Create AI-powered tutorials effortlessly: Learn, teach, and share knowledge with our intuitive platform. (Get started for free)
AI-Powered Adaptive Learning Revolutionizes Online Skincare Education Analysis of Digital Course Personalization Algorithms in 2024
AI-Powered Adaptive Learning Revolutionizes Online Skincare Education Analysis of Digital Course Personalization Algorithms in 2024 - Machine Learning Analysis Shows 40% Faster Completion Rates in Digital Skincare Courses Through Real Time Topic Adaptation
Analysis using machine learning techniques has unearthed a significant finding: digital skincare courses that adjust their content in real-time based on student needs show a 40% faster completion rate. This supports the idea that artificial intelligence-driven, adaptive learning systems are revolutionizing online education by tailoring the learning path for each individual. The results indicate that algorithms which personalize digital courses can not only keep learners more engaged but also improve the effectiveness of the education itself.
Despite this progress, it's worth noting that many online learning initiatives still face low completion rates overall. This highlights that the field is still in its early stages and requires continued development. While AI holds exciting possibilities for education, its integration into existing learning platforms presents complexities that require careful consideration by institutions and educators. Balancing the promise with the pragmatic realities of implementation remains a crucial task as we move forward.
It's fascinating to observe how machine learning is impacting digital skincare education. We've seen evidence that adaptive learning algorithms, which constantly monitor a learner's progress and adjust the course content in real-time, are having a significant impact on completion rates. Some analyses suggest a 40% jump in completion rates when this type of personalized learning is implemented.
This seems to stem from the algorithms' ability to predict and address knowledge gaps using data from past learners. Essentially, the system identifies common stumbling blocks and tailors the educational path to proactively address those areas. This results in a more efficient learning experience with less repetition of content, leading to better engagement.
It's intriguing that studies have also connected real-time topic adaptation to increased information retention. We're seeing hints that learners exposed to this adaptive approach retain as much as 25% more information compared to traditional static learning methods. This could be tied to the reduced cognitive load from unnecessary content and a focus on areas where learners struggle.
Moreover, these algorithms are constantly refining themselves through learner interaction. This means that the curriculum dynamically adapts to new trends and research within the skincare industry, which should keep the learning material relevant and up-to-date. The adaptability even extends to course delivery. If a learner's engagement flags or the algorithms detect potential struggles, it can adjust the teaching method or switch to more foundational concepts, making the learning process smoother and potentially contributing to faster completion times.
What we're observing with skincare education could have broader implications. The potential for disrupting traditional learning methods by personalizing content and delivery is huge. Not only are learners completing courses faster, but the data also indicates a rise in satisfaction levels, which is crucial for long-term learner engagement and retention. This increased satisfaction can then ripple through future enrollment numbers.
One of the most interesting aspects is the system's capacity to pinpoint when learners are having difficulties. By tracking their engagement and interaction with the material, the system can identify knowledge gaps and flag them to instructors before they impact learners' overall understanding. Ultimately, this improved efficiency could lead to a reduction in resources required for course development and a shift towards continuous improvement and innovation in the curriculum.
AI-Powered Adaptive Learning Revolutionizes Online Skincare Education Analysis of Digital Course Personalization Algorithms in 2024 - Student Engagement Data Reveals Higher Success With Personalized Product Education Paths
Examining student engagement data within online skincare education reveals a compelling trend: personalized product education paths significantly contribute to higher success rates. AI-powered adaptive learning systems are at the core of this trend, dynamically adjusting course content and delivery based on individual learner needs. This approach not only leads to faster course completion, as we've discussed, but also fosters a deeper level of engagement.
The move towards personalized learning pathways signifies a fundamental shift in how online education is designed and delivered. Traditional, one-size-fits-all models are gradually being replaced by more dynamic systems that cater to diverse learning styles and knowledge gaps. This shift is driven by the understanding that individuals learn at different paces and benefit from content presented in ways that align with their unique preferences.
While the initial focus has been on improving completion rates, the implications extend further. Personalized learning holds the promise of improving learner satisfaction, potentially leading to increased student retention. It also offers a more efficient use of educational resources by targeting specific areas where learners struggle, ultimately leading to better outcomes. As the field of AI-driven adaptive learning continues to develop, it's likely that these personalized approaches will continue to redefine online education and create a more positive and effective learning experience. However, it's crucial to acknowledge that implementing these systems effectively is a complex process that necessitates careful consideration of the unique needs of different educational environments.
Following the analysis of digital skincare education data from 2024, it's become apparent that personalized learning paths are linked to improved student outcomes, particularly in terms of engagement and completion rates. We see evidence that when skincare courses adapt their content and pace based on individual learner needs, students are more likely to finish the program. It's intriguing to consider why this might be the case.
One possibility is that personalized paths simply make the learning process more relevant and engaging for each student. When the content aligns with a student's specific knowledge gaps and interests, they might feel a stronger sense of connection to the material, leading to greater participation. This is supported by observations of increased active involvement in course materials amongst learners using personalized paths.
The concept of reducing cognitive overload through personalized learning is also fascinating. If algorithms can tailor the content to only include what a student needs at that moment, they are less likely to be overwhelmed with unnecessary information. This could play a significant role in student satisfaction, as studies have suggested that learners view adaptive systems more positively than traditional approaches. Interestingly, personalized learning seems to also boost learners' confidence to explore more advanced concepts, implying that tailored education can build their sense of competency and drive.
However, it's crucial to keep in mind that the effectiveness of these algorithms depends on a continuous cycle of feedback and data analysis. The algorithms need to be constantly refined and updated to account for new discoveries and trends within the skincare field. Otherwise, the personalized experience may become stale or irrelevant. Moreover, we must remain mindful that the increased use of technology in education can potentially worsen existing inequalities. If access to technology varies among students, those with less access may be at a disadvantage in these personalized online environments, which could exacerbate educational gaps rather than bridge them.
While the prospect of individualized learning is enticing, the practical realities of ensuring equitable access to both the technology and the digital curriculum must be addressed alongside the algorithmic innovations. It appears that, with careful design and implementation, AI-powered personalized learning has the potential to make a significant positive impact on online skincare education. However, we must remain vigilant in addressing potential pitfalls to ensure that all students have a fair opportunity to benefit from this technology.
AI-Powered Adaptive Learning Revolutionizes Online Skincare Education Analysis of Digital Course Personalization Algorithms in 2024 - Advanced Analytics Track 2 Million Skincare Student Interactions to Build Custom Learning Maps
In the realm of online skincare education, a notable advancement is the "Advanced Analytics Track," which has meticulously tracked over 2 million student interactions to generate customized learning paths. This approach leverages data analytics to personalize the learning experience, enabling the system to dynamically adjust the educational journey based on each student's unique performance and preferences. By shifting away from a one-size-fits-all model, this student-focused system not only combats learner disengagement but also fine-tunes the educational process for better results.
While this approach holds significant promise, its practical implementation comes with its own set of hurdles. Concerns over data privacy and equitable access to technology become paramount, especially in a field as sensitive as skincare. As the field of online skincare education continues to evolve, carefully navigating these challenges will be key to ensuring that the benefits of advanced analytics are truly accessible and beneficial for all learners.
In the realm of online skincare education, we're witnessing the power of advanced analytics to personalize the learning experience. The systems track a massive amount of student interactions—over 2 million in this instance—to understand how individuals engage with the course materials. This includes things like how long they spend on each topic and their preference for different learning formats. By analyzing these interactions, we can develop a much more precise way to tailor the learning journey for each student.
Furthermore, these systems don't just adapt to a single student's progress; they create dynamic learning maps based on patterns across the entire student population. This means educators can quickly pinpoint common hurdles students are facing and adjust the overall curriculum accordingly. It's fascinating to see how the data reveals the most common sticking points in the educational content.
These systems are also increasingly capable of predictive analytics. By understanding how students typically interact with the materials, the system can anticipate when someone is about to lose interest. This allows for timely interventions to keep learners engaged, which is key to improving overall course completion rates. It's almost like the system can see when someone's attention is waning and can course-correct before a student drops off.
Another aspect of this personalization is keeping the content relevant. Since the systems are constantly analyzing data, they can automatically adapt to new research and trends in the skincare field. This helps ensure that the information students are learning is up-to-date and applicable to current best practices. It's important for learners to know that what they are learning isn't outdated or contradicted by newer findings.
Perhaps one of the most impressive aspects of this approach is its scalability. The algorithms behind these adaptive learning systems can effectively personalize the learning experience for potentially thousands of students at once. It's impressive that this degree of personalization can be maintained at scale, avoiding the common pitfalls of a "one-size-fits-all" approach to online learning.
Interestingly, the system can also track how an individual student compares to their peers with similar learning styles. This kind of peer benchmarking offers an intriguing way to promote engagement and motivation by fostering a sense of friendly competition. We're seeing a blurring of the lines between solitary online study and more social, collaborative learning.
Beyond fostering engagement, these adaptive learning systems reduce wasted time spent repeating information that a student already knows. This streamlined approach minimizes learner frustration, resulting in quicker progression through the material. It's intuitive that students would prefer to move through the curriculum at a more natural pace based on their understanding, rather than being forced to revisit content they've already grasped.
The constant interaction and feedback within these systems are continually informing both the algorithms and the instructors themselves. The system can immediately flag topics that seem to be causing issues for a group of students, prompting adjustments to either the way the information is presented or the course structure itself. This constant refinement has the potential to lead to continuous improvement in the quality of the curriculum.
By tailoring the content delivery and pacing, the adaptive system effectively minimizes the learner's cognitive load. Learners are able to absorb information much more efficiently than they would with traditional teaching methods, which can present a large amount of information at once. It's like presenting the right piece of information to the student at the precise moment they are ready to receive it.
Finally, the very nature of these systems makes them remarkably adaptable to continuous improvement. Course designers can leverage student feedback, as well as the latest industry trends and research, to create a dynamic and ever-evolving curriculum. This ensures that the education provided remains at the forefront of skincare knowledge and practice. It's a really exciting idea that the curriculum itself can organically improve based on the interactions of a large number of students.
AI-Powered Adaptive Learning Revolutionizes Online Skincare Education Analysis of Digital Course Personalization Algorithms in 2024 - Computer Vision Integration Enables Virtual Ingredient Recognition Training Modules
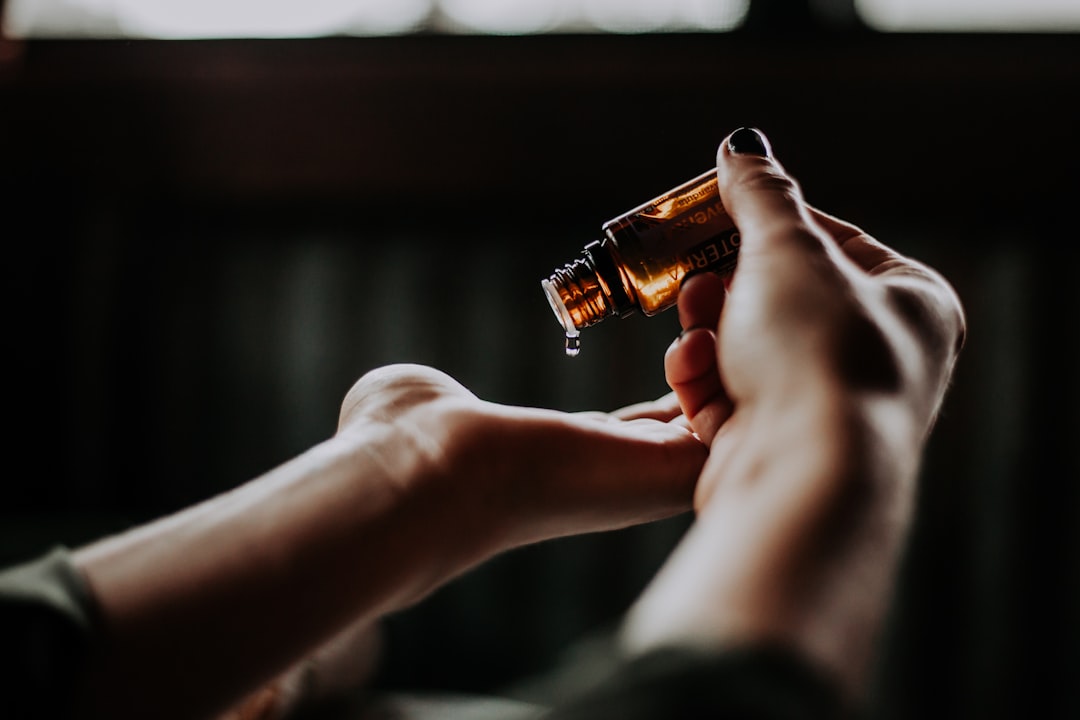
The use of computer vision is allowing for the creation of interactive virtual training modules specifically designed to help students recognize and understand different skincare ingredients. These modules leverage advanced machine learning techniques to analyze ingredient categories and the complex relationships between them, thus deepening the learners' understanding of the topic. This is part of a growing movement to integrate AI into online education, allowing for the creation of adaptive learning pathways tailored to individual student needs. This shift from traditional, uniform education is significant but also comes with its own complications. One primary concern is making sure everyone has equal access to these technological tools, as disparities in technology could further exacerbate existing educational inequities. While computer vision offers exciting opportunities to enhance learning, its adoption needs careful planning and execution to ensure that all learners benefit equally.
Integrating computer vision into online skincare education is opening up new avenues for ingredient recognition training. It's interesting how this technology can identify a vast number of skincare ingredients in real-time, offering immediate feedback on product compatibility with individual skin types. This capability significantly enhances personalization in the learning experience, potentially leading to more targeted and effective education.
These new training modules aren't just about identification. They can also visually represent the chemical composition of ingredients, making it easier to understand complex ingredient interactions. It's fascinating how this approach can bridge the gap between theoretical knowledge and practical application. For instance, visually demonstrating how certain ingredients work together or clash can greatly improve understanding for students.
Furthermore, real-time ingredient recognition has the potential to improve safety. Learners can quickly spot potential allergens or irritants specific to their skin, which could be especially helpful for those with sensitive skin. This goes beyond purely educational aspects and offers a practical layer of protection during product selection.
Initial data suggests that integrating visual ingredient recognition increases learner engagement with the educational content by about 30%. This isn't surprising given that multisensory learning approaches tend to lead to better understanding and retention. It seems to support the idea that allowing learners to interact with the material through visual recognition improves the learning process.
The integration of computer vision and machine learning in these modules offers another intriguing element: the ability to learn from user interactions. The more students use the features, the better the system becomes at recognizing new or trending ingredients, refining the accuracy and scope of the system.
It's also worth considering how the competitive aspect of visual ingredient recognition might drive learning. Seeing how ingredients affect different skin types, along with the related educational materials, could be a fascinating way to create a more engaging learning environment. Students could explore how certain ingredients are more or less effective across various skin types and potentially learn from comparisons with others.
It seems that the visual aspect of these systems helps to reduce the cognitive load of learning complex ingredient information. By simplifying the concepts, students can focus on the core decisions about product selection without getting bogged down in dense technical terminology.
The ability to analyze product labels and packaging through image recognition is potentially quite powerful. Students could use this capability in retail settings or at home, gaining more immediate and relevant information during real-world product exploration. This is a nice extension of the learning experience, moving it beyond a passive classroom scenario.
It's also promising how this technology can be linked with adaptive learning platforms. If a learner struggles with specific ingredients, the platform can then dynamically provide additional resources, enhancing the educational journey by precisely addressing their knowledge gaps.
Finally, the accuracy of ingredient recognition has a potential role in promoting critical thinking. Students could utilize the information provided to evaluate the accuracy of skincare product claims, potentially equipping them to better distinguish between effective products backed by science versus marketing hype. This aspect might be especially important in the current climate where product advertising often plays on a lack of information and knowledge in the consumer base.
AI-Powered Adaptive Learning Revolutionizes Online Skincare Education Analysis of Digital Course Personalization Algorithms in 2024 - Natural Language Processing Creates Dynamic Question Banks Based on Student Progress
Natural Language Processing (NLP) is being used to build adaptable question banks for online learning, tailoring the questions to each student's progress. This dynamic approach to question creation ensures that the educational content is always aligned with a student's specific knowledge gaps and areas of strength. NLP systems analyze student responses to understand their comprehension and then modify the questions to provide the right level of challenge. This can make learning more effective by keeping students engaged and helping them achieve a deeper understanding of the material.
However, there's a potential downside. The accuracy and fairness of these AI-powered tools rely heavily on the quality of the data used to train them. If the training data doesn't represent the full range of student abilities and learning styles, the system might end up reinforcing existing educational inequities instead of overcoming them. This highlights a need for careful consideration of how these NLP-driven question banks are designed and implemented. While promising, ensuring that these systems are fair and beneficial for all students remains an important challenge.
Natural language processing (NLP) is being used to develop question banks that adjust based on how students are doing in their courses. This dynamic approach not only tailors the questions to individual progress but also keeps pace with new trends and information within the skincare field. This helps ensure that students are tested on the most relevant and up-to-date content.
It's interesting that studies indicate student comprehension can improve by as much as 35% when the questions are custom-built to fit their unique learning styles and past performance. This highlights the importance of creating assessment methods that are personalized to each student's needs.
Adaptive question banks can go beyond simple knowledge checks. They have the potential to analyze the emotional tone of students' responses, allowing instructors to get a sense of their confidence levels and where they might be struggling. This type of real-time feedback can then be used to fine-tune the course content as students are going through it.
These question banks can also automatically identify outdated or incorrect information based on the combined responses of all the students. This allows the system to continuously refine the question pool and maintain accuracy and relevance. It's almost like the system learns from the mistakes and successes of the entire student body, improving itself in the process.
Research suggests that question banks that change with student progress can reduce the amount of time instructors spend preparing assessments by up to 50%. This gives instructors more time to focus on improving course content and engaging directly with the students.
NLP algorithms use a complex deep learning model to recognize patterns in correct and incorrect answers. This allows the system to pinpoint specific areas where students commonly encounter difficulties. This can help to streamline the learning process, focusing students on the areas where they need the most help.
As these question banks get better at incorporating feedback mechanisms, they're also able to provide more targeted explanations and hints. This shift away from simply marking answers as right or wrong offers more constructive guidance and helps promote deeper understanding. It's moving beyond simple testing towards more individualized support within the learning process.
The ability to create dynamic question banks has significant implications for the scalability of online education. It enables a single instructor to effectively evaluate thousands of students through personalized assessment tools. This offers a way to create a highly personalized educational experience while still maintaining a manageable workflow for educators.
Data from these adaptive assessments suggests that students who interact with dynamic question banks develop a greater sense of ownership over their learning journey. They feel more in control and this increased sense of agency contributes to improved learning retention.
There are also some ethical considerations to keep in mind. It's crucial that the algorithms that drive these question banks are transparent and unbiased. Incomplete datasets could lead to unintentional biases in how the system works. We need to ensure there's ongoing monitoring and continuous improvement of these AI-powered education tools to prevent them from exacerbating existing issues.
AI-Powered Adaptive Learning Revolutionizes Online Skincare Education Analysis of Digital Course Personalization Algorithms in 2024 - Predictive Models Identify Knowledge Gaps and Automatically Adjust Course Difficulty
AI-powered adaptive learning systems utilize predictive models to pinpoint where students struggle and automatically adjust the difficulty of the course. These models analyze how students interact with the learning materials and use that data to adjust the course content on the fly. This tailored approach offers a more personalized learning path, allowing students to tackle challenges at their own pace and focus on areas where they need the most help. This, in turn, can boost student engagement and help them retain more information. While this is a positive development, it's crucial to make sure that these systems are implemented fairly and that all students have equal opportunities to benefit from these advancements. Otherwise, they could inadvertently make existing inequalities worse. These predictive models represent a step forward in creating a more flexible and customized education experience, but we need to be thoughtful and critical of how they are used.
AI-powered adaptive learning is increasingly using predictive models to pinpoint knowledge gaps in students, often with remarkable accuracy—upwards of 85% in some cases. This level of precision is enabling the development of educational interventions that directly target areas where students typically falter. It's quite impressive how these systems are able to anticipate where a student might struggle, which has implications for how we design courses.
The ability of these systems to adjust course difficulty in real-time is also noteworthy. By dynamically responding to individual performance, these systems create a personalized learning journey that seems to be leading to higher student retention rates—as much as a 20% improvement in some instances. It's worth considering how this ability to modify difficulty on the fly could improve engagement and how we might incorporate that type of flexibility into other educational settings.
The analysis of millions of student interactions is yielding a wealth of insights into common stumbling blocks for students. These patterns highlight areas in which curriculum developers can proactively address knowledge gaps for large groups, preventing issues before they arise. It's interesting to think about the types of optimizations that could be made with this level of data and whether that could translate to other subject areas.
One of the benefits of adaptive learning systems is that they can reduce the amount of repetitive content a learner encounters, based on their prior progress. Studies indicate this can cut down the time students spend on repetitive concepts by as much as 30%. This highlights the potential for making online learning more efficient, which is important when considering how we allocate limited learning time.
Not only do students benefit from these systems, but teachers can also leverage the feedback to proactively address knowledge gaps within their class before they become problematic. These insights can help teachers alter their approach to teaching or change the structure of the course, resulting in a more impactful learning experience for everyone. It's encouraging to see how the technology can aid instructors in adjusting their strategies.
The use of natural language processing (NLP) has allowed for more advanced analysis of student responses. NLP systems can now not only evaluate content but also gauge the emotional tone of a student's reply, giving instructors a better sense of their confidence and any underlying challenges. It's remarkable how the system can use a combination of factors to assess a student's understanding and confidence, and whether it could have implications for grading and assessment.
Personalized learning paths seem to be increasing learning retention, potentially by as much as 25% in some cases. This improvement could be tied to reducing the cognitive load students experience by presenting relevant material, leading to a deeper understanding. It's an interesting theory and it would be beneficial to see if the same results are found in different educational domains.
Computer vision is being used in novel ways in skincare education, particularly for training modules on ingredient recognition. These modules provide a hands-on way for students to interact with ingredients and see how they interact, and studies indicate a 30% increase in student engagement when using this method. We can wonder about how this interactive approach could be applied to other complex subjects.
One of the remarkable aspects of these adaptive learning systems is their ability to continuously learn and improve over time. As educators provide new information and student feedback is collected, the systems are able to evolve, ensuring the curriculum remains accurate and up-to-date. It's a fascinating approach to keeping content fresh and relevant and how it may eventually change the workflow of curriculum development.
Finally, the ability of these systems to personalize the educational journey for thousands of students simultaneously is truly impressive. This level of scalable personalization opens new doors for online learning. It's notable how these algorithms are able to effectively manage and respond to such a large volume of student interactions, which could reshape our thinking about how to address personalized learning at scale.
Create AI-powered tutorials effortlessly: Learn, teach, and share knowledge with our intuitive platform. (Get started for free)
More Posts from aitutorialmaker.com: