AI-Driven Learning Analytics The Next Evolution in Personalized Education Environments for 2025
AI-Driven Learning Analytics The Next Evolution in Personalized Education Environments for 2025 - Machine Learning Models Track Student Progress Through Real Time Assessment Data 2024
In 2024, machine learning is increasingly central to how educators track student progress. These models are capable of sifting through a wide range of data, from quiz results to how students interact with online learning materials. This data deluge provides a much more detailed picture of a student's understanding compared to traditional methods. The ability to identify individual learning gaps and strengths in real-time has opened the door to more focused and tailored educational experiences.
The core concept of "knowledge tracing" relies on these machine learning algorithms to monitor each student's journey through the learning material. This constant monitoring allows educators to intervene proactively, identifying areas where students are struggling and potentially adjusting the learning path to help them succeed. While still relatively new, these AI-driven analytics tools are demonstrating promise in shaping more responsive and personalized educational environments. It remains to be seen how effective and ethical these methods will be in the long run, but the potential for improvement in education is undeniable. The next few years will likely witness a rapid development in this area, potentially leading to substantial changes in how education is delivered by 2025.
In 2024, machine learning models are increasingly being integrated into educational settings to monitor student progress in real-time using assessment data. These models can sift through vast quantities of student interactions and responses, quickly identifying areas where students might be struggling. This rapid analysis, which could take educators weeks with traditional methods, now allows for timely interventions to address knowledge gaps within minutes.
There's also potential in leveraging unsupervised learning techniques to pinpoint patterns in student behavior. This offers the intriguing possibility of detecting early signs of disengagement or potential drop-out risks, potentially before a student even realizes they're losing interest. Natural language processing (NLP) has emerged as a powerful tool within these models, allowing for a more nuanced assessment of student understanding. They can evaluate not just comprehension, but also the ability to critically analyze information – something traditional assessments often struggle to capture.
The adaptive nature of these models allows them to create unique learning paths tailored to each student's individual needs. As a student progresses, the path adjusts dynamically, ensuring that content remains relevant to their current performance and learning style. Some approaches even mimic peer-learning environments, where the system intelligently pairs students with similar learning needs and paces to encourage collaborative problem-solving.
The ability to incorporate diverse data sources from various learning environments, such as classroom interactions and online activities, creates a more comprehensive picture of each learner. This integration of multimodal data is becoming increasingly common in learning analytics platforms, offering a holistic view of a student’s strengths, weaknesses, and learning preferences. We are also seeing the promise of these models in asynchronous learning environments. The instant feedback they can provide enhances student engagement and retention, particularly important for students learning remotely.
Further, there's evidence that some of these models are contributing to a reduction in achievement gaps by using historical data and behavioral indicators to identify at-risk students and provide targeted interventions. These models can also be employed to predict longer-term educational and career pathways by identifying trends in data collected over time. This ability to foresee potential future outcomes is becoming increasingly valuable for educational institutions in providing guidance and supporting student decision-making.
However, the rise of AI in education necessitates careful consideration of ethical concerns. The responsible and transparent use of these technologies, including data privacy and potential algorithmic bias, are crucial discussions as these models continue to shape the future of education.
AI-Driven Learning Analytics The Next Evolution in Personalized Education Environments for 2025 - Neural Networks Map Individual Learning Patterns Across 500 Million Global Students
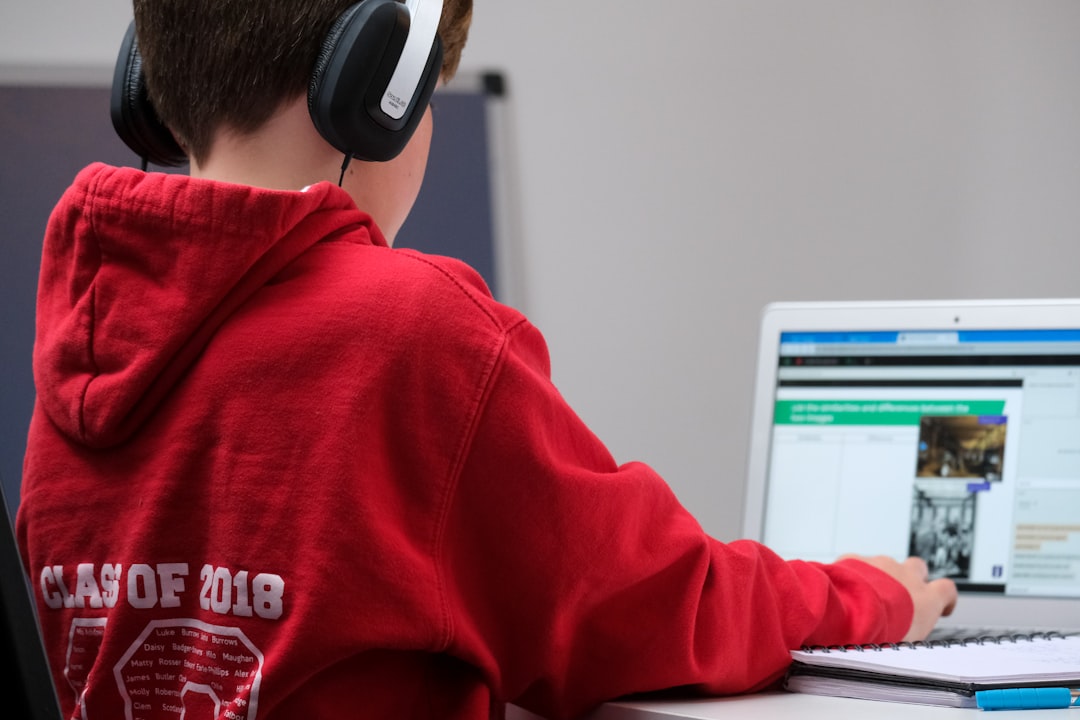
The field of personalized education is experiencing a dramatic shift with the advent of neural networks capable of analyzing learning patterns across a vast global student population, now reaching 500 million individuals. These networks leverage AI's power to process a wide array of data, including student performance, preferences, and engagement with learning materials. This allows for the development of educational experiences uniquely tailored to each student's individual needs. By adapting dynamically to a learner's progress and responses, these systems emulate the complex learning processes of the human brain, fostering more effective educational interactions.
While offering great promise for improving learning outcomes and streamlining the educational process, the integration of AI in this way raises important considerations regarding ethical implications and potential downsides. Concerns surrounding data privacy and the possibility of biased algorithms must be addressed thoughtfully as these technologies evolve. It's crucial to strike a balance between maximizing the benefits of AI-driven learning while mitigating the associated risks and ensuring responsible and transparent use of these powerful tools. Moving forward, a careful and considered approach will be essential in realizing the full potential of AI in shaping the future of education.
The application of neural networks to learning data from a staggering 500 million students globally establishes a truly massive framework for understanding how people learn. This opens up possibilities for identifying common stumbling blocks and areas of success across diverse learner populations. It's fascinating to see how this scale can reveal similarities in learning patterns that might otherwise be hidden within smaller datasets.
One of the intriguing aspects of this is the potential for a much more immediate and refined feedback loop. Not only are these neural network systems capable of providing real-time insights to the students themselves, but educators also gain a much more dynamic understanding of individual student needs. This could eventually shift the role of educators more towards facilitation, where they guide the learning process rather than solely delivering instruction. It remains to be seen whether educators and the educational system will embrace this potential shift in focus.
Beyond simply tracking academic performance, these networks can delve deeper into the complexities of human behavior related to learning. Factors like emotional state and motivation, which can significantly influence learning, can now be potentially modeled and understood within this vast dataset. It's a unique opportunity to potentially integrate elements that have always been understood as critical to learning success but are often very difficult to measure within traditional educational settings. It will be interesting to see what new metrics and insights emerge from this aspect.
By continually analyzing interactions and performance, these systems can suggest highly personalized learning paths designed to capitalize on individual strengths while proactively addressing areas for improvement. These learning paths are not static; they adapt dynamically as the learner's knowledge and skills grow, keeping the learning process relevant and engaging. This is a stark contrast to traditional teaching and assessment models, where a one-size-fits-all approach often leaves some students behind. However, it's critical that we ensure that the dynamic adjustments do not inadvertently lead to unintended consequences like reinforcing biases present in the data or creating educational environments that isolate students.
Further, we can now potentially assess cognitive load. By analyzing engagement patterns, these models can potentially get a better handle on the optimal balance between challenge and comprehension for each student. This could provide a helpful way to avoid pushing students too hard or creating environments where they are simply disengaged or burnout.
There is an exciting potential for global collaboration and knowledge sharing emerging from this data as well. Analyzing the vast amount of data from across the globe offers opportunities to investigate how cultural factors or societal conditions might influence learning styles. This could help identify the most effective teaching practices for different regions, fostering a more culturally sensitive and globally aware educational experience.
We are also seeing the potential to increase the accuracy of predicting learner attrition. By recognizing early warning signs of disengagement, there is a chance that institutions can implement interventions that prevent students from leaving programs prematurely. While we must be careful not to overemphasize predictive capabilities, especially in vulnerable student populations, it's encouraging that these tools can be leveraged to support student retention.
It's interesting to see the use of gamification elements driven by the algorithms. These are tailored to individual learning styles and preferences, potentially making the process of learning more inherently engaging and motivating. How well this works across student populations and in different subjects is something to be observed.
The increased ability to track social interactions within these educational platforms holds great promise for enhancing collaborative learning. We might be able to understand more clearly how collaboration impacts learning and potentially redesign educational environments to better support it. However, it is important to consider potential privacy and ethical concerns. It's crucial to balance the benefits of this approach with protecting student privacy.
The sheer scale of these models means that successful interventions developed in one context could be implemented elsewhere with greater confidence. This offers the opportunity to disseminate successful educational practices and potentially help to drive improvements in educational outcomes across the world.
It is a significant development, but there's still much to explore regarding responsible and ethical use, especially with such large-scale systems. The complexities and challenges associated with interpreting this information are still being explored, and it will be fascinating to witness the evolving role of educators and educational institutions as they attempt to adopt these advanced technologies.
AI-Driven Learning Analytics The Next Evolution in Personalized Education Environments for 2025 - Advanced Natural Language Processing Enables Automated Essay Grading and Feedback
Advanced Natural Language Processing (NLP) is significantly altering how essays are assessed and feedback is provided in education. These improvements allow automated grading systems to better understand human language, moving away from the often subjective nature of traditional grading methods. By incorporating deep learning and intricate algorithms, these systems can now efficiently identify areas where students struggle and deliver personalized feedback that enhances the learning process. The integration of these technologies with learning management systems opens up opportunities for more adaptive and tailored education. However, it's crucial to consider the ramifications of this automation, such as the privacy of student data and the potential for biases within the AI models themselves. There's a need for ongoing scrutiny as these technologies become more ingrained in educational practices.
The increasing sophistication of natural language processing (NLP) is fundamentally changing how we approach essay grading and feedback. These advancements, built upon deep learning and increasingly powerful algorithms, are enabling automated systems to understand and evaluate human language with remarkable accuracy. For instance, NLP-powered systems can now delve into the complexities of writing, going beyond simple grammar checks to analyze sentence structure, vocabulary choices, and the overall coherence of an essay. This more nuanced analysis provides a richer picture of a student's writing ability compared to traditional rubrics.
Beyond simply assigning a grade, NLP is ushering in an era of truly personalized feedback. These systems are now able to pinpoint specific areas where students are struggling, offering tailored guidance on improving things like thesis statement development or argumentation. This personalized feedback could potentially accelerate a student's writing development in ways that traditional, one-size-fits-all feedback might not. The efficiency gained from automation is also noteworthy. Educators can leverage NLP-driven grading to get rapid feedback to students, allowing them to spend more time on instruction and less on grading. This is particularly important in online learning environments where student numbers can be significant.
There's a growing recognition that NLP systems can be used to address some of the challenges of traditional assessment. For example, NLP systems can potentially identify bias within a student's writing, providing insights into how social or cultural influences might be impacting language patterns. This capability, paired with statistical analysis, could offer educators a new lens through which to understand and address potential inequalities within their student population. Interestingly, NLP is being explored to assess student engagement through language itself. Analyzing how a student writes about a topic could potentially provide clues about their interest and motivation levels, potentially offering educators valuable insights into learner psychology.
As NLP evolves, the potential for cross-linguistic evaluation grows. This could transform assessment by allowing for more inclusive educational environments that serve diverse student populations globally. The systems are also able to dynamically adjust grading criteria. Rather than relying on fixed rubrics, NLP systems can adapt their standards over time based on data from previously graded essays, which could lead to a more robust and relevant assessment process that evolves alongside educational standards. The extensive data generated by these systems can fuel valuable research into writing proficiency across diverse demographics, which could then guide improvements in curriculum and teaching practices.
Furthermore, NLP-based tools can also start to detect signs of cognitive overload in students, offering another avenue to improve the learning experience. By analyzing writing style and content, systems can potentially indicate when a student might be struggling with the material, suggesting strategies to educators for adapting their teaching or workload. While these developments hold considerable promise, it's important to acknowledge that the responsible and ethical implementation of these tools are vital. It will be critical to continue researching potential biases or unintended consequences that might arise within these complex systems as they become more integrated into education. It's an exciting time to witness how NLP is changing how we evaluate and support writing development, and the potential implications for education are vast.
AI-Driven Learning Analytics The Next Evolution in Personalized Education Environments for 2025 - Predictive Analytics Identify Learning Gaps Before Test Performance Declines
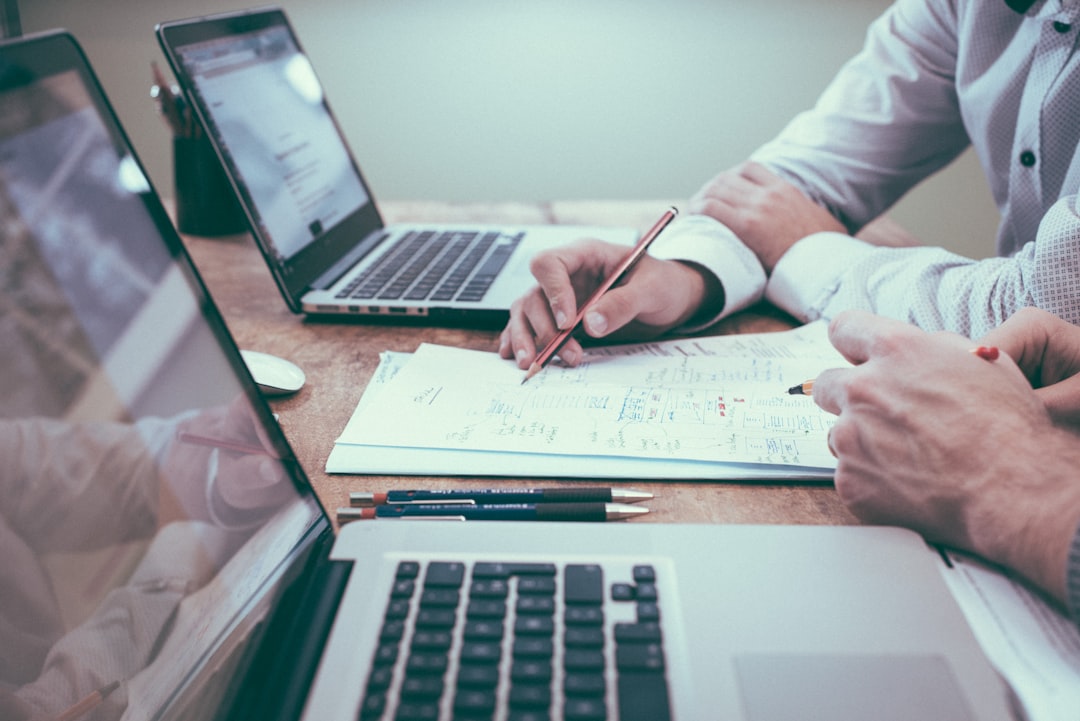
Predictive analytics is increasingly being used in education to pinpoint learning difficulties before they impact test scores. By continuously analyzing student data, educators can anticipate potential problems and offer targeted support, ensuring students remain on the intended learning path. This approach is integral to the development of AI-driven learning analytics, a movement that emphasizes highly personalized educational experiences. These systems strive to create environments that cater to each student's unique learning style and areas of strength and weakness. While these technologies have the potential to greatly enhance student outcomes, they also present a number of considerations. Questions regarding data privacy, potential biases within the algorithms themselves, and the broader impact on educational practices need careful examination. Ultimately, the integration of predictive tools into education offers a significant opportunity to optimize the learning experience and pave the way for greater student success in a challenging educational environment.
Within the evolving landscape of AI-powered education, predictive analytics is emerging as a powerful tool for identifying potential learning roadblocks before they impact test scores. These systems, fueled by vast datasets, can analyze a wide range of student interactions and behaviors, identifying patterns that suggest a student might be struggling with a particular concept or skill. It's notable that in some cases, these models can predict learning difficulties with a remarkable level of accuracy—up to 90% in certain studies. This gives educators a crucial head-start in intervening to support students before they experience a significant drop in performance.
The ability to go beyond just identifying academic challenges is also noteworthy. Predictive models can also start to uncover personal and socio-emotional factors that influence a student's ability to learn. These factors can sometimes be subtle and easily overlooked, but they can have a profound impact on a student's learning trajectory. By incorporating these insights, educators may be able to address the root cause of a student's difficulty, not just the immediate symptom.
It's the speed and real-time nature of predictive analytics that's truly transformative. Instead of educators having to wait for a test score or grade to understand where a student might be falling behind, these models can deliver alerts within minutes of detecting a potential learning gap. This kind of instant feedback changes the nature of educational intervention, allowing for far more proactive and timely support for students.
Predictive analytics also offers potential in the realm of student retention. By incorporating factors like student engagement into the model, educators can start to see early signs of disengagement or potential drop-out risk. This proactive approach can help identify students who may be at risk and allow for interventions that can help keep them engaged with their studies.
There's also growing evidence that these models can play a role in reducing educational disparities, especially for those student groups who have traditionally faced challenges in educational settings. By understanding individual learning patterns and offering tailored interventions, the hope is that predictive analytics can create a more equitable educational experience.
These systems can also be used to craft more customized assessments. Instead of a single standardized assessment that might not capture the diversity of student learning, these models can help create assessments tailored to an individual's specific needs and learning style. This approach could lead to a more accurate and fair way of evaluating student understanding.
It's not just about supporting the students, though. The insight provided by predictive analytics can empower learners themselves. By gaining a deeper understanding of their own learning process and areas where they struggle, students can become more active participants in their own educational journey. They can develop strategies for learning that capitalize on their strengths and weaknesses, increasing their self-efficacy and ownership of their education.
Interestingly, there's emerging evidence suggesting that students who benefit from these predictive insights can exhibit greater motivation and engagement. It's possible that the constant and personalized feedback they receive can help them feel more in control of their learning, encouraging a positive cycle of learning and improvement.
Moving beyond individuals, there's a hint that these analytics could also illuminate larger patterns in how groups of students learn. This knowledge could inform the design of more effective collaborative learning environments, potentially maximizing the benefits of peer interaction.
While the promise of predictive analytics in education is exciting, it's essential to acknowledge that it's not without potential drawbacks. As with any technology that handles personal data, ensuring student data privacy is of utmost importance. It's equally crucial to critically examine these systems for potential biases, as there's always the risk that the algorithms might unintentionally reinforce existing inequalities. Continued research and careful consideration of ethical implications are essential to ensure that these technologies are used responsibly and fairly, leading to a truly positive impact on educational outcomes.
AI-Driven Learning Analytics The Next Evolution in Personalized Education Environments for 2025 - Edge Computing Powers Offline Learning Analytics in Remote Educational Settings
In remote educational environments, consistent internet access isn't always a guarantee. This presents a challenge for AI-driven learning analytics, which often rely on constant connectivity to process data and provide real-time feedback. Edge computing offers a solution by bringing data processing closer to the point of data generation—in this case, the student's device. This approach allows for learning analytics to operate effectively even without a stable internet connection.
With edge computing, educational systems can deploy adaptive learning models that respond quickly to student needs and performance. This means that personalized learning experiences become possible even in areas with limited or unreliable internet infrastructure. Students can receive timely feedback and have their learning paths adjusted based on their individual progress, fostering more effective and engaging learning.
Beyond the practical benefits of offline analytics, edge computing enhances security and data privacy, concerns that are understandably at the forefront of discussions about AI in education. Processing data locally mitigates the need to transmit sensitive student data across networks, reducing the risk of unauthorized access or breaches.
However, as edge computing becomes more integrated into educational systems, it's crucial to examine how this approach can be implemented in a way that is both equitable and maximizes positive outcomes. There's a risk that if not handled carefully, the technology could further exacerbate existing inequalities in educational access or create new biases within the analytics themselves. A thoughtful and critical approach will be needed to navigate these complexities and fully realize the potential of edge computing to improve educational experiences for all students.
The integration of edge computing presents a particularly interesting solution for learning analytics in remote educational settings, particularly those lacking consistent internet access. By shifting data processing to devices at the edge of the network, learning analytics can operate effectively offline. This is quite a shift from the traditional reliance on cloud-based systems, which can struggle in areas with poor network connectivity. The ability to generate immediate insights from student interactions locally, regardless of internet availability, is one of the most promising aspects of this approach.
Furthermore, edge computing can significantly reduce the need for constant internet connectivity, making learning analytics more accessible in remote environments where bandwidth is often limited or expensive. This is a substantial benefit, especially in areas with unreliable or intermittent internet access. It's fascinating to consider how this approach could help address the digital divide in education.
Another important advantage is the potential for enhanced data privacy. Since student data is largely processed locally, the need to transmit sensitive information over potentially insecure networks is reduced. This is a crucial consideration in education, where student data is highly sensitive. However, it remains to be seen how effectively privacy can be guaranteed at the edge device level.
The adaptability of edge computing is also noteworthy. It allows educational systems to incorporate new learning technologies and analytic tools without requiring massive infrastructural upgrades. However, questions arise about the compatibility of diverse device hardware and the challenges of coordinating software and updates across a distributed edge network.
Edge computing further supports personalized learning in remote environments. AI models embedded in student devices can now provide localized adaptive learning experiences tailored to specific needs, strengths, and weaknesses. This approach offers the potential to bridge gaps that can arise due to a lack of resources or the inability to connect to centralized learning platforms. It is an exciting prospect, but also raises questions about equity of access to devices with sufficient computing power to support these features.
Edge-based insights also provide teachers with data to help them allocate resources effectively within the unique context of their class. By analyzing local performance data, they can tailor their teaching approaches more precisely and efficiently for students in their specific learning environment. We need to be cautious about potential biases in this localized approach and ensure the ethical implications are considered as such approaches are implemented.
Edge computing can even contribute to better understanding and management of students' cognitive loads. By analyzing interaction patterns locally, insights into the optimal balance of challenge and comprehension can be determined more effectively and efficiently. This ability to adapt learning activities to specific student needs, without waiting for cloud-based analysis, could prove quite beneficial in improving engagement and learning outcomes. However, more research is needed to understand how accurate these local assessments will be.
Perhaps one of the most impactful advantages is the increased resilience offered in remote environments with unstable internet access. Edge computing ensures that learning resources and analytics remain available even when connectivity is intermittent or disrupted. This fosters continuity and engagement in education, even in settings where infrastructural challenges often impede access to online resources. However, the responsibility of data integrity and updates must be carefully considered as it becomes increasingly decentralized.
The prospect of promoting collaborative learning experiences, even in remote areas, is quite promising. Through edge computing, students can share data and insights about their own learning paths with one another, fostering a sense of community and collective learning, even when they may be geographically isolated. This aspect could be a powerful tool for building educational communities, but further research is needed to explore best practices for facilitating collaborative learning in edge environments.
Overall, the emergence of edge computing in educational settings, especially those in remote locations, is a significant development. It opens the door to a more equitable and accessible educational experience that's capable of adapting to the unique demands of each learning environment. There are certain challenges associated with the implementation of edge computing in education, such as device diversity, data security, and governance of algorithms. Despite these challenges, the potential for improving education and educational equity is worth exploring further, ensuring a responsible and ethical approach to adoption.
AI-Driven Learning Analytics The Next Evolution in Personalized Education Environments for 2025 - Data Privacy Frameworks Balance Analytics Benefits With Student Information Protection
As AI-powered learning analytics become more prevalent in personalized education, robust data privacy frameworks are essential. These frameworks need to strike a balance between the powerful insights gleaned from student data—like improved learning outcomes and customized educational pathways—and the critical need for student information protection. The unique challenges and ethical dilemmas of data use within educational settings demand thoughtful consideration in these frameworks. The increasing reliance on predictive analytics and machine learning models highlights the importance of educators taking a proactive role in ensuring responsible and transparent practices. This includes safeguarding student privacy while realizing the potential of these technologies to personalize education. To pave the way for a future where these benefits are fully realized while respecting students' rights, we need a consistent and ongoing discussion on establishing standardized data protection measures in education, particularly as we approach 2025.
Within the realm of AI-powered education, the benefits of analytics – like predicting learning challenges and tailoring educational experiences – are undeniably appealing. However, the extensive collection and analysis of student data that fuels these systems necessitates a careful balancing act: ensuring student data is handled responsibly and ethically while maximizing the educational advantages. This delicate balance is further complicated by the lack of universally accepted standards for data privacy in education, leaving educators and institutions in a bit of a gray area until firmer regulations are established.
One key issue revolves around the potential for algorithmic bias in predictive analytics. If the data used to train these AI systems reflects historical educational inequalities, the algorithms may unintentionally perpetuate these biases, inadvertently leading to skewed resource allocation or support systems. This is a crucial point to monitor as these technologies evolve, as any bias in the system can have long-lasting and undesirable effects on student outcomes.
Further, ensuring meaningful informed consent from students and their families in the context of increasingly complex AI systems is a significant hurdle. It's not always straightforward for individuals to understand exactly how their data is being used or the implications for their privacy, which raises ethical questions about the transparency of these systems.
While data privacy frameworks are emerging, a false sense of security can arise, leading to complacency. Many data breaches aren't the result of sophisticated external hacking attempts but stem from human error or vulnerabilities in software. This underscores the ongoing need for vigilance and robust security measures beyond just adherence to broad regulations.
There are also interesting research questions concerning student motivation in the face of continuous monitoring. While personalized learning can be incredibly beneficial, the constant data collection can be perceived negatively by some learners, potentially creating undue pressure or hindering their independent exploration of learning. This impact on learner psychology is an important consideration as we design these systems for future use.
Another facet of the privacy dilemma is that of anonymization. While the practice is commonplace, it's increasingly evident that the advanced techniques of AI can potentially "de-anonymize" datasets, making it more challenging than ever to ensure the privacy of individuals. This necessitates revisiting the effectiveness of standard anonymization techniques and seeking more robust methods.
The uneven distribution of resources and technology across educational institutions is another concern. Wealthier schools have a better chance of adopting these sophisticated AI-driven analytics tools, creating a potential disparity between student experiences. This echoes a broader technological gap where some institutions may struggle to even implement basic data privacy measures, highlighting an urgent need for equitable access and support in this area.
Furthermore, the very definition of ethical guidelines and standards for AI in education is continuously evolving as technologies advance. Keeping pace with these changes, ensuring compliance, and establishing responsible governance structures is becoming increasingly complex for educational institutions.
Interestingly, this abundance of educational data also presents intriguing possibilities. These sophisticated AI systems can shed light on socio-emotional factors that influence learning, revealing real-time patterns often missed in traditional assessments. These insights can provide a more nuanced understanding of students' educational experiences beyond mere academic performance.
Lastly, we must contemplate how these changes will affect the role of educators. With the increased reliance on AI-driven analytics, the nature of teaching may shift from content delivery towards more of a facilitator role. Educators will need to develop new skills to interpret and act on the data these systems produce, all while remaining mindful of the delicate balance between maximizing student outcomes and protecting privacy. The coming years will be crucial in defining how this transformation plays out.
The future of personalized learning will rely on these complex issues being addressed proactively and thoughtfully. Navigating these challenges will be essential in order to unleash the full potential of AI to enhance education for all learners, in a way that is both beneficial and ethical.
More Posts from aitutorialmaker.com: