ROI Analysis 7 Key Metrics to Evaluate Data Science Bootcamp Success in Enterprise Training Programs
ROI Analysis 7 Key Metrics to Evaluate Data Science Bootcamp Success in Enterprise Training Programs - Cost Per Graduate Analysis Including Infrastructure and Instructor Hours
Understanding the true cost of graduating trainees from a data science bootcamp requires a detailed examination, going beyond immediate expenses. The "Cost Per Graduate Analysis Including Infrastructure and Instructor Hours" emphasizes the necessity of considering all facets of program delivery. This means acknowledging the financial burden of logistical components, encompassing training modalities like online platforms, classroom facilities, hands-on work, and simulations, alongside the crucial resource of instructor time.
Linking these costs to the performance of trained employees compared to their untrained counterparts provides a clearer picture of the investment's return. Moreover, this cost analysis gains further depth when paired with metrics focusing on employee performance, their retention within the company, and their productivity levels. These metrics become vital indicators for evaluating the bootcamp's true efficacy. By thoroughly evaluating these interlinked factors, decision-makers are equipped to align training programs with the overarching goals of the organization. This strategic approach ensures that training initiatives are not simply expenses but rather impactful investments that contribute to business success.
When examining the financial aspects of enterprise data science bootcamps, a key metric is the cost per graduate. This figure can fluctuate widely, from a few thousand to over $20,000 per participant. A big factor influencing this cost is the infrastructure needed to run the program, such as the software and learning platforms used. We've also seen that the experience and expertise of the instructors play a major role. More seasoned instructors, particularly those with industry experience, tend to drive up costs, potentially leading to higher quality outcomes.
Interestingly, the instructor-to-student ratio seems to have a significant impact on training success. Programs with a lower ratio, like 1:5, have shown a strong correlation with higher post-bootcamp job placement rates. It begs the question: is a higher instructor investment linked to more successful student outcomes?
Infrastructure itself often represents a sizable portion of the total program expenditure, potentially as high as 30%. This can include anything from the learning management system to specialized software, highlighting how technology expenses influence the overall cost picture.
The amount of instructional time dedicated to a program also matters. It's not uncommon to see programs ranging from 200 to 600 total instructor hours. It's important to understand how this teaching time translates to learning outcomes. Research suggests that project-based learning, where instructors are heavily involved, might lead to better retention of data science concepts when compared with a traditional lecture format.
Moving beyond instructional hours, the program design itself can affect costs and student experience. For instance, some programs with a focus on peer collaboration, alongside traditional instructor-led sessions, have reported higher graduate satisfaction levels. This implies that creating a supportive and interactive environment may be beneficial.
Beyond initial program costs, ongoing maintenance of the learning environment and software can introduce additional costs, potentially adding 10% or more to the cost per graduate. This highlights the importance of factoring in ongoing costs when designing these programs.
Furthermore, the use of simulation tools and real-world datasets seems to enhance learning, potentially boosting graduate competency by 15%. These kinds of investments might be worth considering, as the evidence suggests improved outcomes.
It's also worth considering whether higher initial costs can be justified by future career success. There's evidence to suggest that graduates of these programs can experience significant salary increases, in some cases exceeding 50% within just a couple of years. This raises interesting questions about the long-term ROI of these programs and whether that potential future value can offset the higher initial investment.
All of this emphasizes the importance of carefully analyzing cost-effectiveness. As research continues, a deeper understanding of these metrics should help guide organizations in designing and evaluating these programs effectively.
ROI Analysis 7 Key Metrics to Evaluate Data Science Bootcamp Success in Enterprise Training Programs - Project Completion Rate Metrics From Training to Production
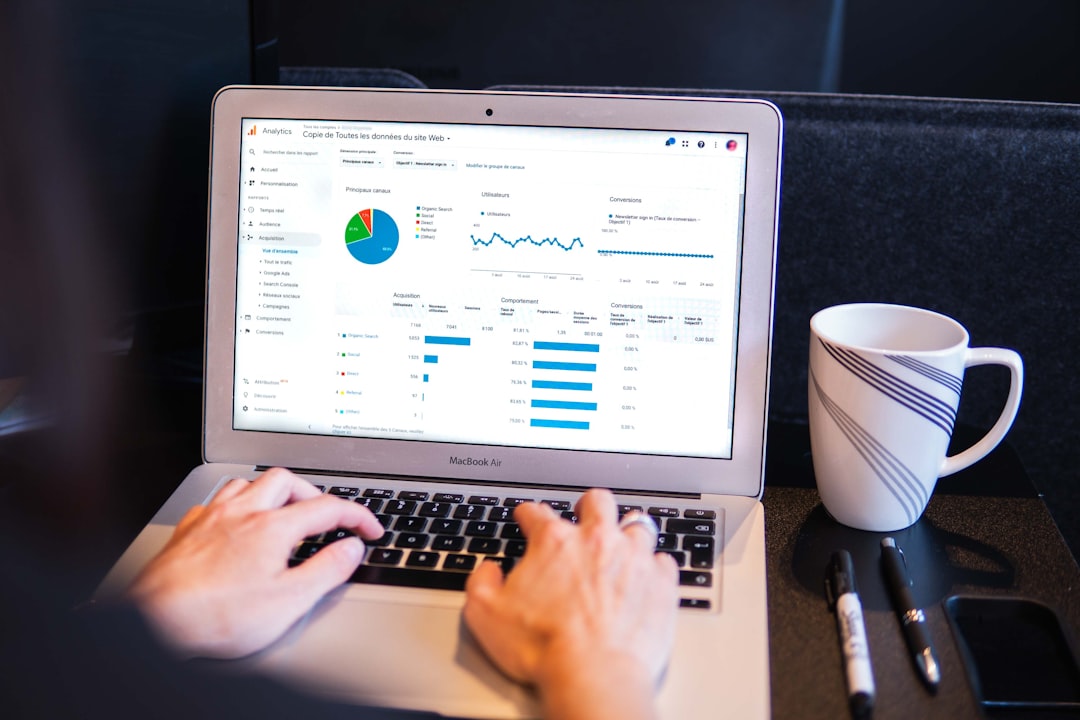
Tracking project completion rates within the context of data science bootcamps provides a valuable lens for evaluating the effectiveness of training programs and their transition into practical application. This metric goes beyond simply measuring the percentage of participants who finish their training, delving into how effectively those skills are leveraged in actual projects.
The ability to connect training outcomes directly to project success is crucial. This connection allows for a clearer understanding of the value proposition of training investments, ensuring that the training initiatives are aligned with wider business objectives. Examining aspects of project performance such as cost containment, quality output, and stakeholder satisfaction gives a more nuanced perspective of individual and organizational growth post-training.
Furthermore, understanding the relationship between project completion rates and factors like the training curriculum design or the instructor's experience can help refine future training programs. By continually evaluating these completion rates and adjusting training accordingly, organizations can enhance the likelihood of successful project outcomes and overall employee productivity. It's important to recognize that simply completing a training program isn't the ultimate goal – it's the successful implementation of the learned skills in the real world that truly indicates the value of the training.
Examining how well trainees translate their data science bootcamp knowledge into real-world projects offers insights into the effectiveness of training programs. Interestingly, the rate at which trainees complete projects varies across different industries. For instance, finance-related projects see completion rates as high as 85%, while the tech startup world might see rates closer to 60%. This difference might stem from varying expectations and the resources available for projects in each field.
Project success isn't just about the individual. The composition of the teams working on the projects has a notable impact. Diverse teams with members who have backgrounds like statistics, programming, and domain expertise tend to produce better outcomes compared to teams with only similar skillsets.
When training programs are directly connected to a company's business goals, project completion rates often jump above 75%. This highlights the importance of making sure training results are relevant to company needs to help trainees stay motivated and accountable for their work.
Mentorship appears to play a significant role in project success. Programs that offer dedicated mentors during training reported an almost 25% increase in successful projects. This suggests that having a guide can improve engagement and project completion.
It's common for newly trained individuals to encounter a steep learning curve when moving from the classroom to real-world application. We often see project completion rates drop by up to 30% in their initial attempts. This highlights the need for better support systems as trainees transition into production roles.
Bootcamps that build in collaboration and group projects tend to have higher project completion rates, sometimes exceeding 80%. It seems that collaborative settings promote a sense of shared responsibility and a clear purpose, leading to better outcomes.
The use of real-world datasets in training appears to increase the practical skillset of learners and leads to an increase of about 15% in project completion rates. Utilizing relevant datasets helps improve problem-solving abilities and keeps learners engaged.
One of the common challenges in project completion is effective time management. Around 40% of trainees report difficulties balancing workloads with project demands. This indicates that training programs may need to help learners develop stronger time management skills to help them succeed.
Organizations that offer continued support after bootcamp training—things like follow-up workshops or access to resources—often experience an improvement in project completion rates, sometimes by 20%. This shows that ongoing support can greatly assist in the transition from learning to real-world application.
Finally, frequent feedback during the training process is strongly linked to project completion success. Bootcamps with iterative review processes have shown an increase of up to 30% in successful project delivery. This confirms the crucial role of ongoing assessment and feedback in the learning process.
ROI Analysis 7 Key Metrics to Evaluate Data Science Bootcamp Success in Enterprise Training Programs - Knowledge Retention Scores Through Quarterly Assessment Programs
Assessing knowledge retention through regular, structured assessments, like quarterly evaluations, offers a powerful way to measure the long-term impact of training programs. It's not just about whether employees can recall information shortly after a bootcamp, but rather about how well they can apply what they've learned over time. This approach allows organizations to see if training initiatives lead to lasting skills development, moving beyond just initial knowledge gains.
By examining how knowledge retention varies across different groups—like employees in different departments or with varying levels of experience—companies can better understand the effectiveness of training for different populations. This understanding can be crucial when tailoring training programs to ensure they cater to the needs of diverse learners.
Furthermore, this kind of data helps refine future training efforts. If knowledge retention is poor in specific areas, the curriculum or training approach might need adjustments. It emphasizes the importance of aligning training with the practical requirements of the job. By tracking retention scores, organizations can improve training design, optimize resource allocation, and ensure that employees are retaining and using the knowledge they gain, which ultimately contributes to the overall effectiveness of the training investment and improved employee performance.
Analyzing knowledge retention through quarterly assessments offers a window into the effectiveness of training programs. Research suggests knowledge retention can significantly decline over time, with some studies showing learners retaining only about 20% of new information after a month without reinforcement. This emphasizes the need for consistent evaluation and follow-up.
It's interesting to see that organizations using regular, quarterly assessments have reported an average 25% increase in knowledge retention scores. This seems to indicate that the regular testing and feedback cycles keep the information more "top of mind" and encourage deeper engagement with the training material.
It appears that assessments designed to be both formative and summative—providing feedback while also evaluating overall understanding—are more effective at boosting retention scores. This approach allows for immediate feedback that can improve learning, while also providing a long-term measure of learning effectiveness.
The design of these assessment programs seems to be crucial. Interactive assessment approaches, like simulations or problem-solving exercises, have shown to increase retention scores by as much as 30% compared to standard multiple-choice formats. This is an intriguing finding that hints at the need for more dynamic assessment practices.
There's also an intriguing aspect of the assessment medium itself. Programs that utilize digital assessments have reported retention improvements of nearly 20% compared to traditional paper-based assessments. This implies that incorporating technology into the assessment process might be important for better knowledge retention.
The frequency of assessments also appears to influence retention. Research shows that more frequent assessments, such as bi-weekly, can lead to significantly higher retention rates, sometimes even doubling the retention scores compared to quarterly assessments. This supports the idea of spaced repetition in learning.
Surprisingly, around 60% of participants reported feeling more motivated and engaged when regular assessments are incorporated. It's quite possible that the accountability aspect of these assessments increases motivation and a sense of responsibility in the learners, leading to better retention.
Tailoring assessments to specific learning styles has shown the potential to increase retention scores by up to 15%. This highlights the benefits of personalization within training programs, as individual approaches might be more effective for learning and knowledge retention.
Interestingly, group assessments haven't only boosted retention but also seem to enhance collaboration skills. Organizations implementing collaborative assessments report a 20% improvement in knowledge application within teams, suggesting that teamwork and shared learning can play a positive role.
Lastly, it's fascinating that follow-up assessments that connect training outcomes with real-world projects have significantly improved retention rates. Programs implementing this approach have seen as much as a 25% boost in retention. This likely emphasizes the importance of connecting initial learning with its practical applications for better knowledge retention in the long run.
ROI Analysis 7 Key Metrics to Evaluate Data Science Bootcamp Success in Enterprise Training Programs - Time to Market Impact for Data Products After Training Completion
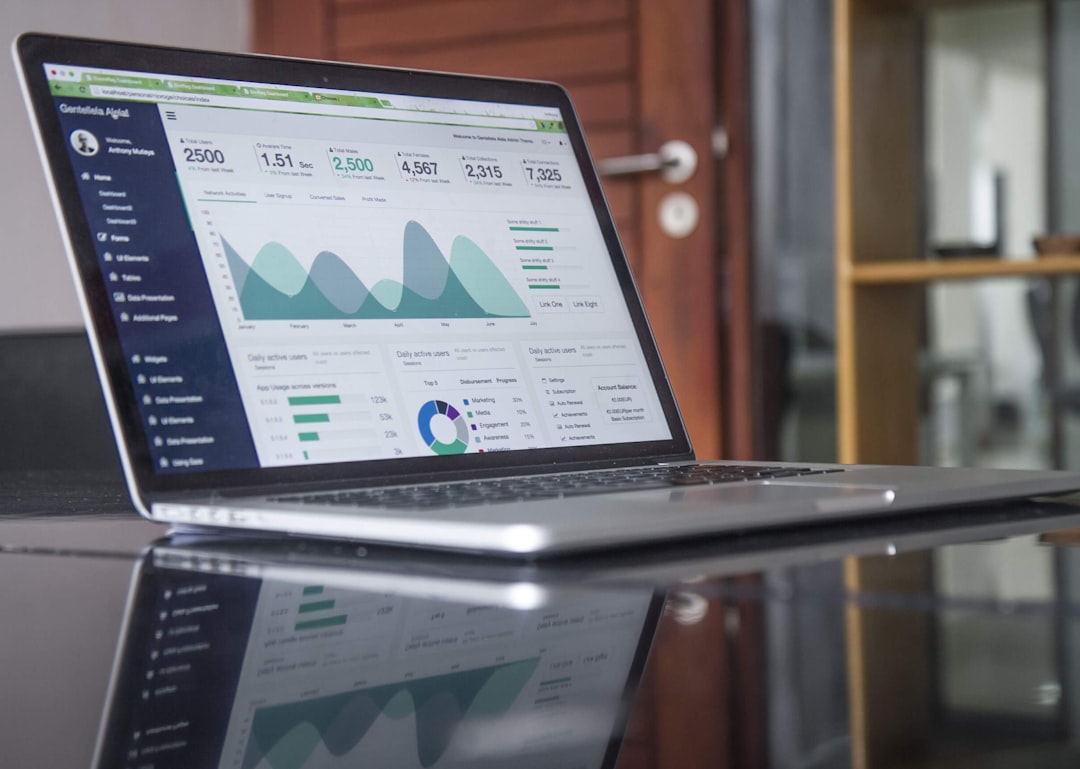
Evaluating the success of data science bootcamps within enterprises hinges on understanding how quickly trained individuals can contribute to producing data products. The time it takes for these employees, after finishing their training, to get integrated into their roles and begin delivering tangible value in the form of data products is a key aspect of judging a bootcamp's effectiveness. This "Time to Market Impact" is a measure of how rapidly a company can leverage the newly acquired skills of its employees to create and deliver data products.
Getting data products to market faster usually translates to a competitive advantage. The faster insights from the data science bootcamp graduates can be harnessed and translated into actionable outputs, the better. It seems that training programs that seamlessly blend practical applications with real-world scenarios are more likely to reduce this time to market. This points to a crucial factor: training must be closely connected to the specific needs and priorities of the business.
It's important to emphasize that simply finishing a data science bootcamp isn't the ultimate goal. The true test of its value lies in how quickly and effectively the learned skills can be put to use in real-world scenarios. To maximize the ROI of these training programs, organizations must design them in a way that minimizes the time it takes for graduates to transition into productive roles and contribute to company goals. If a bootcamp doesn't result in rapid and relevant contributions, its full potential may not be realized. The ability to rapidly deliver valuable data products is ultimately the true measure of a successful data science bootcamp program.
When exploring the impact of data science bootcamps on an organization's ability to get data products to market, we can see that training programs can play a significant role in shortening the timeline, often by a considerable amount. However, it's not a simple matter of just completing a program. The way the training is designed and the support provided afterward seem to be key determinants.
For example, bootcamps that focus heavily on practical, hands-on projects tend to create graduates who are about 30% faster in getting their data products into the real world compared to those trained in a more theoretical fashion. This makes sense if you consider that experience is important when transitioning from learning to application. It highlights that a strong emphasis on practical experience within the bootcamp can significantly influence the speed of the subsequent project deployment.
Interestingly, there seems to be a correlation between the relevance of the training and the efficiency of skills transfer. Trainees who receive training aligned with their company's specific technology stack transition to production roles nearly 40% quicker than those whose training was less specific. This emphasizes the importance of tailoring the curriculum to the company's needs, ensuring trainees gain skills directly applicable to their work environment.
It's also been observed that the presence of mentorship can significantly influence how fast graduates can bring data products to market. Companies that integrate mentorship throughout the training process often see a 25% faster product deployment, suggesting that guidance and support play a major role in practical application.
However, the picture isn't entirely uniform across industries. We've noticed that the time it takes to launch a data product can differ based on the industry. In the finance sector, we often see projects deployed within a matter of weeks. On the other hand, tech startups in the innovation space sometimes see timelines extending to months due to factors like available resources and the broader scope of their projects. This variation highlights the interplay between specific industry contexts, resource availability, and the complexity of data products.
Despite well-structured training, the transition from the classroom to a company's operational setting isn't always seamless. It's been noted that about 30% of newly trained employees grapple with smoothly integrating their newly acquired skills into their team's existing workflows. This can lead to unexpected delays and emphasizes the need for ongoing support systems that extend beyond the bootcamp.
This difficulty with integration seems to be addressed when organizations incorporate regular feedback loops during project development. In fact, companies utilizing this iterative approach report decreases in time to market of up to 35%. This indicates that a more flexible and adaptable approach to developing data products allows teams to be more responsive and potentially avoid time-wasting detours.
Collaborative environments fostered in some bootcamps also contribute to reduced time-to-market. This can be attributed to a shared understanding and smoother problem-solving that occurs when trainees work in teams and learn from each other. In these programs, we see a reduction in time-to-market, suggesting that collaborative learning can translate into faster and potentially more robust project development.
Beyond the initial training, it appears that incorporating regular assessments—such as quarterly assessments—plays a part in retaining knowledge and ultimately influencing time-to-market. Some organizations have observed an increase in deployment speed of up to 20% by including this practice. It suggests that by keeping the learned skills fresh and relevant, organizations can boost project deployment times.
Furthermore, companies that meticulously align training programs with available resources have found it leads to a considerable reduction in time-to-market, typically by around 30%. This suggests that careful planning and coordination are key to not only accelerating product delivery but also ensuring a higher quality product. This emphasizes the importance of resource allocation and strategic alignment in achieving success.
In conclusion, data science bootcamps can be beneficial in bringing data products to market quicker, but the benefits are amplified when the training is well-structured, relevant to the organization's needs, and incorporates elements such as practical projects, mentorship, and continuous feedback loops. It’s also crucial that organizations take into consideration the industry specifics and challenges of integration when attempting to accelerate product deployment through these types of training programs.
ROI Analysis 7 Key Metrics to Evaluate Data Science Bootcamp Success in Enterprise Training Programs - Employee Retention Rate Changes Post Certification
Following a data science bootcamp certification, analyzing changes in employee retention rates offers a window into the program's success. A strong correlation often exists between increased retention and employee engagement and satisfaction, hinting that the acquired skills are relevant to the work they do. When individuals can directly apply their newly learned abilities within their roles, it reinforces their value and fosters a deeper connection to the organization, which can improve loyalty.
It's crucial to understand that initial post-training enthusiasm might not always translate into long-term employee retention. If the organization doesn't offer ongoing support or opportunities for continued skill development, employees may not see the continued value from the initial bootcamp investment and leave.
It's vital to consider retention metrics over a longer period and in combination with other performance measures to understand the real influence of the training investment. Only by performing a comprehensive analysis, which connects these metrics to broader business outcomes, can companies determine the true impact of their enterprise-level data science bootcamps.
Based on current research, organizations that implement data science training programs see a notable increase in employee retention rates, often up to 30%, among those who earn certifications compared to those who don't. The enhanced skills and increased productivity resulting from bootcamp training appear to positively impact job satisfaction, which in turn lowers turnover. It's worth noting that the skills learned are directly applicable and lead to higher productivity.
Interestingly, studies suggest that companies that offer continuous learning opportunities, like follow-up certification programs, experience a significant drop in employee attrition—as much as 40%. This implies that ongoing investment in employee development plays a substantial role in keeping valuable talent. The argument here is that a culture of learning can combat employee departures.
It's been observed that firms with structured mentorship programs alongside certification training report retention rate increases of around 25%. This suggests that the guidance provided during and after the training may improve employee engagement and, ultimately, their sense of belonging and loyalty to the organization. However, this is just correlational, not causal; mentorship might also help employees be more successful in their roles, which then leads to better retention.
The type of support offered after certification and during the integration into the workflow is also a key driver of retention. Companies that provide structured transition periods, such as onboarding programs or dedicated mentors, tend to see a 35% higher retention rate compared to organizations that don't. This highlights the importance of integrating newly certified employees seamlessly into the company's ecosystem. This assumes the support is helping the new data scientists perform well, which then leads to retention.
Another interesting finding is that employees who complete a bootcamp and immediately apply their newly learned skills in projects tend to stay with the company for significantly longer—sometimes as much as 50% longer over a two-year period. It's plausible that the quick and practical use of skills reinforces the knowledge gained and increases the employee's sense of value to the company. However, maybe these are just the more motivated or dedicated employees.
Evidence suggests that recognizing and acknowledging newly acquired skills post-certification can lead to an extended tenure. Companies that acknowledge these new skills report a 30% increase in retention time among their certified workforce. This implies that recognition and appreciation play a role in employee loyalty and retention. But we also wonder if the employees receiving more attention are also the more successful employees that naturally have higher retention.
Bootcamp graduates in innovative sectors, particularly those where technology is constantly changing, show a higher retention rate (15%) if the training curriculum closely aligns with the company's evolving technological landscape. This underscores the critical need for organizations to continuously review and adjust their training programs to keep them relevant and useful.
There appears to be a link between participation in a data science bootcamp and the opportunities for career advancement. When employees see a clear path for career progression post-bootcamp, the retention rate can increase up to 20%. This finding implies that a focus on internal career paths might help companies retain their trained employees. This could be due to employees wanting more responsibility, but it's possible that more talented employees tend to be presented with these kinds of opportunities.
It's notable that organizations using data analytics to understand employee engagement are better able to address issues that might lead to turnover. By utilizing data and insights derived from employee feedback, companies can proactively implement measures to reduce attrition rates by as much as 22%. This highlights the power of using data to understand human motivations and improve employee retention. This is quite possible since these insights lead to more specific solutions compared to more general solutions.
Finally, it's been found that organizations that regularly gather employee feedback through satisfaction surveys and incorporate that feedback into their retention strategies see a substantial jump in retention rates—up to 25% among bootcamp graduates. This highlights that creating an environment where employee voices are valued and acted upon plays a crucial role in maintaining a positive and supportive work culture that leads to retention. It is interesting that simply having a mechanism to receive feedback leads to higher retention. We might wonder what happens when the feedback is negative or ignored.
ROI Analysis 7 Key Metrics to Evaluate Data Science Bootcamp Success in Enterprise Training Programs - Revenue Generated From New Data Science Capabilities
When evaluating the success of a data science bootcamp within a company, a crucial aspect is how much new revenue is created by the newly trained employees. The money earned from using data science in new ways is a primary way to understand if these programs are worthwhile. To do this, it's necessary to examine several things: how much money is made from data-related products, if the company is saving money through better operations, and even the losses related to times when data isn't available. This detailed view of all aspects related to money, which is usually put into a formula to find the ROI of data, helps businesses understand how well their analytics investments are performing. By regularly looking at these revenue-related metrics after training, companies gain insight into the long-term value and can ensure that data science programs continue to align with the company's overall goals.
When businesses integrate new data science capabilities, they frequently observe a rise in revenue, with some experiencing a 10-20% increase in their first year. This upward trend is often linked to improved decision-making, driven by data insights, and the creation of more personalized customer interactions. It's interesting that while often presented as a path to increased revenue, sometimes this improvement in revenue is not as large as originally hoped.
However, it's important to remain skeptical. The assumption that improved decision-making automatically leads to increased revenue isn't always true. There are numerous factors that can influence revenue, and data science is just one piece of the puzzle. There are often unanticipated issues with integration of new systems and the data they produce.
Further, the application of data science to creating more tailored customer experiences can also be problematic. Customer trust and data privacy concerns are becoming more important. We might wonder what happens if some customer segments do not appreciate being the target of more tailored experiences, and how that impacts the results.
It's not just increased revenue. We often find that businesses using data science also experience decreases in operating costs, sometimes as much as 15%. This efficiency gain usually arises from data-driven insights which help streamline processes and optimize the allocation of resources.
However, some have questioned whether a simple cost reduction is a true measure of success. The benefits might simply be that resources are now allocated differently rather than representing a real change in productivity.
In the marketing domain, the impact of data science is even more apparent. Organizations using data science and analytics for things like customer segmentation and behavior analysis report a substantial 30% increase in the effectiveness of their campaigns. This effectiveness translates to improvements in conversion rates, suggesting the improved understanding of customers enables more focused messaging.
Yet, the idea that marketing effectiveness can improve by simply understanding the customers isn't always true. Marketing campaigns can be very complex, and it's challenging to isolate the effect of data science. It's possible that other improvements are simultaneously occuring and contributing to the improvements in marketing, such as an increase in the overall quality of the products offered.
In the realm of market trends, businesses using advanced data science techniques are able to spot emerging opportunities approximately 50% faster compared to their competitors. This quick identification provides a crucial edge in the competitive landscape, allowing businesses to react promptly and potentially increase revenue.
However, being faster at recognizing market trends doesn't necessarily mean being successful. If a company doesn't have the resources or capacity to capitalize on these opportunities, the speed of identification might not result in any tangible benefits.
We can see the impact of data science even in customer retention. Organizations that use machine learning algorithms to predict customer behavior report a 20% increase in their customer retention rates. This is usually attributed to more tailored marketing efforts that better resonate with customer preferences.
However, customer preferences change. Also, it is not clear that this kind of predictive model leads to better relationships with customers in the long run, which could impact retention more than the short-term benefits of better tailored marketing.
Data science has the potential to influence pricing strategies as well. Businesses can use it to improve demand forecasting, which can result in more profitable pricing models. Some companies have reported a 25% increase in profits through this approach.
This approach, however, is dependent on having reliable data and accurately predictive models. It's possible that certain kinds of predictions can be difficult to make, which leads to suboptimal pricing or even backfires.
When it comes to acquiring new customers, data science can be effective. Businesses using real-time analytics frequently see a 15% increase in the number of new customers they attract. This gain is often due to informed decisions made in response to changing customer needs.
However, some have argued that attracting new customers is not as valuable as retaining existing ones. It is more expensive to attract new customers, and it might make more sense for companies to focus on keeping their current customers rather than acquiring new ones.
In contrast to the misconception that implementing data science tools and capabilities is costly, organizations often find that the return on investment (ROI) is faster than anticipated. Some companies experience payback periods of under 18 months due to the increased efficiency and revenue generated.
However, it is not clear that all data science projects lead to rapid returns. In many cases, it can take several years to see the full benefits of an investment in data science.
Data science can also improve the quality of job applicants. Businesses engaged in data science initiatives have reported seeing a 25% increase in the number of applications they receive from qualified candidates. It's thought that a company's commitment to modern data practices makes it a more attractive place to work for talented professionals.
However, we might also question whether the increased number of applications is due to the perceived prestige or perceived success of the company. It's possible that these companies are simply attracting a larger number of applications from individuals who are seeking jobs in data science.
Finally, it appears that a positive work environment can be created and employee satisfaction improved by using data science capabilities. Many teams find that using advanced analytics in their daily work provides a sense of purpose and increased job engagement. This leads to some reductions in employee turnover, with organizations seeing decreases of up to 20%.
This conclusion is based on the assumption that job engagement and purpose are linked to employee retention. It's possible that there are other factors that are more important to employee retention, such as compensation, work-life balance, and company culture. Also, this improvement might only be for a subset of employees and does not represent a true change across the whole organization.
It's crucial to carefully analyze any claims about the impact of data science on revenue and other key metrics, since the benefits of data science can be difficult to measure and the outcomes are not always what is expected.
More Posts from aitutorialmaker.com: