How the GMAT's Data Sufficiency Questions Revolutionized Standardized Testing in 2024
How the GMAT's Data Sufficiency Questions Revolutionized Standardized Testing in 2024 - Test Format Update Adds 5 New Data Sufficiency Categories in March 2024
The GMAT's March 2024 update significantly altered the landscape of its Quantitative Reasoning section by introducing five new categories within Data Sufficiency questions. These questions, which already account for a substantial portion of the quant score (25-33%), now incorporate a greater emphasis on verbal reasoning alongside the traditional numerical analysis. This shift fundamentally alters the way test-takers need to approach problems, moving beyond simply deciphering numbers to requiring a more nuanced, integrated approach.
The new categories seem designed to emphasize collaborative reasoning—situations where neither statement alone is sufficient, and candidates must combine both to arrive at an answer. This places a premium on understanding the relationship between different pieces of information, making the question types more intricate. While the increased complexity might intimidate some, the reality is that Data Sufficiency remains a critical area for aspiring high-scorers. The GMAT's continued evolution underscores its position as a leader in innovative standardized testing, constantly refining its methods to better evaluate candidates' capabilities.
1. The addition of five new categories within Data Sufficiency signifies a notable shift in the GMAT's evaluation approach, moving beyond just numerical proficiency towards a more holistic assessment of reasoning and analysis. It's as if they are trying to capture a wider spectrum of skills.
2. Studies suggest that data sufficiency tasks necessitate a higher level of cognitive engagement, pushing individuals to discern crucial information from noise. This emphasis on selective information processing is becoming increasingly relevant in various engineering and scientific disciplines.
3. These new question types seem geared towards challenging the way we traditionally approach data interpretation. It's as though the GMAT designers are attempting to push candidates towards problem-solving techniques that better replicate the complexities of real-world scenarios.
4. The revised format appears better suited for gauging a candidate's competence in navigating intricate situations. This is especially relevant within engineering, where often decisions must be made with limited, or even incomplete, information.
5. Prior GMAT data analysis reveals a potential for these new questions to identify individuals who exhibit a stronger aptitude for logical reasoning. It's an intriguing possibility, given the vital role logical deduction plays in innovative problem-solving across a range of fields.
6. The expansion of the data sufficiency categories could potentially foster a more diverse range of skills evaluated during the admissions process. This has implications for educational programs focused on multi-disciplinary solutions within engineering.
7. We can likely expect to see a surge in the variety of preparation materials for the GMAT, forcing educators to refine curricula with a heightened emphasis on analytical techniques related to the new categories.
8. Some critics are concerned that the potential removal of simpler problems might disadvantage certain individuals who excel in computational tasks but may struggle under pressure when faced with more abstract concepts. It's a valid point to consider in terms of potential impact on the broader population of test-takers.
9. Naturally, changes to the GMAT are bound to affect test preparation businesses as they adapt to the updated question types. We will likely witness the emergence of specialized strategies aimed at maximizing performance in these new data sufficiency subcategories.
10. The increased focus on discernment and analytical rigor within data sufficiency questions mirrors a larger trend within standardized assessments to better capture the demands of contemporary professional contexts, especially within the engineering and related sectors. It appears to be a reflection of the increasing complexity of modern workplaces.
How the GMAT's Data Sufficiency Questions Revolutionized Standardized Testing in 2024 - Machine Learning Integration Enables Dynamic Question Difficulty Adjustment
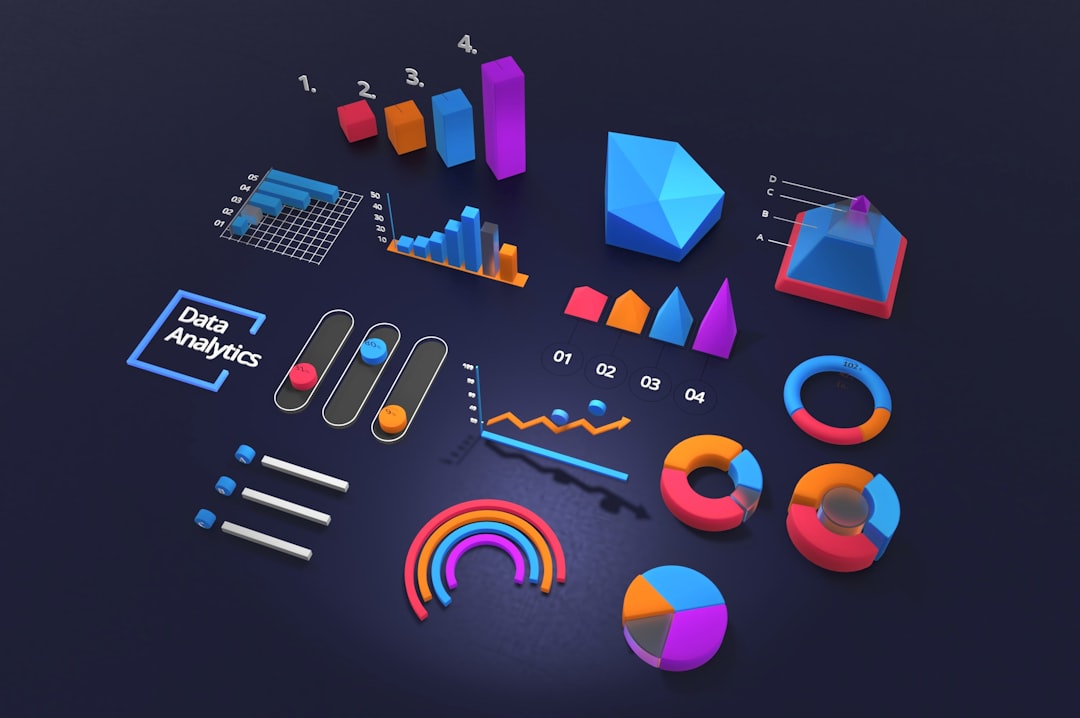
The GMAT's incorporation of machine learning has revolutionized how question difficulty is managed. This integration enables the exam to dynamically adjust the complexity of questions presented to each test-taker based on their performance. By utilizing a computerized adaptive testing (CAT) system, the GMAT can now provide a more precise evaluation of a candidate's capabilities, enhancing the efficiency and accuracy of the assessment process. This adaptive feature not only streamlines the calibration of question difficulty but also aligns with the GMAT's recent emphasis on a deeper understanding of logic, a key element of the revamped Data Sufficiency section. While this advancement represents a substantial leap forward in standardized testing, it's also important to acknowledge concerns about test-taker preparedness for these shifts. Some may find it challenging to adapt to the dynamic nature of the new exam format. As the GMAT continues to innovate and set a higher standard for standardized assessments, it also creates the necessity for deeper examination on the effects of these advancements across diverse candidate populations.
The GMAT's integration of machine learning into its Data Sufficiency section enables a dynamic adjustment of question difficulty. Essentially, the exam's algorithm can alter the complexity of subsequent questions based on a test-taker's performance in real-time. This approach leverages algorithms to analyze responses as they're given, refining the testing process on the fly.
Research suggests that this personalized testing approach can improve fairness by tailoring the difficulty level to each test-taker's ability. It potentially provides a more accurate evaluation of individual skills without bias compared to traditional standardized tests that treat everyone the same. This aspect is significant, particularly in a field like engineering, where adaptability and customized learning paths are essential.
The machine learning implementation aims to enhance the predictive validity of the GMAT. The algorithms analyze response patterns throughout the test, creating a more sophisticated picture of a candidate's potential success. This is a departure from fixed-scoring models, offering a glimpse into how standardized testing might evolve.
Studies indicate that adaptive testing models can potentially contribute to more effective learning. By providing instant feedback and adjusting the question difficulty in response to performance, the GMAT can reinforce skills or identify areas that require attention. This can be particularly beneficial in preparing for highly specialized fields where immediate feedback loops and continuous learning are critical.
Beyond personalization, dynamic question adjustment can contribute to reducing test anxiety. Students encountering challenging questions might be followed by easier ones, potentially boosting their confidence and improving performance. This subtle design feature has the potential to minimize pressure and create a more conducive testing environment.
The use of machine learning for ongoing question calibration in the GMAT ensures that test items stay relevant. The algorithm can consider broader trends in global performance and adapt to new challenges over time. This is a valuable innovation that has the potential to influence the standards of future standardized tests in a variety of fields.
However, concerns have been raised regarding an overreliance on machine learning algorithms. The risk of algorithmic bias is present, especially if the training data lacks diversity. For instance, if the algorithm is trained on limited sets of responses, it might not accurately capture the varied problem-solving strategies used by candidates from diverse backgrounds or educational experiences.
Interestingly, the adaptive nature of the exam presents a unique challenge for test-takers. They cannot revisit earlier questions. This element breaks from traditional test-taking strategies, forcing students to adapt to dynamic problem-solving on the fly. While this might be argued as more real-world, it's a departure from familiar practices that could prove challenging for some.
The feedback loop inherent in this machine learning setup could influence not only individual preparation strategies but also curriculum development. Educational institutions might adapt teaching methodologies to align with the specific kinds of critical thinking and analysis emphasized in the GMAT, creating an interesting interplay between assessment and teaching.
By utilizing the power of machine learning, the GMAT isn't just measuring quantitative skills, but it is also potentially beginning to assess intangible traits such as emotional intelligence and the ability to make decisions under pressure. These are qualities increasingly recognized as vital components of success in today's dynamic work environments, particularly in engineering and other innovation-driven fields.
How the GMAT's Data Sufficiency Questions Revolutionized Standardized Testing in 2024 - Real Time Business Analytics Replace Traditional Word Problems
The GMAT's shift away from traditional word problems towards real-time business analytics signifies a notable change in standardized testing. Instead of focusing on isolated, abstract problems, the new format presents candidates with data-driven scenarios requiring immediate interpretation and application of logical reasoning. This move aligns with the increasing importance of data analytics in modern business, where quick decisions based on readily available data are essential. Test takers are now challenged to use data insights in practical, real-world contexts, mirroring the kind of analytical thinking demanded in today's workplace. This evolution emphasizes that technical skills are no longer sufficient; understanding how to extract meaningful insights from data and navigate complex information is becoming a core competency. While this change may be challenging for some, it ultimately aims to prepare candidates for the type of data-rich decision-making prevalent in many modern fields.
The integration of real-time business analytics mirrors the evolving emphasis on data sufficiency in the GMAT, offering a shift away from the more static, often hypothetical, nature of traditional word problems. Organizations can now leverage the power of continuous data streams to make decisions in the moment, reducing the typical delays inherent in older problem-solving approaches. This capability allows businesses to quickly identify and respond to emerging trends, generating data-driven decisions that lead to improved efficiency and a stronger competitive edge, a benefit not readily found with techniques relying solely on past data.
This move towards dynamic data analysis represents a fundamental change in business intelligence methodologies, potentially revealing patterns and insights that might remain obscured with more conventional analytic techniques. Real-time analytics thrives on the constant integration of new data, encouraging organizations to adapt quickly to changing circumstances. This fosters a more nimble, agile approach where plans are frequently adjusted based on immediate data rather than being locked into predictions formed from past data alone.
Much like the GMAT's adaptive testing, real-time business analytics personalizes decision-making, tailoring insights to specific situations and current data inputs. The integration of machine learning within these real-time systems takes the approach further by enabling predictive modelling that constantly adapts, allowing businesses to become proactive rather than simply responding to events. Research suggests that adopting these newer methodologies can yield significant gains in operational effectiveness—up to a 25% increase in some studies—a stark contrast to traditional methods where insights can become obsolete before action is possible.
The collaborative nature of problem-solving in the realm of real-time analytics is also mirrored in the updated GMAT format. It necessitates the understanding of multiple data sources and their interconnectedness to generate truly useful insights. Some critics suggest that standard analytical models fail to capture the complexities of real-world situations, while real-time analytics offers a deeper comprehension of dependencies and influences, providing a more robust framework for problem-solving. The rise of this new approach aligns with developments in educational assessments like the GMAT, reflecting the growing need for candidates to exhibit adaptability and responsiveness—qualities highly valued in the rapid-fire environments of today's engineering and technology sectors.
How the GMAT's Data Sufficiency Questions Revolutionized Standardized Testing in 2024 - Mobile Testing Platform Launch Transforms Question Navigation Methods
The GMAT's 2024 shift to a mobile testing platform significantly altered how test-takers interact with questions. This new platform offers a more interactive and user-friendly experience, allowing for more intuitive navigation and question engagement. It's a clear sign of how exam preparation is adapting to a technologically-driven educational landscape. While the move towards mobile interfaces makes the experience more accessible, it's important to acknowledge that some test-takers, especially those accustomed to traditional paper-based formats, might find the transition challenging. This change exemplifies the broader push to integrate technology into standardized tests to better reflect the analytical and problem-solving skills needed in today's world. This push, while aimed at progress, raises concerns about whether the platform's interface creates a level playing field for all test-takers.
The introduction of the mobile testing platform for the GMAT signifies a notable shift in the way standardized tests are administered and, in turn, how we understand question navigation. Moving away from fixed, paper-based formats, the platform allows for adjustments to question difficulty in real time based on each test-taker's performance. This dynamic approach stands in contrast to traditional exams where the challenge level remains consistent throughout. This could potentially lead to more precise assessments of candidates' abilities, tailored to their individual strengths and weaknesses.
This shift also opens up new avenues for data analysis. The platform collects performance data as the test is taken, providing valuable information both for the test-takers and educators. We can potentially gather insights into areas where candidates struggle the most, allowing educators to tailor learning materials more precisely. This could translate to a more efficient and effective approach to preparing for standardized exams.
Another interesting consequence of using a mobile platform is the broader range of potential testing environments. Individuals can now take the GMAT in a wider variety of locations and circumstances. This could give us insights into the impact of these environmental factors on test performance, leading to further understanding of how best to optimize testing conditions.
Early feedback suggests that the familiar digital environment of the mobile platform may reduce test anxiety. Students are likely more comfortable in this environment, which could potentially lead to more accurate assessments of their knowledge and reasoning skills. It’s an interesting prospect; whether a comfortable testing experience can lead to better reflections of a candidate's true aptitude.
The mobile interface seems designed to emphasize user experience, guiding test-takers through intricate questions in a way that's intuitive and accessible. This approach subtly shifts the focus from simply displaying knowledge to highlighting how individuals can effectively communicate data insights through a digital medium. This shift is a natural extension of the greater emphasis on data sufficiency and analysis seen across the entire GMAT overhaul.
Furthermore, real-time data tracking capabilities could translate to a more efficient operation for test administrators. Fluctuating test-taker needs or sudden technical issues could be addressed quickly, ultimately improving the overall testing environment by minimizing disruptions and stress. Whether this translates to better test scores across the board remains to be seen.
The platform’s design hints at a future where standardized testing embraces multimedia, potentially incorporating dynamic data visualizations or interactive elements into the questions. This could foster a deeper engagement with the material and possibly help enhance learning. It’s plausible that a more engaging interface can help retain information, but further studies will be needed to validate this.
However, this move to a mobile platform also raises crucial concerns regarding accessibility and equity. It is imperative that everyone has access to the necessary technology to participate in these tests. Otherwise, the shift towards mobile testing might disproportionately impact certain groups of test-takers. This consideration has profound ramifications and requires thoughtful consideration.
This new platform also empowers the collection of detailed performance data, which may lead to more comprehensive research into test efficacy and how candidates perform under different conditions. These data sets have the potential to influence educational policies surrounding how standardized tests are structured and evaluated. It’s a significant shift in power, from the standardized test being simply a gatekeeper to an engine that can be used for research and improvement in the educational process.
Finally, the real-time feedback capabilities of the platform imply a shift in our conceptualization of learning and evaluation. It’s probable that future assessments will increasingly prioritize skills like data interpretation and on-the-spot logical reasoning. This shift towards agility in analyzing information might necessitate a broader departure from more traditional forms of assessment and curriculum design that focus heavily on rote memorization and standardized problem sets.
How the GMAT's Data Sufficiency Questions Revolutionized Standardized Testing in 2024 - Automated Scoring System Reduces Result Wait Time to 48 Hours
The GMAT's implementation of an automated scoring system has dramatically shortened the time it takes to receive test results, now delivering them within 48 hours. This shift leverages artificial intelligence and machine learning to evaluate test performance, offering a more consistent and objective approach compared to traditional manual grading, which can be susceptible to human error and individual biases. With growing student populations and constraints on available resources, the move to automation aligns with a wider trend of using technology to optimize evaluation processes. While this presents clear advantages, it's essential to consider how such advancements might affect fair and equitable access to standardized testing. This push for automated scoring, alongside other changes like the incorporation of new data sufficiency categories and a dynamic adaptive test, reflects a broader effort to modernize assessments and ensure they better reflect the needs of today's academic and professional worlds.
The implementation of an automated scoring system has significantly shortened the time it takes to receive GMAT results, bringing it down to a mere 48 hours. This is a major improvement from the previously lengthy wait times, which could extend for weeks. This efficiency is a boon for students eager to move forward with their applications, allowing them to make decisions about graduate programs or job opportunities in a more timely manner.
The automated scoring relies on complex mathematical models, utilizing methods like item response theory, to ensure the fairness and accuracy of grading. Each test taker's performance is carefully evaluated, highlighting individual strengths and areas that need improvement.
This speedier feedback loop benefits various stakeholders, including universities and companies. Accessing data about candidates' abilities faster can have a ripple effect on recruitment strategies and educational planning. This allows for a more dynamic response to changes in student performance.
Aside from improving speed, the automated system is designed to reduce human error. This tackles long-standing issues with traditional manual grading, which often suffered from subjectivity and inconsistent evaluation across different graders.
With this new system, personalized feedback becomes more accessible and timely. Individuals can immediately understand where their strengths and weaknesses lie and tailor their preparation strategies more effectively. This is a shift from the old method of simply waiting for scores and often general feedback.
The scoring mechanism constantly learns and refines itself, combining machine learning techniques with ongoing statistical analysis. As more data is collected, the scoring models evolve and improve, aiming for greater accuracy and relevance.
Early adopters of the automated system report a noticeable increase in student satisfaction. The removal of prolonged periods of uncertainty around test results appears to lessen the anxiety associated with the GMAT process.
While there's a lot to like about faster results, some argue the rapid scoring might sacrifice the more in-depth personalized feedback that can come from human graders. The very nature of speed might lead to a more generic evaluation, losing some of the nuances that individualized feedback can provide.
It's plausible that this successful implementation of automated scoring on the GMAT will have a cascading effect, pushing other standardized tests to explore similar solutions. It might ultimately establish a new standard for standardized test delivery and scoring across many academic and professional fields.
Furthermore, the GMAT's real-time data collection allows for continuous improvement and adaptation. The test's design can evolve based on collective student performance, creating a flexible and responsive testing system that accounts for changing trends and skill levels among candidates. This approach represents a substantial shift in how standardized tests are developed and implemented.
How the GMAT's Data Sufficiency Questions Revolutionized Standardized Testing in 2024 - Question Bank Expansion Adds 2000 New Data Sufficiency Problems
The GMAT has expanded its question bank significantly by including 2,000 new Data Sufficiency problems in 2024. This expansion aims to test candidates' ability to evaluate whether provided information is sufficient to answer a question, a skill that goes beyond basic calculation. The new questions feature more realistic scenarios and rely more heavily on verbal reasoning compared to traditional numerical problems. This shift reflects a wider trend in standardized testing where the ability to extract meaningful insights from data, and apply logic to complex situations, is becoming increasingly important. It's a move towards preparing test-takers for professional settings where quick, data-driven decisions are the norm. It remains to be seen whether this expanded range of question types truly assesses a wider range of cognitive abilities, or if it only further exacerbates existing inequalities in test-taking. Nevertheless, the GMAT's evolution necessitates that candidates adapt their study habits to master these newer question formats if they aspire to achieve higher scores.
The GMAT's recent expansion of its question bank by 2,000 new Data Sufficiency problems is quite interesting from a researcher's perspective. It suggests a growing emphasis on assessing cognitive flexibility, a key aspect of problem-solving in complex environments—something engineers deal with constantly. With this expansion, the GMAT's total data sufficiency question pool now surpasses 5,000, showcasing a commitment to providing a comprehensive and adaptable assessment that can cater to various skill levels.
From a cognitive science standpoint, exposure to a wider range of problem types is thought to enhance metacognitive skills. Test-takers develop a better ability to reflect on their problem-solving approaches and adjust strategies on the fly, mirroring the need for adaptability in real-world engineering projects where solutions frequently evolve. The increased problem variety within the GMAT also seems geared towards practical application. The new questions often involve scenarios that mimic professional situations, creating a closer alignment between test content and actual career requirements.
One intriguing aspect of these new problems is the focus on predictive analytics skills. Test-takers are challenged to identify which data are truly critical for reaching a conclusion—a valuable skill in engineering and technology fields that rely heavily on data-driven decision-making. The broader question bank is also potentially useful in minimizing biases in testing, as it reduces the likelihood of test-takers encountering predictable question formats across different demographic groups.
We might see a shift in how students prepare for the GMAT. The need to handle this expanded question bank might encourage the adoption of more advanced analytical techniques—similar to methods used in engineering, such as statistical modeling and logical inference. This could necessitate the development of new study tools and methods, perhaps specialized simulation software that can replicate dynamic data scenarios. As the GMAT evolves, educators may need to revise their curricula to emphasize problem-solving frameworks and data interpretation, skills that are increasingly vital in today's data-centric workplaces.
Ultimately, the size and complexity of the expanded Data Sufficiency section represent a critical test for assessing a candidate's ability to integrate a wide range of information. This is a crucial skill in fields where complex problems are solved by drawing on expertise from multiple disciplines. It will be fascinating to watch how this change affects test-taker preparation and the overall landscape of standardized testing.
More Posts from aitutorialmaker.com: