Create AI-powered tutorials effortlessly: Learn, teach, and share knowledge with our intuitive platform. (Get started for free)
The Rise of Interdisciplinary STEM Programs Bridging AI and Traditional Sciences in Higher Education
The Rise of Interdisciplinary STEM Programs Bridging AI and Traditional Sciences in Higher Education - AI and Biology Fusion Programs Gain Traction at Top Universities
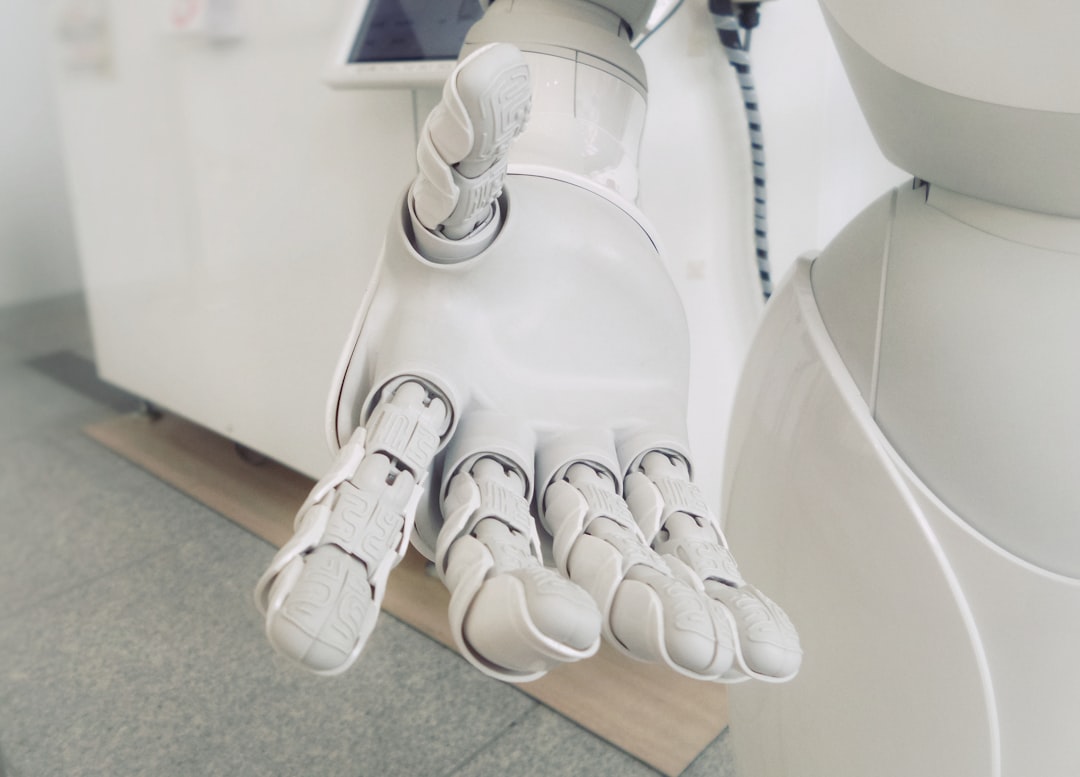
The intersection of artificial intelligence and biological sciences is rapidly gaining ground in top universities. These programs are a testament to the increasing understanding that a collaborative, multidisciplinary approach is essential in STEM fields. Universities are recognizing the transformative potential of AI within biology, incorporating it into courses and research to reshape how experiments are planned and data is analyzed. This integration not only promises advancements in research but also necessitates changes in how educators assess student comprehension in this evolving landscape.
The practical applications of this AI-biology fusion are evident in areas such as protein modeling and materials science, demonstrating its ability to improve outcomes. The integration of AI tools and techniques helps students develop skills relevant to cutting-edge research, potentially improving both their academic success and the overall quality of scientific inquiry. As education adapts to this new technological era, this partnership signifies a departure from more siloed academic practices, promoting a more interconnected and collaborative academic future.
The convergence of AI and biology is no longer confined to specialized research labs at top universities. We're seeing a wider adoption of AI methods into the very core of biology curricula, suggesting a growing recognition that the future of biological research and its applications necessitate interdisciplinary expertise. This trend is reflected in the emergence of courses that incorporate AI techniques into traditional biology subjects, better equipping students for the increasingly AI-driven job market in biotech and related fields.
One of the most compelling examples is the application of machine learning in drug discovery. AI models are now capable of predicting molecular interactions with a precision that outperforms older methods, accelerating the development of new medications. This is a crucial development in a field that is often characterized by lengthy and expensive research processes. Furthermore, breakthroughs in AI-driven genomics are allowing researchers to dissect complex genetic information at unprecedented speeds. This increased efficiency in processing genetic data opens doors to further innovations in personalized medicine, where treatments can be tailored to an individual's unique genetic makeup.
This interdisciplinary movement is also manifest in the growing number of joint degree programs combining biology with computer science or related fields. These programs provide graduates with a powerful skillset, enabling them to navigate the complexities of both biology and computational tools. Consequently, a new breed of specialists is being trained who can tackle complex biological questions using the tools provided by AI. The rapid processing capabilities of AI algorithms are transforming biological research in tangible ways. For example, the task of analyzing vast amounts of biological data like genetic sequences, which would have taken researchers months or years to accomplish previously, can now be completed within minutes. This increase in speed has both obvious and subtle implications for the direction and speed of biological research.
The rise of these hybrid approaches isn't just influencing academia. We are seeing bioinformatics startups led by alumni who have honed their skills in these university programs. These entrepreneurial ventures often develop innovative solutions for longstanding biological issues, potentially changing the future of biological industries. We're also beginning to witness more integrated approaches to ecological studies, where AI models can capture the complexities of both physical environments and species interactions with increased precision. This leads to new types of insights into biological systems that were simply inaccessible with older statistical approaches.
The growing presence of AI within biological research raises critical ethical considerations. Leading universities are realizing the need to incorporate discussions of bioethics into the education of biology and AI students. They understand the importance of ensuring that students grapple with the potential societal impact of their technological advancements.
Finally, we're seeing increased collaboration in research labs, with biologists and AI scientists working in tandem to develop new, innovative methodologies that leverage technology to unravel complicated biological questions. Universities are recognizing the power of AI to transform biological research and are beginning to equip their labs with sophisticated AI tools, accelerating the overall pace of scientific discovery. This trend suggests that the future of biological research may be intrinsically interwoven with advanced computational techniques.
The Rise of Interdisciplinary STEM Programs Bridging AI and Traditional Sciences in Higher Education - New Quantum Computing and Physics Interdisciplinary Degrees Launched
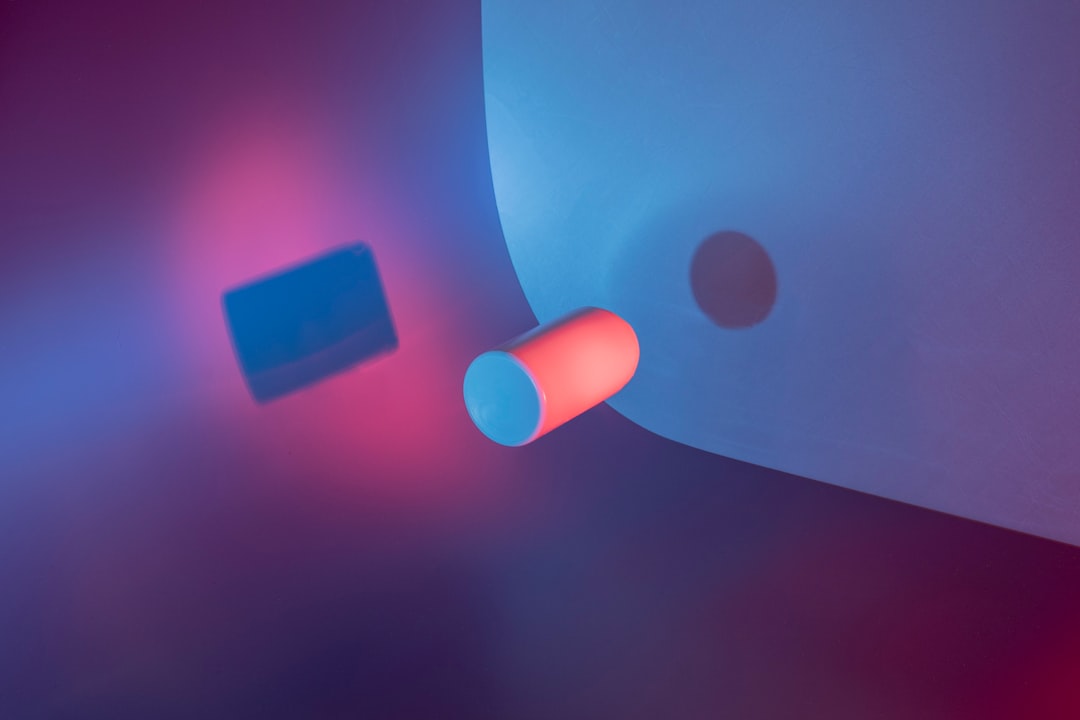
Universities are beginning to recognize the transformative potential of quantum computing and are responding by establishing new interdisciplinary academic programs. These programs combine the study of quantum physics with the development of quantum computing technologies, producing graduates who can navigate the complex intersection of these two rapidly evolving fields. For instance, Princeton University has introduced a PhD program that bridges quantum physics with information science, creating opportunities for deep research into the foundational principles and practical applications of quantum computing. Additionally, Columbia University offers a master's degree focused on quantum science and technology, catering to professionals who want to gain expertise in this field.
The growing emphasis on quantum information science is driven by its potential to revolutionize computational abilities and solve some of the most pressing challenges facing our world, from climate modeling to material science. The hope is that new quantum computing architectures will be able to address problems previously considered intractable. However, it is critical that these new academic offerings focus not just on the technical aspects of quantum computing, but also on developing an understanding of its broader implications and potential impacts. As we move towards a future where quantum computing plays a central role, it's increasingly clear that individuals equipped with this specialized knowledge will be essential to understanding and managing the possibilities it unlocks. These new interdisciplinary approaches to education are critical steps towards preparing the workforce for a world increasingly reliant on this burgeoning technology.
The emergence of new degree programs that blend quantum physics with engineering and computer science is a fascinating development. Universities are recognizing that the potential of quantum computing to revolutionize fields like cryptography and materials science necessitates a new breed of expert. This shift is fueled by the growing awareness that quantum algorithms can outperform classical ones in critical areas, like optimization and simulation, making a solid foundation in quantum mechanics crucial for future engineers.
Intriguingly, some studies suggest that grappling with the often counterintuitive principles of quantum physics can actually improve general problem-solving abilities in engineering. It's theorized that this exposure can promote a more adaptable and innovative mindset when confronting complex challenges. Furthermore, some universities are providing students with early hands-on experience with actual quantum computers, bridging the gap between theoretical concepts and practical applications.
The integration of quantum physics with AI methodologies is particularly exciting, leading to the development of increasingly sophisticated quantum algorithms. This development emphasizes the need for engineers to be proficient in both quantum mechanics and AI techniques, creating a new wave of hybrid skillsets. This push is reflected in research initiatives funded by partnerships between universities and industry leaders, generating breakthroughs in quantum-powered machine learning.
The implications of this interdisciplinary approach extend beyond the research lab. As quantum technologies continue to mature, graduates of these new programs are poised to fill a rising demand for specialists across sectors like finance and pharmaceuticals, where quantum computing can provide competitive advantages. However, the complexities of quantum systems necessitate new forms of collaboration. We are seeing universities establishing advisory committees that include physicists, computer scientists, engineers, and even ethicists to ensure that the curriculum fully addresses both the scientific and philosophical ramifications of this rapidly evolving field.
New research into quantum communications also suggests that phenomena like entanglement and superposition could dramatically impact information transmission efficiency. This makes the study of quantum communications an increasingly relevant domain for engineers. Although many engineering fields are rooted in quantitative approaches, the integration of quantum physics encourages a broader scope of thought, incorporating qualitative reasoning and ethical considerations alongside technical proficiency. This signifies a crucial shift in engineering education, preparing future engineers not only for technological challenges but also for the complex ethical dilemmas that often arise in this field.
The Rise of Interdisciplinary STEM Programs Bridging AI and Traditional Sciences in Higher Education - Data Science Integrated into Traditional Chemistry Curricula
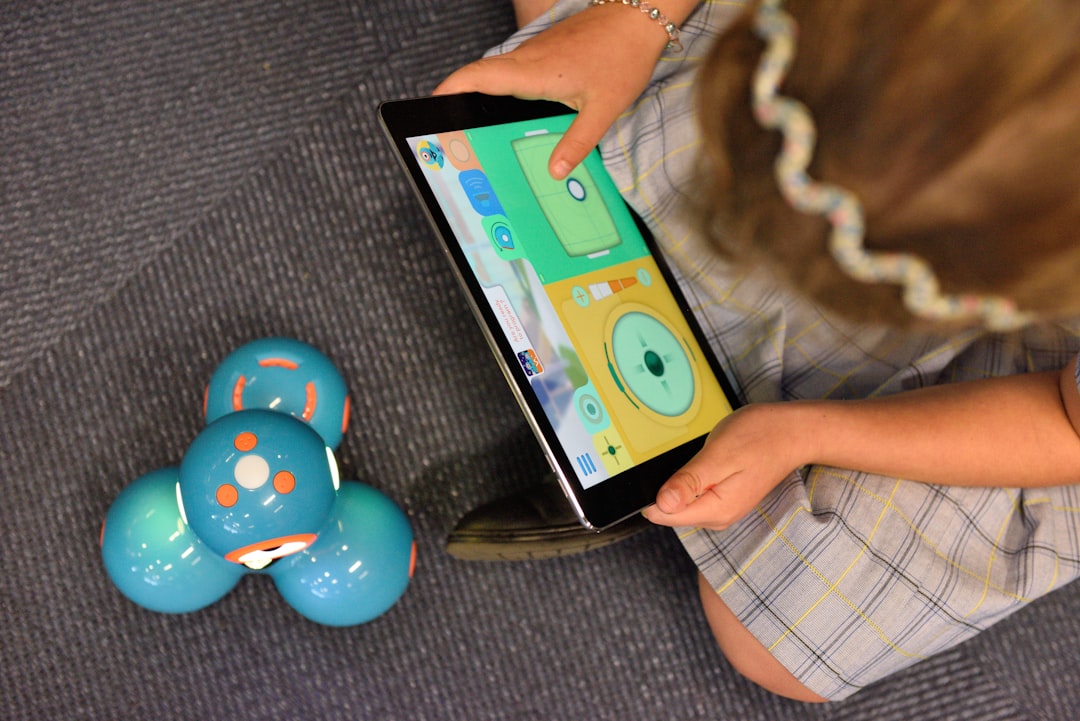
The integration of data science into traditional chemistry education is a necessary response to the changing landscape of the chemical sciences. Chemistry curricula are incorporating data analysis, computational methods, and automation, recognizing the need for students to develop advanced digital skills in this era of rapid technological change. This move towards interdisciplinary learning seeks to better prepare students for the modern chemical workplace and research environment.
However, the current implementation of these integrated programs often lacks a robust evaluation of how well different subjects are intertwined. It's unclear if the curriculum genuinely blends the disciplines in a meaningful way, which raises concerns about the overall quality and depth of this interdisciplinary approach. Despite these implementation issues, the push towards more unified and relevant STEM programs suggests a wider shift in how education is structured and delivered. Universities are recognizing that fostering a more interconnected approach is essential to prepare students for tackling the complex challenges of scientific inquiry, providing them with the multidisciplinary skillsets required in the modern scientific world.
The future of chemistry education, it seems, will likely heavily rely on a deeper integration of data science into its core. This integration will likely become central to adequately preparing future scientists and researchers for the demands of modern chemical research and innovation.
The integration of data science into traditional chemistry education is a response to the growing need for advanced computational skills within the field. This shift emphasizes the importance of data analysis, automation, and computational approaches in understanding and manipulating chemical systems. The growing use of sophisticated software and algorithms in modern chemistry labs highlights the necessity of chemistry students developing a proficiency in these digital tools.
This development stems from the broader trend of interdisciplinary STEM programs arising from perceived talent shortages and a desire to bridge the gap between academic learning and societal demands. While AI is being integrated across STEM fields, the application within chemistry is particularly impactful. For instance, it is becoming commonplace to employ machine learning within chemistry courses to improve the reliability of predicting reaction outcomes. Previously, this process relied heavily on trial-and-error methods, leading to inefficient research cycles. By incorporating data science, students can access vast datasets, learn to leverage pattern recognition techniques for discovering new materials, and build predictive models for things like drug interactions.
However, the integration of data science isn't without its challenges. It raises the issue of interdisciplinary collaboration, necessitating chemists to work closely with computer scientists and data analysts. Moreover, a greater emphasis on reproducibility and data sharing within chemistry research has emerged, pushing for transparency in methodology. The shift towards a data-driven approach has led to the development of "cheminformatics" which essentially uses informatics to process and analyze chemical data, changing the landscape of chemistry labs through virtual simulations and digital libraries. The rise of specialized tracks in chemistry degree programs focusing on data analytics reflects the growing need for students with a combined understanding of chemical principles and data science skills.
Interestingly, data science tools are also improving the ability to visualize and interpret chemical phenomena. For example, interactive 3D modeling of molecular structures can enhance understanding in ways that traditional 2D diagrams could not. Despite the obvious benefits of data-driven chemistry, it's crucial that educators address the potential limitations and biases within the data being used, ensuring that students develop a critical understanding of the tools they employ. Ultimately, the success of this interdisciplinary approach will depend on balancing traditional chemical understanding with new digital competencies, resulting in a future where chemists possess a powerful new toolkit for tackling complex chemical challenges.
The Rise of Interdisciplinary STEM Programs Bridging AI and Traditional Sciences in Higher Education - Robotics and Mechanical Engineering Programs Merge at Leading Institutions
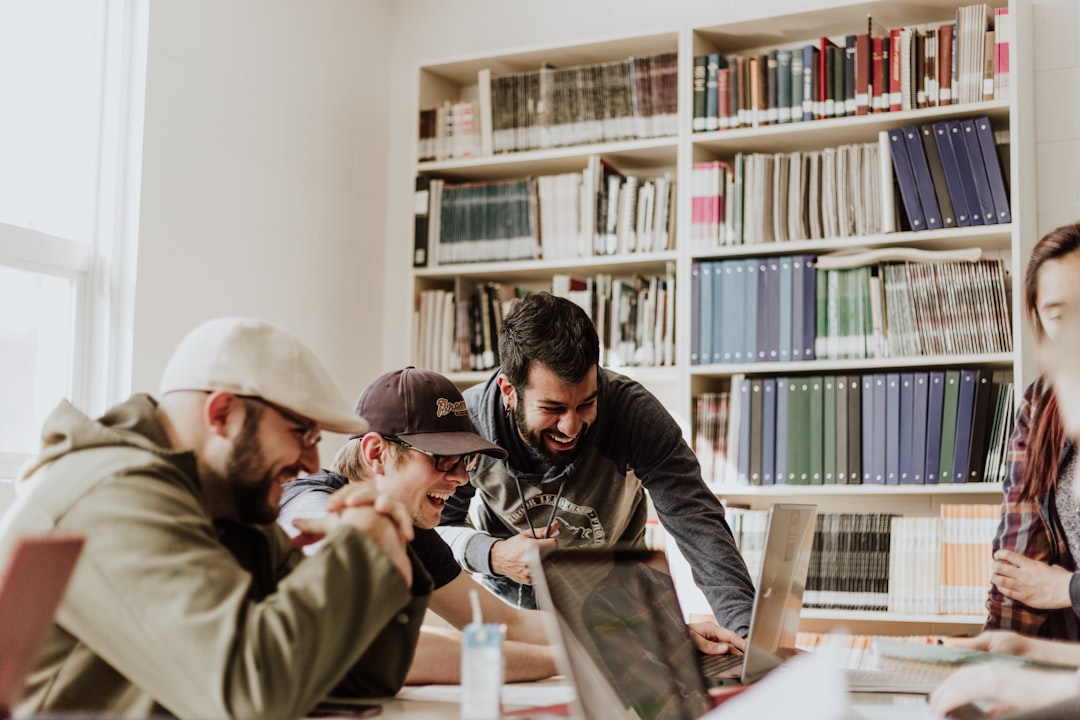
The convergence of robotics and mechanical engineering programs within leading academic institutions marks a significant shift in STEM education. Universities are increasingly recognizing that a more holistic, interdisciplinary approach is necessary to prepare students for the complexities of modern engineering. These merged programs emphasize hands-on learning and skill development in robotics, recognizing the growing importance of automation and advanced technologies in diverse engineering disciplines.
While this trend offers the potential for more engaging and forward-thinking curricula, it's crucial to consider whether the integration of robotics seamlessly connects with the core principles of mechanical engineering. There's a risk that the emphasis on robotics could overshadow or diminish the foundational aspects of mechanical engineering, potentially hindering a deep understanding of its principles. Ultimately, this movement reflects a wider trend towards greater flexibility and interdisciplinary expertise, but careful implementation is needed to ensure these programs produce engineers with both a broad skillset and a solid understanding of the fundamentals. The hope is that these changes prepare students to thrive in a professional landscape increasingly reliant on adaptable engineers who can readily navigate the interconnectedness of modern technologies.
Across leading educational institutions, we're witnessing a growing trend of merging robotics and mechanical engineering programs. This convergence is creating a new breed of "robotic engineer" – individuals equipped to design and build machines that can mimic human actions, pushing the boundaries of automation and AI integration in various sectors.
Some institutions are now embracing virtual reality (VR) within their robotics labs. Students can simulate robotic movements in a virtual environment before physical construction, which potentially reduces errors and improves the efficiency of the design process. This is an interesting approach, though whether it truly improves learning remains a point of inquiry for researchers in education.
Another intriguing development is the rise in emphasis on soft robotics within these combined programs. This focuses on the creation of machines using materials that can alter their shape and stiffness, which would make robots more adaptable and functional within complex environments. It will be exciting to see how far these innovations progress and where they prove most effective in real-world settings.
Interestingly, many mechanical engineering departments are now mandating that students complete "mechatronics" projects. These projects require students to blend principles of mechanical, electronic, and software engineering, fostering a more holistic approach to problem solving. It's logical that this approach is being adopted more widely, though one might wonder if there is a risk of diluting specialization in each core area if these skills are not further developed throughout the student's course of study.
Furthermore, research suggests that collaborative ventures between robotics and mechanical engineering students result in significantly enhanced team-based problem-solving skills. It seems likely that bringing students from different engineering perspectives together might spark more innovative solutions to complex problems. However, a deeper exploration of the benefits and drawbacks of this approach is necessary to ensure the optimal outcome in educational practice.
A surprising focus within these integrated programs is the area of human-robot interaction. Students are now trained in designing robots to work alongside humans, emphasizing considerations like safety and effective communication. While this is a welcome development given the increasing presence of robots in the workforce, it's crucial that there are continued discussions about the ethical implications of increased human-robot interaction and the potential impact on future work and society.
The integration of AI algorithms into the design of mechanical systems is also a major shift. This is leading to the development of innovative solutions for predictive maintenance, where robots can diagnose their own operating state and anticipate failures in advance. This has the potential to change engineering maintenance strategies quite drastically. It will be essential for researchers and industry to evaluate the reliability and effectiveness of this AI-powered predictive maintenance and to consider the implications of such a drastic shift.
However, the move towards interdisciplinary engineering programs is not without its criticisms. Some argue that a strong emphasis on these combined programs might compromise the depth of knowledge that students achieve in traditional mechanical engineering. It remains to be seen if the broad perspective offered by interdisciplinary programs compromises specialization, as there is a trade-off between breadth and depth of knowledge within these programs.
This new emphasis on robotics and mechanics integration has spurred a significant growth in partnerships between universities and industry players that utilize advanced robotics. This presents students with valuable hands-on experience, aligning their education with actual workplace demands, and potentially simplifying the transition from academia into the professional world.
Furthermore, the demand for robotics engineers extends beyond conventional engineering domains. Healthcare, for example, is actively employing individuals with combined robotics and mechanical engineering backgrounds to build assistive technologies and innovative tools for robot-assisted surgery. While robotics is often associated with manufacturing and industrial settings, its application in sectors like healthcare demonstrates its growing versatility and potential to address problems in a variety of fields. It's clear that as robots continue to become integrated into various facets of society, the engineers equipped to build and integrate these machines will become increasingly sought after.
The Rise of Interdisciplinary STEM Programs Bridging AI and Traditional Sciences in Higher Education - Environmental Science Departments Adopt AI-Driven Research Methods
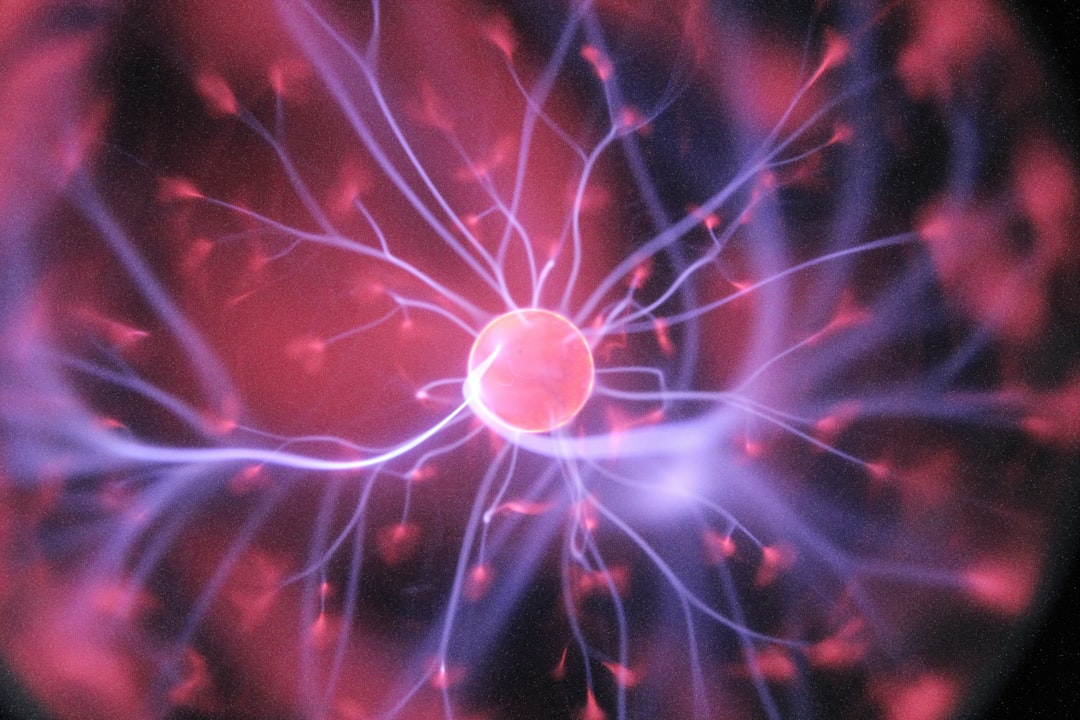
Environmental science departments are increasingly adopting AI-powered research methods, reflecting a broader shift towards incorporating advanced technologies into traditional scientific disciplines. This change is driven by a renewed focus on using AI to tackle critical environmental issues, ranging from climate change mitigation to ecological conservation. While AI's application in environmental science dates back to the mid-20th century, its use has significantly intensified in recent years, fueled by advancements in computing power and data availability.
Modern applications of AI in environmental research extend beyond early efforts, with a particular emphasis on large-scale data collection and analysis. This involves everything from analyzing satellite imagery to track global emissions to deploying smart sensors for localized environmental monitoring. Additionally, AI is being used to optimize the implementation of sustainable solutions, like improving energy efficiency in buildings and developing strategies for renewable energy deployment. These advancements are fostering greater interdisciplinary collaboration, as environmental scientists find themselves needing to integrate AI techniques into their established methodologies.
However, this incorporation of AI into the field isn't without its complexities. It necessitates a thoughtful approach to the ethical considerations that arise with the use of powerful computational tools for analyzing environmental data. There's a need to balance the potential benefits of AI with a critical awareness of its potential biases and limitations. As environmental science departments continue to integrate AI, navigating these complexities will be crucial to ensuring that these innovative tools are used responsibly and effectively to address the pressing environmental issues of the 21st century.
Environmental science departments are increasingly embracing AI-powered research approaches to boost their investigative capabilities and tackle complex environmental issues. The adoption of AI tools in environmental research has been a growing trend over the past decade, particularly when addressing challenges like ecosystem stability and resilience. While not entirely new—early applications emerged in the 1950s and gained traction by the 1980s—the sophistication and widespread availability of AI methods are driving a new wave of implementation.
Current examples of AI applications include improving building energy efficiency, tracking deforestation rates, and enhancing the effectiveness of renewable energy deployment. AI also enables large-scale environmental monitoring, utilizing satellite data for global emissions tracking and smart sensors for localized data collection. This integration highlights the rising importance of interdisciplinary STEM programs to foster collaboration between AI specialists and traditional science researchers.
AI methodologies are quickly becoming crucial tools for environmental scientists and engineers, facilitating both research advancements and practical field applications. Researchers are exploring promising areas for future collaboration, such as predicting and mitigating major environmental changes and studying how human well-being can coexist sustainably with the environment. The integration of AI into environmental research aligns with global sustainability objectives, including carbon neutrality and addressing climate change. Notably, AI's ability to access new datasets and improve data interoperability is crucial for driving deeper understanding and discoveries in environmental science.
However, there are also challenges and questions that arise with these advancements. For example, there's the concern of potential biases inherent in AI models when trained on incomplete data. It's important for researchers to be aware of this, and to be cautious about interpreting the outputs of AI models in a way that might lead to inaccurate or misleading conclusions about ecosystems or species health. Furthermore, while AI can help with identifying environmental problems, it needs careful integration with ecological knowledge to translate complex AI outputs into actionable conservation and policy decisions. It will be interesting to see how these challenges are addressed as the use of AI continues to grow within environmental sciences.
The Rise of Interdisciplinary STEM Programs Bridging AI and Traditional Sciences in Higher Education - Mathematics Departments Introduce Machine Learning Specializations
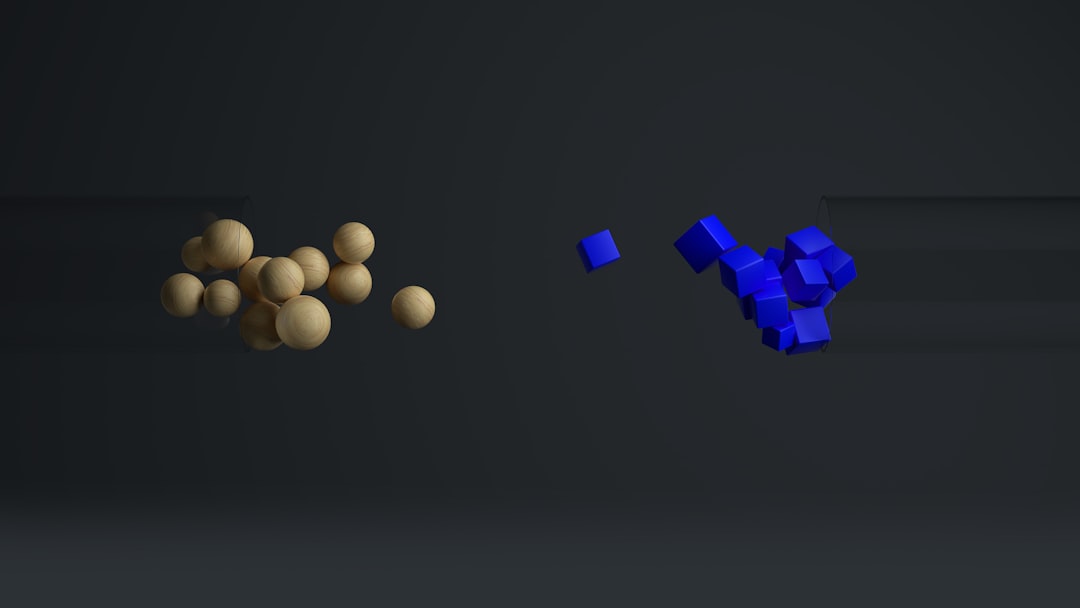
Mathematics departments are increasingly incorporating machine learning into their programs, developing specialized tracks that reflect the expanding connection between mathematics and artificial intelligence. These initiatives are driven by a desire to equip students with the necessary skills for success in today's data-centric world. This shift not only connects established mathematical knowledge with advanced AI technologies but also reflects a broader trend within STEM toward interdisciplinary learning and collaboration. Yet, implementing these specializations presents obstacles. Educators face the challenge of effectively blending diverse AI approaches with existing mathematical coursework, guaranteeing a solid grasp of both. The emergence of machine learning specializations within mathematics departments ultimately responds to evolving industry requirements and an understanding that a thorough knowledge of interwoven disciplines is crucial for future success across various sectors. While this can be a positive trend, ensuring a balance between traditional math foundations and the new skills of AI is a complex challenge.
Mathematics departments are increasingly incorporating machine learning specializations into their programs, moving beyond just teaching algorithms to focusing on the underlying mathematical foundations. This means a stronger emphasis on core concepts like optimization, statistics, and linear algebra, which are essential for practical applications of machine learning. It's fascinating to see some departments using gamification – incorporating competitive elements and interactive simulations – within these courses to make complex topics more engaging and understandable. It's a clever way to connect abstract concepts to practical scenarios.
Interestingly, the introduction of machine learning specializations has broadened the student base in these departments. It's not just attracting traditional math majors; we're also seeing students from physics, engineering, and even the social sciences drawn to these programs, which speaks to the versatility of machine learning and its applications across diverse fields. Many mathematics departments are fostering connections with tech companies to provide real-world case studies and datasets for their students. This collaborative approach gives students invaluable experience working with live data and taking on real-world projects, a significant step in making the curriculum more relevant to industry needs.
However, a crucial question arises: do current mathematics programs effectively incorporate the ethical dimensions of machine learning? With increasing awareness of bias within AI algorithms, there's a call for more coursework addressing the societal implications of these technologies. It seems that this is a necessary and urgent development within this curriculum.
What's intriguing is that the rise of machine learning within mathematics is actually fostering advancements in pure mathematics research. The need for new algorithms and improved data models is driving mathematicians to explore fresh areas in combinatorics and graph theory, which has the potential to enrich both theoretical and applied mathematics. Some programs have even introduced capstone projects that require students to collaborate with other disciplines—such as biology or economics—to tackle complex issues using machine learning. This approach is a smart way to enhance students' ability to communicate and work effectively in diverse teams, which is increasingly important for success in modern work environments.
The emphasis on interpretability of these models is also growing. Students aren't just learning to develop models, they're learning how to communicate the results in a way that is accessible to non-technical audiences. This emphasis is important because businesses increasingly need models that are transparent and understandable by people outside of the data science domain. The trend towards integrating machine learning into mathematics is reflective of a broader shift toward project-based learning. It's a welcome move away from solely relying on lectures and exams, with a focus on building the practical skills that are readily applicable across a wide range of sectors.
As machine learning continues to develop, it seems that mathematics departments are constantly reviewing and updating their curriculum. It's becoming evident that a dynamic interaction between pure mathematics and applied computation is crucial for a complete education in the 21st century. It's a great time to be a mathematician or someone interested in a career that touches upon the areas of applied mathematics and data science.
Create AI-powered tutorials effortlessly: Learn, teach, and share knowledge with our intuitive platform. (Get started for free)
More Posts from aitutorialmaker.com: