Create AI-powered tutorials effortlessly: Learn, teach, and share knowledge with our intuitive platform. (Get started for free)
The Evolution of Sustainability From Brundtland Commission to Modern AI Applications in Environmental Monitoring
The Evolution of Sustainability From Brundtland Commission to Modern AI Applications in Environmental Monitoring - The Brundtland Report 1987 Establishes Global Sustainability Framework
The 1987 Brundtland Report, from the World Commission on Environment and Development, became a critical point in how we understand sustainable development. It connected environmental damage directly to poverty and overconsumption. Led by Gro Harlem Brundtland, the report pushed for a joined-up approach that tried to balance economic progress with looking after the environment and creating social fairness, which called for immediate action worldwide. By introducing the phrase "sustainable development," the document urged countries to think long-term, ensuring that present actions didn't harm future generations’ prospects. Its impact can be seen in later global environmental discussions like the 1992 Earth Summit, and its ideas continue to influence how sustainability is tackled today, including the use of AI technology. The report's conclusions highlight the continued need for a holistic, interconnected view of environmental, economic, and social problems, and how they are interlinked when seeking long term sustainability.
The 1987 Brundtland Report, formally called "Our Common Future", signified a move away from isolated environmental worries towards an interconnected understanding of global predicaments. This document, a joint effort by over one hundred specialists from different backgrounds, underscored the need for many perspectives when dealing with intricate worldwide problems, revealing the interdisciplinary core of sustainability. Critically, it presented the widely-used explanation of sustainable development: meeting the current needs without undermining the ability of future people to meet theirs. This served as a central guideline for decision-makers internationally. The Commission was headed by Gro Harlem Brundtland, a former Norwegian Prime Minister, whose role shaped the basic notions of modern conversations on sustainability. The report highlighted that financial, social, and environmental issues are inseparable, urging countries to deal with these all together, an idea which altered international policy discussions. It pointed out certain key areas – such as lessening poverty, healthcare, and environmental protection – and suggested feasible approaches. These ideas formed the basis of international treaties later on. The report had a key influence in the development of the UNFCCC of 1992, an important milestone in global environmental management. Moreover, it emphasized the importance of regional and native knowledge, accepting that solutions must involve local communities impacted by environmental decay. Going against the then usual method of decision making from the top, it argued for more public involvement in environmental policy, encouraging democratic decision making in planning for sustainability. Engineers and technologists are still pushed by the concepts outlined to develop overall solutions for sustainability, often requesting innovation in fields such as resource handling and energy efficiency.
The Evolution of Sustainability From Brundtland Commission to Modern AI Applications in Environmental Monitoring - From Environmental Protection to Triple Bottom Line 1990 2005
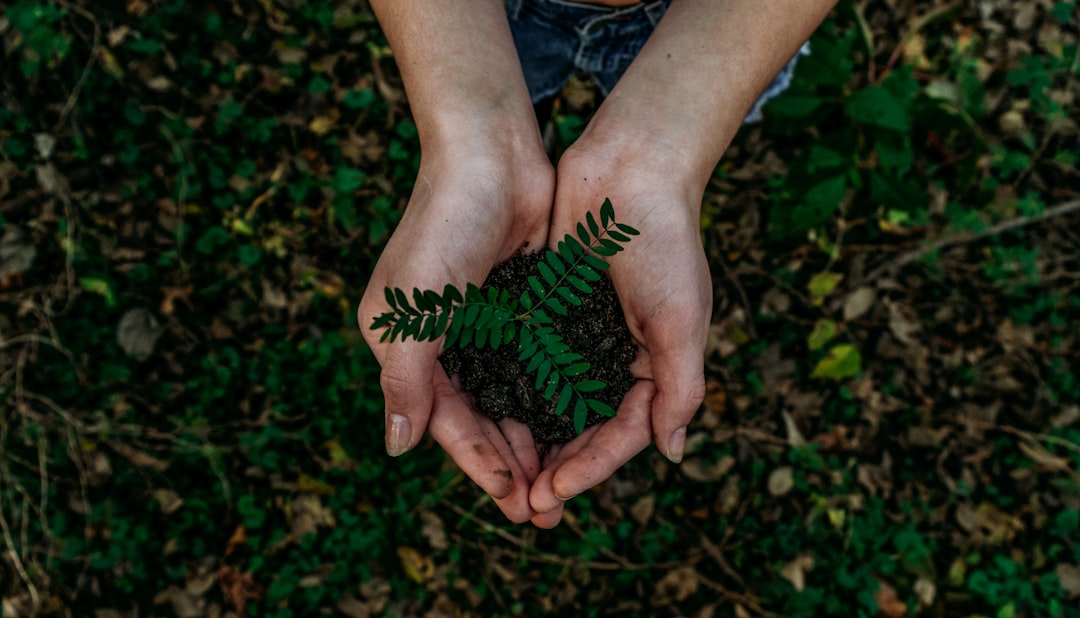
From 1990 to 2005, a notable shift occurred in the approach to sustainability. The idea of simply protecting the environment began to give way to a broader view, with the introduction of the Triple Bottom Line (TBL) by John Elkington in 1994. This framework pushed the conversation to consider not just the environment but also the economic and social consequences of actions. Businesses and other groups were encouraged to look at their impact through these three lenses. This change demonstrated a growing appreciation that environmental, social, and economic issues are linked. While the TBL gained traction, it also faced criticism, mainly around how it could be implemented and whether it was truly achieving its aim. This period was important because it showed a move towards seeing sustainability as something complex, and this laid the foundation for later developments and debates.
In the 1990s, the concept of the "Triple Bottom Line" (TBL) emerged. Moving past pure profit calculations, the TBL pushed for companies to track their impact on society and the environment, a major shift in how success was measured. Around the same time, many companies started using Environmental Management Systems (EMS). These systems aimed to improve how businesses managed their environmental impact and followed existing rules and standards. The 1990 to 2005 period also saw the growing presence of Corporate Social Responsibility (CSR) programs. Here, businesses voluntarily sought to improve society, a tactic which interestingly tended to improve the public perception of their brand and boost consumer confidence. The European Union’s Eco-Management and Audit Scheme (EMAS) in 1995, pushed for increased transparency, as organizations were encouraged to show their environmental performance and adopt more sustainable operational methods. The 1992 Rio Earth Summit also created Agenda 21, a plan which encouraged local governments to develop their own sustainable goals. This led to changes in how cities were designed and managed from an engineering standpoint. Green technologies took off in the early 2000s, which influenced various sectors including building and manufacturing, leading to the development of new energy-efficient systems and better and more sustainable materials. Life-cycle analysis (LCA) grew in popularity. Engineers started to evaluate how products affect the environment from production all the way through to disposal. The "ecological footprint" concept became a way to measure humanity's demand on the planet, prompting businesses and governments to examine resource usage and work towards reducing waste. The establishment of the Global Reporting Initiative (GRI) in 1997 promoted environmental accountability as thousands of organizations worldwide began using the new GRI framework for sustainability reporting. At the same time, increasing awareness of biodiversity loss resulted in the protection and rehabilitation of ecosystems, prompting engineers to modify project designs to protect delicate habitats and reduce the impacts of any planned construction.
The Evolution of Sustainability From Brundtland Commission to Modern AI Applications in Environmental Monitoring - AI Powered Satellite Monitoring Tracks Global Forest Loss 2020
AI-powered satellite monitoring has become a vital method for observing worldwide forest loss, with 2020 serving as a key example of its capabilities. These systems utilize sophisticated AI algorithms that process vast amounts of high-resolution satellite imagery, along with other data types, which results in more accurate and quicker assessments of deforestation. This technology goes beyond simply offering real-time monitoring as it can also identify potential areas of concern, enabling more proactive steps to address environmental changes. The use of AI here marks a progression in sustainability work, building on established ideas while facing current difficulties in how we look after forest ecosystems. Given the pace of technological progress, it is more important than ever to apply these advancements to ensure more effective environmental management.
AI-enhanced satellite systems are now able to sift through huge volumes of high-definition imagery – more than 20 terabytes daily - making forest loss detection much faster and more detailed. These systems use advanced deep learning to tell the difference between diverse plant types, highlighting changes from logging, farming, or natural events, hitting over 90% accuracy in tests. Adding AI to satellite data has dramatically cut the time taken to spot deforestation from months to just a few days, which in theory could help swift action from relevant teams on the ground. Researchers are now using AI to detect forest loss in far-flung and hard-to-reach places, spots which were mostly ignored in the past due to access issues. AI-driven monitoring not only tracks forest loss but can also zero in on its causes, revealing links to human activity, which is critical for specific conservation steps. Data provided by AI allows for tracking illegal logging more or less as it happens, and so law enforcers can react faster and cut down on illegal behavior. By combining satellite information with other datasets related to geography and socioeconomics, recent AI developments allow a wider view of forest ecosystems. This could possibly improve how we write environmental policy. AI allows scientists to spot forest loss down to 30 meters - a huge improvement on earlier tech. The training information necessary for AI models to track forest loss has dropped considerably, due to advancements in machine learning, resulting in more efficient models and less manual help needed. However, even with this powerful tech, problems exist in how accessible and standardized the data is. The reliability of long-term forest monitoring is negatively impacted by satellite coverage being inconsistent and satellite image quality being inconsistent.
The Evolution of Sustainability From Brundtland Commission to Modern AI Applications in Environmental Monitoring - Machine Learning Models Predict Climate Change Impact 2023
As of 2023, machine learning models have become increasingly important for anticipating the consequences of climate change. They use sophisticated algorithms and ways of pulling information from data to understand difficult environmental problems. These AI-based systems are not just better at predicting the weather; they also give us important data on how ice is moving, where pollution is coming from, and how to improve farming, all of which are essential for reducing environmental damage. The need for these solutions is clear, with climate change causing significant economic damage of over $500 billion every year. Working together across different scientific fields is key to using machine learning well in climate work. This makes sure that different types of expertise come together to deal with this global problem. Ultimately, the continuing climate crisis requires a move from just talking to making real changes, by using AI and machine learning to reshape how we monitor the environment and pursue sustainability.
Machine learning is increasingly being used to project the effects of climate change. By using varied algorithms like neural networks and data extraction, it's possible to enhance climate forecasting by analyzing satellite data with high efficiency, reducing analysis time from months to just days. AI systems can now discern forest changes with considerable precision, achieving over 90% accuracy in differentiating plant types and spotting subtle environmental changes through processing over 20 terabytes of satellite imagery daily. Recent methods can integrate geographical and socioeconomic data to create multidimensional views informing environmental policies rather than focusing purely on forest cover. The ability to monitor losses in near real time, allows quick responses to illegal logging, which is good, although some may see it as more policing. Previously ignored remote areas can now also be monitored by advanced AI algorithms potentially altering the scale of environmental efforts. While the training data requirements have come down, inconsistencies in satellite data still hamper some long-term efforts raising issues about data reliability. This kind of system can move past simply monitoring and examine deforestation causes, pointing directly to human activities and enabling targeted approaches. The tech originally developed for forestry is now being used in other fields like agriculture and urban planning, which highlights the broad value of machine learning in many aspects of environmental management.
The Evolution of Sustainability From Brundtland Commission to Modern AI Applications in Environmental Monitoring - Real Time Environmental Data Collection Through IoT Sensors 2024
As of 2024, the use of IoT sensors for gathering real-time environmental data has become vital for sustainability efforts. These sensors allow for ongoing observation of conditions like air and water quality, giving important information on the state of our ecosystems. AI plays a key role in making these systems more effective, providing rapid data analysis that helps us respond to environmental problems faster. However, this reliance on technology brings up questions about the precision and availability of data, which are important for effective environmental management. As we move forward, it’s crucial to develop strong systems to make sure these technological advances truly support sustainability and public well-being.
By late 2024, the deployment of IoT environmental sensors is showing some striking developments. We're seeing very dense sensor deployments, especially in urban zones, with some areas sporting more than 20 sensors per square kilometer. This dense network provides hyper-local environmental monitoring that reflects the environmental variability within micro-climates. Also, it’s possible to now track some air pollutants practically live - sensors are updating their measurements every few seconds, which could be used to provide immediate public health warnings when something like a wildfire hits. Powering these sensors is also changing, with energy-harvesting systems like solar panels and kinetic energy converters starting to become the norm. These help keep sensors running without the need for constant battery changes, reducing maintenance. There also seems to be a move to make sensors interoperable – which means that various sectors from farming to town planning are starting to share the same environmental data, which improves decision making.
These networks are increasingly linked to Artificial Intelligence (AI). AI driven systems are now able to look at current data with machine learning algorithms to predict potential pollution problems or water shortages. Also interesting is how affordable sensor networks are now. Compared to just a few years ago, deployment costs have dropped by half, meaning even small communities can monitor their surroundings. In water management, smart sensors are showing promise too. These devices can flag leaks or monitor quality, enabling authorities to take action quickly to save on water loss and treatment bills. Quality is also key in data collection and new machine learning approaches are being used to improve sensor reliability – something that's really important to researchers. We are also starting to see interesting links between satellite-based remote sensing data and IoT data, giving us a better understanding of what's happening in remote areas – such as deploying sensors in buoys to monitor the conditions of the oceans. Even sensor design is improving with biodegradable and biocompatible materials being introduced, reducing waste and allowing deployment in delicate spots like forests without causing further disruption.
Create AI-powered tutorials effortlessly: Learn, teach, and share knowledge with our intuitive platform. (Get started for free)
More Posts from aitutorialmaker.com: