Create AI-powered tutorials effortlessly: Learn, teach, and share knowledge with our intuitive platform. (Get started for free)
AI-Powered Tissue Engineering Breakthrough Digital Twin Technology Maps Complex Organ System Interactions in Real-Time
AI-Powered Tissue Engineering Breakthrough Digital Twin Technology Maps Complex Organ System Interactions in Real-Time - Digital Twin System Creates Cellular Atlas From 500 Million Patient Data Points
A new digital twin system has been developed that leverages an extensive dataset—a staggering 500 million patient data points—to generate a detailed cellular atlas. This innovative system is intended to provide a more complete understanding of how complex organ systems interact with each other. The hope is that this cellular atlas can ultimately enhance healthcare by allowing for more precise, personalized treatments.
By combining artificial intelligence with the digital twin framework, scientists are now able to identify hidden connections between genetics, molecular processes, and the onset of diseases. This ability to peer into the intricate workings of human biology may significantly improve the practice of personalized medicine, where treatments are tailored to a patient's unique profile.
Furthermore, this approach offers the capacity for ongoing, real-time monitoring of patient health information. As a patient's condition changes over time, the digital twin evolves alongside it. This dynamic aspect could help healthcare professionals gain a more nuanced perspective on how an individual's health evolves, leading to potentially more effective interventions and outcomes. However, the potential ethical implications of such a massive dataset warrant careful consideration. The system's reliance on a huge amount of sensitive data raises valid questions about privacy and data security that need to be thoughtfully addressed.
It's fascinating how a digital twin system can build a cellular atlas by sifting through a massive dataset of 500 million patient records. This level of detail allows for an incredibly in-depth look at how cells behave and interact, particularly in the context of diseases. We are essentially getting a microscope-level view of the inner workings of our biology.
This approach helps researchers see, in a dynamic way, how different organ systems communicate and influence each other in real-time. This ability to visualize these complex interactions is a game-changer, offering a far greater ability to forecast how specific treatments might affect a patient.
The core of this system is an impressive set of AI algorithms designed to discover intricate patterns and connections hidden within this huge dataset. These are connections that would be nearly impossible to spot through traditional research methods. We're potentially uncovering the language our cells use to interact and communicate in ways never seen before.
Not only are researchers looking at the functional side of tissue engineering, they are also incorporating genetics. This means we can delve deeper into why some individuals are more susceptible to certain diseases due to their unique genetic makeup.
Having the capability to simulate treatments in real-time significantly speeds up the drug discovery and development process. Essentially, it's a way to quickly test the efficacy of a treatment within a digital environment before applying it to patients. We're essentially creating a fast-forward button for drug discovery.
Importantly, the system is built to adapt. It learns from new patient data and updates its models to reflect the newest advancements in cellular biology and treatment efficacy. This continuous improvement is a crucial part of its utility.
One of the strengths of this digital twin approach is its ability to consider the individuality of patients. It can create models that address specific populations, not just averages. This brings us closer to realizing personalized medicine, where therapies are tailored to the unique needs of each patient.
Interestingly, this technology could potentially shrink the size and duration of clinical trials. By making more precise predictions about which individuals will likely respond best to a particular treatment, researchers could focus resources more effectively.
It's critical to remember that using such massive amounts of patient data comes with a responsibility to protect individual privacy. Implementing robust anonymization techniques and ethical protocols are key. Balancing the immense scientific potential with the need for patient confidentiality is a crucial balancing act.
Though powerful, these systems aren't foolproof. Their accuracy and the ability to make meaningful predictions hinges on the quality and completeness of the data used to create them. Inaccurate or incomplete data can lead to misleading conclusions about cell behavior or treatment effectiveness. We need to constantly ensure we're feeding the system reliable and validated information for optimal results.
AI-Powered Tissue Engineering Breakthrough Digital Twin Technology Maps Complex Organ System Interactions in Real-Time - Machine Learning Algorithm Detects Early Tissue Rejection Patterns 24 Hours Before Symptoms
A new machine learning algorithm shows promise in detecting early signs of tissue rejection in kidney transplants, potentially up to 24 hours before patients show any symptoms. This development, tested in a substantial group of kidney transplant recipients, offers the hope of intervening earlier and potentially improving outcomes for patients. Traditional methods of detecting transplant rejection rely on biopsies, which are invasive and whose results can vary depending on who's interpreting them. This new approach, utilizing machine learning tools like convolutional neural networks, aims to provide a less invasive and more precise way to assess transplant health. This could revolutionize how we monitor organ transplants and potentially lead to more personalized patient care. However, the increasing use of AI in these delicate medical fields also raises serious questions about data privacy and the ethical implications of using massive datasets to drive these algorithms, concerns which must be addressed as this technology evolves.
A machine learning algorithm has shown promise in detecting early signs of tissue rejection in kidney transplants, potentially up to 24 hours before a patient exhibits any symptoms. This is quite interesting as it could lead to earlier interventions, improving patient outcomes.
A study involving over 1,200 kidney transplant recipients revealed that the algorithm was effective in identifying subtle shifts in the body's immune response that signal the beginnings of rejection. It's fascinating how the algorithm picks up on these subtle changes, which are often undetectable by conventional methods. It seems to be especially adept at identifying HLA II mismatches, a known risk factor, and also seems to be able to recognize the positive effects of certain pre-transplant treatments.
Interestingly, the algorithm's predictive power appears to be built on logistic regression, which is a statistically-based approach that's used quite a bit in machine learning. Additionally, researchers have explored applying convolutional neural networks (CNNs), a type of deep learning technique often used for image analysis, to the interpretation of kidney tissue biopsies. This suggests that AI is making inroads into the way we visually assess transplant health.
Currently, the gold standard for tissue rejection is a kidney biopsy. However, the process is invasive, and it depends on a human interpretation of the tissue which can introduce some variability in diagnoses. There is increasing interest in using digital pathology tools combined with AI to create more consistent and reliable assessments.
It is encouraging that machine learning is being increasingly used in the broader field of tissue engineering. There's a growing body of research that explores its applications, including technologies like surface-enhanced Raman spectroscopy (SERS), a sensitive method for examining the biomolecules associated with rejection. The hope is that this can provide more precise information about tissue health and aid in the development of new diagnostic approaches.
Of course, it's worth noting that incorporating this new technology is a process. There are concerns regarding algorithm transparency, particularly regarding how the algorithms are able to make such precise predictions. It's crucial for both doctors and patients to be confident in the methods used. It's not just about transplant rejection; these algorithms may have the capability to aid in diagnosing a wide range of medical conditions by identifying specific early-warning signs, making them a potentially versatile tool in healthcare. And of course, this approach is in line with the burgeoning field of personalized medicine—developing tailored treatments based on individual patient characteristics. But, we need to be thoughtful about how this technology is developed and deployed, ensuring data privacy and safety as we move forward.
AI-Powered Tissue Engineering Breakthrough Digital Twin Technology Maps Complex Organ System Interactions in Real-Time - 3D Organ Mapping Shows Blood Flow Networks During Transplant Procedures
3D organ mapping offers a new way to visualize the intricate network of blood vessels within organs during transplant procedures. This real-time visualization provides surgeons with a more detailed understanding of blood flow, potentially improving surgical planning and precision. This development is a step forward in addressing the challenges faced in transplant surgery, where ensuring proper vascularization is critical for the organ's survival.
Coupled with the progress in AI-powered tissue engineering, 3D organ mapping contributes to the broader effort of creating functional human organs outside the body. Addressing the significant organ shortage is a major focus, and these technologies could play a crucial role in developing solutions.
While these advances offer tremendous potential, they also necessitate careful consideration of ethical concerns. The use of AI and large datasets raises important questions about data privacy and security, which need to be carefully addressed as these technologies mature and are implemented in clinical settings.
Researchers have integrated 3D organ mapping into transplant procedures, allowing surgeons to get a real-time view of the intricate blood vessel networks within the transplanted organ. This visual feedback offers a significant advantage during surgery, as it helps optimize organ placement and potentially identify issues with blood flow before they become critical.
Interestingly, the ability to see how blood moves through a transplanted organ in 3D during surgery may be a game-changer for graft survival rates. Surgeons are now able to use this real-time blood flow information to make decisions that can positively impact how well the new organ functions.
This 3D mapping capability relies on advanced imaging techniques like MRI and CT angiography. These methods create detailed 3D models of the organ's blood vessels, offering a level of anatomical understanding that traditional methods couldn't provide. It's like having a detailed map of the organ's circulatory system during the operation, which is invaluable.
Beyond visualization during surgery, this technology provides an excellent tool for studying how the organ responds to changes in blood pressure or volume. This is critical as blood flow is tightly linked to the health of tissue. The insights gained from mapping the blood flow under various conditions can help us better understand how to maintain the viability of transplanted organs, which is crucial for successful transplantation.
One of the potentially practical outcomes of 3D organ mapping is the reduction in the time needed for transplant procedures. Shorter surgery times can lead to decreased risks to the patient and potentially improve overall patient outcomes, which is certainly a desired goal.
There's been some fascinating research showcasing how 3D mapping can identify unexpected problems with the organ's blood vessels. By seeing these issues ahead of time, surgeons can take steps during the procedure to address them, reducing complications linked to poor blood supply.
Another interesting aspect of this technology is the ability to create digital models that simulate different surgical scenarios. Surgeons can use these models to test the impact of different surgical techniques on the organ's blood flow, allowing them to explore optimal approaches before actually performing the operation.
3D organ mapping can also play a role in optimizing organ storage and transportation protocols. By studying how preservation methods affect the blood flow in donor organs, we can develop better practices to ensure organs arrive in the best possible condition for transplantation.
Since each person's blood flow networks are unique, 3D mapping may help researchers develop personalized surgical approaches that are optimized for the individual recipient. This customization could be a big leap forward for transplantation.
While the potential benefits are clear, there are limitations. The quality of the 3D maps is dependent on the quality of the imaging data. Generating truly useful 3D models requires high-quality imaging, and the integration of this data into the surgical workflow requires further development. The accuracy of the 3D mapping directly impacts the reliability of the insights that are derived from it.
AI-Powered Tissue Engineering Breakthrough Digital Twin Technology Maps Complex Organ System Interactions in Real-Time - Neural Networks Track 200 Cell Types Simultaneously Through Advanced Microscopy
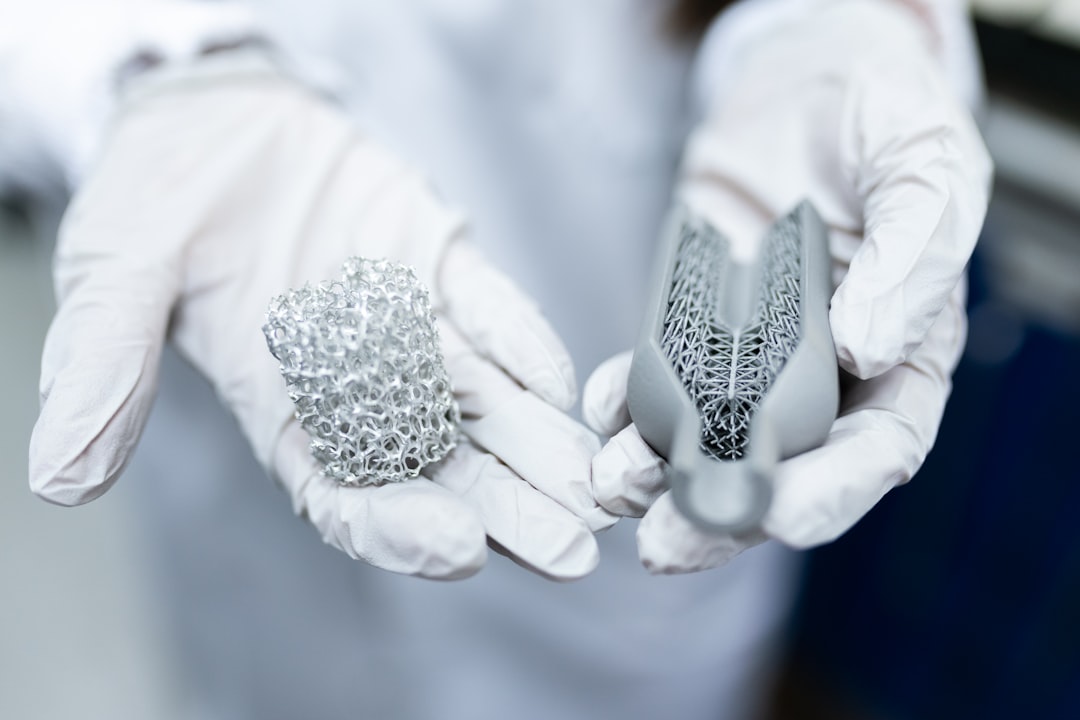
Neural networks are now capable of simultaneously tracking over 200 different cell types using advanced microscopy methods. This capability stems from the development of sophisticated AI algorithms that can analyze complex images and differentiate between various cell types. For instance, neural networks are now being used to classify cells based on the spatial distribution of proteins within cells, providing a far more detailed view of cellular behavior than previously possible. These algorithms are integrated with advanced imaging techniques such as two-photon microscopy, which allows researchers to study live cells within complex tissues, including the brain, in real-time. This advancement has implications for understanding how various cell types interact within complex environments and how these interactions might influence health and disease. However, it is important to acknowledge the dependence on high-quality datasets. Without accurate and diverse data, the ability of these AI algorithms to make reliable inferences about cell behavior may be compromised, emphasizing the need for robust data collection and validation in this field.
Researchers are now employing neural networks in conjunction with advanced microscopy techniques to simultaneously track over 200 different cell types within complex tissues. This level of detail is groundbreaking, allowing us to see cellular interactions in ways never before possible. It relies on high-resolution imaging methods like light sheet fluorescence microscopy, which lets us view entire tissues in 3D while distinguishing individual cells based on their unique optical characteristics.
The sheer volume of data generated by these advanced microscopy techniques requires incredibly fast processing capabilities, which neural networks provide. They can analyze these massive datasets incredibly quickly, potentially reducing the time it takes to achieve significant findings from days to a matter of hours. This speed increase alone is quite valuable.
One of the most compelling features of this method is the ability to observe cellular behavior dynamically, in real-time. This lets us explore processes like tissue regeneration or disease progression with an unprecedented level of detail. Previously, many of these biological events could only be studied retrospectively, which limits the depth of understanding we can obtain.
By meticulously mapping and quantifying how different cell types interact, we can create more accurate models of disease states. This ability to visually distinguish between healthy and diseased tissue is crucial for designing targeted therapies that address the root causes of disease, rather than just treating symptoms.
Furthermore, being able to track multiple cell types in parallel enhances our understanding of immune responses. This deeper insight into immune system dynamics has the potential to advance the treatment of autoimmune diseases. Additionally, it may lead to improvements in transplant success rates by providing more precise measures of organ compatibility.
However, there are certain challenges that come along with using neural networks for interpreting imaging data. We must grapple with concerns about the accuracy and interpretability of the algorithms. If the networks make mistakes in identifying cell types, the downstream analyses could be compromised, highlighting the need for careful validation.
Moreover, the quality of the neural networks depends heavily on the training datasets used. The networks need to be trained with high-quality, diverse data so they can accurately generalize across different biological contexts. This emphasis on data quality is a crucial aspect of the technology.
This combination of advanced imaging and neural networks may also give rise to better "organ-on-a-chip" models. These models can mimic human tissue responses under various conditions without relying on animal models. This could have significant implications for refining biomedical research methods.
In the long term, this technology could revolutionize how we approach drug discovery. It could provide a means to rapidly assess the effects of new compounds on specific cell types within a tissue context. This accelerated testing process could streamline the transition from lab discovery to clinical trials.
While we are still in the early stages, the potential implications of this technology are remarkable. It holds immense promise in advancing our understanding of complex biological systems and holds the potential to improve human health in numerous ways.
AI-Powered Tissue Engineering Breakthrough Digital Twin Technology Maps Complex Organ System Interactions in Real-Time - Real Time Tissue Analysis Links Microbiome Changes to Organ Function
Researchers are making strides in understanding how the microbiome impacts organ function through real-time tissue analysis. By using engineered tissues that can mimic the behavior of real organs, scientists can now study how changes in the makeup of the microbiome affect organ activity. This capability, combined with AI-powered analysis of tissue samples, is leading to better ways to diagnose and treat diseases. It's becoming increasingly clear that the balance of our internal microbial ecosystems is a critical factor in our overall health. While the potential benefits of this research are significant, the ethical implications, particularly those relating to the vast amounts of data generated and the privacy of patients, must be carefully considered as these technologies develop. This area of study also raises questions about how our understanding of the microbiome can improve the development of new treatments and preventative strategies.
Recent work using real-time tissue analysis is revealing a fascinating link between changes in the microbiome—the collection of microorganisms living in and on our bodies—and the function of our organs. It appears that shifts in the composition of these microbial communities can have a direct impact on how organs work, sometimes triggering inflammatory responses that ultimately affect organ health.
This real-time approach is particularly exciting because it creates a kind of feedback loop. Researchers can immediately observe changes in the microbiome and, almost instantly, see how those changes influence organ function. This speed of response allows clinicians to rapidly adapt treatment plans to match a patient's immediate needs and could potentially lead to more precise and effective care. However, the complexity of this relationship, with countless microbial interactions and varying responses from different organs, makes it a considerable challenge to fully understand.
Interestingly, a more diverse microbiome seems to be associated with better overall health, hinting that maintaining a balanced microbial environment could be protective against various diseases. This discovery adds another dimension to personalized medicine, where the focus now extends to tailoring treatments based on the unique makeup of each individual's microbiome. Of course, more research is needed to validate this relationship in more robust studies.
Further, the microbiome seems to be heavily involved in how our bodies process medications. This means that the effectiveness of certain drugs can differ from person to person due to the unique composition of their microbiome. If we can better understand how these microbial communities interact with various drugs, it could lead to more precise dosing and improved treatment outcomes.
Unfortunately, the same mechanisms that influence drug responses can also be problematic. For instance, the disruption of microbial balance caused by broad-spectrum antibiotics may be linked to some complications in organ function. These kinds of findings underscore the importance of taking a more thoughtful approach to the use of antibiotics, recognizing the potential unintended consequences.
Furthermore, this technology opens up new avenues for predicting health complications, particularly concerning organ transplants. By analyzing microbiome shifts, it may be possible to foresee organ rejection—a major complication—much sooner than traditional methods. An earlier warning system would allow for more timely interventions, potentially enhancing the success rate of transplant surgeries. It's still early days, but the potential to intervene and save lives is huge.
The link between the microbiome and organ function also opens doors to understanding the role of nutrition. Our diets can significantly impact the composition of our microbiomes, so it's possible that certain foods or dietary patterns can strengthen or improve organ function. This fascinating concept may lead to novel strategies for preventative healthcare, potentially influencing public health in profound ways.
Moreover, we can now utilize AI algorithms to map the microbiome and find specific microbial signatures associated with healthy organ function. This may lead to the development of targeted interventions, allowing for treatment approaches custom-tailored to an individual's unique microbiome and specific organs. However, such personalization requires the development of highly specific and reliable AI algorithms. Otherwise, the risks of inaccuracy and potentially harmful outcomes are increased.
Additionally, each organ seems to have its own unique microbiome, highlighting the intricate, organ-specific nature of microbial communities. This means that treatments may need to be designed not just for the individual patient but also considering the specific microbiome residing in the targeted organ. Understanding these complexities will be vital to realizing the potential of personalized therapies.
Finally, the ability to modulate the microbiome through specific interventions may provide a proactive approach to preventing organ dysfunction. This burgeoning area of research suggests exciting potential for both organ transplantation and managing various chronic conditions. However, more careful study is needed to ensure the long-term safety and effectiveness of such interventions.
AI-Powered Tissue Engineering Breakthrough Digital Twin Technology Maps Complex Organ System Interactions in Real-Time - Quantum Computing Integration Processes Tissue Engineering Data At 100x Speed
The integration of quantum computing into tissue engineering processes is introducing a new level of speed and efficiency in data analysis. It's claimed that these systems can process tissue engineering data up to 100 times faster than conventional computing, allowing researchers to analyze complex datasets in real-time. This accelerates the development of models and simulations crucial for fostering tissue regeneration, which is vital for advancing personalized medicine and regenerative therapies. The ability to work with data in real-time is crucial for understanding how our bodies' intricate systems interact and how to tailor treatments accordingly. However, with this advancement come some caveats. Ensuring the quality and the meaning of the data and results from quantum computers remains a challenge that requires ongoing investigation. We must proceed with care, developing a deeper understanding of these quantum processes to ensure we maximize their benefits responsibly.
The prospect of quantum computing accelerating tissue engineering processes by up to 100 times is quite intriguing. It's promising to think that real-time analysis and adjustments during experiments might become commonplace, leading to quicker feedback loops and a more efficient research cycle.
Integrating vast datasets from various tissue models using quantum algorithms could provide us with a significantly improved understanding of the interactions that happen within and between tissues. The speed with which quantum systems can sift through this data could potentially accelerate the development of truly personalized treatments.
The inherent ability of quantum computing to perform parallel processing is an attractive feature. Imagine being able to simultaneously analyze multiple cellular interactions within a tissue. That's a game changer for modeling complex biological systems, allowing for faster, more comprehensive evaluations.
It's interesting to note that quantum computers, due to their specific designs, are intrinsically better at handling computational errors than traditional computers. This is quite important in tissue engineering since even tiny errors in calculations can lead to flawed representations of cellular behavior. So, this inherent robustness could be beneficial.
The possibility of using quantum computing for simulations at the molecular level is also exciting. This could give us much more accurate depictions of biological processes, potentially unlocking discoveries that classical computers might not be able to find.
It's fascinating to see the convergence of fields that quantum computing brings to tissue engineering. It requires collaborations across disciplines like computer science, biology, and engineering. This interdisciplinary nature highlights the complexity of the biomedical challenges we're attempting to solve.
The prospect of speeding up drug design using quantum computing is appealing. If we could rapidly simulate the interaction of new drugs with cellular targets, it could bridge the gap between laboratory discoveries and real-world patient application much faster.
I also wonder how it might improve the quality and fidelity of tissue models. Perhaps quantum computers could create much more detailed representations that incorporate things like timed responses and molecular pathways, offering a deeper understanding of disease mechanisms.
The rapid processing power could potentially allow us to model causality in experiments. Being able to observe the potential outcomes of cell interactions in a simulation before actually performing the experiment in a lab is something to think about.
Looking forward, the scalability of quantum systems is something to keep an eye on. As the technology evolves, the ability to analyze increasingly larger datasets could be revolutionary not only for tissue engineering, but also for a wide variety of scientific fields relying on large and complex datasets. This expanded research potential is very appealing.
While there are still many challenges, these are early but very exciting times for quantum computing and its application to tissue engineering. It's possible this could provide profound advancements for regenerative medicine and the treatment of a variety of human diseases.
Create AI-powered tutorials effortlessly: Learn, teach, and share knowledge with our intuitive platform. (Get started for free)
More Posts from aitutorialmaker.com: