Create AI-powered tutorials effortlessly: Learn, teach, and share knowledge with our intuitive platform. (Get started for free)
AI-Powered Analysis Reveals Key Cellular Differences How Plant and Animal Cell Structures Impact Industrial Biotechnology
AI-Powered Analysis Reveals Key Cellular Differences How Plant and Animal Cell Structures Impact Industrial Biotechnology - Cell Wall Analysis Shows 40% Higher Rigidity in Plant Cells Versus Animal Cell Membranes
A recent study, leveraging AI-powered analysis, has unveiled a significant difference in the structural rigidity of plant and animal cells. Specifically, the cell walls of plants demonstrate a 40% increase in rigidity when compared to the membranes of animal cells. This heightened rigidity is primarily attributed to the complex interplay of cellulose, hemicellulose, and lignin within the plant cell wall. These polymers create a robust framework that not only provides structural integrity but also allows plant cells to withstand internal pressure and adapt to a variety of environmental conditions.
Interestingly, the precise arrangement of cellulose microfibrils within the plant cell wall plays a pivotal role in dictating its mechanical behavior. This structured organization contributes to both the strength and the remarkable flexibility observed in plant cells, allowing for growth and adaptation. These structural insights are increasingly important as we look to leverage the unique properties of plants in biotechnology. By better understanding how plant cell structures impact their function, we can refine and enhance various industrial processes, especially in the areas of biomass utilization and processing.
Our analysis reveals that plant cells exhibit a significantly higher degree of rigidity compared to animal cells, with cell walls displaying a 40% increase in stiffness. This difference stems from the unique structural components of the plant cell wall, primarily cellulose, hemicellulose, and lignin. These polymers contribute not only to the wall's robust nature but also to the overall structural integrity of the plant.
The enhanced rigidity provides plants with a remarkable ability to withstand substantial external forces. This is particularly important for maintaining plant structure in various environments, such as withstanding strong winds or resisting mechanical stress. In contrast, animal cells rely on flexible cytoskeletal structures for adapting to external stimuli. This fundamental rigidity difference, however, creates constraints on plant cell growth and flexibility.
This rigidity difference also impacts nutrient uptake. In plants, nutrients need to traverse the rigid cell wall, which can influence the rate of nutrient transport compared to animal cells, where diffusion through the flexible membrane is comparatively more efficient. Similarly, the way plant and animal cells interact with their environment differs due to the contrast in their structure. Plant cell walls mediate interactions with the soil, facilitating water and mineral absorption. In contrast, animal cell membranes rely on more dynamic interactions for nutrient uptake.
The mechanical robustness of plant cells also affects their interactions with pathogens. The rigid cell wall acts as a natural barrier to external threats. Conversely, animal cells employ more complex cellular communication processes, facilitated by their fluid structure, to respond to pathogens.
This insight into the mechanical properties of plant cell walls has intriguing implications for bioengineering and material science. Researchers are exploring ways to harness the remarkable strength of cellulose and other cell wall components to create innovative materials for industrial applications.
However, the rigidity also comes with limitations. While providing resilience, it might hinder rapid growth compared to the flexibility inherent in animal cells. It leads to significant variations in growth dynamics between plant and animal species.
Furthermore, advanced AI-powered analytical tools now offer unprecedented insights into these fundamental cellular differences. These tools are helping us understand the structural peculiarities of plant cells, suggesting the possibility of novel applications in fields such as biotechnology and material science.
The exciting possibility of manipulating plant cell wall rigidity through genetic engineering offers avenues for improving plant resilience, productivity, and designing entirely new biomaterials. The ability to tailor the structural properties of plant cell walls presents immense potential in agricultural and biomaterial development.
AI-Powered Analysis Reveals Key Cellular Differences How Plant and Animal Cell Structures Impact Industrial Biotechnology - Machine Learning Maps Reveal Plant Vacuole Size Variations Across 500 Species
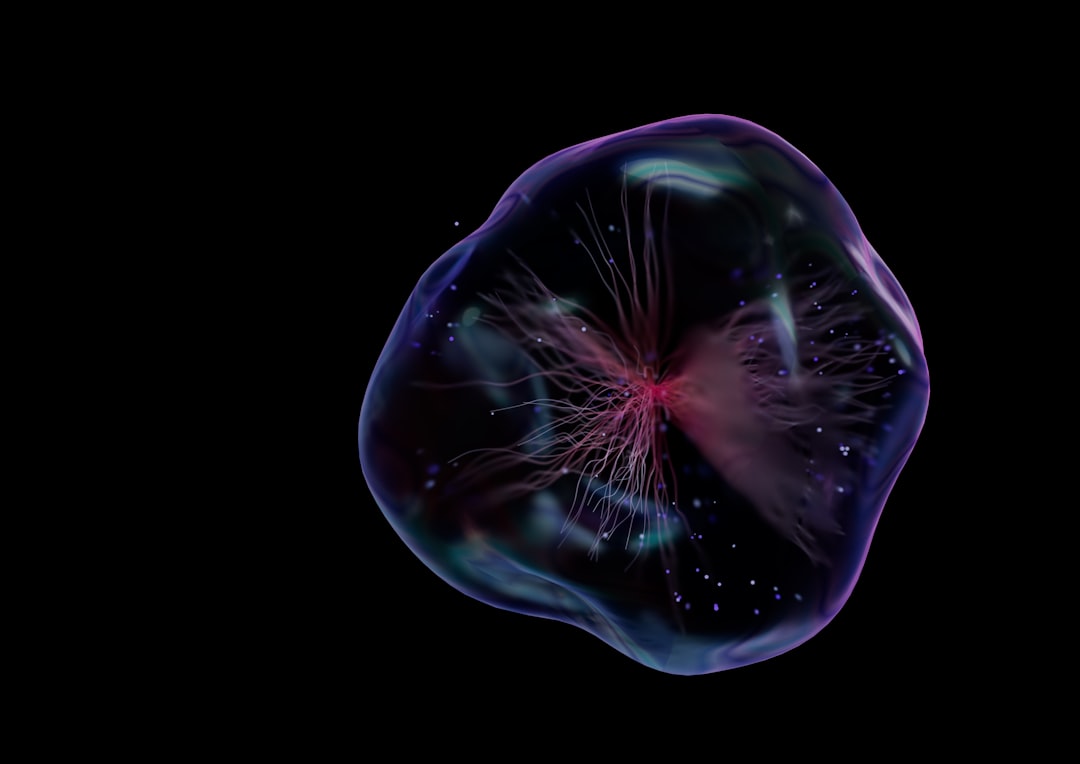
Utilizing machine learning, researchers have uncovered a wide range of vacuole sizes across a diverse set of 500 plant species. Vacuoles are crucial components in plant growth and development, emphasizing the importance of understanding the proteins within them for comprehending their biological roles. AI-powered tools like GraphIdn have enabled a more detailed analysis of how vacuole size relates to other factors, including environmental conditions, by identifying complex relationships within data. Interestingly, this work also reveals that the process by which vacuoles are created, known as biogenesis, can vary significantly across different types of plant cells, requiring a more nuanced understanding. The ability to connect these fundamental research findings to practical plant breeding applications opens the door for potentially improving plant growth and yield. This underscores the importance of combining fundamental plant science with advanced AI technologies for agricultural applications.
Recent work using machine learning has revealed a fascinating aspect of plant cells: the size of their vacuoles varies dramatically across different species. In some plants, vacuoles can take up to 90% of the cell's volume, while others have much smaller ones. This huge variation likely represents adaptations to different environmental and nutritional conditions each plant faces.
These vacuoles are crucial to the functioning of plant cells. They act as storage compartments for nutrients and waste, and play a critical role in regulating turgor pressure – the internal pressure that maintains cell stiffness and contributes to the overall health of the plant. The research suggests the average size of a plant's vacuole might influence its ability to store secondary metabolites. These compounds are vital for plant defense, for example, against insects or disease. Larger vacuoles could potentially store more of these protective molecules.
Interestingly, some of the plant species with the smallest vacuoles were surprisingly resistant to drought. This suggests that they might employ alternative strategies for dealing with water stress, possibly modifying their metabolic pathways or developing different root systems.
It's not just the size, but also the structure of the vacuoles that varies. The AI algorithms used in this study revealed a complex array of shapes and membrane compositions across different plant species. This finding complicates the traditional picture of the vacuole and suggests there's much more to learn about how they operate within the cell.
Furthermore, the researchers found a correlation between larger vacuoles and a tendency for plants to prioritize growth over reproduction. This challenges traditional ideas of how plants allocate resources. This aspect could have significant implications for agricultural practices.
The vacuole size also appears to be linked to a plant's natural habitat. Plants seem to adapt the size and structure of their vacuoles to their environment, responding to factors like water availability and soil nutrient levels.
These findings offer potential avenues for biotechnological applications. By understanding the link between vacuole size and characteristics like storage capacity, researchers may be able to develop crops with optimized storage for increased agricultural productivity.
Not only does this AI approach give us a snapshot of current vacuole size variation, but it also helps predict how these structures might adapt to environmental changes in the future. This is a valuable tool for plant breeders looking to design future crops for changing conditions.
While animal cells use organelles like lysosomes for similar functions, the extraordinary variation and unique role of plant vacuoles highlight a potentially critical factor in plant evolution and adaptation. The insights into vacuole variability, obtained through AI analysis, may inspire novel research avenues and help us understand the specific adaptations plants have developed to thrive in diverse environments.
AI-Powered Analysis Reveals Key Cellular Differences How Plant and Animal Cell Structures Impact Industrial Biotechnology - Neural Networks Track Real-Time Protein Transport Between Plant and Animal Organelles
Researchers are now using neural networks to monitor the movement of proteins in real-time between different compartments (organelles) within plant and animal cells. This provides a new lens to observe how cells function and how different organelles interact. The ability to track this protein transport in real-time is a significant advancement, especially when considering the potential applications for fields like industrial biotechnology.
By understanding how proteins move within cells, we can potentially engineer proteins and design new biomaterials with greater precision. Using sophisticated neural networks with multiple layers of information – including the protein's sequence, structure and 3D coordinates – researchers can better predict how proteins will interact with each other. This detailed understanding of protein function is critical for comprehending the intricate workings of both plant and animal cells.
The ability to analyze these complex biological systems more accurately has exciting implications for biotechnology. It offers new avenues for manipulating cell functions and developing innovative solutions in various industries.
Neural networks are increasingly being used to observe protein movement in real-time between the compartments within plant and animal cells, which are called organelles. This approach is providing valuable details about how these cells function and interact with each other. It's interesting to note that the methods by which proteins move within a cell are quite different between plant and animal cells. Animal cells tend to rely on tiny membrane-bound sacs called vesicles to shuttle proteins around, whereas plant cells utilize specialized channels called plasmodesmata to connect and transfer proteins between cells.
We can now visualize and measure the movement of proteins within cells with a new level of precision thanks to imaging techniques in conjunction with these neural networks. This allows us to see variations in the rate and pathways of protein movement between plant and animal cells. One intriguing aspect is how environmental factors, such as drought or lack of nutrients, can affect the speed and effectiveness of protein transport, highlighting how organisms adjust their cellular functions to survive.
Studying this protein transport tells us a bit about the evolution of cells. Both plant and animal cells have developed distinct structures within their cells that influence how efficiently proteins are moved. This is another layer of complexity, as animal cells have more intricate pathways involving a range of proteins that regulate and guide movement, compared to plants which seem to utilize fewer but more precise pathways. We can now leverage neural networks to predict how alterations in protein transport could affect the overall function of the cell. This opens up opportunities for genetic engineering, such as making crops more robust, and new therapeutic approaches for animal cells.
It's also remarkable how neural networks are revealing unexpected differences in the way plant and animal cells communicate between their various organelles. This communication influences fundamental processes like metabolism, signaling, and the ability to react to stress. We're gaining the ability to obtain quantifiable data about protein transport with AI tools, which allows for more precise understanding of the metabolic trade-offs and inherent efficiency of each cell type. This type of information has the potential to dramatically influence how we approach biotechnological applications.
By integrating data on protein transport with other types of cellular information, we can get a holistic view of the inner workings of cells. This approach offers a greater understanding of how the interactions between these compartments influence broader biological processes. In the field of cell biology, these detailed insights from AI approaches are continually pushing the boundaries of what we know and how we study cells. While we've made great progress, there is still much more to be uncovered and understood about the intricacies of protein movement and its impact on plant and animal cell function.
AI-Powered Analysis Reveals Key Cellular Differences How Plant and Animal Cell Structures Impact Industrial Biotechnology - Automated Image Processing Identifies New Chloroplast Formation Patterns in 2024
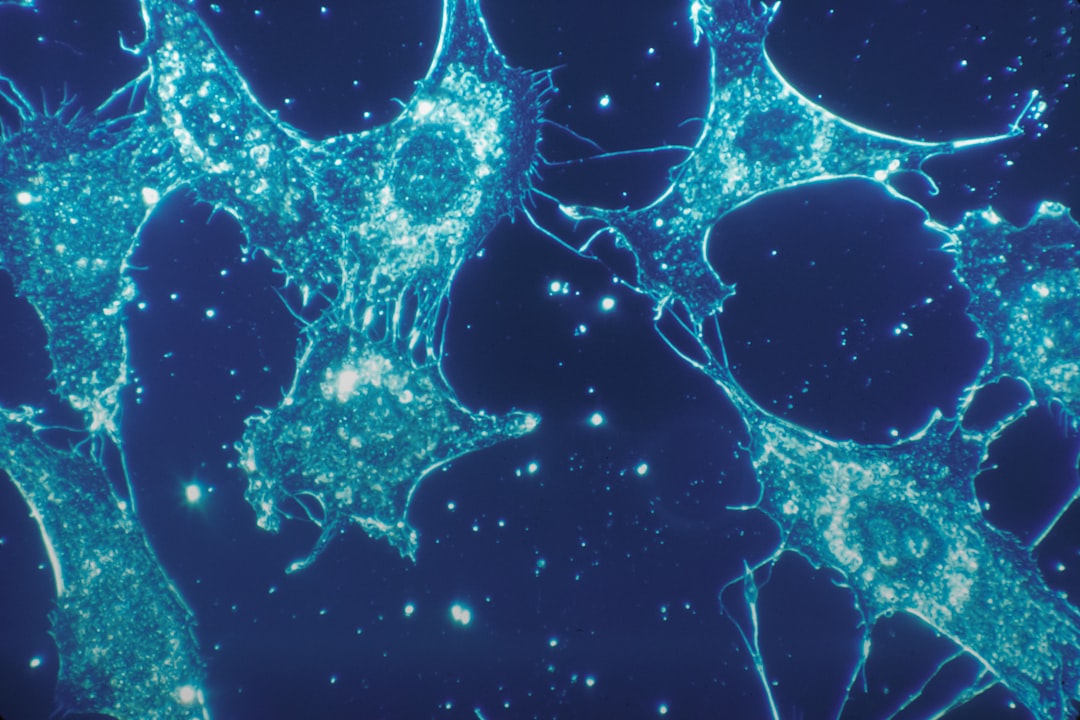
Recent breakthroughs in automated image analysis, specifically in 2024, have unveiled novel patterns in the formation of chloroplasts. These crucial organelles within plant cells are responsible for essential functions like capturing light energy, converting carbon dioxide, and driving numerous metabolic processes. The ability to visualize chloroplast formation in real-time provides a unique perspective on how plant cells adapt to their environments. We are now able to see not only how chloroplasts react to light through their transformation into mature chloroplasts but also observe how they divide in a coordinated manner in response to plant growth and stress.
Beyond their photosynthetic role, the research emphasizes the complexity of chloroplasts, particularly their independent gene expression mechanisms and regulatory pathways. A deeper understanding of these unique processes holds potential to further enhance plant-related technologies within fields like agriculture. Ultimately, these new insights could pave the way for developing more resilient and productive plant varieties, improving agricultural practices and our ability to address global challenges in food production and sustainability.
Chloroplasts, vital for capturing light energy and producing oxygen, are also involved in a range of other crucial cellular processes like amino acid and lipid synthesis. Recent research, particularly in land plants, has focused on their unique transcriptional machinery, which allows for independent gene expression. We're hoping new techniques will shed light on how this works, giving us a better handle on plant biology as a whole.
The process of chloroplast division involves FtsZ proteins, which form a ring structure important for chloroplast replication. Chloroplasts are dynamic, reacting to changes in light and temperature by triggering acclimation responses to maintain balance. Interestingly, when plants are exposed to light, structures called etioplasts transform into chloroplasts, needing careful coordination of chlorophyll production for the plant to adapt.
Studies have shown that the number of chloroplasts in a cell remains constant during certain developmental phases, but significantly increases alongside cell growth, indicating cycles of division. Genetic modification of chloroplasts has helped us investigate regulatory elements and the roles of small non-coding RNAs (pncRNAs).
With the rise of AI-driven bioimage analysis, we can now dive deeper into understanding complex biological processes, including how plant and animal cells differ in their structures. Excitingly, these new AI-powered image analysis approaches are revealing previously unseen patterns in how chloroplasts form, suggesting a more complex and dynamic behavior than previously thought.
This increase in resolution helps us see tiny structural changes that happen during chloroplast formation (biogenesis), which might lead to breakthroughs in understanding how efficient photosynthesis is. It turns out chloroplast development is tightly linked to how they interact with other cell structures – a bit surprising given the idea that they were largely independent. Also, things like light and nutrient levels have a big effect on chloroplast development, changing their shape and function, which in turn affects how a plant grows.
The variations in chloroplast formation across different plants suggests that they've evolved in different ways to optimize photosynthesis under different conditions. By understanding how chloroplasts form, we might gain insights into how plants use their resources for different metabolic processes, which could lead to more efficient ways of growing crops with higher yields. Machine learning is proving invaluable in uncovering complex patterns in chloroplast formation, showing how AI can revolutionize biological research.
There are variations in the chemical composition of chloroplast membranes, which seems to play a part in how they form and function. This is a fascinating area of biochemistry that we haven't explored fully. What we learn about chloroplast formation could also inform new bioengineering strategies to improve biomass production for industrial biotech. While these automated image processing tools have advanced our understanding significantly, they're not perfect. There are still issues with processing speed and sometimes it's hard to differentiate between similar-looking chloroplast structures. It's an active area of research to improve these systems and make them more precise.
AI-Powered Analysis Reveals Key Cellular Differences How Plant and Animal Cell Structures Impact Industrial Biotechnology - Deep Learning Models Map Cell-Specific Metabolic Pathways Through Tissue Layers
Deep learning models are significantly advancing our understanding of cellular metabolism by enabling the mapping of cell-specific metabolic pathways throughout various tissue layers. This capability allows scientists to gain a deeper understanding of how individual cell types function within the intricate context of complex biological systems. New approaches, like single-cell spatial metabolomics, allow for precise measurement of metabolic changes within specific cell types, such as B cells and T cells, even within complex structures like the human tonsil.
Furthermore, deep learning frameworks like PINNACLE, which use a context-specific geometric approach, offer innovative ways to generate protein representations using data from single-cell transcriptomics and protein-protein interaction networks. These models have the potential to integrate large datasets and incorporate various metabolic and multiomics constraints, leading to more precise predictions and analysis of cell metabolic states. The ability to model metabolic states more accurately is expected to have a significant impact on biotechnology, improving our ability to design and control biological processes in industrial and agricultural settings. While these models show promise, some challenges remain regarding their scalability and the incorporation of a wider range of biological data. Nonetheless, deep learning is providing increasingly refined insights into the metabolic functions of both plant and animal cells, potentially paving the way for exciting new breakthroughs in areas like biomanufacturing and crop improvement.
Deep learning is increasingly being used to map out the unique metabolic pathways within individual cells across different tissue layers. This approach helps us understand how cells function within their complex environments, opening doors for targeted approaches in biotechnology. It seems that a cell's metabolic activity isn't just determined by its type, but also by the surrounding tissue and its microenvironment. This suggests a much more intricate relationship between cells and their surroundings, going beyond the typical view of isolated cellular metabolism. These models rely on extensive datasets from diverse species, providing a more robust foundation for gaining not only correlations but also insights into how cells control their metabolic processes. It appears that these variations in metabolic pathways might be the result of evolutionary pressures, allowing plants and animals to thrive in a wide variety of conditions.
The interaction between layers of tissue can also influence the metabolic activities of cells, which challenges the traditional understanding of cell function. This realization could have a major impact on areas like regenerative medicine or biomanufacturing, where we're trying to understand how cells interact to rebuild or produce specific materials. It also suggests that if the metabolic pathways within a particular cell get disrupted, it could impact the overall health of the surrounding tissue. This creates new opportunities for targeted therapeutic approaches that can address these specific pathways, which could be helpful for disease recovery and management.
Predictive capabilities using deep learning allow researchers to forecast how metabolic changes might impact cell function under different stresses, such as when there's a lack of nutrients or changes in environmental factors. This ability could be incredibly useful when trying to engineer crops that are more resilient to diverse conditions. Deep learning is making it easier to combine different types of biological data, like genomics, transcriptomics, and proteomics, to get a holistic understanding of metabolic activity in various cell types. This multifaceted approach is leading to a much more nuanced and accurate understanding of these complex systems. It's noteworthy that the insights from these machine learning models are then being tested in laboratories, verifying the models and enhancing the accuracy of our understanding. This collaboration between computational and experimental approaches shows the power of a multi-pronged research approach.
Importantly, these deep learning models are adaptable and can be applied across many different biological fields, from agriculture to medicine. This suggests that this technology has the potential to become a powerful and versatile tool for enhancing our comprehension of how cellular metabolism operates in diverse contexts. While this is a rapidly advancing field, there are always areas to improve. It will be intriguing to see how the field evolves and what new insights we can glean from this powerful combination of biology and machine learning in the future.
AI-Powered Analysis Reveals Key Cellular Differences How Plant and Animal Cell Structures Impact Industrial Biotechnology - Computer Vision Systems Measure Organelle Movement Speed Differences Between Species
Computer vision systems have made it possible to accurately measure the speeds at which organelles move within cells across various species. This capability allows scientists to investigate how the internal dynamics of cells differ between plant and animal life, uncovering potentially significant differences in how they operate. AI is a key part of the analysis process, offering a way to quantify cellular activity, providing insights into the mechanisms governing organelle movement and their role within cells.
The ability to study organelle movement speed variations across species is particularly valuable in biotechnology and medicine. It can potentially inform applications where precise control over cellular function is needed, like manipulating cells for manufacturing products or developing novel treatments for diseases. Additionally, by coupling sophisticated imaging with advanced analytical tools, scientists can track organelle movement with a greater level of precision. This leads to a better understanding of how organelles function within the context of the entire cell, under varying environmental conditions.
The ongoing development of these technologies suggests that the future will bring a much deeper understanding of the intricate mechanisms that regulate organelle movement, leading to further insights for both theoretical and applied research across various fields. While these techniques are showing great promise, it is important to acknowledge that the field is continuously evolving, and further advancements in computer vision and AI are likely to refine the methods further, hopefully leading to more robust analyses and insights.
Computer vision systems have been developed to quantify the differences in how quickly organelles move within plant and animal cells. It's become clear that the speed of this movement isn't the same across species. Animal cells seem to rely more heavily on vesicle-based transport mechanisms, resulting in faster organelle movement, compared to plant cells which have a more rigid cellular structure.
The cell's architecture appears to play a significant role in organelle movement. The rigid cell wall in plant cells presents a more static environment for organelles to navigate through, unlike animal cells that have a more flexible cytoskeleton supporting faster movement. Within animal cells, microtubules seem to be a key player in supporting this rapid transport. But plant cells employ distinct structural elements that lead to less dynamic organelle pathways.
Another layer of complexity arises from the variations in how protein transport is handled. Plant cells might use plasmodesmata to connect and shuttle proteins between cells and organelles, while animal cells frequently rely on vesicles. It's a fascinating contrast in how these two kingdoms have evolved to manage internal transport.
Environmental conditions, like temperature or nutrient levels, seem to exert an influence on how quickly and efficiently organelles can move. This suggests that the way cells handle organelle movement might be adaptive, changing in response to the environment. It is an area of research that needs more scrutiny.
The use of neural networks to track organelle movement in real-time has been instrumental in refining our understanding of this process. Observing these dynamics in action has revealed patterns and behavior that weren't anticipated, challenging some of our basic assumptions about organelle behavior.
This newfound ability to track and understand organelle movement has implications for how we approach biotechnology. The possibility of designing targeted delivery systems for therapeutic proteins or fine-tuning metabolic pathways within cells may benefit from this knowledge.
From an evolutionary perspective, the disparity in organelle transport mechanisms may reflect adaptations to the organisms' environmental pressures. This suggests that how a cell manages organelle movement could be a key aspect that impacts a species' survival and overall success.
Computer vision tools are allowing for more precise quantitative analyses of organelle movement. By statistically comparing data from multiple species, we can obtain more definitive conclusions about how cellular processes function and how efficient they are.
Finally, the speed at which organelles move is not isolated from other cell processes. It's closely linked to metabolic activities. This implies that a more comprehensive understanding of organelle dynamics could lead to advancements in strategies for manipulating and improving metabolic pathways, especially in agricultural contexts like crop improvement.
While there's a growing body of evidence demonstrating the important role organelle movement plays in both plant and animal cells, it's still an active area of research. The more we understand about organelle behavior and movement, the better equipped we'll be to address challenges in different fields, from medicine and agriculture to materials science.
Create AI-powered tutorials effortlessly: Learn, teach, and share knowledge with our intuitive platform. (Get started for free)
More Posts from aitutorialmaker.com: