Create AI-powered tutorials effortlessly: Learn, teach, and share knowledge with our intuitive platform. (Get started for free)
AI-Powered Tool Revolutionizes Learning Material Generation A Deep Dive into Efficiency and Personalization
AI-Powered Tool Revolutionizes Learning Material Generation A Deep Dive into Efficiency and Personalization - AI algorithms analyze learning patterns for tailored content creation
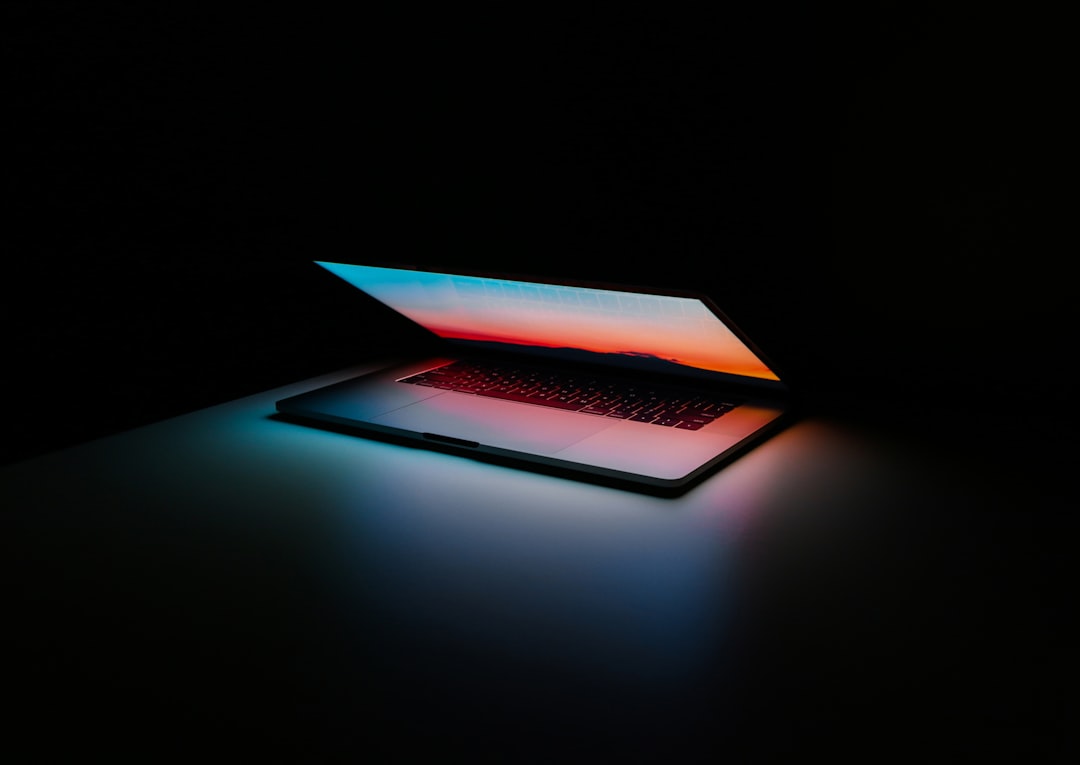
Artificial intelligence algorithms are revolutionizing the creation of learning materials by scrutinizing individual learning patterns. This includes analyzing things like how well a student does on assignments and what they seem to prefer. This data-driven method centers the student's experience, allowing educational content to adapt to a wide range of needs. A goal of this approach is to curb the common issue of students losing interest in their learning. AI's ability to use machine learning lets it create and gather interactive learning resources, enriching the learner's experience and making the materials more flexible. These advanced algorithms also help to ensure a constant stream of relevant materials, making education not just personalized but also adaptable to how students' needs change over time. Despite this promise, relying on AI for generating content does bring up concerns about how deep and high-quality the learning interaction is. As AI's role in education grows, careful consideration of these aspects is necessary.
At the core of this AI-powered revolution in learning material creation lies the ability of algorithms to meticulously analyze how learners interact with content. By scrutinizing patterns in user behavior, such as the pace of learning, areas of struggle, and even emotional responses, these AI systems can create a dynamic and personalized learning experience.
It's intriguing how these algorithms can discern individual preferences, adjusting content delivery in real-time to match a learner's unique style. While the potential of tailored content to enhance learning outcomes is significant, it's crucial to acknowledge that these benefits are often based on correlations rather than causal relationships.
Furthermore, AI's capacity to interpret emotional cues embedded in user responses allows it to adapt the content not just in terms of complexity but also in emotional tone. This is a fascinating avenue, as it potentially allows for content that is not just informative, but also motivational and engaging. However, it's vital that this functionality is carefully designed to prevent potential biases or manipulative effects.
The potential for AI to reduce the time needed for learners to achieve mastery is also a compelling area of research. It's quite remarkable how machine learning algorithms can tailor the learning pace to each individual, potentially accelerating the learning process. Yet, there's a concern about this approach possibly creating a pressure to learn faster, which may not always be conducive to deeper understanding and knowledge retention.
Another interesting development is the capacity of AI to analyze demographic trends within learner interactions. This opens a pathway to tailoring content in ways that resonate better with different cultural contexts, ensuring that educational material feels relevant and engaging for a wider spectrum of students. However, this is a double-edged sword; we need to guard against inadvertently amplifying existing social biases through biased data sets or unintended consequences in the AI-generated content.
It's also noteworthy that these AI systems are designed to constantly learn and refine their understanding of effective educational approaches. This continuous feedback loop, while potentially powerful, also raises questions regarding the evolution of the algorithms themselves and how human educators can retain agency and control over the learning experience.
A rather surprising finding is the influence of gamified learning on learner engagement. AI tools are now incorporating game-like elements into content, based on the insights extracted from user data. Yet, it is crucial to strike a balance between creating a motivating and engaging learning experience and possibly reinforcing an overly entertainment-focused approach to education.
Beyond the immediate benefit of personalization, the use of AI in education presents a unique opportunity for uncovering hidden patterns in learner behavior. The insights gleaned from analyzing these patterns can lead to valuable insights about instructional techniques that are applicable across various subjects. However, this process should always respect individual privacy and data security.
Furthermore, these algorithms can be incredibly useful in identifying potential challenges ahead of time, potentially mitigating student frustration and dropout rates. Anticipating difficulty allows educators and AI systems to offer support before a learner gets discouraged. However, this prediction capacity shouldn't be applied to label or stigmatize particular types of learners, or be used for social engineering.
In conclusion, while the potential of AI-powered educational tools to personalize learning and optimize outcomes is undeniably exciting, it's crucial to navigate this technological advancement with a critical and thoughtful eye. We need to be aware of the potential for biases and unintended consequences, and prioritize ethical considerations as we develop and deploy these increasingly sophisticated tools.
AI-Powered Tool Revolutionizes Learning Material Generation A Deep Dive into Efficiency and Personalization - Real-time feedback systems enhance student engagement
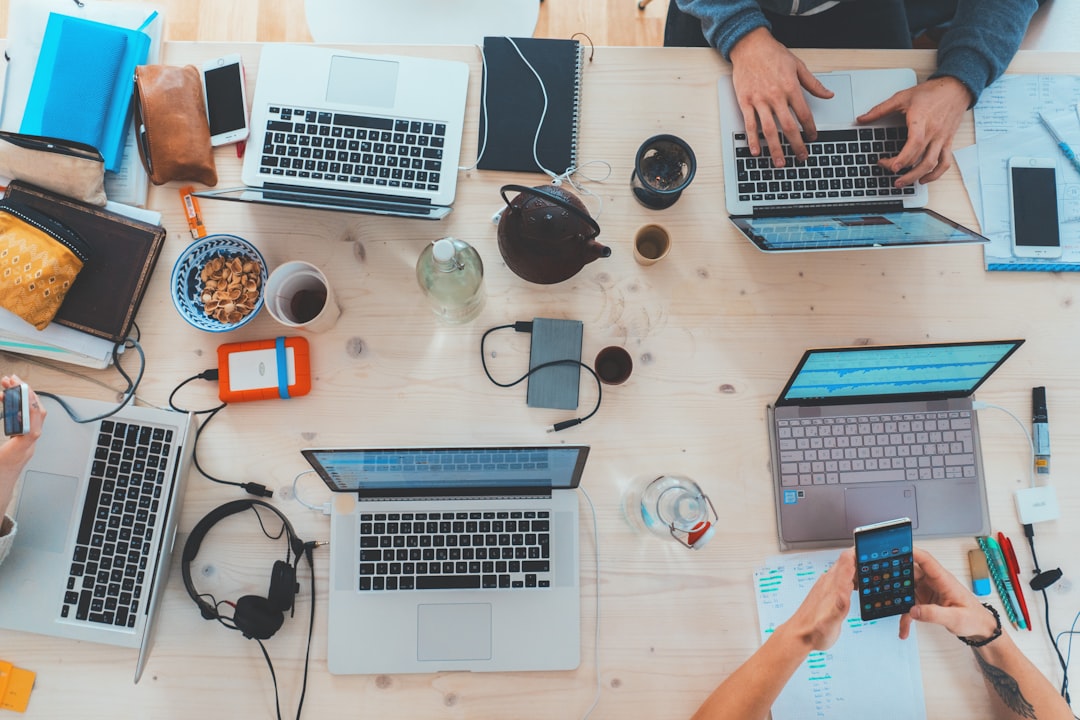
Real-time feedback systems are increasingly important in boosting student engagement. They offer immediate insights into student performance, allowing teachers to adjust their teaching approaches to match the specific needs of each student. These AI-powered systems enable continuous tracking of a student's progress, facilitating personalized learning pathways that adapt to individual learning styles and speeds. By recognizing areas where a student might struggle and giving customized guidance, these systems can offer timely assistance, making sure students have the help they need to succeed. Moreover, AI's ability to design interactive and captivating learning environments can motivate students and deepen their engagement with the subject matter, ultimately leading to better learning results. However, it's crucial to acknowledge that over-dependence on these technologies could be problematic and potentially harm the quality of learning interactions. We need to be mindful of these risks as AI evolves in the educational landscape.
Real-time feedback mechanisms, powered by AI, are increasingly being recognized for their potential to boost student engagement. By providing immediate insights into students' performance, these systems empower instructors to tailor their teaching methods to individual needs. This continuous feedback loop, facilitated by AI tools, fosters a more adaptive and personalized learning experience, potentially aligning with each student's unique learning pace and style.
Research from Stanford University indicates that AI's ability to provide instant feedback during classroom activities can significantly improve interactions between students and teachers. The algorithms behind these systems can pinpoint areas where a student is struggling and offer customized recommendations for support, ensuring timely interventions. These AI-driven feedback mechanisms are designed to create customized learning paths, utilizing adaptive assessment tools and personalized learning algorithms, ultimately aiming to keep students more actively engaged in the learning process.
AI-powered tutoring systems represent another intriguing application of real-time feedback. These systems can adapt their instructional approach based on each student's specific strengths, weaknesses, and preferred learning styles, potentially transforming the way learning outcomes are achieved. Flipped classroom models can also benefit from integrating AI technologies, encouraging active learning and further individualizing the educational experience.
Furthermore, real-time feedback promotes continuous assessment, enabling students to stay on track and receive ongoing guidance. AI's capacity to create interactive learning environments has the potential to immerse students in their learning, sparking increased motivation and participation. However, it is worth considering whether an over-reliance on this immediate feedback can potentially lead to a superficial approach to learning, with students becoming overly focused on short-term results rather than developing a deeper understanding of the material.
Despite this potential pitfall, AI technologies clearly offer a multitude of opportunities to redefine education by improving feedback loops and enhancing the overall effectiveness of instruction. But as with any rapidly evolving technology, there is a need for ongoing research and careful consideration to ensure that these new approaches benefit learners, rather than potentially exacerbating existing inequalities or prioritizing superficial learning over true comprehension. The potential for bias in AI algorithms and the need for maintaining human control over the learning process are points to continuously be mindful of in this developing field.
AI-Powered Tool Revolutionizes Learning Material Generation A Deep Dive into Efficiency and Personalization - Adaptive difficulty levels respond to individual progress
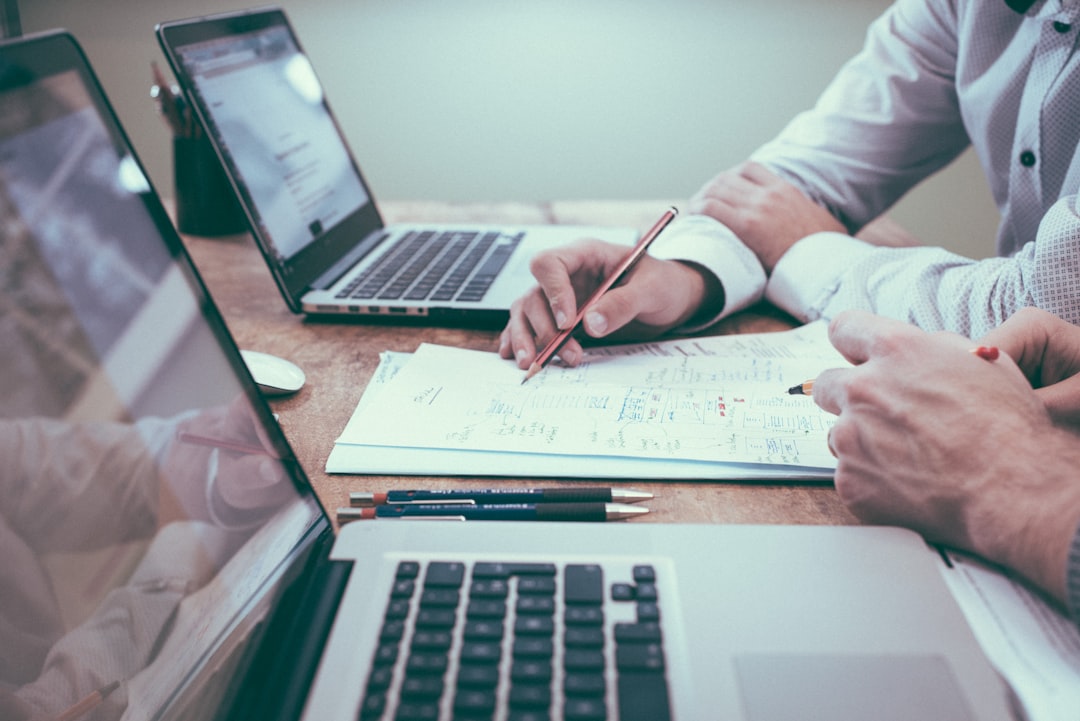
AI is increasingly allowing learning materials to adjust their difficulty in real-time based on how well a student is doing. This means content can be tailored to each person, providing a challenge that's just right for their current level and pace. These adaptive difficulty levels, powered by AI that tracks performance and engagement, create personalized learning paths designed to meet individual needs. This approach can boost motivation and lead to a deeper understanding of the material. But, there's a risk that overly relying on this kind of adaptive system could simplify complex subjects too much. There's also a concern that students who need more specific and individualized support may be overlooked. It's crucial that we consider carefully how these innovations affect teaching and learning as they continue to develop.
Adaptive difficulty levels are a fascinating aspect of AI-powered learning tools. They dynamically adjust the complexity of the content in real-time, keeping learners engaged and challenged without overwhelming them. This constant adjustment creates a learning environment where the content's difficulty seamlessly adapts to the learner's growing abilities.
AI systems achieve this by analyzing a wealth of data about the learner's performance. It's not just about whether they get answers right or wrong, but also factors like how engaged they are, how long they take to answer, and other behavioral patterns. This fine-grained analysis lets the system provide a learning experience truly tailored to the learner's present state.
This dynamic adjustment ties into the idea of cognitive load. Learners can get lost in complexity, especially if the presented material is far too challenging. Adaptive difficulty uses this principle to strategically lessen or amplify the demand placed on the learner. This dynamic interplay can enhance how much they retain and make them less likely to get frustrated.
Furthermore, machine learning algorithms within these systems can detect subtle changes in student behavior. Maybe their engagement drops or they keep making the same kind of mistake. These systems can then adjust teaching methods and the materials themselves to address those specific issues, ultimately making learning more efficient.
Interestingly, these systems can also attempt to predict when a learner might struggle. By analyzing past data, they can somewhat accurately identify potential hurdles before they arise. This predictive ability, however, shouldn't be taken to remove learners' agency or control. It's about providing more support where it's needed, and tailoring that support to each person.
Additionally, AI systems with emotional recognition capabilities can assess students' emotional responses. They can then tweak the difficulty as well as the emotional tone of the learning material to make the learning experience more encouraging and engaging. This presents an interesting question of how AI might create a more supportive learning environment.
Adaptive systems can also create custom learning paths for each learner. As they progress, the path itself evolves. This keeps learners motivated because they're continually making progress and accomplishing goals rather than just stuck at a fixed difficulty level. It makes the learning experience more personal.
The idea of using game-like elements in learning, sometimes called gamification, has been incorporated into some of these AI systems as well. The difficulty levels in these games change based on learner feedback and performance. It's an intriguing development, but it raises a question about the balance between stimulating learning and making education too focused on entertainment.
Ultimately, these adaptive difficulty features align learning with individual goals. Students aren't just completing tasks, but are trying to hit specific targets related to their learning. This emphasizes a more focused effort toward true mastery of a subject.
However, there's a crucial need to make sure that these adaptive systems don't inadvertently perpetuate inequalities. If the data used to train these algorithms isn't well-represented or inclusive, the result could be that these tools worsen existing educational disparities rather than help mitigate them. We must focus on ensuring that these systems are designed to include and cater to all diverse learners.
AI-Powered Tool Revolutionizes Learning Material Generation A Deep Dive into Efficiency and Personalization - Multi-modal content generation expands learning options
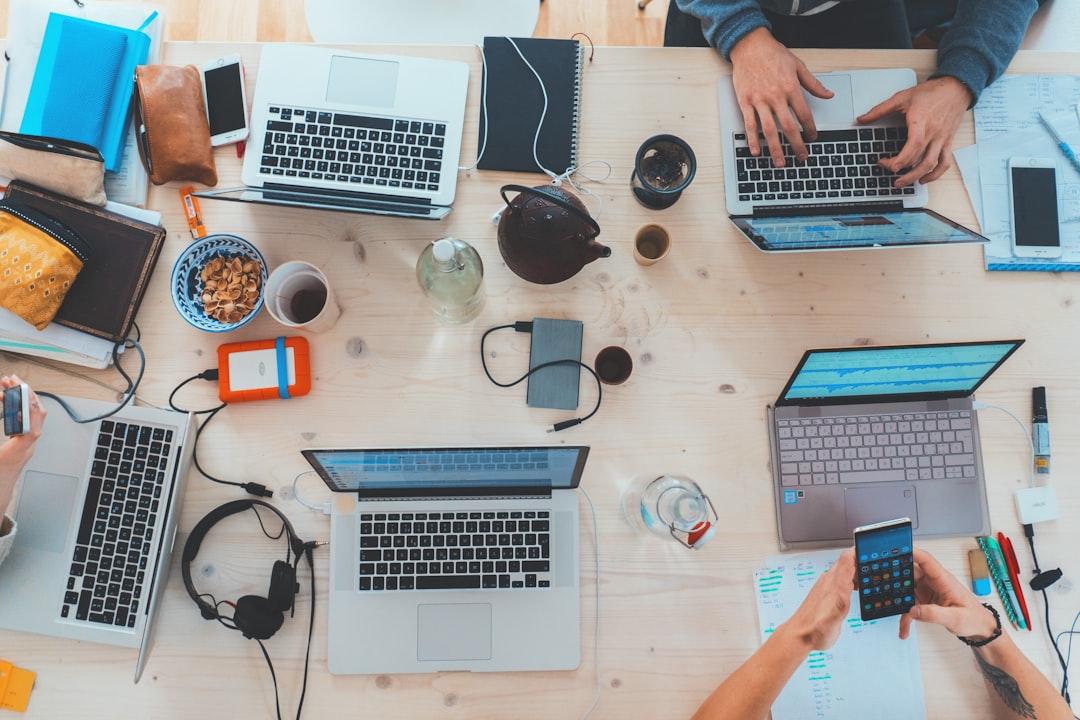
The emergence of multi-modal content generation marks a significant shift in how learning materials are created and consumed. By seamlessly blending different forms of data—like text, visuals, and sound—it allows for a far richer and more engaging educational experience. This approach not only diversifies the ways students can learn but also offers a pathway to a more personalized and flexible education. AI's ability to automate multi-modal content creation is especially valuable in addressing the growing need for constantly updated and relevant learning resources.
However, as AI tools expand into this more complex realm of multi-modal content, concerns regarding the quality and efficiency of the generated materials become increasingly important. There's a risk that the focus on innovation overshadows the crucial need for accuracy and effectiveness in the learning experience. Nonetheless, multi-modal content generation presents a compelling opportunity to create a more accessible and inclusive educational landscape. It can foster a stronger sense of connection between students and the material, but it's critical to be mindful of possible biases or unforeseen negative consequences that might arise from the technology's integration into education. Carefully considering these potential drawbacks is paramount as we embrace the promise of multi-modal learning.
The capacity of AI to generate content across multiple formats, like text, images, and audio, is expanding the ways we can learn. This multi-modal approach acknowledges that people learn differently and aims to cater to a broader spectrum of learning styles. We see this in how it can incorporate diverse content types, which can make learning more engaging. For instance, it's interesting how cognitive load theory suggests that using multiple formats, instead of just one, can make complex concepts easier to grasp.
It's also interesting how these AI systems can look at how someone interacts with various types of educational content to suggest other resources that could help. This adaptive nature of the content, customized to how each student does and what they seem to prefer, can make learning more personalized and potentially even boost engagement. There's also a positive impact on inclusivity. Multi-modal materials mean students who may have certain learning differences, such as visual or auditory challenges, might be able to access information in a way that best works for them. However, this comes with a bit of caution. If not done right, having multiple content types could lead to fragmented learning experiences that can do more to confuse than to help.
Another intriguing area is how this multi-modal approach can help with things like gamification. Game-like features have the potential to boost motivation. But, there's a risk of over-emphasizing this entertainment aspect. The key is finding a good balance between fun and learning. Furthermore, these systems can offer something quite valuable in terms of gathering data on how people learn from different content. This can lead to insights into specific challenges students encounter and provide personalized support to address them before a problem gets bigger. It's fascinating how AI can collect information about how students are feeling during learning using multi-modal data and then modify the tone and style of the content to improve the experience. However, the ethical aspects of using this type of information, particularly concerning privacy, deserve serious consideration.
The ability of AI to scale up the creation of multi-modal learning materials opens up huge possibilities, but it's important to address the challenges that come along with this. Producing a massive volume of diverse materials quickly can be beneficial. Yet, we need to be vigilant in ensuring that the content quality is maintained and is appropriate for what people are trying to learn. It raises the question of how to maintain the educational value while also making the experience as effective as possible. Overall, it seems that multi-modal content generation offers promising new ways to engage students with education. But, it's clear that research and thoughtful development are needed to manage potential downsides and maximize its potential.
AI-Powered Tool Revolutionizes Learning Material Generation A Deep Dive into Efficiency and Personalization - Integration with existing Learning Management Systems
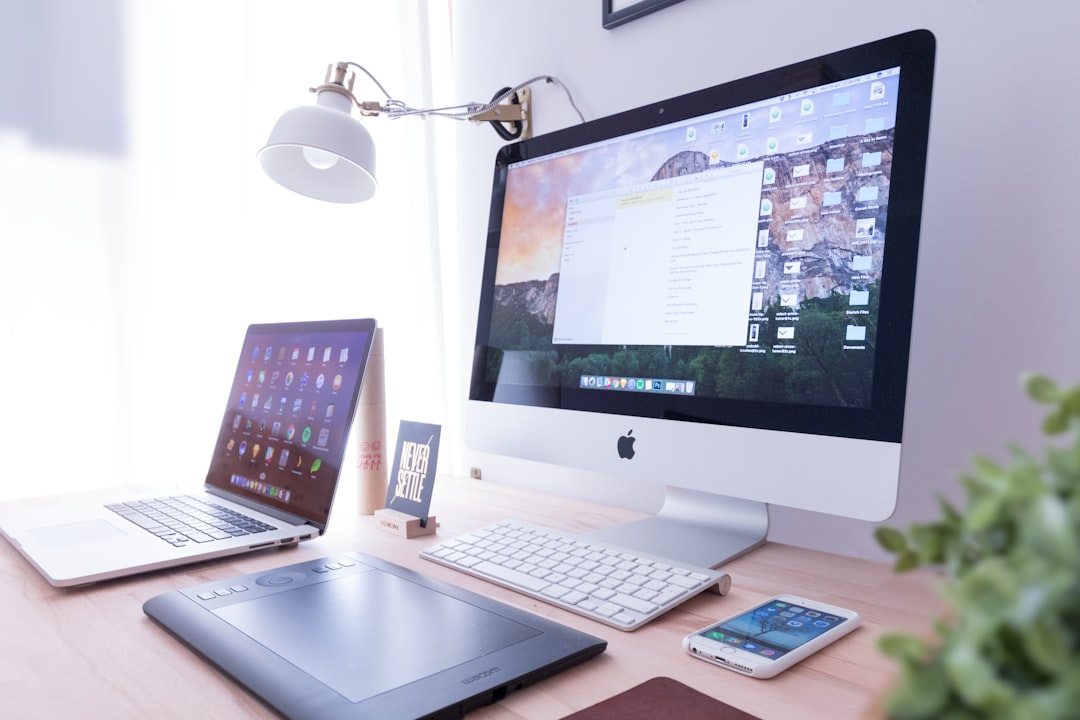
Integrating AI into existing Learning Management Systems (LMS) presents a chance to improve the learning experience. AI can analyze student data to create customized recommendations, adaptive tests, and unique learning paths. This tailored approach aims to meet individual learning needs more effectively. However, while the idea of increased efficiency and personalization is appealing, questions remain regarding the level of in-depth learning and the quality of interactions facilitated within AI-integrated LMS. There's also worry that a strong reliance on these systems might make students too dependent on technology and diminish the role of human teachers. Given how important these integrations are becoming in education, carefully considering the consequences and finding a good balance between innovation and the core goals of learning is vital. We need to make sure that technological progress doesn't overshadow the true purpose of education.
The potential for blending AI-powered tools with existing Learning Management Systems (LMS) is an interesting area of research. Using Application Programming Interfaces (APIs), we can achieve a level of interoperability that allows for real-time updates and material syncing between platforms. This can potentially expand the usefulness of pre-existing educational resources.
However, a key aspect of such integration is the ability of AI to leverage the historical data already stored within an LMS. By analyzing things like past student performance, engagement levels, and successful learning pathways, AI algorithms can enhance their suggestions and tailor individual student learning paths more accurately. The question of whether this approach really results in significantly more relevant material is still being investigated.
Furthermore, the potential for reducing costs associated with educational content is a significant driver in this area. If AI can automate content generation and updates, it could lessen the need for substantial human intervention. But it's important to consider that simply lowering the cost of producing educational materials doesn't automatically ensure that the content itself is of high quality.
The integration of AI can also influence the user experience. Dashboards within an LMS, if designed effectively with AI's help, can provide a clearer picture of student progress, outcomes, and areas that need attention. This improved visualization of data could be valuable for teachers in making informed decisions about their teaching approaches. However, it's crucial to be cautious about potential biases within such dashboards and ensure they don't misrepresent student abilities or unfairly influence teachers' perspectives.
Many LMS are built with interoperability standards in mind (like SCORM or xAPI). This adherence to standards means we might potentially see an interconnected ecosystem where educational resources and assessments can move seamlessly between different AI-powered tools and LMS platforms. It's important to acknowledge that there isn't currently a unified standard for AI integration across all LMS, so further research in this area could lead to more efficient and effective integrations.
The combination of AI and LMS can also improve our analytical capabilities. By gathering and analyzing learner data, we could gain deeper insights into student behavior and outcomes. This data could then be used to make more informed choices regarding instructional methods and resource allocation. However, this increased data gathering raises privacy and ethical considerations that require careful attention. It's important to develop systems that protect learner privacy while also providing educators with valuable insights.
Furthermore, integrating AI into an LMS creates the potential to scale up educational content efficiently. Institutions could customize and distribute learning materials to large student populations without a corresponding surge in administrative tasks. This scale could potentially make education more accessible, but it's important to make sure that such expansion doesn't sacrifice the quality of the learning experience for students.
Incorporating adaptive learning elements within the LMS through AI is another interesting area of development. AI can personalize content based on a learner's unique style and pace, potentially optimizing the learning experience while still aligning with broader curriculum standards. The challenge remains to ensure the adaptive learning components don't become overly simplistic or inadvertently create limitations in the types of skills or content students can learn.
The potential to increase accessibility for learners with disabilities is also significant. AI could help integrate features like text-to-speech, language translation, and alternative content formats within the LMS environment. This would make learning more inclusive for students with various needs, but further research is needed to investigate how effective these solutions are for a diverse range of learners.
Finally, a fascinating aspect of integrating AI with LMS is the possibility of pinpointing skill gaps at a larger scale. By analyzing student performance across a wider range of learners, we could potentially gain insights into the alignment of educational programs with industry skill demands. This data could then inform curriculum revisions and targeted interventions. However, this raises questions about how such data is interpreted and used. We need to ensure that this approach doesn't lead to narrow or biased curricula.
In essence, the integration of AI-powered tools with LMS offers both opportunities and challenges. While the promise of streamlined content, personalized learning, and enhanced analytics is alluring, it's essential to proceed with a critical and research-driven approach. It's important to be mindful of potential biases, ensure data privacy, and strive for truly personalized learning experiences that cater to the diverse needs of all students.
AI-Powered Tool Revolutionizes Learning Material Generation A Deep Dive into Efficiency and Personalization - Data privacy and ethical considerations in AI-driven education
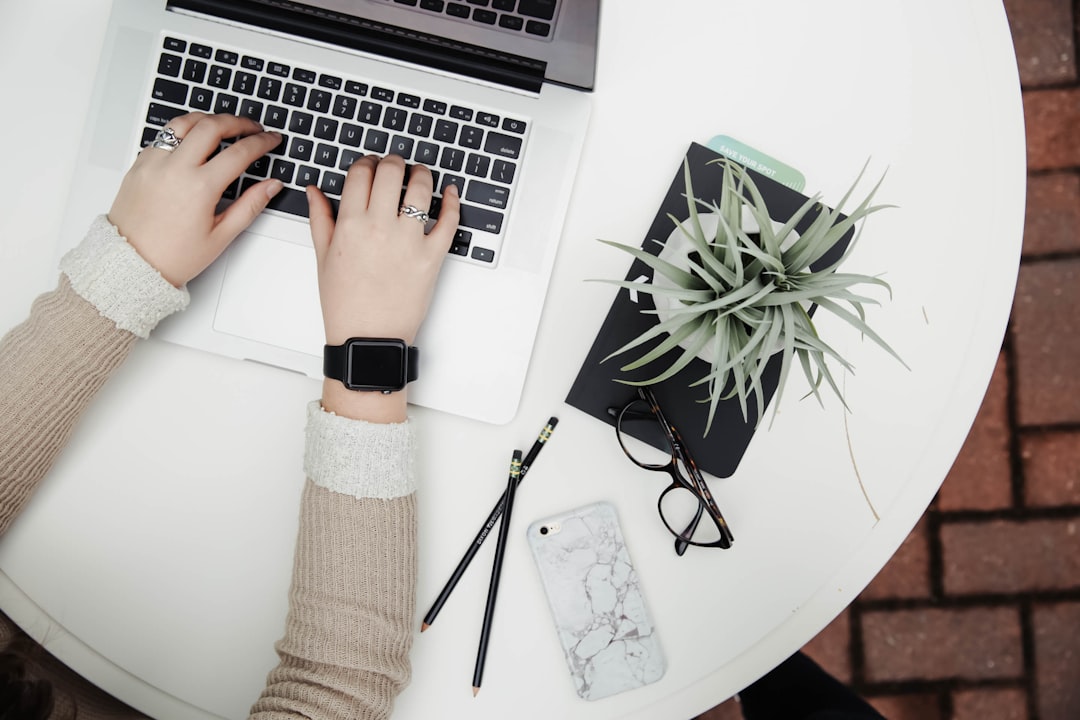
The increasing use of AI in education brings with it a crucial need to address data privacy and ethical concerns. The vast amount of student data collected and analyzed by AI systems raises questions about the security of this information and whether educational institutions can maintain ethical standards in their AI practices. There's a real worry about the potential for bias in AI algorithms, which could lead to unfair or discriminatory outcomes. To ensure a fair and inclusive learning experience, educational settings must develop strategies to lessen the impact of bias and guarantee impartiality in AI-driven decision-making. Furthermore, transparency about how AI tools work and who is responsible for their use is vital to building trust between students, educators, and the institutions themselves. As AI integration in education progresses rapidly, prioritizing ethical considerations and fostering responsible technology use becomes absolutely necessary to protect students and uphold the integrity of education.
The use of AI in education, while promising, brings up some significant issues concerning data privacy and ethical considerations, especially given the sensitive nature of educational data. Student data, including details about learning difficulties, mental well-being, and performance, can have long-term consequences, making data breaches especially problematic.
Currently, many places are still figuring out the best ways to regulate AI in education. While guidelines like the GDPR are setting standards, there's a lack of consistency across the globe, leading to different levels of data protection. This raises questions about how we can ensure fairness and equal protection for all learners regardless of their location.
Getting students (and their guardians for those under 18) to genuinely understand and agree to how their data will be used is a major challenge. Often, users aren't fully aware of the implications of what they're consenting to, which raises questions about whether they are truly making an informed decision.
Another area of concern is the potential for AI systems to reflect biases found in the data they're trained on. If the historical data used to develop the AI contains biases, the AI could unintentionally create unequal learning experiences. These biases can perpetuate societal inequalities without students or even educators fully realizing it.
Some AI systems are even designed to analyze a learner's emotional reactions to the material. While this can potentially make the learning experience more engaging, it also leads to questions about whether it's ethical to manipulate a learner's emotions for better retention or engagement. We need to be cautious about the implications of such technologies.
The rise of always-on monitoring systems like keystroke trackers or webcams in the learning environment presents further ethical concerns. These practices raise questions about surveillance and the potential for misuse of personal behavioral data. There needs to be careful consideration of when, where, and how such technologies are applied.
Data portability, or the ability for students to easily move their learning data between different schools or platforms, is another area of concern. The lack of standard ways to transfer this information can result in data loss and fragmented learning experiences, creating hurdles for students who might want to change institutions or use different learning tools.
We're increasingly pushing for more transparency in how AI algorithms work in education. Unfortunately, many of these models are still quite opaque, making it hard for educators and students to understand how AI influences their learning experience. This lack of transparency poses a challenge to establishing trust and ensuring fairness.
The introduction of AI tools has also led to questions about how teachers' roles will change. While teachers can benefit from the insights AI provides, concerns arise about the reduction of human interactions, which are often vital for students' educational and personal development. Finding the right balance between technology and human connection is vital.
Finally, we haven't reached a consensus on how long educational data should be kept. Determining appropriate data retention periods is crucial to protecting students' privacy while satisfying the needs of schools. This is an especially important issue as regulations and standards in this area are still evolving.
These issues show that while AI has a lot of potential for transforming education, we need to address these critical questions before it becomes widely adopted. The ethical implications of AI-powered learning are complex, but thoughtful consideration of these issues is necessary to ensure that the use of AI benefits all learners and promotes a fair and equitable educational experience.
Create AI-powered tutorials effortlessly: Learn, teach, and share knowledge with our intuitive platform. (Get started for free)
More Posts from aitutorialmaker.com: