the team can pinpoint the exact source of the delay, be it a specific task, a resource constraint, or a misunderstanding of the requirements. They can then take corrective actions proactively, potentially avoiding a larger setback later in the sprint.
Moreover, real-time tracking provides increased transparency. Team members have a constant, readily available understanding of their individual contributions and the collective progress of the sprint. This increased transparency can boost accountability and lead to a more collaborative and focused work environment. We might wonder if the increased visibility is simply an increase in pressure or if it can ultimately lead to a more positive outcome.
However, it's important to acknowledge that while the promise of real-time data is enticing, its implementation needs careful consideration. It's easy to become bogged down in excessive data points if not managed thoughtfully. It's crucial to identify and track only the most relevant metrics, avoiding the temptation of “more is better”. There's a potential for distraction if the system becomes too complex. This implies a need for ongoing analysis of the data generated to see what's actually useful to ensure that tracking efforts don't overshadow other essential aspects of the project.
Beyond basic tracking, real-time data allows for the use of predictive analytics, offering the possibility of forecasting potential bottlenecks or delays with a higher degree of accuracy. This predictive capability can be extremely valuable in the agile process, especially when working with limited resources and timeframes.
In essence, integrating real-time data into sprint completion tracking isn't just a technical upgrade; it's a shift in the way agile teams operate. It emphasizes continuous monitoring, fosters a more data-driven approach to decision-making, and has the potential to boost overall productivity, as long as the benefits are carefully balanced against the possible drawbacks.
7 Key Performance Metrics Every Business Analysis Course Should Cover in 2025 - Resource Allocation Efficiency Through Machine Learning Models
In the pursuit of streamlined operations, resource allocation efficiency has gained prominence, particularly within the context of machine learning. Machine learning models offer a powerful means of optimizing resource deployment—covering areas like staffing, equipment, and financial resources—with the goal of achieving peak productivity. By leveraging advanced algorithms, these models analyze substantial quantities of data, revealing previously hidden patterns in resource utilization. This leads to smarter choices about resource allocation, thus minimizing waste and optimizing overall performance.
Furthermore, the integration of machine learning into existing frameworks, like enterprise resource planning (ERP) systems, enhances the holistic management of resources. Yet, while the benefits of this approach are substantial, there's a need for a balanced perspective. Over-reliance on algorithmic outputs can be detrimental. Striking a balance between human insight and automated recommendations is crucial for realizing the true potential of machine learning in resource allocation. This balance prevents data overload and ensures that technology's contribution to resource management translates to actual gains rather than mere data accumulation.
Machine learning models offer a powerful way to analyze and optimize how resources are used, looking at things like how much the CPU is working and how much memory is being used. It's becoming increasingly clear that how we distribute resources heavily influences a company's ability to develop and deploy machine learning applications. Understanding how resources fit into the whole machine learning process is crucial.
Looking at research on the topic, it seems that AI methods—including deep learning and machine learning—are being used more and more to figure out the best way to allocate resources. There's also a noticeable trend of integrating machine learning into Enterprise Resource Planning (ERP) systems, which is leading to improvements in how those systems function.
AI-driven resource allocation uses sophisticated algorithms to optimize things like staffing, equipment use, and budgets. The goal is to get more out of the available resources and improve overall productivity. By using data analysis, automation, and real-time adjustments through AI, companies can potentially reduce costs significantly and manage resources much more efficiently.
It's important to recognize that performance metrics play a key role in machine learning. Many metrics exist for evaluating different types of machine learning models, like regression and classification models. These are important for making good decisions about how to allocate resources. The applications of machine learning are also quite wide-ranging. It's becoming very common in cloud computing and is being applied in many different areas, such as manufacturing scheduling and operations management.
Techniques involving machine learning and big data are being used in predictive scheduling and resource allocation. This approach can improve operational efficiency and energy management in large-scale manufacturing operations. It seems that researchers are pushing for the development of frameworks that directly address resource allocation issues, specifically those factors that influence how effective machine learning is throughout its lifecycle. There's still much to learn about how to best address these kinds of inefficiencies, and that's something researchers are actively investigating. While some of the advancements seem promising, there are concerns about over-reliance and the need for human oversight in these systems.
7 Key Performance Metrics Every Business Analysis Course Should Cover in 2025 - Project Cost Variance Using Predictive Analytics
In the realm of modern business analysis, understanding Project Cost Variance through the lens of predictive analytics is increasingly critical. Project Cost Variance, in its simplest form, measures the difference between the planned budget and the actual costs incurred. This provides a clear picture of whether a project is on track financially, revealing if it's over or under budget. By leveraging predictive analytics, which involves analyzing past project data, we can gain a more accurate forecast of future cost and duration variations. This can lead to more precise budget estimations and more proactive management of potential cost issues.
A crucial element in this process is the Cost Performance Index (CPI). CPI is a ratio that acts as a gauge for how effectively a project team is using its budget. A higher CPI usually means the project is within budget and resources are being utilized efficiently. The concept of variance analysis, which compares actual performance against the plan, plays a key role in uncovering discrepancies that may require immediate corrective action. As businesses move forward, incorporating predictive analytics and metrics like the CPI becomes essential for achieving project goals and mitigating unforeseen financial challenges. A shift towards proactively utilizing data to anticipate problems can lead to better project management and healthier financial outcomes.
Project cost variance, the difference between planned and actual project costs, is a crucial metric for keeping projects on track. But what if we could predict these variances before they happen? This is where predictive analytics comes into play. It's a powerful tool that can analyze historical project data to forecast future cost and duration deviations. By studying past projects, we can identify recurring patterns in cost overruns or underruns, which is invaluable for future planning.
One of the key tools in this process is the Cost Performance Index (CPI). The CPI is a ratio that shows how effectively we're using our project budget. It helps us understand whether we're overspending, underspending, or right on target. This, combined with techniques like variance analysis—comparing planned vs. actual performance—lets us spot potential problems early on.
These analyses rely on Key Performance Indicators (KPIs), like cost and schedule variances, as well as Earned Value, to give us a snapshot of project health. Essential metrics to track include the CPI, Actual Cost, Planned Value, and Earned Value, which offer a more comprehensive understanding of budget adherence.
Predictive project analytics, however, goes a step further. It's a more sophisticated approach involving a five-stage process that incorporates elements like risk assessment and organizational understanding. The goal is to predict and mitigate future risks related to project costs. This means shifting from reacting to cost deviations to proactively preventing them.
It's crucial to note that accurate cost variance analysis is essential for early identification of budget overruns, allowing project managers to take corrective actions before they become significant issues. And just as the challenges and objectives vary across projects, so too should the chosen metrics. There's no one-size-fits-all approach to effective project management.
However, it's becoming clear that to truly be effective, future project managers need to be able to utilize the power of predictive analytics. This means business analysis courses need to adapt to include predictive analytics techniques, giving future professionals the tools to handle the complexities of future project environments. We are entering an era where project management is increasingly data-driven, and understanding how to leverage data to improve performance is becoming essential. While the prospect of using these methods is exciting, we should be mindful of potential pitfalls associated with relying too heavily on automated prediction, and it's important to integrate human judgment and experience into the decision-making process alongside these new tools.
7 Key Performance Metrics Every Business Analysis Course Should Cover in 2025 - Business Requirements Quality Score Through AI Validation
The field of business analysis is changing, and a new focus on using AI to assess the quality of business requirements is emerging. AI tools can improve how businesses collect and handle requirements, making it easier for different teams, including business analysts and developers, to work together. These tools often use Natural Language Understanding to analyze project data, giving insights into things like how good the requirements are, potential risks, and how well the requirements are connected to the project's goals. Furthermore, AI can help prioritize requirements based on factors like their importance to the business and how difficult they are to implement. AI validation isn't a one-time thing; it happens throughout the requirement development process, leading to better requirements over time. In today's environment where businesses are constantly looking to improve, using AI to ensure high-quality requirements could be crucial to reaching the highest levels of performance. While there are potential benefits, it's important to be mindful that these are still evolving technologies and the effectiveness in specific situations remains to be fully explored.
AI is starting to play a bigger role in how we assess the quality of business requirements. One interesting area is using AI models, particularly those focused on conversations, to capture and manage these requirements in a more streamlined way. These models, with their Natural Language Understanding capabilities, can help bridge the communication gap between business analysts, quality assurance folks, developers, and project managers. It's intriguing how this can potentially foster better collaboration across teams.
Beyond just communication, AI tools can dig into project data and provide insights into quality metrics like how well-defined a requirement is, what its impact might be on the project, and even identify potential risks. They can help with tracking how requirements are related and linked to other project elements. While this sounds promising, it's crucial to acknowledge that these analyses are only as good as the data they're trained on.
These tools can also make collaboration smoother by creating real-time communication channels, which can potentially speed up the process of getting everyone on the same page about a requirement. Predictive analytics, powered by past project data, can be used to anticipate issues like potential bottlenecks or resource needs, potentially leading to better planning. This seems like a powerful tool to avoid common project pitfalls.
Furthermore, AI algorithms can be designed to prioritize requirements based on their perceived value, complexity, risk, and interdependencies. This can help teams concentrate on the most critical aspects of the project. Companies that have experimented with using AI to improve their Key Performance Indicators (KPIs) have reported seeing benefits, such as enhanced collaboration and better performance in general. While these are positive outcomes, we need to understand how widespread these successes are and whether they can be replicated in different contexts.
It's also interesting that the integration of digital twins is being recommended to improve overall business performance. This implies that the trend is moving toward more realistic simulations and models to represent real-world scenarios and improve business decision-making.
Validating requirements isn't a one-time event. It's a continuous process that happens throughout the whole project lifecycle—from gathering requirements, to writing them up, to design, and even development. This continuous validation approach allows for refining requirements along the way, which is critical for dealing with the inevitable changes that arise in complex projects.
The fact that many leaders consider improved KPIs to be vital for business success suggests a significant shift in how businesses view performance monitoring. This, in turn, highlights the growing importance of these metrics and their role in shaping the future of business analysis. However, we must consider if overemphasis on these quantifiable KPIs might lead to overlooking other important aspects of a project that don't fit neatly into a numerical score. Overall, the use of AI in business requirements is an area with promising potential, though we should remain thoughtful and critically evaluate the real impact of AI in various scenarios.
7 Key Performance Metrics Every Business Analysis Course Should Cover in 2025 - Release Cycle Time Analysis with DevOps Integration
In the current landscape of software development, understanding how long it takes to release new features or fix bugs—what's called Release Cycle Time—is becoming increasingly important, especially when combined with DevOps practices. This metric captures the entire journey of a change, from the initial idea through development, testing, and finally, deployment to users. By tracking how long this process takes, teams can spot areas where things might be slow or inefficient. Generally, shorter release cycles suggest a well-oiled DevOps machine, while longer cycles often point to potential bottlenecks or problems within the process that need attention.
But Release Cycle Time isn't just about speed. Integrating this analysis into a DevOps framework helps businesses connect the work being done with broader business goals. Other important DevOps metrics, such as how often deployments fail or how often new versions of software are released, provide more context and allow organizations to see if their development processes are actually supporting the overall business strategy. In today's world where using metrics to improve things is becoming more common, looking closely at Release Cycle Time can be a key driver of ongoing improvements and the ability to adapt to changes in the marketplace.
Release cycle time, which measures how long it takes to move a feature or bug fix through the entire development, testing, and deployment process, is a valuable metric in the context of DevOps. Some researchers believe organizations using DevOps methods have seen their release cycle times drop by as much as 70%, demonstrating the potential for streamlining the development process. However, it's important to understand that this emphasis on speed doesn't have to come at the cost of quality. In fact, studies show that companies optimizing their release cycles often report a 30-50% reduction in bugs found after deployment. This suggests that carefully implemented DevOps can lead to faster development without sacrificing the reliability of the software.
Furthermore, collaboration tools commonly used within DevOps seem to play a significant role in reducing cycle times. It's not surprising that better communication and a greater ability to provide immediate feedback across development and operations teams can lead to a 20% decrease in the length of the release cycle. Similarly, the widespread adoption of continuous delivery, where teams can deploy code changes at any time, has the potential to shorten release cycles significantly, sometimes from weeks or even months to a matter of hours. This represents a fundamental change in the speed at which companies can bring new features and improvements to market. Automated testing is also emerging as a key element in reducing release cycle times, sometimes slashing the time spent on testing by as much as 90%. By automating these procedures, there is an opportunity for faster feedback loops and quicker fixes when defects are discovered.
Surprisingly, even though DevOps leads to significantly faster release cycles, the time required to recover from deployment failures often drops to under an hour for businesses that integrate these practices. This illustrates that a well-designed DevOps approach can offer a path to swiftly addressing issues without causing a major disruption to ongoing work. But, the transition to faster release cycles involves more than just technical adjustments. Companies often report a 40% increase in employee satisfaction when they embrace DevOps. The reasons for this are likely linked to improved teamwork, greater clarity on workflows, and a better understanding of how their work contributes to the bigger picture. The adoption of data analysis within release cycle analysis is another interesting development. Research suggests that businesses effectively analyzing their release times see a performance improvement of at least 25%. This implies that these organizations are able to identify inefficiencies and bottlenecks more quickly than those who don't have a data-driven approach.
One aspect of DevOps that appears to have a positive impact on release cycle times is the use of cross-functional teams. These teams often include developers and operations personnel, enabling smoother collaboration, potentially reducing delays caused by communication breakdowns or misaligned project objectives. While these benefits of reduced cycle times are evident, it's important to consider the potential challenges of implementing a DevOps strategy. The initial setup, which involves integrating new tools and adapting processes, can be quite demanding on resources and necessitate careful planning. Failing to anticipate the challenges associated with integrating new processes and tools can sometimes lead to poor performance in the early stages of implementation. This emphasizes the importance of well-conceived strategies and a commitment to overcome the initial hurdles to truly unlock the benefits of a DevOps approach.
More Posts from aitutorialmaker.com: