Create AI-powered tutorials effortlessly: Learn, teach, and share knowledge with our intuitive platform. (Get started for free)
AI-Driven Anomaly Detection The Future of Cybersecurity Analytics in 2024
AI-Driven Anomaly Detection The Future of Cybersecurity Analytics in 2024 - AI-powered network traffic analysis unveils hidden threats
AI is increasingly vital in dissecting network traffic to reveal hidden dangers within the intricate web of cybersecurity. These systems, fueled by machine learning, sift through massive datasets of network activity, identifying anomalies that could indicate malicious actions or security breaches. Traditional methods, struggling to keep pace with the ever-evolving nature of cyber threats, are being outmaneuvered.
AI excels in this environment by utilizing historical network behavior to create a baseline of "normal" activity. This allows it to flag deviations in real-time, providing a rapid response capability that human analysts simply cannot match. The result is a proactive security posture with a higher accuracy rate in identifying threats that could slip past human observation.
As we move further into 2024, the reliance on AI within cybersecurity is accelerating. Organizations are embracing these advanced technologies to adapt their defenses to the new landscape of cyber threats. The ability to automatically detect and respond to threats in real-time promises a significant shift in how we fortify our digital infrastructure and counter emerging cyber risks.
AI-powered network traffic analysis is increasingly crucial for uncovering hidden threats within the vast sea of network data. The sheer volume of network traffic generated in today's interconnected world overwhelms traditional security systems, often making it difficult to identify subtle anomalies that can signal malicious activity. AI, with its ability to process massive datasets in real-time, can analyze traffic patterns at a speed and scale previously unimaginable. This allows it to pick up on deviations that human analysts might miss, leading to a significantly faster response to emerging threats.
The algorithms employed in these systems are constantly evolving, refining their ability to distinguish between harmless and malicious traffic. While impressive accuracy rates are claimed, we need to remain vigilant about potential blind spots in these systems. The ability to adapt to new attack techniques is a critical feature, as cybercriminals continuously develop sophisticated methods. AI's potential to learn recursively makes it much more flexible than older systems relying on pre-defined signatures.
However, the unsupervised nature of some AI approaches is both a strength and a potential worry. While it allows for discovery of unknown threats and the automatic creation of new attack signatures, it's not without challenges. How do we verify the AI's interpretation of novel threats? How do we ensure this is done without generating false positives that create unnecessary noise and stress on security teams? These are questions we need to continue investigating.
Furthermore, the ability to spot subtle behavioral changes in network traffic is another intriguing capability. AI can track the normal operational flow of devices and pinpoint unusual activity that might signify an early stage of an intrusion. This proactive approach is essential for reducing damage before a breach fully manifests. Yet, it also raises the question of what constitutes 'normal' and 'abnormal' in increasingly complex network environments. The line can be blurry at times, especially when considering the variability of human behavior on a network.
While there's clearly great promise in AI-driven network security, we need to balance the benefits with ethical considerations. We need to address issues of privacy, and how vast amounts of sensitive data within network traffic are handled and utilized. As we increasingly rely on AI for network security, it's vital that we also develop a deeper understanding of the AI system's logic and decision-making processes to avoid unintended consequences. The evolution of AI in cybersecurity is a fascinating and fast-moving field, but responsible development and critical analysis remain paramount.
AI-Driven Anomaly Detection The Future of Cybersecurity Analytics in 2024 - Machine learning algorithms adapt to evolving attack patterns
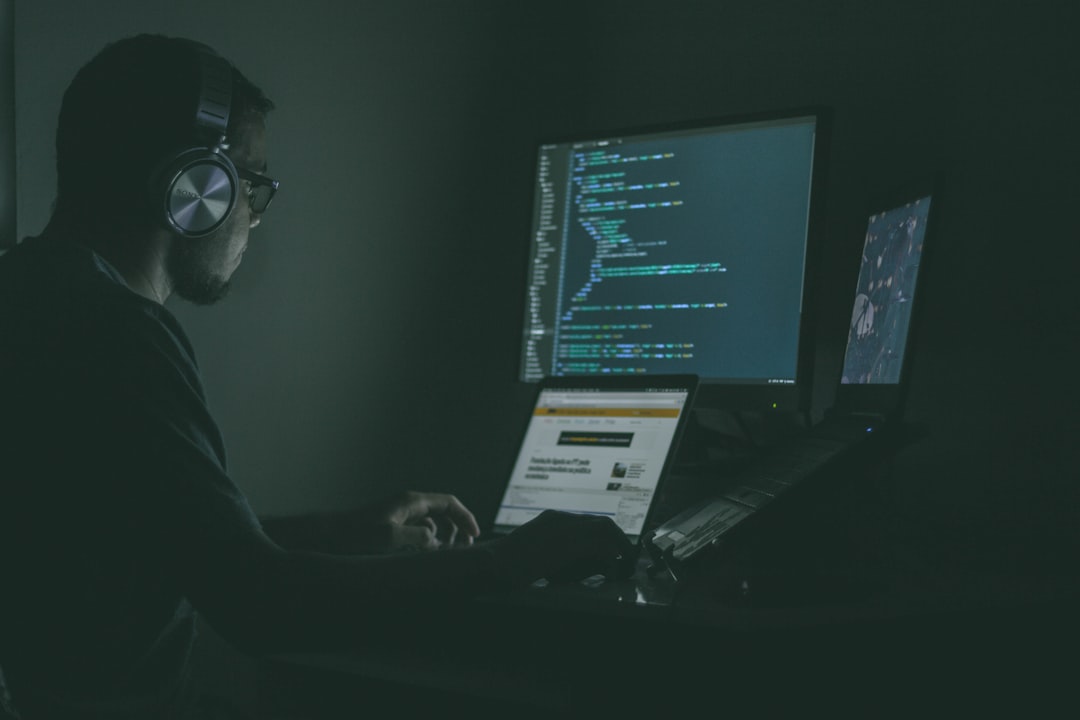
Machine learning algorithms are becoming increasingly adept at adapting to the ever-changing landscape of cyberattacks. This adaptability is crucial because threat actors are constantly refining their tactics, creating more sophisticated and dynamic threats. These algorithms are trained to analyze massive amounts of data in real-time, which allows them to detect subtle changes in network behavior that might suggest a new attack is emerging. Traditional security measures often rely on fixed rules or signatures, making them ill-equipped to handle the rapid evolution of cyber threats. Modern machine learning systems, in contrast, are capable of continuously refining their understanding of what constitutes 'normal' network behavior, enabling them to better discern between harmless and malicious activities.
The capacity of machine learning algorithms to learn and adjust in response to novel attack techniques is a significant advantage in the ongoing cyber security arms race. As attackers devise new ways to infiltrate systems and exploit vulnerabilities, these algorithms can adapt their defenses to stay ahead of the curve. This dynamic approach is vital for maintaining a robust security posture in the face of increasingly advanced cyber threats.
However, this flexibility also presents challenges. The continuous updating of these algorithms raises questions about the reliability of their interpretations, and the potential for an increase in false positives. False positives can be disruptive, causing unnecessary alarms and potentially diverting security resources from genuine threats. Therefore, ongoing evaluation and refinement of these AI-driven solutions are essential to ensure their effectiveness and minimize the risk of errors. We need to strike a careful balance between the advantages of adaptability and the need for reliable, accurate responses to cyber threats.
Machine learning algorithms are increasingly adept at keeping pace with the ever-changing landscape of cyberattacks. They achieve this through techniques like online learning, which allows them to continually update their models using new data without requiring a complete retraining process. This dynamic approach makes them more responsive to real-time threats.
Beyond just content, these algorithms can scrutinize metadata like timestamps and network communication patterns, leading to richer insights into potential anomalies. Interestingly, the training process often includes synthetic datasets, simulating various attack scenarios to provide a broader understanding of potential threats compared to just relying on historical incident data.
The integration of reinforcement learning is another fascinating avenue, with algorithms learning not just from successful but also failed threat detection attempts. This allows them to refine their strategies over time based on outcomes.
However, this adaptation process isn't without its hurdles. "Concept drift," where the underlying data patterns change, can impact the accuracy of anomaly detection. Robust algorithms need to be able to recognize these shifts to maintain effectiveness.
Transfer learning offers a promising solution by applying insights from one environment to another, potentially speeding up the adaptability of security defenses across various contexts. One surprising aspect is the ability of certain machine learning approaches to detect anomalies even with minimal labeled data, reducing the burden on security teams to provide vast datasets for training.
Furthermore, combining multiple models through ensemble methods enhances the overall robustness of detection systems. This helps manage diverse attack tactics and minimizes the risk of overlooking threats (false negatives) or generating unnecessary alarms (false positives).
Researchers are currently exploring the intersection of machine learning and behavioral analytics. The aim is for algorithms to go beyond simply detecting technical anomalies to also interpret shifts in user behavior, leading to better insights into potential insider threats.
Unfortunately, this evolutionary arms race in cybersecurity is also characterized by a corresponding rise in adversarial machine learning. As the defenses strengthen, so do the offensive tactics employed by cybercriminals. This necessitates continuous refinement and development of more sophisticated algorithms to maintain the upper hand in detection. Staying ahead of the curve in this ongoing battle requires ongoing research and innovation.
AI-Driven Anomaly Detection The Future of Cybersecurity Analytics in 2024 - Automated threat prioritization reduces analyst workload
Automated threat prioritization is transforming how cybersecurity teams handle their workload, especially as the volume and intricacy of cyberattacks grow. AI algorithms now empower security systems to automatically evaluate and rank threats in real-time, enabling analysts to focus their attention on the most severe vulnerabilities. This automation reduces the burden on security personnel and improves the effectiveness of incident response. Organizations adopting these advanced tools see potential for better accuracy and efficiency in managing security risks, shifting the cybersecurity landscape towards more intelligent, automated solutions. Yet, it's important to acknowledge the challenges of adapting to evolving threats and to be aware of potential biases within AI decision-making processes.
One of the more interesting aspects of AI in cybersecurity is its ability to automatically prioritize threats. This capability lessens the burden on human analysts, who often get bogged down by the sheer volume of alerts. By automatically ranking threats based on severity and potential impact, these systems help analysts focus on the most critical issues. It's like having a smart assistant that sorts through a massive inbox, pulling out only the most urgent emails. Studies have shown this can significantly reduce the time analysts spend on routine tasks, potentially freeing them up for more complex investigations or strategic security planning.
While promising, the effectiveness of automated threat prioritization depends heavily on the quality of the underlying data and the sophistication of the algorithms employed. The systems often rely on various factors to determine the priority of a threat, such as the type of attack, affected systems, and potential consequences. Some systems also incorporate external threat intelligence feeds, gaining context from recent global cyber incidents to refine their assessment.
Beyond just prioritizing, some systems also adapt and learn over time. They analyze past incidents to refine their decision-making processes, constantly adjusting to the ever-evolving tactics used by cybercriminals. This continuous improvement aspect is crucial in the ongoing cyber security arms race. Of course, it introduces some new complexities like ensuring the AI doesn't develop unintended biases or create false positives.
The potential benefits are intriguing: a reduction in the volume of alerts, faster response times to actual threats, and a more focused approach to incident response. We need to consider the trade-offs, too. Are the AI systems themselves vulnerable to manipulation or attack? What happens if an algorithm starts to prioritize based on incorrect or incomplete data? The reliability of these systems is paramount, and the algorithms need to be transparent and explainable for human oversight. It's an interesting interplay between automation and human expertise that will be critical to the evolution of cybersecurity.
AI-Driven Anomaly Detection The Future of Cybersecurity Analytics in 2024 - Real-time detection of zero-day exploits through behavioral analysis
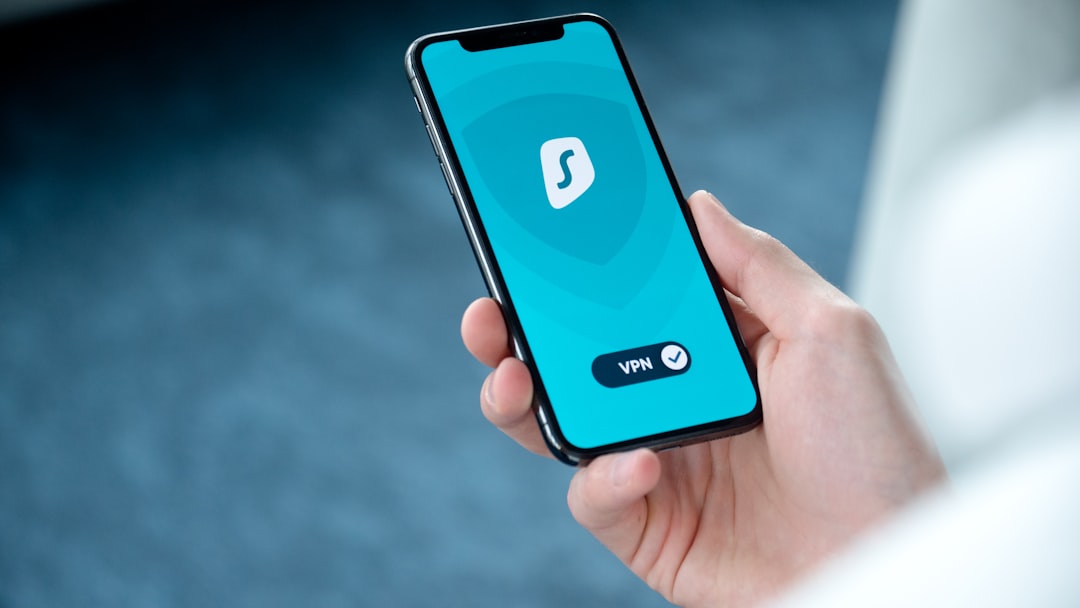
Real-time detection of zero-day exploits using behavioral analysis is a crucial area of cybersecurity development in 2024. This approach involves monitoring the actions of users and systems to develop a standard of "normal" operations. Deviations from this standard can signal potential threats, including previously unknown exploits (zero-day exploits). AI and machine learning play a vital role in enhancing these capabilities, adapting to new patterns of malicious behavior and improving the speed and accuracy of threat detection compared to older security measures. This is particularly crucial given the increasing sophistication of cyberattacks.
While highly promising, there's a need for continued evaluation and improvement to manage issues like false positives, which can overwhelm security teams with unnecessary alerts. The ability of the algorithms to reliably interpret new types of attacks, especially as the attack methods evolve rapidly, is essential. Overall, the trend towards real-time cybersecurity monitoring through behavioral analysis and AI highlights the need for robust systems capable of swiftly identifying and mitigating even the most advanced threats.
Real-time zero-day exploit detection relies on sophisticated algorithms that pinpoint suspicious behavior before it can be leveraged for malicious purposes. These systems excel by analyzing user and system actions to establish a baseline of normal activity, enabling the identification of deviations that might signal a security threat. AI-driven anomaly detection systems leverage machine learning to adapt to new malicious behaviors, enhancing conventional security practices.
The effectiveness of this approach hinges on continuously updating detection algorithms with fresh data, allowing the systems to learn and adapt. In the cybersecurity landscape of 2024, we anticipate a surge in the integration of AI, which promises faster and more precise threat identification. There's a clear trend towards automating threat responses using behavioral analysis to mitigate zero-day risks without needing human intervention. The future of cybersecurity analytics points to the creation of more user-friendly interfaces that facilitate analysts' visualization of anomalies and threats.
By proactively identifying potential weaknesses through behavioral analysis, organizations can bolster their security stance. This real-time monitoring trend stems from increasingly sophisticated cyber threats and the imperative for swift incident responses. The development of standardized methodologies for behavioral analysis and anomaly detection requires collaborative efforts from stakeholders across the cybersecurity sector.
While promising, some challenges persist. For instance, traditional antivirus solutions, even with their updates, are only successful in identifying a small portion of zero-day exploits. This stark reality underscores the need for more refined techniques. Further, it's becoming increasingly clear that observing user behavior is an insightful way to pinpoint potentially malicious activity, including the often-missed reconnaissance phases of an attack. We need to take note that some of these systems can process millions of data points per second, a rate that surpasses legacy methods.
Instead of fixed thresholds, the adoption of dynamic baselines based on user behavior is a more robust approach, as it readily adapts to shifting patterns of activity. It's important to understand that the AI behind these detection systems utilizes data from numerous sources to achieve a broader context of any threats. The ability to understand the "where", "when", and "who" related to an attack is incredibly valuable, though unfortunately these systems aren't perfect and can produce many false positive alerts.
In the hunt for compromises, systems are moving away from simply relying on indicators of compromise (IOCs) associated with known malware to evaluating the behaviors observed. This behavioral analysis approach is particularly beneficial in identifying zero-day attacks that have no known signatures. One noticeable difference between behavioral analysis and static code analysis is that behavioral analysis looks at what the code is actually doing, not just the code itself. This is an advantage in identifying hidden threats.
However, even in 2024 these systems are still not without challenges. One major hurdle is scalability in very large and complex networks. The sheer volume of data produced in some environments can overwhelm the computational resources and necessitate ongoing innovations in the algorithms to improve efficiency. As the field moves forward, these are the issues researchers will need to overcome if the promise of real-time behavioral analysis is to be fully realized.
AI-Driven Anomaly Detection The Future of Cybersecurity Analytics in 2024 - Integration of AI with SIEM platforms enhances incident response
Integrating AI with SIEM platforms is revolutionizing incident response in cybersecurity. AI-powered SIEM systems automate the process of collecting, parsing, and analyzing security data, allowing for much faster threat detection and prioritization than traditional methods. Security teams can more efficiently focus on critical threats rather than getting bogged down in a sea of alerts. This shift promises to optimize cybersecurity operations and improve the speed at which incidents are addressed.
However, there's an ongoing need to scrutinize the reliability of AI interpretations within these systems. False positives, though less frequent than in older SIEM systems, still pose a risk of diverting attention from true security incidents. The ever-changing nature of cyber threats also necessitates ongoing evaluation of AI-powered SIEM solutions to ensure their accuracy remains effective. As we move through 2024, the role of AI in SIEM systems continues to grow, highlighting both its powerful potential and the need for continuous vigilance in ensuring its dependability within the evolving cybersecurity landscape.
The integration of AI with SIEM platforms is significantly enhancing how we respond to security incidents. By analyzing vast amounts of data and identifying patterns, AI can now help us predict potential threats, allowing for more proactive defenses. This predictive capability goes beyond simply reacting to attacks and shifts towards preemptively hardening our security posture.
Beyond prediction, AI-powered SIEMs also offer a richer understanding of the context surrounding a security event. They can correlate alerts with broader events, like geopolitics or recent cyberattacks, which helps us make more strategic decisions regarding response prioritization. In a world where threats are increasingly interconnected, understanding the "big picture" is crucial.
Further, the forensic process itself is becoming more automated. AI algorithms are now able to quickly sift through logs and events, reconstructing attack timelines and quickly identifying how a breach occurred. This streamlined approach can speed up the process of mitigation and remediation, helping us learn faster from each incident and improve our future defenses.
We also see that establishing more nuanced, organization-specific "normal" operating patterns is now possible with AI. This leads to a reduction of false positives, which plagued some older systems. By precisely tailoring these baselines to our own networks, we get a more accurate picture of anomalous activity. This ultimately improves the quality of threat detection.
One of the biggest advantages of these AI-enhanced SIEMs is their ability to automate tedious tasks. This frees up security analysts to focus on more strategic concerns, rather than getting bogged down in mundane data analysis or alert triage. The efficiency gains can be significant, and a more strategic focus can lead to better outcomes.
In a world of rapidly evolving threats, a key feature of AI-powered SIEMs is their rapid adaptability. These systems can learn new attack methods and adapt their detection strategies on the fly. This reduces the need for manual intervention and the downtime associated with system upgrades. We're moving from a reactive to a more proactive model of security.
Furthermore, we can now seamlessly integrate a variety of external threat intelligence sources into our SIEMs. This brings a wealth of contextual information into our security analyses, helping us paint a more complete picture of the threats facing us. Being able to understand both internal and external signals significantly improves our situational awareness.
Additionally, the rise of AI within SIEMs has significantly improved how we visualize security data. We now have intuitive dashboards that present threat trends and patterns in easily digestible formats. This allows analysts to quickly spot emerging threats and make timely decisions. The faster we can react, the less damage a threat can cause.
The most interesting development is the increasingly collaborative relationship between humans and AI in the security domain. AI handles the heavy lifting of data processing and preliminary analysis, while analysts bring their intuition and expertise to make critical decisions. This collaboration is critical in managing complex threats, ensuring that we leverage both human and machine strengths.
However, we also need to be mindful of potential bias in AI systems. The decision-making process needs to be transparent and fair to avoid unintended consequences. We don't want a system that exacerbates existing biases or unfairly targets specific users or systems. Ensuring that these systems are ethical is paramount in maintaining a secure and equitable digital environment.
AI-Driven Anomaly Detection The Future of Cybersecurity Analytics in 2024 - Predictive analytics forecast emerging cybersecurity risks for 2025
Looking ahead to 2025, the cybersecurity landscape is expected to become even more intricate and challenging. We can anticipate a rise in AI-powered cyberattacks, necessitating more robust defenses. Organizations will likely need to adopt more advanced solutions, including AI-driven predictive analytics, to stay ahead of these evolving threats.
The combination of human expertise and artificial intelligence will play a key role in shaping the future of cybersecurity. Attackers are likely to use AI to create increasingly sophisticated and adaptive attacks, while defenders will use it to build more proactive and effective security systems. Predictive analytics will likely be central to this effort, helping organizations predict and potentially prevent emerging risks. This includes identifying vulnerabilities, such as zero-day exploits, that might otherwise go unnoticed.
While AI offers many potential benefits in the fight against cybercrime, it's important to be aware of the ethical concerns and potential biases that AI systems can introduce. Ensuring that AI-powered security solutions are fair and equitable will be crucial. Maintaining a balanced and thoughtful approach to the use of AI in cybersecurity will be critical as we move forward.
Looking ahead to 2025, the cybersecurity landscape is projected to be significantly reshaped by emerging threats and the evolving role of AI. We can expect more complex attack strategies, potentially leveraging both technological and human vulnerabilities, requiring more adaptive AI models to stay ahead. The increased accessibility of quantum computing presents a serious risk, as it could render existing cryptographic protections obsolete. Organizations will need to shift towards quantum-resistant algorithms to mitigate these potential future threats.
AI's influence on social engineering is likely to grow, with algorithms becoming capable of crafting ever more believable phishing attempts tailored to individual users. The "Ransomware as a Service" model, where cybercriminals essentially rent out attack tools, could also gain wider adoption, making ransomware a more pervasive threat. We might also see attacks focus on exploiting biometric data, given its increasing use in authentication. This shift could lead to more widespread identity theft and introduce new challenges for individuals and organizations alike.
Furthermore, we're likely to see more emphasis on using AI to identify insider threats. Machine learning algorithms could be used to spot subtle changes in employee behavior that might suggest a breach from within. Combining AI with UEBA will offer a richer understanding of user behavior by comparing individuals to their peers, potentially uncovering threats that might be missed when only looking at individual activity.
The regulatory landscape is also expected to change, with more stringent laws governing data privacy and cybersecurity. This will force organizations to not only enhance their AI-driven anomaly detection systems but also ensure compliance with the evolving legal framework. However, the constant evolution of threats may lead to a phenomenon called "concept drift" where existing AI models lose accuracy over time. This means constant re-training and updates will be critical to maintain the effectiveness of these systems.
Finally, the increased reliance on AI within cybersecurity could lead to a growing skills gap. Security analysts may need more specialized training to interpret the insights generated by sophisticated AI systems. This suggests the need for increased investment in training and ongoing education within the cybersecurity workforce. Overall, while AI provides potent tools for mitigating future cyber threats, the field is a constantly evolving arms race that requires proactive vigilance and ongoing development to keep pace with the increasingly sophisticated attack tactics that will emerge in 2025 and beyond.
Create AI-powered tutorials effortlessly: Learn, teach, and share knowledge with our intuitive platform. (Get started for free)
More Posts from aitutorialmaker.com: