Create AI-powered tutorials effortlessly: Learn, teach, and share knowledge with our intuitive platform. (Get started for free)
Neural Network Models for Analyzing Infant and Toddler Development Patterns A Data-Driven Approach
Neural Network Models for Analyzing Infant and Toddler Development Patterns A Data-Driven Approach - Neural Networks Track Infant Motor Development Through MRI Analysis
Analyzing infant brain development through MRI scans has become more sophisticated with the introduction of neural networks. These networks, especially deep learning models, offer a unique advantage in automatically extracting intricate details from the images, a task that was previously dependent on manually defined features. This automated approach opens the door for uncovering hidden relationships in the brain's structure and function.
A key finding within this research area is the correlation between enhanced connectivity within the brain's motor regions—including connections to areas like the prefrontal cortex—and more mature motor skills in infants. Such insights could lead to the development of early interventions targeted at specific developmental areas. Furthermore, the capacity of neural networks to assess functional connectivity is proving particularly valuable in predicting cognitive outcomes, particularly for infants born prematurely. By identifying potential delays early on, these models help build a more complete picture of neurodevelopment.
This trend towards data-driven approaches signifies a shift away from traditional methods. The ability to generate more precise and individualized assessments of infant development based on MRI data is becoming increasingly possible, fostering a better understanding of individual developmental trajectories.
Infant brain development, particularly in relation to motor skills, is a complex and fascinating field. Neural networks are emerging as powerful tools to understand this intricate process by analyzing MRI data. Interestingly, they can detect subtle changes in brain activity related to motor milestones, sometimes even before these changes are outwardly visible through behavior. By combining advanced MRI techniques with neural network analysis, researchers can map out intricate neural connectivity patterns. These patterns may potentially predict future motor skills remarkably early, possibly up to six months in advance. This contrasts with traditional methods, which often rely on visual observations and can be prone to subjective interpretations. Neural networks offer an objective and quantifiable approach, minimizing human bias.
One intriguing aspect uncovered by neural network analysis is that the development of motor skill-related neural pathways seems to occur in a non-linear fashion. This type of intricate development is something neural networks are well-suited to model due to their ability to learn and capture complex relationships within datasets. The computational power of these models also allows them to process massive datasets in a fraction of the time it would take a human researcher. Early research suggests that the observed patterns in brain development linked to motor skills might also relate to predicting future cognitive abilities. This highlights how motor and cognitive development are intertwined in early life.
The preliminary use of these networks in analyzing infant MRI data shows promising results in generalizability across different infant populations. This suggests potential for wider clinical applications in the future. Researchers are further exploring the idea of leveraging these networks to personalize developmental assessments. This could pave the way for highly customized interventions designed specifically for an individual infant's predicted developmental path. As we gather more data and improve network architectures, our understanding of infant motor development will likely evolve continually. The adaptability of neural networks allows them to learn from new information, fostering a continuous cycle of refinement. It is also exciting to consider that future iterations of these networks may be able to incorporate various data sources, such as combining MRI data with behavioral observations. This kind of multimodal approach could yield a holistic view of early childhood development and significantly advance our understanding of the intricate interplay of factors influencing it.
Neural Network Models for Analyzing Infant and Toddler Development Patterns A Data-Driven Approach - Machine Learning Models Map Toddler Language Acquisition Milestones
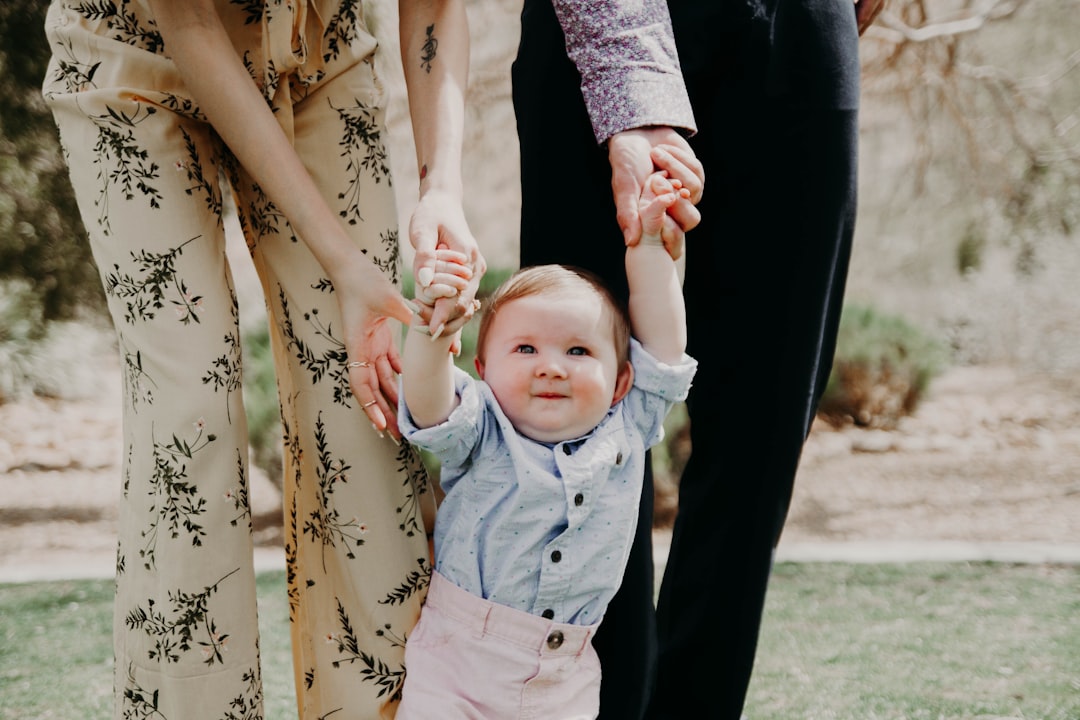
Machine learning models are increasingly being used to understand how toddlers acquire language, offering a detailed view of the developmental milestones involved. These models can help pinpoint and potentially predict language delays, including serious conditions like Developmental Language Disorder (DLD), which affects a substantial number of children. By analyzing large collections of audio recordings and utilizing sophisticated AI techniques, researchers are questioning conventional wisdom surrounding language development, particularly in children from diverse backgrounds. This includes exploring how social and economic factors might influence language acquisition. Moreover, these machine learning models are showing promise in identifying behavioral patterns linked to conditions like autism, offering a pathway to create interventions tailored to individual needs. This intersection of technology and research has the potential to reshape how we understand and support language development during the crucial early years of a child's life. It's important to acknowledge that while these tools hold great promise, further refinement and validation are needed to ensure their accuracy and reliability in different contexts.
Early language development, especially around the time toddlers start producing their first words (often around 12 months), appears to be quite well-mapped by machine learning models. This is intriguing as it suggests these models can potentially improve the accuracy of developmental screenings by providing more quantitative insights.
It's remarkable how machine learning algorithms can sift through vast amounts of recorded speech, picking up subtle patterns linked to vocabulary growth at different ages. This suggests the potential for spotting deviations from typical development earlier than traditional methods, which are often reliant on subjective assessments.
The ability to analyze toddler language, with its inherent complexity, using natural language processing techniques within these models, is particularly interesting. It opens doors to understanding how a combination of environment and individual cognitive abilities contribute to early language skills.
Furthermore, these models are not limited to just analyzing spoken words; they can also incorporate non-verbal communication like gestures, which are crucial to a toddler's early language journey. This multi-modal approach offers a more holistic view of language acquisition than simply counting words.
One promising aspect is that the computational speed of these networks allows them to pinpoint potential developmental landmarks by examining subtle speech transitions and phonetic changes. This might lead to identifying critical periods for intervention and support, helping to maximize the impact of any support offered.
It seems that the development of expressive (speaking) and receptive (understanding) language skills doesn't always happen in perfect sync. Machine learning models can help illustrate that some toddlers may understand language earlier than they can express it, highlighting a potential disconnect that might not be readily apparent through other observations.
It's also quite surprising that machine learning can pinpoint outliers in language development. This offers a more systematic way to identify toddlers who could benefit from early intervention programs tailored specifically to language development, potentially improving the efficiency and effectiveness of such programs.
As these machine learning models evolve, a key focus is increasing the generalizability of their findings across different demographic groups. This could pave the way for identifying universal language acquisition patterns and reveal how socioeconomic factors play a role in language development.
We're also seeing how machine learning models can shed light on the impact of bilingualism on toddler language acquisition. The research suggests that exposure to multiple languages can lead to unique developmental trajectories, potentially challenging traditional norms that often focus on monolingual language acquisition.
In conclusion, the use of machine learning in toddler language development offers a new window into understanding this complex process. It highlights the potential for improving how we support language learning, but also brings ethical considerations to the forefront. How can we ensure that these tools are used responsibly in educational settings to make sure that every child receives the language support they need? There's a lot of potential here but also a need to proceed thoughtfully as these models evolve and find broader application.
Neural Network Models for Analyzing Infant and Toddler Development Patterns A Data-Driven Approach - Deep Learning Framework Detects Early Signs of Developmental Delays
Deep learning frameworks are proving valuable in identifying early signs of developmental delays, particularly in infants born prematurely. These frameworks analyze brain scans and other data to detect subtle patterns that may indicate atypical brain development. Using a combination of labeled and unlabeled data for training, these models are improving their ability to predict cognitive challenges. However, a major hurdle remains: the need for extensive, labeled medical datasets to refine these models and make them more accurate. This field is pushing the boundaries of how we understand early brain development, but it also highlights the inherent complexities and data limitations we face in designing interventions for very young children. While promising, further development and refinement of these methods are essential before widespread clinical use can be considered. The potential benefits of early detection are significant, but the challenges of data collection and model accuracy need careful consideration in this evolving research area.
Deep learning approaches are being explored for their ability to analyze extensive datasets related to a child's motor development, potentially uncovering subtle signs of developmental delays more accurately than traditional methods. These models can sometimes identify shifts in neural connections linked to motor abilities up to six months before these changes become apparent in a child's behavior, highlighting the value of early, proactive monitoring.
The strength of these neural networks extends beyond just analyzing brain scans. They have the potential to incorporate diverse data sources, such as genetic information or environmental factors, to build a more holistic understanding of the factors that shape a child's development. Research suggests that deep learning models are adept at capturing the complex, non-linear patterns of neural pathway development during infancy, which may not be readily apparent using more simplistic analytical methods.
The computational speed of deep learning models is a considerable advantage, enabling rapid processing of MRI data. This speed could be particularly valuable in clinical settings, potentially allowing for real-time feedback and analysis during assessments. These models aren't static; they can continuously adapt and refine their accuracy as more data becomes available, providing a dynamic alternative to the use of fixed developmental benchmarks.
It's noteworthy that the initial applications of these models have shown potential in predicting not just motor skills but also aspects of cognitive development, further emphasizing the intricate connection between different developmental domains in early childhood. While promising, preliminary findings hint at potential variations in patterns across various demographic groups, highlighting the crucial need for inclusivity and careful consideration of equity in developmental research.
Ultimately, the application of these deep learning frameworks in clinical practice could lead to a more individualized approach to interventions. Instead of relying solely on broad developmental categories, we could move towards personalized developmental plans, each tailored to a child's unique profile. As this area of research continues to advance, ethical considerations remain a key focus. Issues of data privacy and responsible use of sensitive information must be addressed through the establishment of clear guidelines to ensure these technologies are applied in a way that prioritizes the well-being and safety of children.
Neural Network Models for Analyzing Infant and Toddler Development Patterns A Data-Driven Approach - Pattern Recognition Systems Monitor Child Behavior Changes
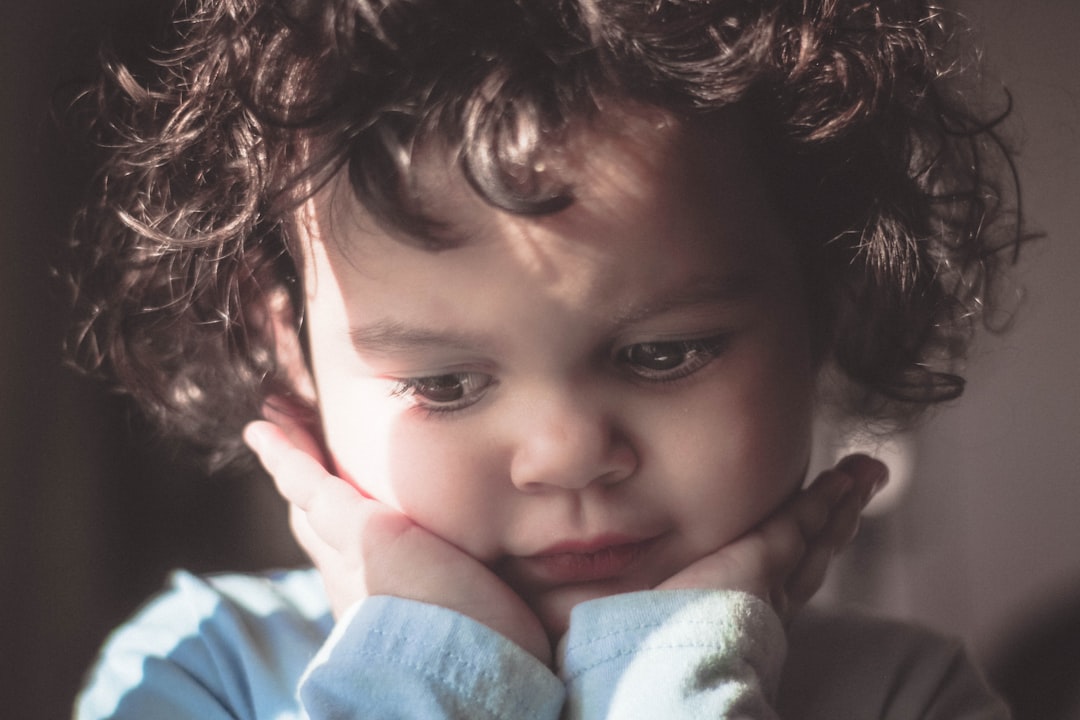
Pattern recognition systems are becoming more sophisticated in their ability to track shifts in children's behavior. These systems, powered by machine learning and neural networks, analyze intricate patterns in various data sources. This includes things like how a child looks at things (gaze patterns), how they move (motor skills), and even how they use language. By identifying these patterns, the systems can help categorize developmental stages and flag potential issues like cognitive or language delays early on. Combining different types of data like video and audio can paint a more detailed picture of a child's behavior and the associated emotional signals that impact their development.
However, it's important to recognize the ongoing hurdles with this technology. Ensuring data quality is crucial, and large amounts of training data are needed to make these systems reliable. There are also ethical considerations related to the collection and use of sensitive data on children that need careful attention. As this area of research matures, further refinement of these pattern recognition models will be key to realizing their full potential in real-world settings and ensuring they're used responsibly.
Pattern recognition systems are increasingly being used to monitor and analyze children's behavior, offering a new lens through which to understand development. These systems, powered by machine learning algorithms, are constantly refining their ability to identify patterns in how children behave, potentially leading to more personalized insights into a child's developmental journey.
One area where these systems are proving useful is establishing baseline behavioral norms. This allows researchers and clinicians to more easily spot deviations from typical development, which might point to developmental concerns. This continuous monitoring approach is a shift from the more traditional one-time assessment model. These systems are not limited to a single data type either. Instead, they can integrate data from different sources, like videos of children playing, parent-reported questionnaires, or even physiological data like heart rate. The benefit is a richer picture of child behavior and, as a result, more robust predictions.
Interestingly, the patterns these systems identify can sometimes serve as early warning indicators of developmental delays or disorders. For example, shifts in a child's social interaction or play patterns might be an early sign that requires a closer look. Furthermore, automated classification of these patterns can often be more precise than human observation, reducing the possibility of subjective biases that can creep into traditional assessment methods.
A key aspect of these systems is their ability to analyze changes in behavior over time. This temporal analysis allows researchers to detect not just the behaviors themselves but also how those behaviors evolve and change over time. This can reveal trends and cyclical patterns that might not be visible in snapshots of behavior.
The application of these systems is also extending beyond the research laboratory. We're seeing their use in educational and clinical settings where they can inform individualized intervention strategies, providing real-time data about a child's behavior to guide support and interventions. However, these systems are also revealing how diverse cultural contexts can shape child development. Data collected using these methods suggests that developmental milestones can vary widely across cultures, underscoring the role that environment plays in behavior.
Despite the significant potential, a key challenge is the need for high-quality, labeled data to refine these models. This is especially true when it comes to enhancing the accuracy of these systems across different populations. Furthermore, as these systems become more prevalent, important ethical questions emerge. Concerns about data privacy, particularly sensitive information related to children's development, and potential impacts on parental perceptions of child development need careful consideration. It's crucial to establish clear guidelines to ensure these systems are used responsibly and in a way that prioritizes children's well-being. While still a relatively new area of research, the use of pattern recognition systems holds considerable promise for enhancing our understanding of child development.
Neural Network Models for Analyzing Infant and Toddler Development Patterns A Data-Driven Approach - Data Analytics Predict Growth Curves Based on Historical Markers
Data analytics, particularly when combined with predictive modeling, offers a novel approach to predicting developmental growth curves using historical data. This is increasingly relevant when examining the intricate patterns of infant and toddler development. By analyzing large datasets of developmental markers, researchers can project future milestones and get a more in-depth understanding of how children typically grow. Neural networks, with their capability to discern complex patterns and relationships within datasets, are proving exceptionally valuable in this field. They facilitate the detection of potential developmental issues at an earlier stage compared to traditional methods. The move towards data-driven approaches provides a more objective and consistent alternative to methods heavily reliant on subjective observations. As research advances, integrating diverse datasets could further refine the accuracy and personalization of developmental assessments, potentially leading to more effective early intervention strategies tailored to individual children. However, it's important to be aware that this is a rapidly evolving field with ongoing refinements.
Data analytics, powered by the processing capabilities of modern computers, has begun to reveal the hidden predictive power within historical markers of infant and toddler development. It's quite intriguing that these methods can foresee developmental growth curves with some degree of accuracy. This means we might be able to predict future milestones, sometimes even spotting potential delays months before they are noticed through traditional observation and assessment.
This is further complicated by the fact that early development, it turns out, often doesn't follow a simple, linear path. It is more nuanced, with skills emerging out of sequence and in unexpected ways. This inherent complexity is something that neural network models are well-equipped to handle. Their ability to learn intricate relationships within datasets allows them to capture this non-linearity effectively.
Traditionally, developmental assessments have relied on the analysis of a single data type. Modern predictive models, however, are changing the game. They can draw upon a rich mix of information—including genetics, environmental factors, and behavioral cues—to create a more complete picture of the multifaceted influences on a child's development. This is a significant departure from older methods that often lacked this breadth of information.
The computational muscle of these analytics systems allows them to process massive amounts of data in a fraction of the time it would take a human researcher. This remarkable speed gives us insights at a pace that wasn't previously possible. We can now analyze and understand trends far quicker than was feasible before.
Beyond physical milestones, these data-driven approaches are also shedding light on cognitive development. The models we're developing aren't just tracking motor skills; they can potentially predict how cognitive abilities might unfold as well. This opens up a fascinating new angle, hinting at the intricate connections between different developmental domains in early childhood.
Moreover, sophisticated pattern recognition systems are able to detect subtle behavioral shifts that might not be easily spotted by humans. For example, shifts in how a child looks at things, or how they respond to certain stimuli, can reveal potential developmental needs before they are readily apparent in their overt actions.
While we see patterns, this type of research has also shown that developmental milestones aren't universally fixed. There's evidence that cultural contexts significantly impact the timing and nature of these milestones. It’s an important reminder that developmental assessments must account for the child's unique environment and background.
The insights gained from predictive models also pave the way for more individualized interventions. We can potentially tailor early childhood programs more effectively by identifying specific areas where a child might need support. These individualized interventions could offer a more refined and potentially powerful approach to helping children reach their full potential.
Machine learning models are dynamic, constantly adapting and improving as we feed them new data. This continuous learning process is in stark contrast to the fixed benchmarks of traditional assessments. This ability to adapt provides a far more flexible and fluid understanding of development.
The potential benefits of using these data-driven techniques are significant, but they also bring ethical challenges. We need to carefully consider the privacy and security of the sensitive information we collect. Establishing responsible guidelines for data collection and use will be crucial to harnessing the potential of predictive analytics while protecting the children whose data we're analyzing.
Neural Network Models for Analyzing Infant and Toddler Development Patterns A Data-Driven Approach - Computational Methods Transform Traditional Developmental Assessment
Computational methods are transforming how we assess developmental milestones in infants and toddlers. Traditional assessment methods, often reliant on direct observation, are being augmented—and in some cases, replaced—by a more data-driven approach. This shift leverages sophisticated mathematical models and machine learning, particularly neural networks, to analyze a wealth of data. This data can include behavioral observations, brain scans, and even language samples, allowing researchers to uncover complex patterns that may not be readily apparent through simpler methods.
The use of these computational approaches allows for the earlier detection of potential developmental delays. Neural networks can detect subtle alterations in brain activity and cognitive function, often before these changes are visible in a child's outward behavior. These models can also help create a more complete picture of development by integrating various types of data into a holistic understanding of an individual's developmental journey. This opens up exciting possibilities for individualized interventions, where strategies can be tailored to a child's specific needs and projected developmental path.
While these advancements are promising, they also bring a set of ethical questions. As these methods become more integrated into educational and clinical settings, it becomes vital to establish guidelines for responsible data collection and usage, particularly concerning the privacy and security of sensitive data relating to children. There is a need to carefully consider how this technology is implemented and to ensure that its use is equitable and beneficial for all children.
Computational approaches are increasingly transforming how we understand and assess child development, moving beyond traditional methods that often rely on subjective observation. Neural networks, a core component of many of these new methods, can analyze diverse types of data, including behavioral, genetic, and environmental factors. This opens doors to building a more comprehensive model of development that considers a wider range of influences. For instance, we can now utilize these methods to understand previously hidden elements influencing language acquisition, such as socioeconomic backgrounds and parental involvement, which were often difficult to quantify in conventional settings.
Perhaps the most startling aspect is the predictive power of some of these models. Some can identify potential developmental delays, particularly in motor skills, an astonishing six months prior to their being noticeable in a child's behavior. This allows a significant window of opportunity for early interventions. Furthermore, these networks have shown the ability to model the non-linear nature of developmental paths, a feat that has been challenging for earlier models which often rely on simpler, linear projections of development. This is particularly important as we realize that many skills develop in a complex and less straightforward manner than previously thought.
Another benefit of using computational methods like machine learning models lies in their ability to analyze large volumes of data with remarkable speed. For example, in language development assessments, they can quickly process vast amounts of audio recordings—thousands of hours worth—in a small fraction of the time that it would take a human expert. This not only speeds up the diagnostic process but could also potentially lead to greater accuracy. Similarly, the use of pattern recognition systems to analyze children's behaviors, including their gaze, movements, and language use, offers a new means of quantifying and objectifying behavioral assessments, mitigating some of the biases that can arise in more traditional, observational assessment approaches.
Moreover, these networks are capable of pinpointing outlying patterns in developmental pathways, revealing unique trajectories that may not be captured by typical developmental benchmarks. This allows for targeted interventions and support for children who may need individualized approaches to therapy or learning. It also facilitates the shift from one-time assessments to continuous monitoring of child development, providing a more dynamic perspective that considers the ongoing changes in a child's development.
These evolving computational models could change how we understand and define developmental milestones. Early evidence hints at the possibility of creating culturally sensitive assessments that consider variations in developmental trajectories across diverse societal and cultural settings. They can also help uncover the complex interplay between domains like motor skill development and cognitive development, highlighting that these elements are interconnected and do not progress in isolation. While there's significant promise in these new methods, it's crucial to continue to evaluate and refine them while being mindful of the ethical implications related to data privacy and the potential for biases in data collection and model development.
Create AI-powered tutorials effortlessly: Learn, teach, and share knowledge with our intuitive platform. (Get started for free)
More Posts from aitutorialmaker.com: