7 AI Research Paper Summarization Tools That Process Complex Academic Texts in Under 60 Seconds
7 AI Research Paper Summarization Tools That Process Complex Academic Texts in Under 60 Seconds - MyMapAI Analysis Engine Processes PDF Research Papers in 45 Seconds
MyMapAI's analysis engine distinguishes itself by processing PDF research papers remarkably fast, within 45 seconds. This capability proves particularly helpful for students, educators, and researchers seeking quick summaries of complex academic works. However, while delivering speed, the trade-off might be a loss of nuanced understanding in the pursuit of brevity. The development of tools like MyMapAI underscores the increasing need for efficient methods to handle the vast volume of academic literature. The evolution of AI-powered summarization tools holds the potential to transform how scholars navigate and comprehend extensive research materials, though the limitations of such approaches should remain in mind.
MyMapAI's engine leverages natural language processing to break down complex research papers found in PDF format into digestible insights, potentially changing how researchers manage their literature review process. It's notable that the processing time is remarkably quick, at just 45 seconds. This speed offers a potential advantage, matching human reading speeds while mitigating the exhaustion and understanding challenges associated with lengthy research texts.
The core of this engine uses machine learning, a process that allows the engine to constantly adapt and enhance its summarization abilities. It accomplishes this by learning from user interactions and feedback, as well as gaining a deeper understanding of the subtleties within different areas of academia. It is also noteworthy that the engine can analyze citation networks within PDFs, which leads to the intriguing prospect of predicting upcoming trends in research, giving researchers a look into what might be coming next.
MyMapAI's ability to process a variety of subjects creates a potential benefit by bridging knowledge gaps across distinct disciplines. This is important for facilitating a more interdisciplinary approach to research and making it easier for scientists with diverse backgrounds to collaborate. It's important to realize that the engine isn't only for textual information. It handles charts and graphs, which is essential for a complete comprehension of experimental studies.
By identifying and highlighting core concepts and research methodologies, MyMapAI could reduce the need to sift through mountains of text to find the most pertinent materials. Furthermore, it allows for a comparative analysis of the findings across different papers, allowing researchers to see where viewpoints and study results diverge or converge. Unlike other summarization tools, it offers contextual clarifications that add to the meaning of uncommon academic language and ideas. This can be useful for navigating intricate research discussions. The potential integration with other research management software could streamline the overall research process. This could make it easier for researchers to shift from summarization to citation management and other parts of their projects.
7 AI Research Paper Summarization Tools That Process Complex Academic Texts in Under 60 Seconds - Scholarcy Research Assistant Breaks Down Complex Scientific Language
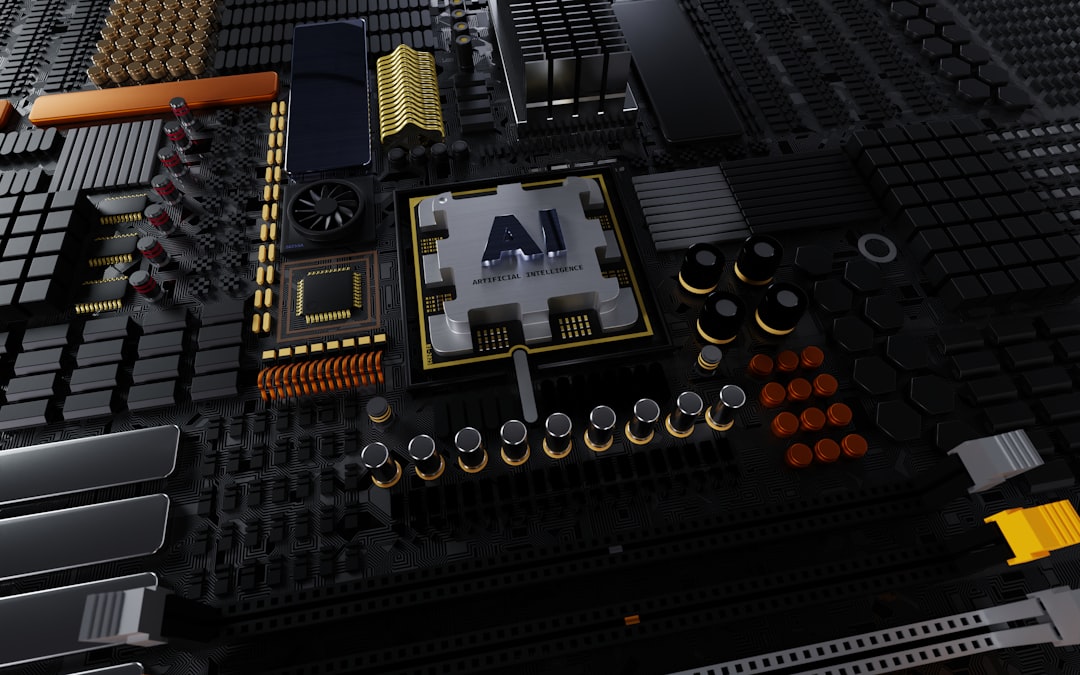
Scholarcy, powered by artificial intelligence, tackles the difficulty of understanding complex scientific language by making research more efficient. It creates organized summaries, highlighting key concepts, findings, and arguments from research papers, articles, and book chapters using a flashcard format. This AI-driven tool quickly breaks down complex language, saving researchers potentially up to 70% of the time usually spent sifting through materials. This speed boost is particularly useful when conducting literature reviews or identifying core information. Furthermore, Scholarcy goes beyond just summarizing, offering convenient features like generating bibliographies and exporting the summaries into standard citation management software. These features are designed to improve the overall workflow of researchers. Scholarcy, like many emerging AI tools, demonstrates how these technologies can help researchers tackle the often dense and convoluted nature of academic texts.
Scholarcy is an AI tool designed to help researchers sift through complex academic papers quickly. It processes PDFs, articles, and book chapters, automatically creating summaries and organized information. It achieves this by recognizing key terms, claims, and findings within a paper, delivering these in a well-structured format like flashcards. This makes it possible to get the gist of a paper's core arguments and results in just seconds, potentially saving researchers a significant amount of time – up to 70% according to some estimates. This is particularly useful during literature reviews or when screening a large number of articles.
One of Scholarcy's neat tricks is that it can generate bibliographies and export these flashcards to citation management tools like Zotero or Mendeley with a single click. This is useful for quickly building up a collection of relevant papers. However, I wonder about the level of accuracy in this process, especially with specialized journals or fields that have specific citation styles.
Scholarcy has a browser extension, so if you're reading a paper online, you can easily get the key takeaways. This provides a level of convenience not always available with other similar tools. Beyond just summaries, Scholarcy generates different types of outlines, offering more control over how you want to review the information. While this may help with research productivity, I'm curious about how useful these various formats are in the long run. Do they really help organize the information more effectively than a simple summary?
The idea behind most of these AI research paper summarization tools, including Scholarcy, is to translate complicated academic language into something easier to grasp. This is especially helpful for researchers and students new to a field or who are tackling a topic with very dense writing. While this approach is intuitive, one needs to be mindful of the potential loss of nuance when condensing complex arguments into bite-sized chunks. It's important to ensure that these summarization tools don't end up oversimplifying or misrepresenting crucial parts of the original work.
Scholarcy, like many others, seems to constantly learn and improve based on user feedback and trends in academic publishing. This is a plus as the field of research is constantly changing, but it does make me wonder how quickly these algorithms can adapt to emerging areas of study or the subtle changes in language that occur over time. Also, will these tools be able to capture the evolving nature of academic discourse and its often highly specialized terminology? I suppose only time will tell.
7 AI Research Paper Summarization Tools That Process Complex Academic Texts in Under 60 Seconds - Genei Platform Tags Key Research Findings Through Machine Learning
Genei employs machine learning to assist researchers in making sense of academic literature. It streamlines research by summarizing dense articles, identifying key findings, and extracting crucial information quickly. Genei's capabilities, powered by sophisticated machine learning models, include semantic search and the ability to automatically answer questions related to the research being analyzed. These tools have reportedly saved researchers considerable time, with a majority finding Genei helpful in quickly understanding key concepts. While efficiency gains are certainly beneficial, researchers should always be mindful of the potential for simplification to obscure subtle, field-specific details during the summarization process. The platform's constant evolution, fueled by its machine learning algorithms, suggests a potential future where research is further streamlined, but one must question how well the AI can handle the complexities of specific disciplines and keep up with the constantly changing landscape of academic language.
Genei is an AI tool designed to enhance research by using a system of tags that are created through machine learning. Essentially, Genei learns what's important to a researcher based on their interactions and usage, then categorizes research findings based on these learned preferences. This dynamic categorization system allows for more relevant insights over time as the system learns.
Genei's ability to go beyond just recognizing keywords and actually understanding the context in which they are used is an interesting feature. It leverages natural language processing (NLP) to ensure that summaries generated from complex texts are more accurate, although I'm curious how it handles highly specialized vocabulary or different writing styles.
One unexpected aspect of Genei is its capacity to connect with external data sources. This can lead to a richer understanding of the research topic by incorporating additional context. This could be particularly useful for interdisciplinary research, where concepts may span multiple fields, but it raises questions about the trustworthiness and biases of external datasets.
Genei stands out by incorporating citation analysis, which helps to map the relationships between different research papers. This feature could greatly aid in identifying key research and influential papers within a field, potentially leading to a quicker and more efficient understanding of existing knowledge on a topic.
It's worth noting that Genei optimizes its summaries by assessing keyword frequencies and how often they appear together (co-occurrence). This helps to sift out less crucial information and focus on the most important insights within a given paper, but it makes me wonder if certain nuances or unique perspectives are potentially lost in the process.
Researchers can directly interact with Genei's summaries. The interface allows for real-time edits and annotations, personalizing the summaries to fit individual researcher needs. This capability may be beneficial for those who want to refine the output and create a customized summary.
One limitation I've considered with Genei is its ability to deal with interdisciplinary research. Terms and research methods can vary greatly between fields, making it a challenge for AI tools to summarize accurately. It's good to know that Genei is actively improving algorithms to minimize misinterpretations across diverse research areas, though this remains a concern in my opinion.
A wide range of document types are supported by Genei, including less common ones such as Markdown and LaTeX. This flexibility is advantageous since researchers frequently encounter a variety of formats during their work. I'd like to know more about the specific implementation and handling of these various formats within the tool.
The time it takes Genei to create a summary varies with the complexity of the source material, a point that also holds true for other summarization tools. This raises questions about whether a compromise between speed and the depth of understanding is a necessary trade-off, and if the focus on speed impacts researcher's confidence in the results.
By revealing emerging trends in specific research domains, Genei confronts the challenge of academic information overload. Researchers can access vital information and remain updated on their fields without being buried by the sheer volume of published research. It's a positive approach but how effectively it captures the nuances of research fields and whether it is biased toward some areas is a matter that needs further evaluation.
7 AI Research Paper Summarization Tools That Process Complex Academic Texts in Under 60 Seconds - Paper Digest Creates Citation Networks From Multiple Academic Sources
Paper Digest is an AI platform designed to assist researchers with various aspects of their work, including writing, reviewing, and staying current with the latest research. It has been used by millions of individuals since its launch in 2018, primarily in fields involving technological trends and data analysis. A notable feature of Paper Digest is its ability to create citation networks from a large collection of academic papers. This feature effectively weaves together the interconnectedness of research, potentially providing a better understanding of a field's evolution and key contributions. By aggregating and linking sources, it helps researchers understand how research builds upon itself, which is useful during literature reviews. However, relying on AI-generated citation networks introduces the need for caution. Questions about the accuracy of these automated analyses, especially in complex research areas with intricate arguments and nuances, remain. The continuous evolution of research and the increasing diversity of academic fields present challenges for these AI-powered tools. As we see a growing need for researchers to streamline their workflows, relying on AI tools to manage citations and build networks necessitates a careful consideration of potential limitations and biases that can arise.
Paper Digest, an AI-powered research platform, aims to assist researchers in navigating the vast ocean of academic literature. It does this by building what it calls "citation networks" which are essentially maps showing how different research papers relate to each other through citations. This can be a useful way to see the progression of ideas within a field over time and potentially spot hidden connections between different research areas. While interesting, I find myself questioning the overall reliability of this approach. Paper Digest gathers information from a wide variety of academic sources, but not all sources are created equal. This means the quality of insights gleaned from the network can be impacted if less rigorous or reputable journals are included.
Beyond simply showing relationships, Paper Digest tries to use these citation patterns to predict future trends in research. It's a fascinating notion, to be able to anticipate where a field might be heading. However, I'm always cautious about predictive capabilities, especially in complex areas like academic research.
The tool's ability to connect diverse research areas is quite useful as research becomes more interdisciplinary. It could, in theory, foster collaborations and help researchers see the connections between seemingly disparate fields. However, the question remains: can it effectively navigate the nuances of specialized vocabulary and concepts that differ significantly across fields? I have my doubts about this.
One interesting feature is that Paper Digest automatically updates its citation networks as new research emerges, which can be very helpful in keeping researchers current. However, it can also be overwhelming, as it creates the need to constantly stay informed on the latest research—a constant race to keep up.
Initially, the visualization of these networks can be quite daunting if you're not already familiar with the research being cited. I believe that some kind of learning curve might be required to really understand how to leverage the full potential of the visualization.
Another potential issue I see is the danger of misinterpretations due to the lack of context for citations. Simply because a paper cites another doesn't necessarily imply agreement or endorsement. The AI may miss the specific reasons why a paper is cited within a given argument, which can lead to inaccuracies.
I'm also concerned about the potential for biases in the way Paper Digest builds these networks. The databases it uses and the selection criteria it employs could inherently favor certain types of research or viewpoints, leading to a potentially skewed perception of the evolution of a particular field.
Though it's designed to aid researchers, the integration with other citation management tools can be a bit spotty in my experience. This can complicate the process of moving data between different tools, which can be frustrating.
Paper Digest learns from how researchers use the system, improving its analysis over time. However, this learning process is dependent on user feedback. Feedback from novice researchers may not be as insightful as that of experts, potentially leading to less targeted improvements.
In summary, Paper Digest holds potential as a tool for navigating academic literature. However, it's essential to consider the limitations that may arise from data quality, potential biases, the need for careful interpretation, and usability concerns. As with any AI tool, there's a need for caution and critical evaluation.
7 AI Research Paper Summarization Tools That Process Complex Academic Texts in Under 60 Seconds - QuillBot Academic Parser Identifies Core Arguments in Technical Papers
QuillBot's Academic Parser is built to pinpoint and isolate the central arguments within technical papers, making it easier to grasp the core ideas of complex research. By using AI, this tool tries to overcome the difficulty researchers face when trying to understand dense academic text. While the ability to quickly summarize research can improve efficiency, there are worries that this speed might result in a loss of subtle, important details. Given the ever-growing amount of academic material, tools like QuillBot become more important for researchers trying to make sense of the information, although the need to balance quick processing with a deep understanding remains a significant issue.
QuillBot's Academic Parser is designed to pinpoint the core arguments within technical papers. It does this by examining both how the paper is written and the actual language used, which helps in understanding complex scientific discussions without getting lost in the details. This focus on the central arguments is important because scientific papers are often packed with dense information, and quickly identifying the main points is a big help.
While other tools might lose some of the fine points during summarization, QuillBot seems to make a concerted effort to preserve the overall context of the arguments. This means that the unique perspectives and ideas of the authors are hopefully less likely to be lost or misrepresented. This focus on context is really useful for understanding the 'why' behind the research, not just the 'what'.
The developers seem to have designed the tool with the user in mind. This means researchers can interact with the summarized content and tailor it to fit their needs or specific research questions. This sort of interactivity gives the user a more active role in the process, leading to potentially more useful outputs.
Unlike some tools that only handle text, QuillBot can also examine figures, equations, and the references in a paper. This multi-faceted approach seems like a good way to get a broader understanding of the whole paper rather than just a few sections.
QuillBot's AI learns over time as researchers use it. The tool is constantly adapting and improving through user feedback and by staying up-to-date with the vocabulary and research trends within different academic fields. This learning process could be valuable for keeping the summarization relevant and accurate across many areas of study.
Beyond just summarizing, the tool also maps out the structural elements of academic papers. This can include things like hypotheses, research methods, and conclusions. It's likely that this structural approach helps researchers get to the information they need quicker by providing a clearer way to navigate the output of the summary.
QuillBot also plays nice with common citation software, so it is possible to move the main points identified by the summarizer into a broader research workflow without a lot of extra steps. This is quite helpful for those that have a very systematic way of managing their research.
Another interesting facet of QuillBot is that it potentially helps move research forward by making it easier to distill the main arguments in a paper. This feature could support scholarly debates and make it easier for researchers to plan presentations or work on collaborative projects by having a clear way to communicate differences in opinions or approaches.
Naturally, with AI there's always the question of potential bias and misinterpretations. In QuillBot's case, users need to be aware that the AI might oversimplify complex arguments or overlook some important details in certain fields. It's vital for researchers to critically evaluate summaries from AI and not simply accept them as fully accurate.
Finally, QuillBot's interface seems pretty simple and easy to use. It doesn't appear to have a steep learning curve, so researchers from different backgrounds are likely to be able to benefit from the tool without too much extra effort. This accessibility is an important feature that will likely increase adoption and use among researchers.
7 AI Research Paper Summarization Tools That Process Complex Academic Texts in Under 60 Seconds - Semantic Scholar Research Engine Maps Connected Studies
Semantic Scholar's research engine employs AI to delve deeper into the meaning of research papers, going beyond just keywords. This allows it to create visual maps that illustrate the connections between different studies. By understanding the semantic relationships between papers, researchers can explore related research and potentially gain a better grasp of trends within their field. While this ability to map connected studies can be incredibly helpful, there's a need to be cautious. There's always a possibility that the AI might oversimplify or misinterpret the complex relationships between studies. The ever-increasing volume of academic publications makes tools like Semantic Scholar increasingly relevant for researchers trying to navigate this vast information landscape. However, the use of such AI-driven tools necessitates careful scrutiny to avoid overlooking subtle details or accepting AI-generated insights without question. If these mapping capabilities are further integrated with AI-powered summarization, it could potentially create a more comprehensive view of the current state of knowledge within a particular research area, enabling scholars to make more informed decisions in their work.
Semantic Scholar, powered by AI, goes beyond just summarizing research papers; it creates visual maps of how studies are connected through citations. This gives researchers a bird's-eye view of a field, showing them which papers are highly influential and where potential gaps in knowledge might exist. It uses something called "semantic search," which means it tries to understand the meaning of a paper rather than just looking for specific keywords. This is especially important in fields where the same idea might be expressed in different ways.
Semantic Scholar dives deep into the abstracts and references of papers to help researchers get a better grasp of the big picture within a field. It can help identify trends and changes happening in a specific area of study, potentially inspiring researchers to explore new and emerging research avenues. However, as it continuously updates its database with new papers, there's a risk of information overload as researchers constantly grapple with new connections and insights. It integrates with OpenAI's models, attempting to generate deeper insights and explanations of complex concepts found within papers. This could be a powerful way to expand research, but it also raises questions about how accurate the generated interpretations might be.
It's interesting that Semantic Scholar tries to identify influential papers based on citations, but it's worth being cautious about solely relying on these automated metrics. Context and nuanced understanding are crucial in academic discussions, and just because a paper is cited a lot doesn't necessarily make it the best or most important. The way Semantic Scholar's algorithms work is constantly being improved through machine learning, but this process raises some concerns about whether inherent biases in the training data might influence the tool's overall reliability.
By providing context and impact metrics alongside citations, Semantic Scholar tries to guide researchers towards the most impactful studies. But whether this approach is truly effective can vary significantly depending on the field of research. The platform attempts to stay current by adjusting its algorithms to recognize changes in writing styles and terminology used in academic papers. This is helpful, but it also makes me wonder if the speed of these changes will always stay ahead of the tool's interpretation capabilities.
While Semantic Scholar is designed to simplify literature reviews, its mapping capabilities might unintentionally cause researchers to favor certain papers based on how relevant they seem rather than a thorough exploration of a broader range of literature. It’s a good reminder that, while AI tools like this can be beneficial, it's critical to evaluate their outputs carefully and not take them at face value.
7 AI Research Paper Summarization Tools That Process Complex Academic Texts in Under 60 Seconds - Iris AI Knowledge Graph Generator Links Research Concepts
Iris AI's Knowledge Graph Generator helps researchers connect ideas within scientific literature by creating visual maps of concepts. This feature allows researchers to quickly understand how different aspects of a research area relate to one another, making complex relationships more accessible. The tool compares original research papers to AI-generated summaries to find inconsistencies, aiding in ensuring accuracy. It's designed to be intuitive, needing no special technical knowledge to create detailed graphs, allowing a wide range of researchers to use it effectively. Iris AI also tries to avoid bias in research by using methods other than standard citation systems, which can sometimes skew results. The ability to create visual displays of the interconnectedness of ideas can be helpful for getting a quick sense of how a field is structured, and it's a useful addition to the growing set of AI-driven tools for research. Nonetheless, it's essential to keep in mind the risk that such simplification might lead to the loss of important or subtle points often seen in complex academic discourse.
Iris AI is developing a tool that aims to bridge the gap between individual research and the broader academic landscape. It does this by constructing a knowledge graph—essentially a visual map—that links research concepts together. This dynamic graph is built by comparing the original text of research papers with summaries generated by AI, looking for potential discrepancies. One interesting aspect is that the platform doesn't rely on a standard citation system; instead, it tries to build connections based on a deeper understanding of the actual concepts discussed in the papers. This approach has the potential to reveal interdisciplinary links that might be missed through traditional citation analysis.
Users can interact with Iris AI's Researcher Workspace by uploading and organizing their papers into folders, allowing them to interact with their documents using AI. This helps researchers to quickly get a feel for the key concepts discussed in a given paper, as well as how these concepts connect to other relevant studies. A core element of Iris AI is this knowledge graph generator, which produces visualizations of the relationships between different research areas, highlighting potentially interesting connections in a matter of seconds. This rapid creation of knowledge graphs offers a significant time-saving advantage over manually building such relationships. Moreover, it’s built with a focus on user-friendliness, so researchers without a strong technical background can use it effectively.
However, as with other AI tools, there are potential downsides to consider. For instance, Iris AI's reliance on domain adaptation techniques to improve its accuracy in specific research areas is crucial, but it also highlights the challenges involved in capturing the subtleties of diverse academic fields. Furthermore, the visual representations of these complex relationships can sometimes be overwhelming, particularly for researchers who are new to knowledge graphs. It's also worth remembering that the accuracy of the generated knowledge graph is tied to the quality of the input data. If the underlying dataset is biased or incomplete, then the connections and inferences made by the AI could potentially be flawed. Finally, the increasing reliance on AI to summarize and link research raises interesting questions about authorship and intellectual property, issues that will need careful consideration within the academic community.
Iris AI offers an intriguing approach to navigating the vast landscape of academic research. The ability to quickly generate interconnected maps of research areas and the capacity for researchers to interact with their documents through AI has potential to accelerate research. However, critical thinking and a careful evaluation of the output of these AI-powered tools are always important, particularly as the technology continues to evolve and take on new roles in the academic world.
More Posts from aitutorialmaker.com: