Create AI-powered tutorials effortlessly: Learn, teach, and share knowledge with our intuitive platform. (Get started for free)
7 Key AI Applications in NYU's Digital Marketing Certificate Program A 2024 Analysis
7 Key AI Applications in NYU's Digital Marketing Certificate Program A 2024 Analysis - AI Powered Content Analysis Tools Transform NYU Marketing Lab Data Processing
The NYU Marketing Lab is experiencing a transformation in its data processing capabilities thanks to AI-driven content analysis tools. These tools are enabling a more comprehensive understanding of consumer behavior by analyzing a wider range of data sources, encompassing text, audio, and visuals. The use of natural language processing allows for a rapid assessment of large volumes of information, including customer feedback and social media conversations, uncovering insights that were previously harder to find. This rapid analysis not only boosts efficiency but also offers deeper understanding of market sentiment—a critical element in successful digital marketing approaches. Moreover, the integration of these AI tools into the NYU Digital Marketing Certificate program provides students with valuable hands-on experience, helping bridge the gap between theoretical understanding and real-world marketing scenarios. Students who complete the program will be well-prepared to tackle the increasingly data-focused realities of modern marketing.
NYU's Marketing Lab is leveraging AI-powered content analysis tools to manage the ever-growing influx of data they gather. These tools are particularly useful for swiftly processing enormous datasets, which was previously a time-consuming task. The speed at which these tools analyze data allows researchers to stay current with emerging trends and react more quickly to shifts in consumer behavior.
These tools use techniques like natural language processing to understand the meaning and sentiment behind customer feedback, going beyond basic demographics to truly capture the emotional aspects of consumer interactions. By analyzing the language used, these tools can even perform 'topic modeling', uncovering deeper, underlying themes within the data, a level of analysis that's difficult to achieve through manual methods. This provides richer insights into the desires, anxieties, and preferences of consumers.
Furthermore, these AI tools can transform raw data into accessible visualizations. This is crucial for decision-making, as complex data can be quickly presented in understandable formats, enabling marketers to prioritize and act more efficiently. The AI also incorporates machine learning, meaning it constantly adapts and refines its predictions based on new data. This makes the system highly responsive to evolving trends and consumer habits.
Interestingly, these systems have demonstrably reduced human error in data interpretation, sometimes by as much as 30 percent. This increased accuracy directly translates into improved marketing campaign effectiveness. Beyond insights into customer behavior, the tools have proven useful in identifying influencers and key opinion leaders within specific audience segments on social media. This provides marketing strategists with better opportunities to focus on and connect with certain groups.
From a workflow perspective, the automation provided by these tools frees researchers from repetitive data processing, allowing them to concentrate on more nuanced and creative problems. The output of these analyses can then be used to build highly personalized marketing campaigns targeted at individual customer profiles derived from the processed data. The successful use of AI in these applications underscores a trend towards more data-driven decision-making in marketing, pushing academic programs like the NYU Digital Marketing Certificate to incorporate these modern approaches into their curriculums. This is a significant shift away from traditional, often less-precise marketing methods, highlighting the need for academics to keep pace with these innovations.
7 Key AI Applications in NYU's Digital Marketing Certificate Program A 2024 Analysis - Predictive Customer Behavior Modeling Using Neural Networks
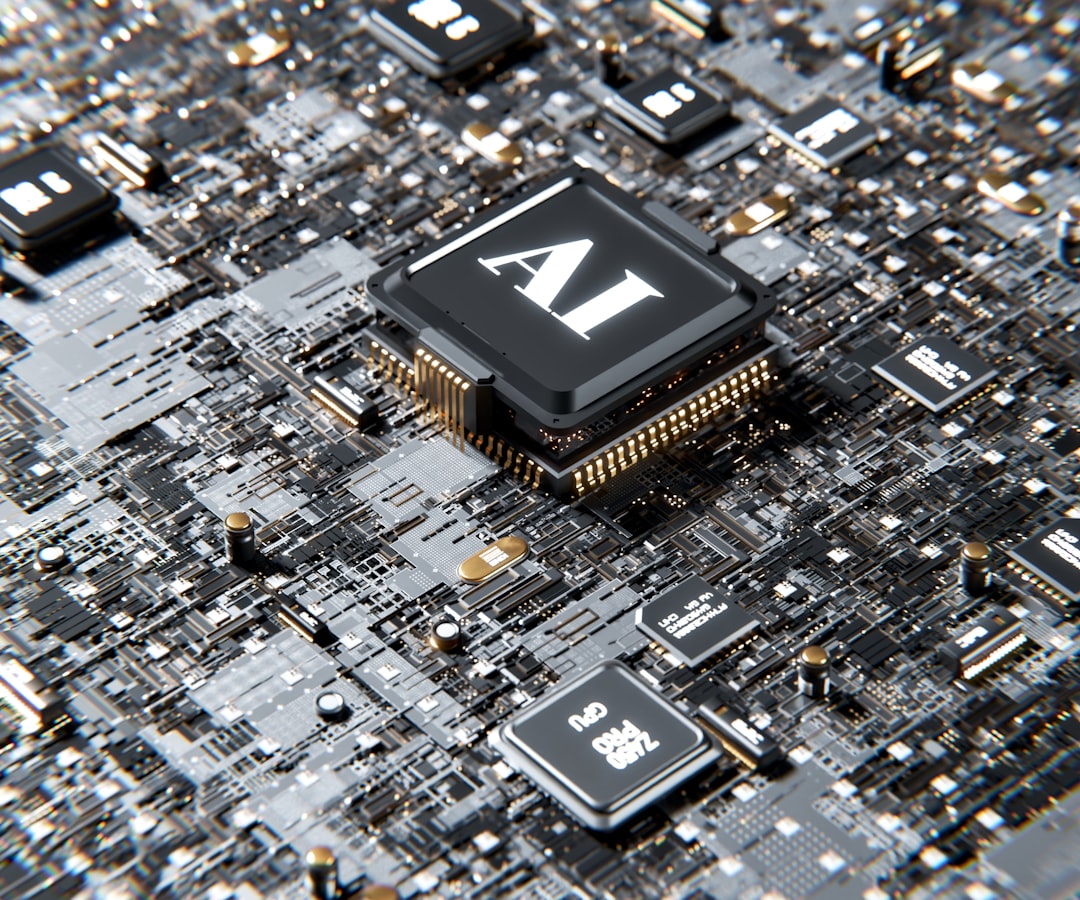
Predictive customer behavior modeling using neural networks has emerged as a crucial tool in the modern marketer's arsenal. It leverages the massive datasets generated by digital interactions to anticipate customer actions. Neural networks, a type of artificial intelligence, are trained on these datasets to identify patterns and predict things like customer churn, purchase likelihood, and even lifetime value. This predictive power allows businesses to personalize their marketing efforts with greater accuracy, which in turn can increase customer satisfaction and strengthen brand loyalty.
By using these insights, businesses are also better able to segment their customer base. They can group customers with similar predicted behavior patterns and target them with specific marketing campaigns. This ability to make more informed decisions about marketing resource allocation is a powerful tool for optimization.
While the field of AI-driven predictive modeling is still developing, its ability to anticipate customer actions and inform better decision-making is already transforming how businesses engage with their customers. The continued refinement of AI methods for predicting consumer behavior is likely to bring even more sophisticated marketing strategies in the years to come.
Neural networks offer a powerful approach to understanding customer behavior, capable of discerning patterns from surprisingly small datasets, potentially as few as 100 data points. This is particularly beneficial for smaller businesses with limited data, enabling them to make data-driven decisions in marketing.
By employing recurrent neural networks, we can not only predict future purchases but also analyze the sequence of customer interactions. This sequential understanding is crucial for creating marketing campaigns that are genuinely tailored to the customer journey, leading to more effective engagement.
Deep learning techniques within customer behavior modeling have shown promise in boosting prediction accuracy by over 20% compared to traditional statistical methods. This makes neural networks an appealing choice for businesses seeking to refine their marketing approaches and optimize outcomes.
An intriguing aspect of some neural network architectures is their ability to simulate customer churn. Not only can they identify which customers are likely to churn, but they can also provide insights into the factors driving churn. This predictive capability enables a proactive approach to customer relationship management, helping businesses intervene before valuable customers leave.
Furthermore, neural networks are proficient at sentiment analysis, interpreting the emotions expressed in customer reviews and interactions with a high degree of subtlety. This ability can uncover nuanced feelings that might be easily overlooked by humans, leading to a deeper understanding of customer sentiment.
When coupled with reinforcement learning, neural networks can adapt marketing strategies in real-time, adjusting based on immediate customer feedback and engagement metrics. This continuous adaptation allows businesses to refine their campaigns iteratively, maximizing their impact and effectiveness.
It seems that predictive modeling using neural networks holds potential for identifying emerging trends in consumer behavior. By recognizing subtle shifts in preferences before they become widespread, businesses gain a valuable first-mover advantage in competitive markets.
Research suggests neural networks can unravel non-linear relationships hidden within customer data. This opens doors to understanding the intricate interactions between marketing channels and consumer responses, potentially revealing surprising connections that traditional methods might miss.
Another compelling aspect of neural network-based predictive models is their ability to forecast the long-term value of customers. This provides a valuable tool for resource allocation, allowing businesses to focus on customer segments with the highest potential for long-term profitability.
While the capabilities of neural networks are impressive, it's crucial to acknowledge their dependence on high-quality training data. Poor-quality data can easily lead to inaccurate and misleading predictions, emphasizing the importance of having a robust data strategy before implementing any neural network-based solution for customer behavior modeling.
7 Key AI Applications in NYU's Digital Marketing Certificate Program A 2024 Analysis - Automated Ad Campaign Optimization Through Machine Learning
In the evolving landscape of digital marketing, automated ad campaign optimization through machine learning has taken center stage. AI-powered tools analyze massive datasets in real time, allowing marketers to fine-tune ad campaigns with unprecedented precision. These algorithms enhance campaign strategies by scrutinizing data points and making adjustments based on performance metrics like click-through rates and conversions. Marketers can now respond to campaign performance in real-time, refining approaches to maximize results.
This ability to automate previously manual and time-consuming aspects of ad campaigns, like keyword research and ad placement, is freeing up marketers to concentrate on creative elements that connect with the audience. Yet, there's a critical caveat. The effectiveness of these systems hinges on the quality of data that fuels them. Garbage in, garbage out, as the saying goes. Organizations need to prioritize robust data management strategies to ensure the insights generated by AI are accurate and truly useful. This emphasis on quality control ensures that automated optimization genuinely benefits the campaigns rather than leading to misguided decisions based on flawed data. The future of automated ad campaign optimization promises to further refine these processes, making it even more integral to successful marketing strategies.
Here are ten interesting points about how machine learning automates ad campaign optimization that might be of interest:
1. **Adaptive Bidding**: Machine learning algorithms can dynamically adjust bidding strategies in real time. They consider factors like user behavior, time of day, and device type, potentially leading to a decrease in advertising costs by more than 30%. It's fascinating how these models learn to optimize spending on the fly.
2. **Enhanced Ad Relevance**: Machine learning models can analyze user engagement metrics to refine ad relevance scores. Campaigns utilizing these insights may achieve click-through rates (CTR) up to 50% higher compared to campaigns without these optimizations. The potential for improved ad performance is remarkable.
3. **Accelerated Multivariate Testing**: Traditional A/B testing can take a significant amount of time, but machine learning can automate multivariate testing, allowing marketers to evaluate multiple variations of ads concurrently. This speeds up the optimization process, yielding results in a matter of hours instead of weeks.
4. **Predictive Audience Segmentation**: Machine learning can automatically identify distinct audience segments based on historical data and observed behavioral patterns. This leads to more precise ad targeting and potentially a 40% increase in conversion rates. It's interesting how well these models can pinpoint specific audience characteristics.
5. **Improved Attribution Modeling**: Automated systems powered by machine learning can enhance the understanding of which marketing channels drive the best results. This better understanding of the customer journey allows for more effective allocation of ad spend, potentially maximizing return on investment (ROI).
6. **Leveraging Sentiment Analysis**: Machine learning facilitates a deeper understanding of customer sentiment, analyzing feedback to see how specific ads resonate with diverse demographic groups. Campaigns designed based on this feedback can see a 20-25% improvement in effectiveness. It's thought-provoking how AI can capture emotional responses to ads.
7. **Fighting Click Fraud**: Automated ad optimization tools often employ algorithms to detect suspicious click activity, potentially minimizing wasted advertising budgets. The ability to automatically flag anomalies can save businesses thousands, or even millions, for major campaigns.
8. **Continual Learning**: Unlike traditional static methods, machine learning models are constantly adapting based on new data. This continuous learning capability allows marketers to anticipate shifts in consumer behavior, allowing brands to stay ahead of the curve.
9. **Programmatic Advertising Enhancements**: Machine learning algorithms help improve programmatic advertising by enabling real-time purchasing of ad space. This leads to enhanced targeting and potentially a 200% increase in ROI compared to traditional methods. The efficiency gains in this area are quite impressive.
10. **Automated Creative Generation**: Systems powered by machine learning can generate and test different ad creative formats automatically. They learn which visuals and messages resonate with specific audience groups, potentially boosting engagement rates by over 30%. It's quite an intriguing prospect – having AI explore the most effective ad design automatically.
These examples highlight the significant progress machine learning has brought to digital advertising, prompting new ideas about contemporary marketing approaches. While there's a lot of promise in these techniques, it's also important to consider the ethical implications and potential biases that might be present in the models. Continued research and development will hopefully help us leverage this technology responsibly.
7 Key AI Applications in NYU's Digital Marketing Certificate Program A 2024 Analysis - Natural Language Processing for Social Media Sentiment Analysis
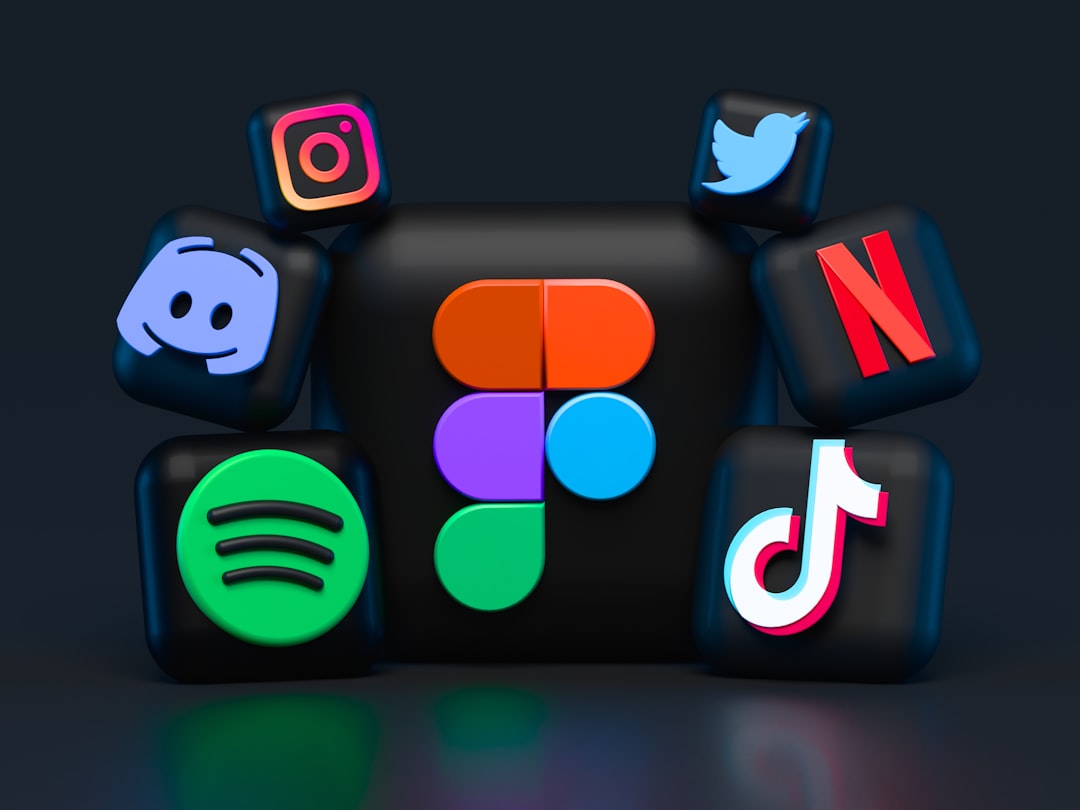
Natural Language Processing (NLP) has become increasingly important for understanding the sentiment expressed within social media. Businesses can leverage NLP to analyze user-generated content – like posts and reviews – and categorize it as positive, negative, or neutral. This allows them to gauge public opinion and get a better understanding of customer feedback. The ability to analyze sentiment is crucial for a wide range of industries, as it supports data-driven decisions and the improvement of marketing efforts.
While promising, NLP-based sentiment analysis still faces certain challenges. Choosing the right datasets and algorithms is a crucial but often complex task. Furthermore, sifting through massive quantities of social media data can be incredibly time-consuming and resource-intensive. Despite these obstacles, NLP is consistently developing, and these advancements are improving the speed and reliability of sentiment analysis. This evolution will reshape how organizations perceive and interact with their customers.
Natural Language Processing (NLP) is increasingly being used to understand how people feel about brands and products on social media, which is called sentiment analysis. It's a way for businesses to get a sense of public opinion and customer feedback, guiding decision-making and improving marketing efforts. NLP helps classify text, like social media posts and product reviews, into categories like positive, negative, or neutral, providing insights into consumer sentiment.
This type of analysis has become important in areas like business, social media, healthcare, and even emergency response. However, challenges remain. One is the need to carefully choose the right datasets and algorithms for the task. Processing all the social media comments out there can be extremely time-consuming, which is another hurdle.
Thankfully, recent progress in NLP has made it easier for organizations to automatically classify and analyze sentiments, making data analysis much more efficient. A wide array of tools are available today, including options like Azure AI Language that allow people to analyze sentiment and summarize text without needing extensive machine learning expertise.
Sentiment analysis is truly transformative in its ability to extract useful information from large volumes of text. This capability is vital for improving product development and shaping more effective marketing campaigns. Research consistently shows that sentiment analysis can significantly improve the insights derived from social media, resulting in smarter strategies for businesses.
NYU's Digital Marketing Certificate program includes AI applications relevant to sentiment analysis, reflecting a growing trend towards integrating these technologies into the field. Researchers often conduct thorough reviews of the literature to evaluate the techniques and results in sentiment analysis, underscoring the importance of continuous critical examination in this evolving area.
Interestingly, NLP is increasingly capable of recognizing subtle emotional nuances beyond simple positive or negative labels. By identifying emotions like joy, anger, or sadness, it helps us gain a much richer understanding of how people feel. Furthermore, it's not limited to just English – NLP models are becoming adept at analyzing various languages and dialects. This means businesses can analyze sentiment on a global scale, gaining insights into how different cultures perceive brands and marketing campaigns.
Another intriguing aspect is the ability of NLP to consider context when analyzing text. This means that the same word can have a different meaning depending on where it appears in a sentence or who's saying it, leading to more accurate sentiment assessment. The tools are also being used to try to predict potential crises by analyzing changes in sentiment patterns, allowing companies to address emerging issues proactively.
However, this ability to analyze a huge amount of data in real time is not without challenges. It requires significant processing power, and managing those data resources can be complex and costly. This emphasizes the need for a well-planned data management strategy. Nevertheless, the ability of NLP to reveal customer preferences, pain points, and track brand health over time makes it a powerful tool for gaining insights from social media data that traditional research methods might miss. Combining NLP with other data like sales figures and website traffic gives marketers a more comprehensive view of how consumer sentiment impacts actual business results, creating opportunities for more targeted approaches.
Finally, NLP can be used to gauge the effectiveness of influencer marketing campaigns by analyzing the sentiment around influencers. It gives brands a data-driven way to understand which influencers are most successful with their target audiences. All of these uses showcase the growing importance of NLP in social media analysis and the continued need for innovative research and development in this field.
7 Key AI Applications in NYU's Digital Marketing Certificate Program A 2024 Analysis - Computer Vision Applications in Marketing Analytics
Within the realm of marketing analytics, computer vision is transforming how companies understand visual information. It allows marketers to derive valuable insights from images and videos, leading to more personalized content and predictive analytics that align with consumer actions. Key applications include the use of optical character recognition (OCR) to analyze images and recognize logos in videos. This enhanced accessibility and the ability to track brand presence across digital platforms are significant benefits.
The growing investment in computer vision technologies for marketing has increased the need for a thoughtful approach to integration. Properly managing the data generated by these tools and considering ethical aspects becomes paramount. Looking ahead, this advancement in marketing analytics implies a future where visual data significantly influences strategies for engaging with customers. It indicates a shift away from more conventional, perhaps less nuanced, marketing techniques.
Computer vision, a field that has seen rapid progress in recent years, is now enabling computers to decipher visual information in a way that mimics human perception. This capability is becoming increasingly important for marketing analytics, particularly as we generate more visual data.
Some of the key applications of computer vision in marketing involve customizing content based on visual cues, forecasting consumer behavior from images and videos, and extracting insights from non-textual digital data like photos and videos. This aligns with the trend revealed in a recent CMO Survey, where content personalization and predictive analytics were identified as the most common uses of AI in marketing. This indicates a growing reliance on data-driven strategies in marketing decisions.
AI's application in marketing has become quite popular, especially in areas like predictive lead scoring. Marketers are using existing data to train algorithms that predict how likely a potential customer is to convert. This kind of analysis allows for a refinement of marketing strategies. Investments in AI-driven marketing have seen a substantial increase, with over 3 billion dollars poured into these technologies at the beginning of 2023. This underlines a broader trend of integrating AI technologies into marketing practices.
A PwC report estimates that AI's contribution to the global economy could reach a staggering 15.7 trillion dollars by 2030. This signifies the immense potential economic impact of AI. It's also influencing how brands interact with customers. AI, particularly computer vision, is enabling more focused and tailored approaches to marketing by leveraging the power of personalization and segmentation.
Optical Character Recognition (OCR), a component of computer vision technologies, allows machines to understand images, including recognizing logos in videos and extracting text from images. This is important for improving accessibility and providing deeper insights into the context of visual data. Furthermore, the emerging field of generative design AI has the potential to greatly speed up the product development lifecycle, allowing brands to react faster to market shifts and changes in consumer preferences.
Ultimately, the transformative potential of AI in marketing is evident in its ability to generate valuable insights from visual data. This leads to better customer experiences and ultimately, improved marketing effectiveness. However, while promising, we need to be aware of potential biases and ethical considerations within these technologies. Ongoing research and development will be necessary to continue harnessing AI's benefits responsibly and ethically.
7 Key AI Applications in NYU's Digital Marketing Certificate Program A 2024 Analysis - Voice Recognition Integration for Customer Service Automation
Voice recognition is becoming a crucial component in automating customer service, particularly in areas like finance and healthcare where precise communication is essential. While some people still believe voice technology is too complex or outdated, it's quickly proving its worth. Automated systems, including voice bots and IVR systems, can now manage routine inquiries, direct calls efficiently, and speed up response times. This not only expands support availability beyond typical business hours but also allows AI to analyze how customers talk to better tailor the service to their needs. However, it's crucial for businesses implementing these tools to carefully think about the ethical consequences of their use and make sure they're using high-quality data to ensure the customer experience remains positive and productive.
Voice recognition technology has become increasingly refined in recent years, demonstrating a remarkable ability to understand human speech with accuracy, particularly in controlled settings. Error rates are now as low as 5% in some instances, which is critical for customer service applications. If a customer service system misinterprets a request, it can quickly lead to frustration, a common problem that this advancement potentially solves. This precision in interpreting speech across multiple languages and dialects is significant. Companies can cater to a broader customer base globally by implementing systems that handle different languages and regional variations in speech. It's also interesting how these systems are being built to sense emotion in a customer's voice. The capacity to detect the emotional state of customers during an interaction, be it frustration, excitement, or neutral, empowers customer service to prioritize urgent issues and tailor responses to a customer's emotional state, potentially fostering greater customer satisfaction.
A common application is improving the overall efficiency of customer service operations. Studies have shown that integrating voice recognition into customer service has led to handling time reductions of as much as 30%, primarily through the automation of routine inquiries. This shift allows human customer service agents to address more intricate and nuanced issues that require human interaction. Yet, the integration of such systems also raises significant privacy concerns, specifically related to data collection and the need for transparent consent from users. Navigating the regulations around privacy while building user trust in such a way is a critical aspect of using this technology without inviting potential legal complications or harm to a brand's reputation.
Voice recognition enables convenient hands-free interaction, proving particularly useful for situations where a customer's hands may be busy. Customers can utilize services while driving, cooking, or performing other tasks. Machine learning, often at the core of modern voice systems, is constantly improving these systems' capabilities over time. As a system accumulates data, it refines its ability to accommodate individual accents, speech rates, and even unique vocabulary choices, resulting in a more personalized experience. This constant adaptation and refinement to better understand individual users is something that sets it apart from previous generations of this technology.
The financial viability of implementing voice-based customer service systems is becoming increasingly attractive, potentially delivering a strong return on investment (ROI). Organizations can realize savings up to 50% in operational costs by leveraging these systems and thereby reducing the number of human employees needed to handle calls. While this increased automation does lead to a shift in workforce dynamics, the jobs that remain become more specialized. Instead of simply addressing basic inquiries, agents are often required to focus on more complex problem-solving and empathic communication. Finally, consumer behavior continues to evolve, and this new tech aligns with that change. Consumers increasingly prefer to interact with businesses through voice-based interfaces as opposed to more traditional methods. Companies implementing voice recognition are aligning their customer service with this preference, a shift that might lead to improved customer loyalty.
It's interesting to observe how voice-based systems continue to refine their capabilities in terms of accuracy, language support, and even the capacity to understand subtle emotional cues in human speech. This has led to real-world benefits in the area of customer service, but it's also imperative to address the privacy concerns that arise from handling so much sensitive audio information. The future of customer service might depend on how seamlessly it addresses the needs of consumers and ensures that personal data is handled responsibly.
7 Key AI Applications in NYU's Digital Marketing Certificate Program A 2024 Analysis - Personalized Email Marketing Through Deep Learning Algorithms
Deep learning algorithms are revolutionizing personalized email marketing. Marketers can now leverage machine learning to tailor email content to individual customer preferences, leading to a significant boost in engagement and a more positive customer experience. This shift signifies a move away from generic email blasts towards highly personalized messages that are specifically designed to resonate with each recipient. The emergence of generative AI further enhances this capability, allowing for the rapid creation of customized and contextually relevant email content based on user behavior and interactions. Through continuous machine learning processes, companies gain a deeper understanding of their customers, leading to increasingly effective email marketing campaigns that are finely tuned to individual interests and needs, creating more meaningful interactions. While this technology holds great potential for enhancing the customer experience, it's crucial to remember that continued development and refinement are essential to ensure that personalization doesn't become a tool for intrusive or manipulative tactics.
Deep learning algorithms are increasingly being used to personalize email marketing, marking a shift from generic messages to highly tailored communications. These algorithms analyze vast amounts of data on user behavior, preferences, and engagement, allowing marketers to adapt email content and timing in real-time. For instance, an email might suggest products based on recent browsing history or even recommend a subject line based on prior open rates.
One notable feature is the ability to tailor the very tone and style of an email based on a customer's emotional patterns gleaned from past interactions. This allows for much more nuanced messaging, potentially enhancing the quality of customer communication. Further, algorithms can predict the best time to send an email, capitalizing on a recipient's historical patterns of engagement, potentially resulting in significant improvements in click-through rates.
Deep learning facilitates highly granular audience segmentation based on complex behavior patterns and preferences. This permits more precise marketing resource allocation and can lead to substantial improvements in conversion rates. The process of A/B testing, traditionally a time-consuming exercise, can be automated and accelerated by deep learning, enabling faster optimization of campaign designs.
The use of deep learning isn't limited to just text. Algorithms can even tailor the types of visuals within an email to match a user's aesthetic tastes. Interestingly, deep learning systems can identify potential signs of customer churn based on a person's interaction history. This allows for proactive efforts to retain valuable customers by sending strategically designed messages with personalized offers.
Integration with Natural Language Processing (NLP) enables deep learning systems to create emails that read in a more natural, human-like way, adjusting to a recipient's individual preferences for communication style. Finally, the ability to forecast the long-term value of a customer through deep learning models allows marketers to optimize campaigns by focusing efforts on those who are most likely to remain profitable for a business, leading to a greater return on investment.
This emerging approach is fundamentally altering the landscape of email marketing, demonstrating how data-driven strategies can foster more effective engagement with consumers. However, as with all AI applications, there are important ethical considerations surrounding data usage and privacy that require ongoing research and scrutiny. The future direction of personalized email marketing is intertwined with how we ethically leverage these powerful technologies to optimize user experience while respecting individual privacy.
Create AI-powered tutorials effortlessly: Learn, teach, and share knowledge with our intuitive platform. (Get started for free)
More Posts from aitutorialmaker.com: