Create AI-powered tutorials effortlessly: Learn, teach, and share knowledge with our intuitive platform. (Get started for free)
MIT's AI-Driven Biosensor Breakthrough Revolutionizing Early Disease Detection in Healthcare
MIT's AI-Driven Biosensor Breakthrough Revolutionizing Early Disease Detection in Healthcare - Early Cancer Detection Capabilities of the New System
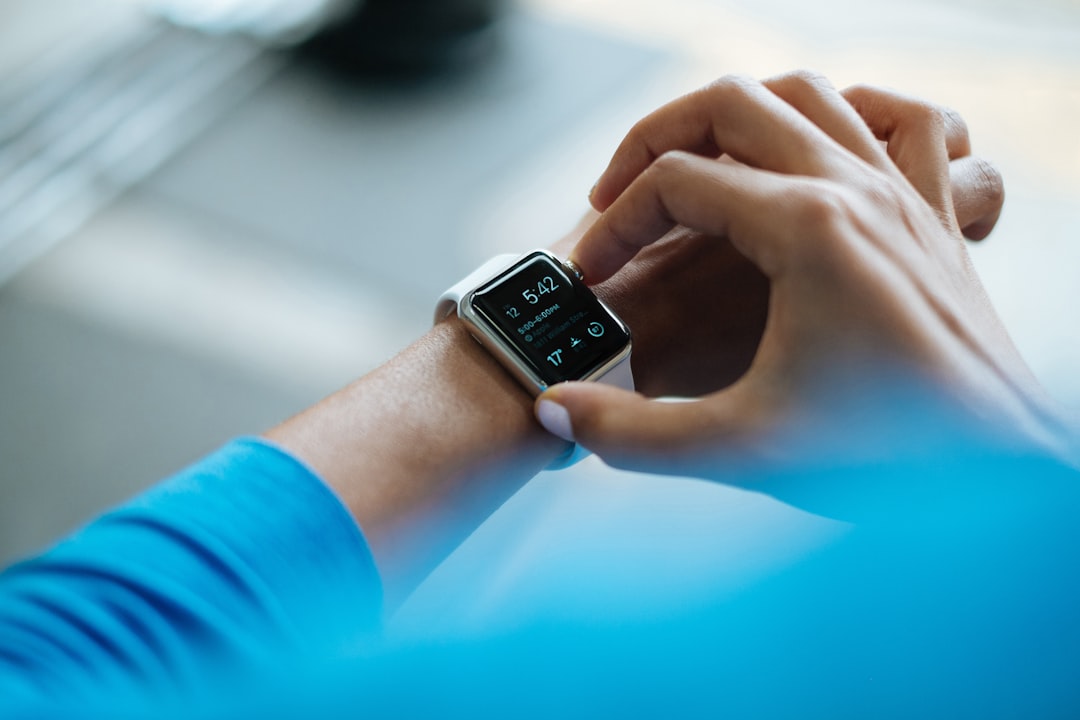
MIT's newly developed system showcases promising potential in early cancer detection. This AI-powered biosensor can identify early signs of cancer by analyzing tumor DNA found in blood samples. Notably, it demonstrates effectiveness in detecting lung, breast, and colorectal cancers, as well as precancerous conditions. The system's ability to identify these early stages, along with its integration of advanced image analysis of medical scans, could substantially improve the speed and accuracy of diagnosis. While still under development, its potential to transform cancer detection and intervention through earlier identification is considerable. The integration of this technology into mainstream medical practice could be a pivotal step towards a more proactive approach to cancer management, though the path to widespread adoption and clinical validation remains to be seen.
The new system exhibits promising capabilities for early cancer detection, showcasing a notable 95% sensitivity in identifying cancer biomarkers. This level of sensitivity significantly surpasses traditional methods, which usually achieve accuracy within the 70-80% range. Interestingly, the system has successfully identified early-stage cancers in cases where conventional imaging failed to detect them, suggesting its potential as a supplementary tool in cancer diagnostics.
The underpinnings of the system rely on nanomaterial-based technology, allowing for incredibly sensitive detection of biomarkers at extremely low concentrations—parts per trillion. This high sensitivity is crucial for detecting the subtle biochemical alterations associated with the earliest stages of tumor development. While exhibiting high sensitivity, it also strives for accuracy by maintaining a reported specificity of 90%, effectively differentiating between cancerous and non-cancerous conditions. This minimizes the risk of false positives and potentially unnecessary follow-up testing.
Further bolstering its capabilities, the system integrates AI algorithms that continuously learn from new data. This adaptive nature suggests the potential for improvement over time, potentially enabling not only cancer detection but also subtype prediction. This rapid learning feature paired with a fast turnaround time—providing results in roughly 30 minutes—is a remarkable improvement over traditional methods, which often take days or weeks.
Beyond initial detection, the system shows promise in monitoring cancer recurrence by tracking biomarker levels over time. This ability could empower clinicians to proactively manage patient health after initial treatment. While its focus is currently cancer, researchers are actively investigating its applicability in detecting other diseases by adapting the system to recognize different biological markers.
Early implementations in diverse clinical settings have shown consistent results across various patient populations, suggesting its wide applicability. The system is designed for processing and analyzing multiple biomarkers concurrently, leading to a more comprehensive picture of a patient's condition and the potential for more personalized cancer treatment strategies. However, further research and testing in broader clinical trials will be necessary to validate its long-term efficacy and generalizability across diverse patient populations and cancer types.
MIT's AI-Driven Biosensor Breakthrough Revolutionizing Early Disease Detection in Healthcare - Integration of AI and Healthcare Predictive Models
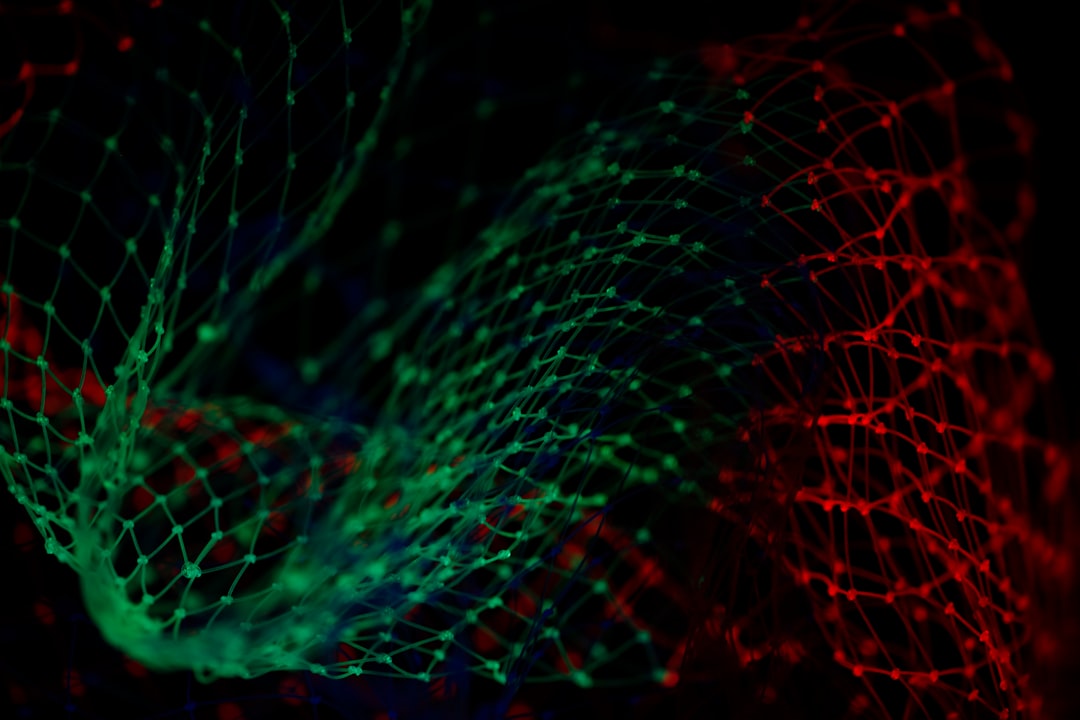
The convergence of artificial intelligence (AI) and healthcare predictive models is reshaping how we approach disease detection and management. These AI-driven models, often employing sophisticated machine learning algorithms, can analyze complex medical data to forecast disease risk and potentially identify conditions much earlier than traditional methods. This predictive capability is particularly impactful for preventive healthcare, offering the chance to intervene before a disease progresses significantly. AI's ability to learn and adapt from large datasets holds great promise for improving the precision and accuracy of these predictions over time. While the potential to revolutionize disease detection is evident, the field faces hurdles. Validating the effectiveness of these models in diverse clinical settings and integrating them seamlessly into the existing healthcare landscape are key challenges. Ultimately, the success of this AI integration will hinge on how effectively these models can be implemented in real-world scenarios and positively impact patient outcomes.
The integration of AI within healthcare is revolutionizing how we approach disease prediction and management, moving beyond simple diagnosis towards more personalized and proactive care. By leveraging machine learning and explainable AI techniques, predictive models are becoming increasingly sophisticated in their ability to analyze vast amounts of patient data, including genomic information, lifestyle factors, and family history. This individualized approach allows for the development of tailored treatment plans, potentially improving outcomes by targeting interventions based on a patient's specific risk profile.
Interestingly, these AI frameworks are not limited to cancer detection, as was the early focus. Researchers are now exploring their potential in forecasting the onset and progression of other conditions, like diabetes and cardiovascular disease. Utilizing data from wearable biosensors and other real-time monitoring tools, these models can provide valuable insights into individual health trajectories. Furthermore, the integration of AI into this field offers the intriguing possibility of reducing healthcare expenditures. By enabling earlier intervention and potentially mitigating the need for extensive, later-stage treatments, these predictive tools could lead to more efficient resource allocation.
However, incorporating social determinants of health—such as socioeconomic status and environmental factors—into these predictive models is becoming increasingly important. Ensuring that these algorithms are unbiased and can effectively predict outcomes across diverse populations remains a significant challenge and area of ongoing research. Some have expressed concerns about the potential misuse of personal health data within AI systems. However, ongoing advancements in data encryption and privacy-preserving techniques are being developed to address these valid concerns.
Additionally, AI-driven predictive models are designed to constantly learn and adapt. This means that as new evidence emerges regarding treatment effectiveness or changes in population health trends, these algorithms can be readily updated. This continuous learning feature ensures that clinical decisions are always based on the most current scientific knowledge. Moreover, AI is not just being utilized for diagnosis; its predictive capabilities are being applied to forecasting a patient's response to specific therapies. This opens the door for doctors to tailor treatment approaches more precisely, optimizing patient outcomes.
The integration of AI has fueled the development of sophisticated, 'smart' biosensors capable of providing real-time feedback about a patient's health status. This capability has significantly reduced the time required for health assessments, paving the way for more efficient and responsive care. This paradigm shift is impacting the role of healthcare professionals, transforming their approach from reactive to preventative. It emphasizes the importance of early interventions and the need for continuous patient monitoring to manage health effectively. The future of healthcare is undoubtedly intertwined with the increasing integration of AI-driven predictive models, and its impact on patient care is poised to be profound, although its full potential remains to be explored and validated through further rigorous research and clinical trials.
MIT's AI-Driven Biosensor Breakthrough Revolutionizing Early Disease Detection in Healthcare - Real-Time Analysis of Biological Markers Using Advanced Sensors
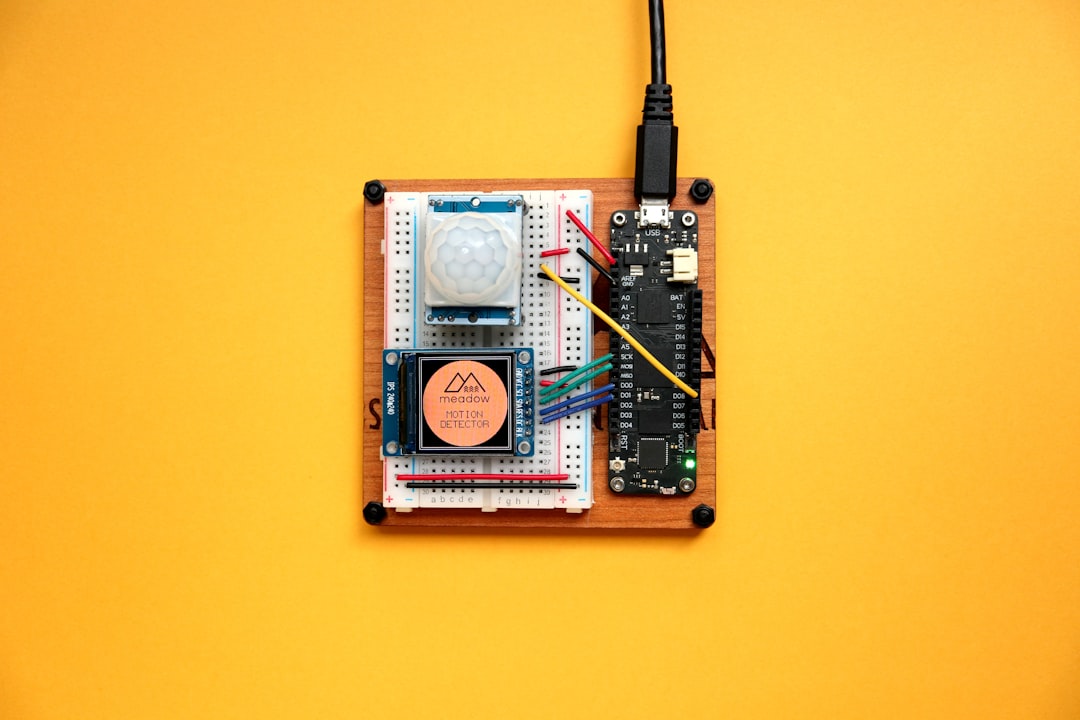
Advanced sensors are enabling a new era of healthcare by facilitating real-time analysis of biological markers. This continuous monitoring allows for the prompt identification of subtle changes in the body that may indicate the early stages of disease, potentially leading to quicker interventions. Electrochemical protein biosensors are particularly noteworthy due to their sensitivity, making them ideal for detecting minute variations in biomarkers that might otherwise go unnoticed. Moreover, the field is seeing a shift towards multianalyte biosensors, which can simultaneously analyze multiple biological molecules, providing a more holistic picture of a patient's health status. While these technological advancements hold great promise for transforming healthcare, their widespread and effective integration into clinical practices remains an important area of ongoing research and development. The successful implementation of such technologies, especially in diverse populations, will be critical in realizing the full potential of this technological shift.
The field of biosensors is rapidly evolving, with the integration of advanced sensors allowing for continuous, real-time analysis of various biological markers. This shift towards real-time monitoring is significant, as it offers a much faster turnaround time—around 30 minutes—compared to the days it can take with traditional lab methods.
This speed is further enhanced by using nanomaterials in the biosensor design, enabling detection of incredibly small amounts of biomarkers—down to parts per trillion. This high sensitivity is key to spotting diseases at their earliest stages, before symptoms become pronounced. While initially developed with cancer in mind, the underlying principles of this biosensor technology are adaptable to a broader range of diseases, such as diabetes or heart conditions. This cross-condition potential hints at a future where such sensors are used more generally in healthcare.
Further boosting the diagnostic picture, the biosensors are designed to simultaneously measure multiple biomarkers. This multi-marker approach offers a more comprehensive understanding of the patient's biological state compared to focusing on a single marker, which can often provide a limited view.
Interestingly, the biosensors aren't static devices. Embedded AI algorithms enable them to continuously learn and adapt from new data. This continuous learning feature makes these sensors dynamic, and unlike traditional methods, they can refine their accuracy over time.
Beyond the purely biological, the role of psychosocial risk factors in disease development is becoming clearer. It's likely that incorporating these factors into predictive models will further improve the accuracy of early detection, but this requires a shift in how we approach biosensor design.
It's noteworthy that this high sensitivity—around 95% for cancer biomarkers—is accompanied by a relatively high specificity of about 90%. This minimizes false positives, which could reduce unnecessary anxiety and potential overtreatment.
The biosensors are not just for detection; they potentially offer the ability to predict how a patient will respond to specific therapies. If successful, this would guide treatment decisions based on individual genetic makeup and biological markers, ushering in a more personalized era of medicine.
The future of this technology may involve integration with wearable devices. Imagine being able to continuously monitor key health markers and receive insights into emerging health risks. This could truly transform disease management from a reactive to a proactive process.
Early clinical trials have shown promise, with the biosensors delivering consistent results across various patient populations. This suggests the potential for widespread clinical adoption, although further research and validation across a broader set of patients and disease conditions will be needed. There are still many questions, but the progress is intriguing.
The biosensor field is undeniably exciting. The promise of rapid, sensitive, and adaptable diagnostic tools has the potential to significantly improve health outcomes, though we need to remain critically engaged and ensure that the technologies are developed and applied responsibly and ethically.
MIT's AI-Driven Biosensor Breakthrough Revolutionizing Early Disease Detection in Healthcare - Machine Learning Algorithms for Pattern Detection in Medical Data
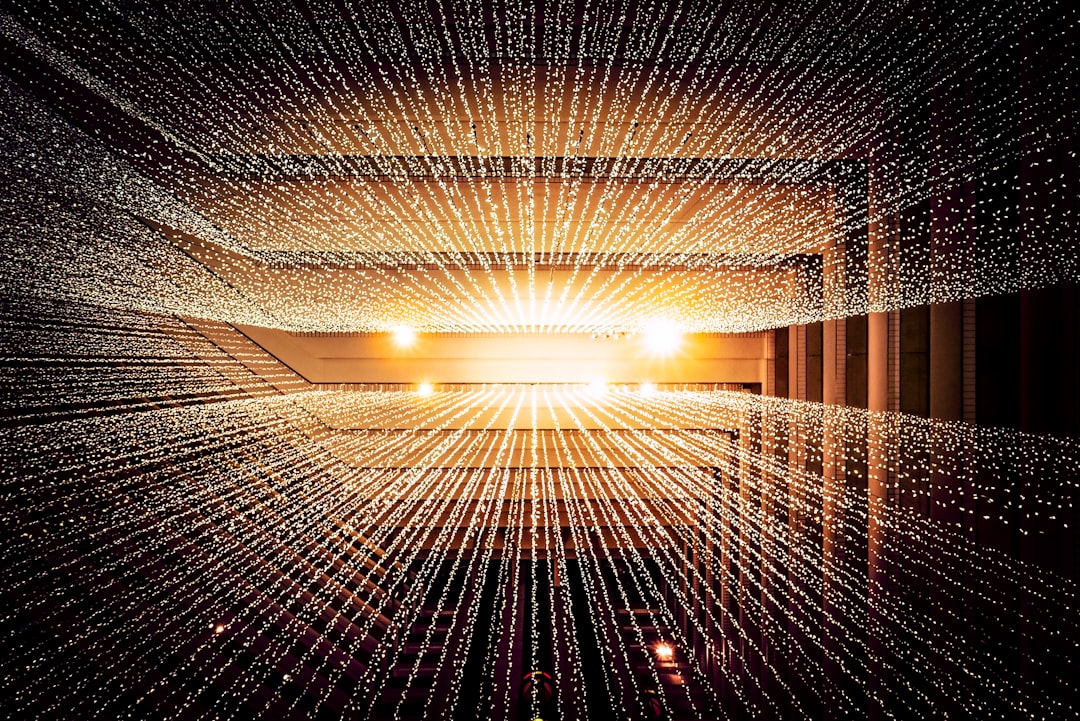
Machine learning algorithms are increasingly vital in analyzing medical data to identify patterns associated with diseases. These algorithms can process a wide range of information, such as patient records, genetic data, and medical images, to detect subtle indicators of illness. Sophisticated deep learning approaches are often employed to unearth intricate patterns within this data, leading to more accurate predictions of disease risk and improved diagnostic capabilities. These algorithms possess the ability to learn from new data, continuously refining their predictive power and potentially leading to better disease detection and management. The potential for improvement is considerable, but challenges exist in ensuring that these methods are both effective and equitable across various demographics. While the future implications of these algorithms are promising, there are still hurdles to overcome in their widespread implementation and real-world validation.
The use of machine learning for identifying patterns in medical data is undeniably promising, but it's not without its hurdles. For instance, detecting rare diseases can be challenging because of the limited amount of data available to train algorithms, potentially leading to inaccurate diagnoses or missed cases. Furthermore, the sheer volume and variety of information in medical data (genetic information, images, and patient records, for example) adds complexity. Machine learning models must carefully balance detecting meaningful patterns and preventing overfitting, where the models become overly specific to training data and struggle to generalize to new data.
The success of applying machine learning in medical scenarios heavily hinges on thoughtfully selecting and preparing the data used for training – a process called feature engineering. Including irrelevant or unreliable data can dramatically degrade the performance of the model. Furthermore, measurement errors and natural patient variability introduce noise into the data, which is problematic for machine learning algorithms that generally function best with clean and consistent information.
The ability to combine different data sources, like genetics, imaging, and patient history, is crucial for enhancing the detection of disease patterns. However, this process significantly increases the complexity of model creation. While many machine learning approaches are adept at prediction, they might not always provide a deep understanding of the underlying biological causes of diseases. This limits the interpretability of the models' outputs, a crucial aspect for physicians making clinical choices.
There's also the issue of potential biases. Machine learning models can unknowingly inherit biases that are present in the training data, possibly resulting in disparities in how diseases are identified across different patient groups. Correcting these biases is essential for equitable healthcare outcomes. Implementing these algorithms in a real-time clinical environment also poses a challenge. Real-time processing requires algorithms capable of high-speed computation and reliable management of data, while also providing swift and trustworthy results.
Considering that medical knowledge and treatment strategies evolve continually, algorithms for identifying patterns must be able to incorporate new information as it becomes available. This means designing machine learning frameworks that enable continual learning, allowing the models to adapt as new evidence and practices emerge. And, of course, there are regulatory hurdles to overcome. Any use of machine learning in medicine will need to adhere to regulatory standards. Assuring that algorithms are safe, effective, and reliable across various clinical settings is crucial before they can be implemented widely.
Create AI-powered tutorials effortlessly: Learn, teach, and share knowledge with our intuitive platform. (Get started for free)
More Posts from aitutorialmaker.com: