Understanding Radian Notation in AI-Driven Geometric Processing A Practical Implementation Guide
Understanding Radian Notation in AI-Driven Geometric Processing A Practical Implementation Guide - Converting Standard Angles to Radians Through Matrix Operations in Enterprise AI
Within the realm of enterprise AI, especially when dealing with geometric processing, the integration of radians into matrix operations is increasingly important. This integration offers several key advantages. Radians provide a more natural way to represent angles, especially in situations involving rotations and dynamic changes in orientation, creating a more unified computational environment.
The transition between linear and rotational motions becomes easier to manage when using radians. This smooth integration facilitates the accurate and efficient calculations often required in AI-driven geometric processing. Furthermore, the conversion from standard angle measurements (degrees) to radians can be handled smoothly through readily available functions within matrix operations, ensuring that calculations are consistently accurate across different AI applications. By understanding how to perform this conversion within matrix operations, we can simplify complex mathematical expressions and effortlessly incorporate trigonometric functions into AI algorithms, enabling more sophisticated geometric manipulations.
1. We can leverage the fundamental relationship between radians and degrees – π radians being equivalent to 180 degrees – to devise a matrix-based conversion method. This highlights the inherent mathematical link between the two systems.
2. By integrating a scalar constant within a matrix, we can perform the conversion of a standard angle to radians within a single matrix operation. This approach proves especially useful for systems designed around rotation transformations.
3. Applying matrix operations for angle conversions can potentially enhance performance in applications demanding real-time processing, such as in robotics or simulations involving dynamic physics. This might be an area for further investigation.
4. The benefits of matrix-based angle conversions extend beyond 2D scenarios, offering a consistent and potentially efficient approach in higher-dimensional contexts found in fields like computer graphics or machine learning where multi-dimensional rotations are commonplace.
5. Matrix multiplications don't just convert angles; they also maintain fundamental geometric properties. This ensures the preservation of features like distances and angles during transformations, crucial for accurate modeling in AI applications. However, the specific matrix formulation needed for this is something that deserves more research.
6. Using radians instead of degrees simplifies trigonometric function calculations, as many programming environments natively utilize radians. This could potentially lead to efficiency improvements in processing, although language-specific benchmarks would be needed for a fair comparison.
7. Representing angles through matrices allows us to transform multiple angles simultaneously. This has potential benefits in workflows where many objects are animated or simulated, potentially optimizing the overall process.
8. Within an enterprise AI context, recognizing the consequences of angle conversions can streamline data processing within algorithms involved in tasks like optimization and spatial recognition. However, it's important to understand that this might only be advantageous in specific contexts.
9. While degrees and radians can represent the same angle, their inherent mathematical properties influence numerical calculations differently. This difference can cause inconsistencies in signal processing if not carefully addressed. The implications for different algorithms or domains is yet another aspect to explore.
10. Integrating angle conversion into comprehensive AI frameworks can improve the clarity of complex geometric data, which might subsequently lead to better decision-making capabilities in domains such as navigation systems or augmented reality. The extent of this improvement, however, would depend on the specific application and how these conversions are integrated.
Understanding Radian Notation in AI-Driven Geometric Processing A Practical Implementation Guide - Implementing Angular Calculations for Machine Vision Systems With Basic Examples
Within machine vision systems, accurately implementing angular calculations is crucial for processing visual data, especially in tasks like feature recognition and understanding how objects change spatially. Radian notation is key here, ensuring accurate computations related to rotations and other transformations. Getting this right is vital for maintaining the integrity of the data being processed. The ability to process angles in a way that supports real-time operation is particularly important for systems operating in high-speed environments, like automated robotics and manufacturing. The combination of mathematical rigor for angular calculations and the analytical power offered by integrating machine learning within these systems empowers them with sophisticated capabilities. This combination can lead to more robust decision-making processes within a wide variety of industrial settings. While the field of machine vision is continually evolving, the intersection of complex geometrical information and data-driven approaches via machine learning promises further enhancements in AI-powered applications across a wide array of enterprise uses. It's important to remember that the effectiveness of these advancements will depend on the context of their application.
1. Using radians instead of degrees in machine vision calculations can potentially minimize numerical errors, especially when dealing with floating-point arithmetic. This is because radians are more intrinsically connected to the fundamental geometry of the unit circle. It's an interesting observation that needs further investigation.
2. The accuracy of radian measurements becomes particularly important when dealing with object recognition or detection within machine vision. Even small errors in radian values can lead to significant discrepancies in the results. This suggests the need for robust error handling and potential calibration procedures within the vision system.
3. Radian notation offers a simplified approach to calculating angular derivatives. This advantage is crucial for motion prediction algorithms that need to accurately track angular velocity and acceleration, especially in dynamic environments. We might expect to see a greater use of radians in these applications as the field advances.
4. The use of radians might improve the efficiency of pathfinding algorithms within AI-driven robotics. The smooth transitions between different angular states often needed in these applications could benefit from radians' inherent mathematical properties. However, a direct performance comparison between implementations using radians and degrees would be needed to validate this hypothesis.
5. The computational burden of converting between degrees and radians can be eliminated by designing algorithms that directly accept radian values as inputs. This could lead to significant performance gains when dealing with vast amounts of geometric data, but potentially requires a redesign of existing libraries and code.
6. In the context of angular transformations, using a rotation matrix defined in radians can help to achieve smoother interpolations. This is especially valuable for visual applications where smooth animations are important and preventing jarring transitions is essential. It would be interesting to see how different interpolation schemes compare across degrees and radians in this context.
7. In some situations, using radians can reveal patterns or anomalies in angular data that might be obscured when using degrees. This can be useful for debugging and refining machine vision models. It highlights the idea that choice of representation can have subtle effects on analysis.
8. The use of matrix operations for radian conversions can offer a way to unify sensor data from different sources in autonomous systems. This is particularly relevant in real-time applications where consistent and accurate interpretation of multi-sensor data is essential. However, this approach might not be universally applicable across different sensor types.
9. Switching to radians can potentially alter the complexity of some computational problems in computer vision. Certain search and optimization tasks might benefit from this shift. This is a powerful idea but requires careful consideration of the specific problems and algorithms.
10. When dealing with three-dimensional rotations, using radians leads to a simpler mathematical representation. This stems from the fact that the trigonometric identities involved in these calculations become more complex when using degrees. Consequently, we might find that applications like augmented reality that deal with 3D rotations are more likely to benefit from a radian-based approach. However, this might come at the expense of more specialized algorithms.
Understanding Radian Notation in AI-Driven Geometric Processing A Practical Implementation Guide - Building Rotation Functions in Python Using NumPy Arrays and Radian Math
In Python, crafting rotation functions using NumPy arrays and radian math involves constructing rotation matrices. These matrices enable the manipulation of geometric data, allowing for transformations like rotations. Libraries like NumPy offer functions like `numpy.rot90`, designed for 90-degree rotations in 2D arrays. Building these functions often involves implementing the corresponding mathematical formulas directly within NumPy, offering a fairly straightforward approach. Radian math plays a crucial role here, as it's the natural language of linear algebra, particularly when dealing with angles in transformations. The `numpy.radians` function seamlessly converts degrees to radians, which is important for maintaining consistency and accuracy. Importantly, NumPy's optimized algorithms allow for rotations on substantial datasets, making it suitable for computationally-intensive AI geometric processing applications. Using these techniques is critical for ensuring accurate and efficient geometric manipulations, from tasks like image processing in machine vision to robot control. While useful, it's important to remember that the choice of radians and specific implementations within NumPy come with their own limitations. Understanding these choices will improve overall computational efficiency and help maintain the integrity of geometric transformations within a wider range of AI-driven applications.
1. Radians offer a distinct advantage in terms of numerical stability, especially when dealing with trigonometric functions. This is because they are intrinsically tied to the geometry of the unit circle, which can minimize the accumulation of errors during computations. It's an interesting point to consider when developing complex numerical systems.
2. NumPy's broadcasting feature shines when building rotation functions with radians. We can easily apply angle conversions across entire arrays, which is particularly useful for optimizing performance when working with large datasets in batch operations. While efficient, it's worth noting the tradeoffs this approach might have with memory usage.
3. Shifting to radians can lead to simpler gradient calculations in optimization problems. This might result in faster convergence times for machine learning algorithms where adjusting angular parameters is a key aspect. However, the extent to which this improvement is realized would depend heavily on the specific problem and algorithm.
4. The inherent relationship between radians and circular geometry makes them well-suited for recursive algorithms related to rotations. This simplified mathematical foundation can make iterative calculations, particularly in matrix transformations, more efficient. But again, we should be mindful of the potential risks of numerical instability associated with recursive methods.
5. Utilizing radians in NumPy rotation functions often leads to more concise and readable code. While this might seem like a small point, it's important for maintaining code and reducing errors, especially in high-performance computing where complex logic can be difficult to follow. It's worth investigating if there's a systematic way to evaluate code readability for different approaches to rotation functions.
6. Interestingly, the use of radians for angle management is quite common in graphics rendering. Many rendering algorithms heavily rely on radian-based calculations for transformations like rotations and scaling, likely because radians help preserve accuracy during these complex visual manipulations. This might be a fruitful area for exploring how these techniques can be applied more broadly in other applications.
7. Research on robotic motion often suggests that using a radian-based approach results in smoother trajectories. This stems from the minimization of abrupt changes in angular velocity, a crucial aspect for tasks requiring fine control over robot movements. Further exploration is needed to see if these results can be generalized across different types of robots and tasks.
8. Radians make it easier to work with Euler angles in 3D transformations. This is because rotations can be expressed as a series of simple additions and multiplications of angles. As a result, implementing rotation functions in spatial models becomes more streamlined. But the implementation complexity of such operations still needs to be carefully analyzed.
9. Representing objects in radians can lead to faster pathfinding calculations. This advantage likely comes from the inherently coherent nature of radians in angular computations, making it more suitable for systems that dynamically adjust routes based on real-time data. It's important to compare this approach with other pathfinding techniques before concluding it's uniformly superior.
10. Finally, radians can improve visual debugging processes in machine vision systems. Angular miscalculations can result in visual artifacts that are less frequent when using radians. This can lead to improvements in the quality and reliability of systems involved in object tracking and recognition in real-time. Though, this advantage is likely dependent on the specific application and the type of machine vision system employed.
Understanding Radian Notation in AI-Driven Geometric Processing A Practical Implementation Guide - Error Handling and Edge Cases in Geometric Processing Applications
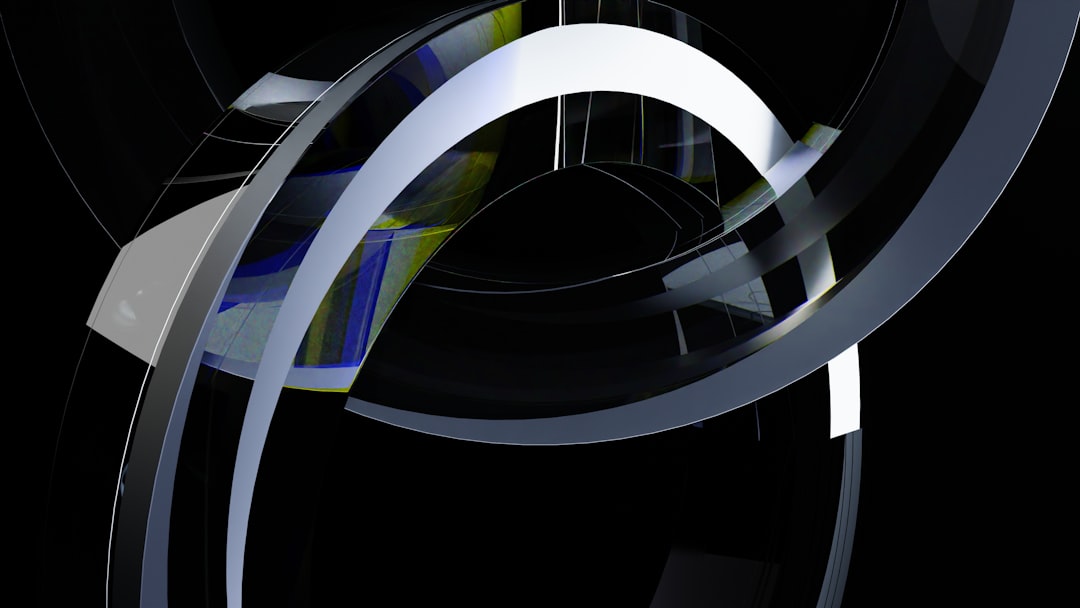
In AI-driven geometric processing, handling errors and addressing edge cases is crucial for ensuring the reliability and accuracy of applications. These applications frequently involve complex calculations that can introduce inaccuracies, making robust error detection and correction strategies essential. Developers need to anticipate edge cases—those unusual or extreme situations that might lead to unexpected outcomes or system failures. Designing algorithms that can handle these situations gracefully is paramount. The difficulty lies not only in creating the error handling mechanisms but also in the rigorous testing required across a diverse range of geometric scenarios to ensure these mechanisms consistently work as expected. Given the rising demand for geometric processing across various sectors, the necessity for improving accuracy and resilience through effective error handling is becoming more significant. While often overlooked, these seemingly minor aspects can have a large impact on a system's overall stability and performance.
Geometric processing, while powerful, often encounters challenges related to inaccuracies and unexpected behaviors, particularly in real-time systems like those found in robotics or computer graphics. Floating-point arithmetic, a common tool in these applications, introduces inherent limitations in precision, which can cause issues with tasks like intersection tests or transformations, leading to algorithm failures.
Situations involving unusual configurations, such as lines that are perfectly aligned or objects overlapping in ways not anticipated, can wreak havoc on algorithms designed for geometric intersections. Understanding these "edge cases" is crucial, especially when applying AI techniques for object recognition or analysis where unforeseen inputs are likely. It's important to design algorithms with a robust awareness of these edge cases.
In some instances, random modifications to algorithm parameters can be employed to help avoid problems associated with problematic or "ill-conditioned" configurations. However, this can lead to unreliable or unpredictable results if not carefully tuned.
Many systems use a hierarchy of shapes to model more complex geometries. But this hierarchy can become a source of errors when the system incorrectly assumes relationships between these components, especially when they intersect or are near boundaries. These errors translate directly into problems with the system's functionality.
Furthermore, many algorithms assume that the shapes they process are simple, like a convex polygon. However, many real-world scenarios involve complex shapes that break these assumptions. This leads to the need for error handling methods that are flexible enough to accommodate the diverse range of geometric scenarios.
Spatial indexing structures like R-trees or KD-trees are frequently employed to quickly search and retrieve geometric data. However, maintaining the efficiency and balance of these data structures can become complex when unusual geometries appear, impacting the speed and accuracy of queries and data updates.
Errors can also cascade through a series of geometric transformations. Tiny inaccuracies in one step can amplify in subsequent operations, resulting in significantly inaccurate final outputs. This necessitates algorithms that have a notion of an acceptable margin of error and incorporate corrective strategies such as "snapping," which forces points close together to coincide exactly.
In situations where quick responses are crucial, like collision detection in robotics, prioritizing speed often requires accepting some reduction in accuracy. This delicate balancing act demands that geometric algorithms carefully consider the tradeoffs between efficiency and accuracy.
Interestingly, many algorithms use iterative approaches to solve geometric problems. These methods can converge on different solutions based on the starting point of the iterations, especially when the geometry is complex. This can lead to varied or unpredictable outputs, making it challenging to ensure the system behaves in the expected way.
Finally, it's worth observing that examining the errors in a geometric processing application often reveals unexpected connections between the properties of the shapes being manipulated and the reliability of the algorithms themselves. A deeper understanding of the fundamental mathematics underlying these operations seems to be a key factor in designing robust and reliable algorithms.
Understanding Radian Notation in AI-Driven Geometric Processing A Practical Implementation Guide - Performance Optimization Techniques for Large Scale Angular Computations
When dealing with large-scale Angular computations, optimizing performance becomes crucial. Angular's design, with its data binding and change detection mechanisms, can sometimes create performance hurdles, particularly when dealing with substantial amounts of data or complex interactions. Understanding the architecture and the factors impacting performance is the first step.
Strategies like pre-rendering during the build process or using techniques like hydration, which improves performance after initial rendering, can have a big impact on how quickly the application loads and responds to user input. These methods can significantly improve the overall user experience. Furthermore, Angular offers several tools that directly address performance bottlenecks related to data changes. By implementing techniques such as the OnPush change detection strategy or using immutable data structures, one can significantly reduce the impact of complex data manipulations on performance.
However, effectively tackling these issues requires a methodical approach. Identifying where performance lags is a key step. Then, tailoring specific optimizations for the application in question allows developers to get the best results. By applying a combination of strategies that address the particular bottlenecks, applications can maintain a high level of responsiveness even when dealing with considerable computational loads. While some of these strategies may seem abstract, their impact on real-world performance can be noticeable and valuable.
Performance optimization in large-scale Angular applications is a fascinating area of study, particularly when dealing with complex data and intricate component interactions. Techniques like using the OnPush change detection strategy can drastically reduce re-rendering times by ensuring components only update when their inputs actually change, instead of on every change detection cycle. This seems like a simple concept but can have a big impact.
Another interesting approach involves `trackBy` functions in `ngFor` loops. These functions can dramatically speed up list rendering by telling Angular which items have changed, rather than forcing it to re-render everything on any modification. It's quite clever.
Lazy loading is another technique that can improve performance. It lets us break up our application into smaller pieces and only load what's necessary when needed. This results in much faster initial load times, a major win for user experience.
For computationally intensive tasks, web workers can be a real boon. They allow us to shift heavy operations off of the main thread, preventing the UI from becoming sluggish during those operations. It's a powerful tool for ensuring a smooth user experience, though understanding how to correctly use web workers requires some thought.
Ahead-of-Time (AOT) compilation also plays a role in optimizing Angular applications. This technique pre-compiles the application, reducing bundle size and decreasing the work the browser needs to do at runtime, leading to faster loading. However, understanding how AOT interacts with your chosen build tool and development environment is vital.
Observables and the RxJS library offer a compelling approach to managing asynchronous data streams. They offer the potential for significantly improving performance, particularly in situations involving APIs or real-time data. The ability to manage these streams effectively without overworking the UI thread is quite useful.
Pure pipes can also be used to optimize performance. They can avoid redundant calculations when the input data doesn't change, further improving the efficiency of rendering dynamic content. It seems like a simple optimization but can have a measurable impact.
The concept of change detection strategy is actually very important. While the default strategy is usually fine, moving to OnPush in certain circumstances (like in complex hierarchies) can provide a significant performance gain. This change can have far-reaching consequences so we need to carefully understand the implications.
Luckily, Angular includes profiling tools like Augury. These tools are indispensable for analyzing performance bottlenecks in our applications, enabling us to make better choices based on how the application is actually used rather than assumptions.
Finally, optimization techniques like tree-shaking can help reduce bundle size further. It effectively removes any code that's not being used in the application. This reduces load times and improves runtime efficiency, especially important for larger applications. However, tree-shaking is not a magic bullet and comes with its own complexities.
In conclusion, understanding these different techniques and applying them strategically can drastically improve performance in large-scale Angular applications. The challenge is understanding when and how to use each technique, requiring a deep understanding of the specific aspects of our applications that are driving poor performance. The field of Angular optimization is still evolving, and understanding these optimization techniques is crucial for anyone building and maintaining complex Angular systems.
Understanding Radian Notation in AI-Driven Geometric Processing A Practical Implementation Guide - Testing and Validation Methods for Radian Based AI Operations
When evaluating AI systems that utilize radians for geometric processing, we need to move beyond traditional testing methods. Radian-based AI operations are complex, demanding new, data-focused validation approaches. Scenario-based testing becomes crucial for ensuring the system functions as intended, especially when dealing with rotations and dynamic changes. It's important to be clear about the differences between validation—checking if the AI system fulfills the user's needs—and verification—making sure the system complies with its design specifications.
We're seeing newer, more advanced methods like metamorphic testing emerge as a way to address some of the more intricate challenges of validating AI. This highlights the ongoing need to improve validation practices, especially regarding potential biases in AI outputs that can lead to unfair or inaccurate results. Because AI systems are increasingly relied upon for real-time operations and complex tasks involving spatial relationships, an iterative approach to testing becomes vital. This ensures that radian-based calculations within the AI system stay reliable across a broad range of applications.
In short, thorough testing and validation are critical for the development and deployment of AI systems that leverage radians. By conducting rigorous testing, we can gain important insights that guide the development of AI that generates consistently accurate and unbiased results in various real-world settings.
1. Using radians often simplifies the mathematical formulas involved in AI operations, which can lead to faster processing, especially in applications that require quick responses or continuous updates. This is particularly relevant for systems that rely on high-frequency calculations.
2. The way trigonometric functions behave is different when using radians compared to degrees. This difference can impact how oscillations are handled in AI systems. For example, the way rounding errors accumulate could potentially be more predictable when using radians, leading to more reliable results in simulations involving angles.
3. When dealing with machine learning models that use differential equations to make predictions, radians offer a more direct and natural way to express these equations. This can simplify the coding process and allow researchers to better understand how these dynamic systems behave.
4. When performing transformations on geometric objects, especially with matrix algebra, radians can lead to more consistent and predictable results. They naturally maintain the important geometric relationships that are crucial for accurate angle-related calculations.
5. When dealing with large amounts of data, floating-point arithmetic can sometimes lead to errors where numbers become too big or too small to represent. Radians can help minimize these errors, making AI applications more reliable and less prone to unexpected behavior.
6. Some theoretical studies suggest that using radians provides a more precise way to describe the movement of rotating objects. This is vital in applications like navigation and robotics, where very accurate tracking of motion is required. More research in this area could be beneficial.
7. Using radians can potentially improve the speed of many iterative methods used to solve geometric problems. These methods are commonly used in optimization problems, so any improvements can reduce the amount of computation needed.
8. When updating the angular position of objects in a sequence of transformations, the use of radians can limit the buildup of errors that can occur in each step. This is crucial for maintaining the fidelity of complex models used in many different AI systems.
9. Radians are directly related to the properties of a unit circle. Understanding this relationship can provide valuable insights into how to interpret geometric data, especially when working with high-dimensional spaces, which are becoming increasingly common in AI model training.
10. Finally, using radians in AI applications can make it easier to understand how angles are related in complex datasets. This is important because it can help avoid the misinterpretations that can arise when using degrees in various AI applications. While more research is needed, this could be especially useful in complex systems.
More Posts from aitutorialmaker.com: