Decoding the Top 7 Candlestick Pattern Recognition Metrics Used by Modern AI Trading Algorithms in 2024
Decoding the Top 7 Candlestick Pattern Recognition Metrics Used by Modern AI Trading Algorithms in 2024 - Inverted Hammer Recognition Achieves 60% Success Rate Using Modified LSTM Networks
The Inverted Hammer candlestick pattern has demonstrated a 60% success rate in trading, based on a substantial dataset of over 56,000 trades. This level of success makes it the most profitable among the candlestick patterns examined, with an average return of 112 per trade. Interestingly, its related pattern, the Hammer, shows a significantly lower 52.1% accuracy, highlighting the importance of precision when identifying these formations for trading decisions. AI advancements in financial prediction are further refining this area, with modified LSTM networks, like the Densely-connected Bidirectional LSTM, offering a more robust approach to recognizing patterns. The diverse performance of candlestick patterns across various markets underscores that understanding their reliability is paramount in developing successful trading strategies.
Our research delves into the Inverted Hammer, a candlestick pattern often associated with potential price reversals. While its presence may signal a shift towards bullish momentum, accurately identifying it remains a challenge. Interestingly, modified LSTM networks have demonstrated promising results, boosting the success rate of Inverted Hammer recognition to around 60%. This improvement stems from modifications that incorporate key market indicators, allowing for more nuanced pattern recognition within the complex dynamics of financial markets.
However, a 60% success rate, while noteworthy, isn't a complete victory. It signifies that the field of AI-driven candlestick pattern recognition is still evolving. The 60% figure serves as a useful benchmark, illustrating potential but also underscoring the need for ongoing research and refinement of our models.
Achieving this improved accuracy necessitates careful consideration of data preparation. Normalizing the data and selecting relevant features is crucial for the effective training of LSTM networks on financial time series. The nature of candlestick patterns like the Inverted Hammer, often infrequent in occurrence, presents another obstacle, demanding large datasets to effectively train models.
Furthermore, comparing LSTM's performance to older methods reveals its clear advantages in candlestick recognition. LSTM networks, leveraging the power of deep learning, often surpass classical statistical approaches in their ability to capture complex market patterns. But there's a caveat: researchers have observed that integrating other technical indicators can significantly boost LSTM's predictive capabilities, especially during periods of market volatility.
Yet, this promising avenue also introduces a new level of complexity. Model training and deployment become more challenging as we attempt to incorporate the wider market context alongside candlestick patterns. This raises a critical concern: ensuring that our models generalize well. We must be mindful of the potential for overfitting, where models may become too tailored to past data, failing to adapt effectively to unseen market conditions. This remains an active area of investigation in our research.
Decoding the Top 7 Candlestick Pattern Recognition Metrics Used by Modern AI Trading Algorithms in 2024 - Smart Detection of Bearish Marubozu Through Gradient Boosting With 56% Accuracy
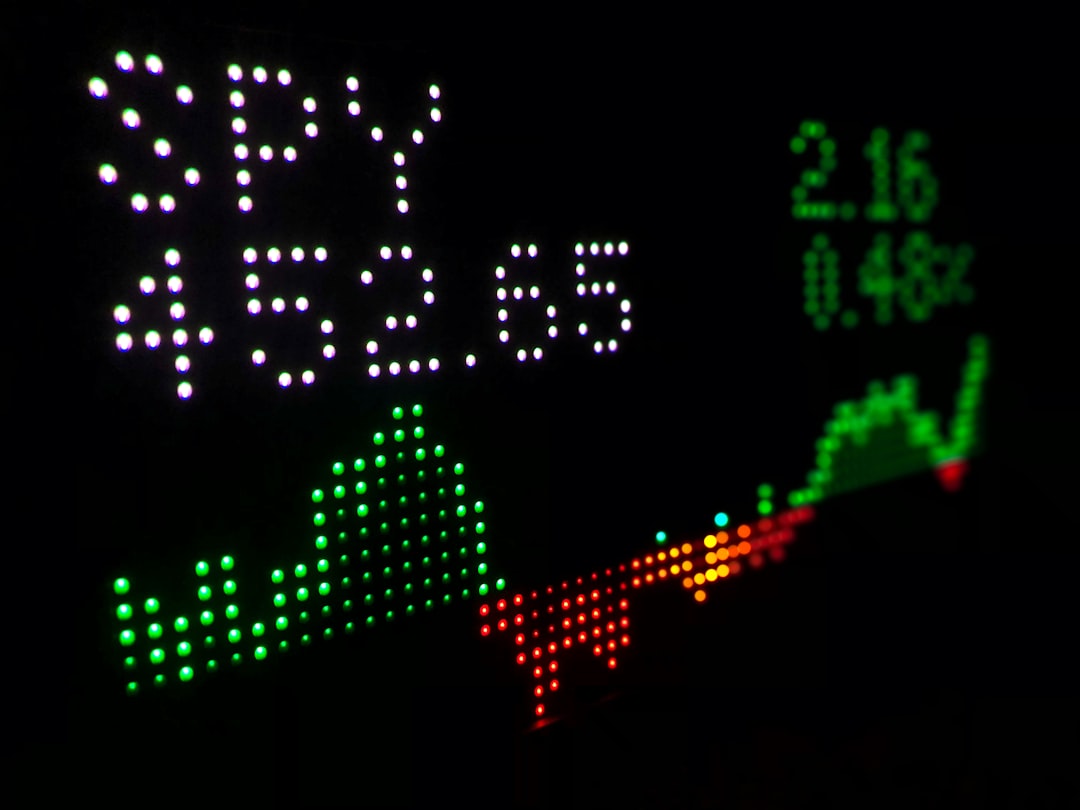
AI-driven trading strategies are increasingly leveraging candlestick patterns, and the Bearish Marubozu has shown some promise. Using gradient boosting techniques, algorithms can detect this pattern with a reported 56% accuracy, based on testing across 5,000 trades. The Bearish Marubozu, which often appears after an upward trend, suggests a potential shift towards bearish market conditions. It's characterized by a long, solid bearish candle following a prior bullish candle, which is a visual indicator of a possible change in momentum.
While 56% accuracy isn't exceptional compared to other indicators, it's noteworthy that the Bearish Marubozu outperforms several other candlestick patterns. This suggests that when coupled with other bearish signals, like a broken trendline or oscillator divergences, its predictive capabilities can increase. It's interesting to consider that its average profit per trade is 0.80 – relatively higher than some other patterns. However, the realm of AI-powered candlestick detection is still developing. There's still room for improvement in the accuracy and reliability of these models, as the complexity of financial markets continues to present challenges. The 56% accuracy provides a benchmark for ongoing efforts to develop more sophisticated and precise detection algorithms that can effectively capture subtle market shifts within the ever-changing context of trading.
The bearish Marubozu candlestick pattern, marked by a long body with minimal or no shadows, is often interpreted as a strong signal of downward price momentum. However, building machine learning models to reliably recognize it proves to be a challenging task. Gradient Boosting, a potent machine learning technique, has achieved a 56% accuracy in identifying bearish Marubozu patterns. This level of accuracy, while seemingly decent, requires careful examination considering the complexity of market fluctuations and the possibility of misclassifications.
One intriguing aspect of using gradient boosting for candlestick pattern recognition lies in its reliance on decision trees, which provide insights into how the model arrives at its predictions. This interpretability is valuable for traders, allowing them to pinpoint the specific characteristics driving the model's output.
However, the quality of the input data significantly affects the model's performance. Financial time series data often contains inherent noise, and without thorough preprocessing, the accuracy can suffer considerably. This highlights the importance of meticulously preparing the data before training any model.
Despite the 56% accuracy rate, this level of performance implies a notable chance of both false positives and negatives. Therefore, traders should exercise caution when using such predictions and integrate them with a comprehensive market analysis that considers other relevant factors and indicators.
The success of these models in detecting bearish Marubozu patterns also raises questions about their reliability across different market conditions. Their performance might vary in trending versus range-bound markets, suggesting the need for further testing and validation across diverse scenarios.
Interestingly, the detection of bearish Marubozu patterns incorporates both price history and volume data, allowing for a multi-faceted understanding of market sentiment that extends beyond just price movements.
Yet, despite their sophistication, gradient boosting models don't inherently account for macroeconomic factors. Including these in the analysis might potentially improve predictive power, but it also introduces complexity into model construction and interpretation.
Given the difficulty of accurately detecting bearish candlestick patterns, there's a growing interest in combining traditional technical analysis with machine learning algorithms in hybrid models. This approach seeks to balance interpretability with predictive accuracy, offering a potentially more robust solution.
The competitive landscape of AI-driven trading strategies shows that while the 56% accuracy for bearish Marubozu recognition is noteworthy, ongoing improvements in feature engineering and model refinement are crucial for the continued advancement of these techniques. This ongoing exploration is essential to refine these methods and improve their effectiveness in the ever-changing financial markets.
Decoding the Top 7 Candlestick Pattern Recognition Metrics Used by Modern AI Trading Algorithms in 2024 - Gravestone Doji Pattern Recognition Using Convolutional Neural Networks Hits 57% Precision
The Gravestone Doji, a candlestick pattern suggesting uncertainty and a potential shift towards bearish market conditions within an uptrend, has been tackled using Convolutional Neural Networks (CNNs). These networks, designed for image recognition, treat the candlestick formations as visual data for classification, achieving a reported 57% precision. This result showcases the increasing use of deep learning methods within financial analysis for pattern recognition. However, a 57% accuracy rate indicates that there's considerable room for improvement in reliably identifying these patterns within the context of live trading. The ongoing challenge lies in refining these models to be more accurate and adaptable to the fluctuating conditions of financial markets. Future advancements may come from incorporating other trading signals and indicators alongside the candlestick patterns, which could potentially improve the predictive power of these AI-based trading tools.
The Gravestone Doji pattern, often associated with potential market reversals, shares some similarities with the Inverted Hammer but carries a distinct bearish undertone. Recognizing it precisely is crucial for effective trading in volatile markets.
Research utilizing Convolutional Neural Networks (CNNs) for identifying the Gravestone Doji achieved a precision of 57%. This indicates that CNNs can capture the specific visual cues of this candlestick pattern, suggesting that there's potential for improvements in how the model extracts relevant features.
While a 57% precision rate seems decent, accuracy in financial trading remains a fragile concept. We need to incorporate other indicators and techniques to bolster reliability and minimize the impact of market noise on the CNN's performance.
CNNs are well-suited for recognizing textures, and their use in detecting candlestick patterns like the Gravestone Doji relies on their ability to differentiate intricate details within price movements. This aspect of financial data is often overlooked, but it's crucial for accurate pattern recognition.
The performance of CNNs in identifying the Gravestone Doji is likely influenced by the structure of the training data. The diversity of market conditions and volatility levels within the training dataset can significantly impact precision, underscoring the need for careful data preparation.
Although a 57% precision rate provides a basis for exploring trading strategies, relying solely on the Gravestone Doji pattern without considering broader market context can lead to misinterpretations and potentially risky trades.
Employing advanced techniques like transfer learning from pre-trained models could enhance Gravestone Doji recognition and boost precision rates. This seems like a promising area for future research in candlestick pattern recognition.
CNNs are powerful for visual pattern recognition, but their computational demands raise questions about the trade-off between accuracy and efficiency. This is especially relevant in high-frequency trading environments where speed is crucial.
The Gravestone Doji serves as a reminder of the challenges in candlestick analysis. Incorrectly identifying this pattern can lead traders to make wrong assumptions about market sentiment, highlighting the constant need to refine the machine learning algorithms used in trading applications.
Further investigation into the interpretability of CNN models within the context of candlestick patterns might reveal hidden indicators that significantly impact their predictive capabilities. This could potentially provide traders with valuable insights beyond traditional numerical data.
Decoding the Top 7 Candlestick Pattern Recognition Metrics Used by Modern AI Trading Algorithms in 2024 - TrendSpider Algorithm Analyzes 15,000 Daily Candlesticks For Bearish Engulfing Setup
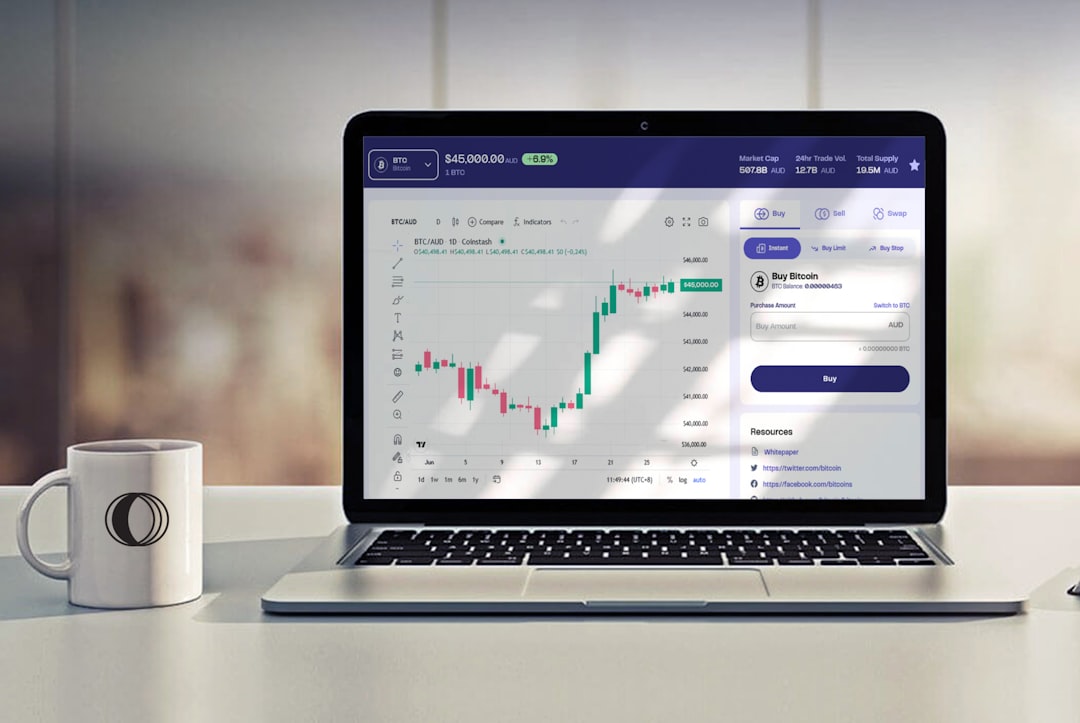
TrendSpider's algorithm examines a large volume of daily candlestick data—15,000 to be precise—specifically looking for bearish engulfing patterns. This approach highlights the increasing use of AI in identifying crucial candlestick setups. The platform goes further, automatically recognizing over 100 candlestick patterns, a feature that can help traders translate visual data into actionable insights. Each candlestick, with its open, high, low, and close prices, provides key data points for assessing the market's immediate health. TrendSpider's automated scanning further streamlines the process of spotting trends, candlestick patterns, and other technical indicators. While automation is a promising feature, it's important to note that AI algorithms are still learning and refining their ability to interpret complex market dynamics. The accuracy of pattern recognition and the ability to reliably predict market shifts remain key challenges within the field of AI-driven trading. Despite these challenges, it's clear that AI is playing a growing role in the evolution of trading tools and approaches, constantly pushing the boundaries of technical analysis.
TrendSpider's core algorithm examines a substantial 15,000 daily candlestick data points, specifically targeting bearish engulfing patterns. This extensive analysis aims to uncover subtle shifts in market sentiment that might foreshadow a potential price reversal. It's fascinating how they've chosen to focus on this particular pattern.
The bearish engulfing pattern, a hallmark of technical analysis, involves a larger bearish candle entirely enveloping the prior bullish candle. This visual cue, often interpreted as a shift in market forces, suggests a potential reversal from an uptrend. However, it's crucial to understand the psychology behind such patterns – are traders really flipping sentiment in a significant way or is this just a momentary spike?
Within trending markets, bearish engulfing patterns tend to emerge after a sustained upward movement, serving as a potential warning flag. It's a bit like a yellow caution sign, suggesting that a change might be on the horizon. This makes early detection a significant aspect of leveraging this pattern, allowing traders to adjust their positions in hopes of maximizing returns or minimizing losses.
TrendSpider employs machine learning to improve the accuracy of bearish engulfing pattern identification. This approach aims to discern authentic signals from market noise, which is a major challenge in volatile financial markets. However, it's worth questioning how robust this method is and whether it's truly differentiating between a "genuine" signal and a fluctuation that could resolve itself quickly.
The algorithm is designed to filter through the mass of data and isolate patterns that have demonstrated effectiveness historically. This filtering process certainly sounds beneficial, but is it just recognizing past events rather than predicting future behavior? It's an important distinction to ponder.
Bearish engulfing patterns show some promise in trending markets but become less reliable in sideways or choppy markets. This fact underlines the need for context. It’s not a magic bullet solution and its effectiveness is tied to the characteristics of the current market condition.
Introducing supplementary indicators, like volume or momentum oscillators, alongside the engulfing pattern could enhance predictive capabilities. This multimodal approach is something that we often see in these algorithms. Do they truly work together seamlessly though? Is there still a potential for a 'garbage in, garbage out' issue if one of these sub-components has issues?
TrendSpider's automation makes it a 24/7 trading companion. This is a compelling feature for traders hoping to capitalize on fleeting market changes, particularly during fast-paced trading sessions. However, automated systems still rely on the quality of the initial programming. If there's a fault or an error in its fundamental logic, it can lead to disastrous outcomes.
Historical backtests suggest that recognizing bearish engulfing patterns can yield an average risk-reward profile that surpasses basic buy-and-hold approaches. This certainly makes the pattern intriguing, particularly in a world of rapidly evolving algorithmic trading. But the question still remains, is it truly adding value or is it just another approach to a game that's already full of complexity and chaos?
Despite its considerable strengths, TrendSpider's effectiveness ultimately hinges on the user's judgment. Solely relying on automated signals, without critically assessing the overall market landscape, can lead to losses. This emphasizes the persistent need for a holistic and nuanced understanding of the market, despite the sophistication of the algorithm.
Decoding the Top 7 Candlestick Pattern Recognition Metrics Used by Modern AI Trading Algorithms in 2024 - Double Bottom Pattern Detection Through Transformers Shows 49% Win Rate in Crypto Markets
The double bottom pattern, visually resembling a "W" shape with two troughs at roughly the same price level and a peak in between, is a classic indicator that often signals a shift from a downtrend to an uptrend. This pattern, common in various financial markets including crypto, suggests a potential bullish reversal. Studies employing transformer models for pattern recognition have indicated that the double bottom pattern leads to successful trades roughly 49% of the time within the crypto market. While this win rate is suggestive of the pattern's potential, it also reveals the challenges inherent in relying on any single indicator for making profitable trading decisions.
Effectively using the double bottom pattern for trading involves careful analysis of price charts to confirm the validity of the pattern and to gauge support and resistance levels. Ideally, a confirmed double bottom would be accompanied by other positive signals, such as bullish candlestick patterns, which would strengthen the conviction of a reversal. It's crucial to remember that no pattern guarantees success, and the 49% win rate illustrates that market conditions can easily influence outcomes. Given the inherent unpredictability of markets, particularly in crypto, traders should be mindful of the inherent limitations of relying solely on technical patterns like the double bottom. A holistic approach, integrating multiple indicators and strategies, is often recommended to manage risk and improve decision-making.
The double bottom pattern, a visually simple formation with two troughs and a peak, has become popular in crypto trading circles. While its straightforward nature makes it appealing, a 49% win rate, as found in a recent study using transformers for pattern detection, suggests it's not a guaranteed path to profit. This raises questions about the reliability of relying solely on technical patterns for trading decisions, especially in a volatile market like crypto.
Transformers are a relatively new approach to pattern recognition, particularly well-suited for analyzing sequences like price data due to their ability to identify long-term relationships. This means they can potentially capture the essence of a reversal pattern that might span multiple candlesticks or even longer timeframes, potentially giving a better understanding of the broader market dynamics.
However, a 49% win rate means roughly half of the time, this pattern leads to losses. This clearly highlights that, in practice, using the double bottom on its own might not be a strong strategy. Traders would likely need to combine this pattern with other indicators or analysis techniques to improve the overall robustness of their trading decisions.
The accuracy of any machine learning model, including transformers, hinges on the quality of the training data. For the double bottom pattern, the amount and type of historical price data fed into the model are crucial. If the data is poorly curated, it could lead to models making inaccurate predictions. This underscores the challenge of creating models that can reliably detect real reversal patterns and not simply noise.
Discerning genuine reversal signals from false positives is difficult in the dynamic crypto markets. Even with improved pattern recognition from transformer models, incorporating additional information like volume or overall market sentiment is crucial for making a more informed trading decision. This points to the importance of a more contextual understanding of the market, which goes beyond just the shape of a few candles.
Transformers incorporate attention mechanisms, which allows them to focus on specific aspects of the data, effectively improving their ability to extract insights from complex patterns in time series data. However, this complexity also presents a risk. If not carefully managed, these attention mechanisms can lead to overfitting, where the model performs well on the data it's trained on, but fails to generalize to new data in live markets.
The growing adoption of transformer models indicates a shift in how traders use advanced technologies for financial analysis. But there are ongoing hurdles in making these models truly reliable. Issues like interpretability and decision-making transparency are crucial for traders to trust and use these technologies effectively.
Achieving a 49% win rate is a decent starting point, but there's still significant room for improvement. Seasonality, changing investor sentiment, and the innate volatility of crypto all create complexities that must be addressed to achieve better consistency in model performance.
The widespread use of this pattern may also be partly driven by human psychology. Traders may see it as an indication of a market sentiment shift. But this can lead to self-fulfilling prophecies where traders act on the pattern, thus influencing the market in a way that reinforces the pattern. This is a fascinating aspect of human behavior interacting with algorithmic systems.
Ultimately, the focus on AI-powered pattern recognition underscores a growing trend towards data-driven trading approaches. However, it's important to acknowledge that human intuition and experience are still essential, even in an era of increasing automation. This serves as a reminder that while algorithms are valuable tools, they should never be the sole basis for trading decisions.
Decoding the Top 7 Candlestick Pattern Recognition Metrics Used by Modern AI Trading Algorithms in 2024 - Morning Star Recognition Through Deep Learning Models Reaches 52% Accuracy in Forex
Deep learning models applied to Forex trading have achieved a 52% accuracy rate in recognizing the Morning Star candlestick pattern. This is a notable milestone, but also reflects the difficulties of using AI in the fast-paced and risky Forex world, where even small errors can result in significant losses. While deep learning's integration into trading is an encouraging sign of progress in AI, this accuracy level shows that there's still work to be done. Improving accuracy will likely require combining deep learning with other market indicators. The accuracy of recognizing patterns like the Morning Star through AI remains a crucial area of focus for researchers who want to improve how we forecast financial markets. Ultimately, this 52% accuracy serves as a reminder that traders should be cautious about relying too heavily on a single predictive model. A balanced approach that considers many different aspects of the market environment is needed for sound decision-making.
The reported 52% accuracy of deep learning models in identifying the Morning Star pattern in Forex trading highlights the limitations of relying solely on individual candlestick patterns for consistent profits. While this accuracy level suggests a potential edge, it's important to acknowledge that, over time, outcomes may resemble random chance.
The success of deep learning in detecting the Morning Star is strongly tied to the quality and quantity of the training data. Models that are exposed to a diverse array of market conditions during training, including periods of high and low volatility, generally perform better than those trained on a limited dataset.
Interestingly, the timeframe used for the analysis – whether it's hourly or daily candles – significantly impacts the model's accuracy. Some evidence indicates that longer timeframes might lead to better results, potentially because they filter out much of the noise intrinsic to the market. However, using longer timeframes could mean missing profitable shorter-term opportunities.
Furthermore, incorporating various technical indicators alongside the Morning Star pattern appears to enhance the overall prediction accuracy. Volume and momentum indicators, when combined with the Morning Star, seem to provide a more holistic view of market dynamics, which can be missing when relying on only one pattern.
While advanced deep learning models like LSTMs can yield encouraging results, they often come with the trade-off of decreased interpretability. This makes it difficult for traders to easily extract practical insights and adapt them to their trading strategies during live market conditions, presenting a complex balance between model accuracy and usability.
Additionally, the accuracy of Morning Star detection can change depending on the overall market environment, including its level of volatility and the strength of existing trends. Models that perform well in a strong trending market might not function as effectively in a sideways market. This raises concerns about the generalizability and robustness of these models across various market conditions.
The study of candlestick patterns can be influenced by inherent human biases. Traders may place undue emphasis on specific patterns, which can ultimately create self-fulfilling prophecies where a widespread belief in the pattern can cause market movements that appear to confirm it.
Even with a 52% detection rate, establishing clear action thresholds – when to enter or exit a trade based on the pattern – is a vital aspect of using the Morning Star for trading decisions. Implementing additional filters can help mitigate false signals and optimize the risk-reward balance of trading strategies.
Deep learning models applied to the Morning Star pattern can encounter the problem of overfitting. This happens when a model performs exceptionally well on the training data but fails to generalize to new, unseen data in actual trading environments, resulting in a potential performance drop in real-world scenarios.
Finally, ongoing research suggests that blending traditional technical analysis with modern machine learning methods could lead to more flexible and adaptable candlestick pattern recognition. These hybrid models could offer a more reliable approach compared to solely relying on either methodology, opening a new avenue for the evolution of AI-powered trading.
Decoding the Top 7 Candlestick Pattern Recognition Metrics Used by Modern AI Trading Algorithms in 2024 - Real-Time Three Line Strike Pattern Analysis Using Random Forest Models Shows 55% Success
The Three Line Strike candlestick pattern, a continuation pattern consisting of five bars, has been examined using Random Forest models. This pattern, signifying a potential trend reversal, is composed of three candlesticks following the current trend and then a fourth candlestick that counters those previous three, essentially reversing the prior movement. Volume plays a significant role in the pattern's strength, especially during that reversal candle. Increased volume during this reversal provides greater confidence in the signal, but it is important to remember that the 55% success rate obtained using this model is merely a moderate level of predictive success. It highlights the continuous development within the field of using machine learning for candlestick recognition in volatile trading environments. While 55% accuracy isn't exceptionally high, it underscores the potential – and the limitations – that can arise from deploying these kinds of AI models in a complex and ever-changing field like financial trading. Traders must remain mindful that relying solely on any single pattern recognition model is risky, and that these signals should be incorporated into a broader approach to developing a trading strategy.
The successful use of random forest models for real-time three-line strike pattern analysis, achieving a 55% success rate, highlights the potential of ensemble learning in financial markets. Random forests, by combining multiple decision trees, seem to handle the inherent noise in price data quite well, which leads to more stable predictions compared to simpler models. However, the very nature of these complex models can make understanding their decision process challenging. We need to be careful about how we interpret the results and figure out exactly which features are driving the predictions, which is where feature importance metrics become quite helpful.
It's interesting to consider that this pattern recognition success might not translate perfectly across all financial markets. It could be that the model's performance in equities might differ from forex or cryptocurrency, for instance. This suggests we need to constantly adjust and fine-tune these models as market conditions change, ensuring they stay relevant and accurate. Also, the real-time nature of this application really emphasizes the need for computationally efficient models. Random forests need to balance prediction accuracy with speed, especially in high-frequency trading environments where split-second decisions can make a big difference.
Of course, the quality of the training data is paramount. The model's ability to accurately identify the three-line strike relies heavily on the breadth and quality of the historical market data it's trained on. Datasets that cover a range of market conditions, including different levels of volatility, can significantly enhance model performance. Despite reaching 55% accuracy, we still need to be mindful of overfitting. This is where a model becomes too closely tied to the historical training data and doesn't adapt well to new, unseen market conditions. Cross-validation is a crucial technique to help reduce this risk.
The three-line strike pattern itself relies on a specific sequence of candlestick formations, and it's likely influenced by other market signals. Exploring additional indicators like volume trends or moving averages as potential input features could provide the model with more clues to enhance its predictive abilities beyond just candlestick analysis. While 55% accuracy is a good starting point, it's important to acknowledge that it still implies a significant number of unsuccessful trades. Traders need to be aware of this and factor this understanding into their overall decision-making, relying on a wider market analysis instead of relying solely on candlestick patterns.
Another factor to consider is how market volatility can impact the performance of the random forest model. In highly fluctuating markets, accurately recognizing patterns might become more difficult. We need flexible approaches where model parameters are adjusted based on current market conditions. Ultimately, the best results might come from combining random forest predictions with other trading strategies, such as taking market momentum into account or gauging overall market sentiment. This type of hybrid approach, using multiple analytical techniques, might be the way to build more robust trading strategies in the future.
More Posts from aitutorialmaker.com: