AI's Impact on Interest Rate Forecasting A Market for Loanable Funds Perspective
AI's Impact on Interest Rate Forecasting A Market for Loanable Funds Perspective - AI-driven analysis of supply and demand in the loanable funds market
The application of AI to analyzing supply and demand within the loanable funds market offers a powerful new lens for understanding interest rate movements. AI algorithms can meticulously examine the interplay between those who save and those who borrow, uncovering how shifts in these behaviors affect the equilibrium interest rate. This more detailed understanding boosts the precision of interest rate forecasts and facilitates a more refined analysis of how external elements, like economic conditions and policy choices, affect market activity. As financial models continue to adapt, AI integration has the potential to reshape our traditional understanding of the loanable funds market, possibly leading to a reevaluation of the established concept of the natural rate of interest. Nonetheless, it's vital to proceed with prudence, acknowledging that relying solely on AI might disregard the complexities and inherent uncertainties that stem from human economic decision-making. The loanable funds market, while conceptually straightforward, remains susceptible to unexpected shifts in human behavior, which AI models may struggle to fully capture.
AI's ability to analyze the loanable funds market by examining supply and demand offers a promising avenue for improving interest rate forecasting. These AI models can process enormous datasets in real-time, making interest rate predictions more dynamic and responsive than traditional methods. Machine learning, through its algorithms, can pick up on subtle shifts in borrower and lender behaviors—nuances that might go unnoticed in traditional analyses, enhancing forecast accuracy.
The potential of neural networks is especially noteworthy. They can integrate unstructured data like news reports and social media sentiment, offering a broader understanding of the various factors influencing interest rates. Further, AI can simulate a wide range of economic situations, enabling banks and financial institutions to develop more robust risk management strategies.
Interestingly, AI might be able to detect early warning signs of economic downturns through the identification of patterns in loan defaults and borrowing behavior. This could potentially lead to more timely policy interventions. AI also offers the possibility of fine-tuning loan pricing by more accurately assessing individual borrowers’ risk profiles. This could produce interest rates that more accurately reflect the actual cost of borrowing.
The incorporation of AI into the loanable funds market analysis could lead to changes in central bank strategies. Real-time data analysis could lead to faster and more responsive monetary policy adjustments, potentially influencing overall economic stability. AI can also reveal regional variations in the demand for loanable funds, illuminating areas experiencing potential economic growth or decline and suggesting potential targeted financial solutions.
Historical data analysis using AI often reveals patterns and cycles that may be overlooked by human analysts. This suggests that AI could enhance the understanding of long-term economic trends. In a market often hindered by information imbalances, AI can fill in those gaps by providing comprehensive borrower profiles for lenders, leading to a potential transformation of the traditional lending process. While still in its early stages, AI's application to the loanable funds market is an area worthy of continued research and exploration.
AI's Impact on Interest Rate Forecasting A Market for Loanable Funds Perspective - Machine learning models for real interest rate predictions
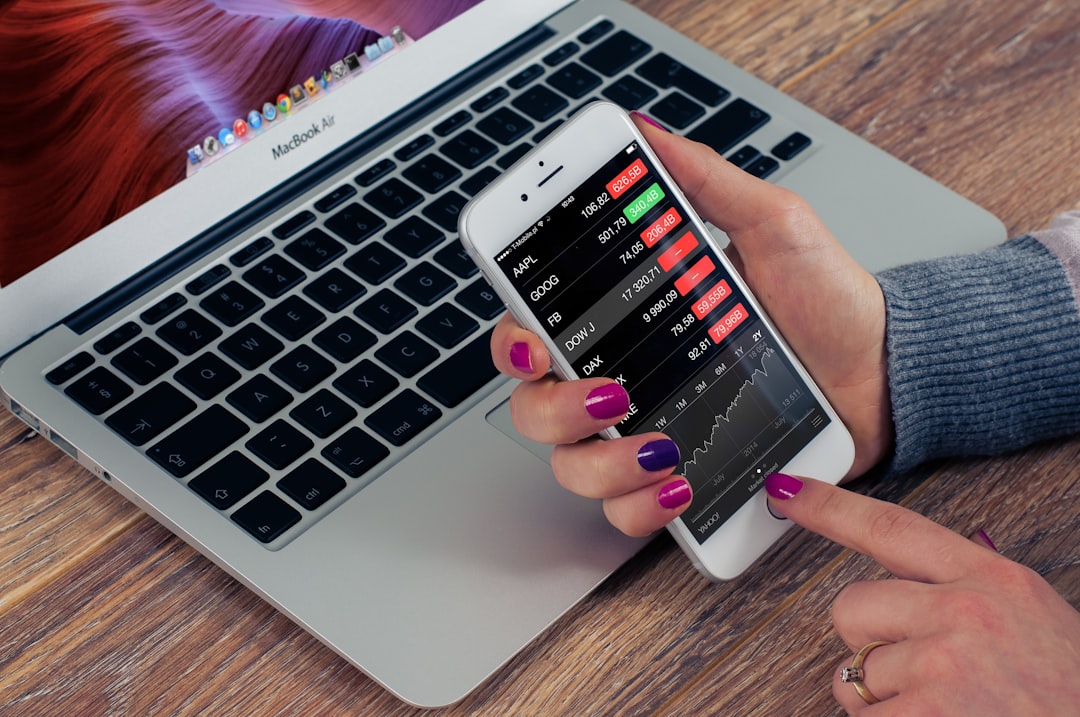
Machine learning models are increasingly important for forecasting real interest rates. They can analyze massive datasets using sophisticated algorithms, offering advantages over traditional methods. These models automate data extraction and provide a deeper understanding of the forces driving interest rate changes. By adjusting parameters, selecting relevant features, and fine-tuning model structure, researchers can improve the accuracy and ability of these models to adapt to evolving market conditions. Interestingly, machine learning models can incorporate unconventional data, like social media sentiment, which broadens the scope of financial analysis and captures aspects of market behavior that older approaches might miss. In today's volatile markets, these models are crucial for improving risk management and for making more informed monetary policy decisions. While promising, it's essential to remember that human judgment and understanding of the complexities of economic decision-making remain crucial elements in the financial landscape.
Machine learning models can tap into extensive historical data, encompassing decades of economic indicators. This allows them to uncover intricate relationships and trends that traditional models might overlook, potentially leading to more accurate predictions of real interest rates. It's intriguing that including non-traditional datasets, like online purchasing patterns or social media engagement, has shown promise in improving the predictive power of these machine learning models for interest rate forecasting. These models can now be more sensitive to changes in consumer sentiment and behavior.
Many machine learning models rely on ensemble methods, where multiple prediction algorithms are combined. This approach enhances the overall robustness of the model and mitigates the risk of overfitting, a common challenge in forecasting economic indicators like real interest rates. Research suggests that machine learning techniques can outperform traditional econometric models, especially during volatile economic periods where traditional models may struggle to maintain accuracy.
Certain machine learning algorithms, such as recurrent neural networks (RNNs), are specifically designed to handle sequential data. This makes them particularly well-suited for analyzing time-series data related to interest rates, capturing temporal dependencies that linear models often miss. It's interesting that machine learning can expose potential biases within traditional economic data interpretations. This suggests that certain indicators may not fully reflect the actual economic conditions influencing interest rates.
A challenge with many machine learning models is transparency. While they can produce very accurate predictions, the complexity of their decision-making processes can make it hard to understand the logic behind specific forecasts. This is crucial in financial decision-making, where understanding the 'why' is often as important as the 'what'. Some machine learning models are capable of identifying non-linear relationships in economic data, allowing for more sophisticated modeling of interest rate dynamics. This could potentially offer better insights, especially in emerging or volatile markets.
The application of reinforcement learning in finance is growing. In this context, algorithms autonomously adapt their strategies based on real-time economic feedback, refining their interest rate predictions as economic conditions evolve. It's fascinating to think that integrating machine learning into interest rate forecasting could fundamentally change investment behavior. As the insights derived from these models become more critical tools for investors, it could significantly alter asset management and the overall landscape of financial strategy development.
AI's Impact on Interest Rate Forecasting A Market for Loanable Funds Perspective - Impact of AI on DeFi protocols and interest rate mechanisms
AI's integration into DeFi protocols is reshaping the landscape of decentralized finance. DeFi protocols, built on smart contracts, aim to create efficient markets for lending and borrowing by automatically adjusting interest rates based on supply and demand. AI's role in these protocols is becoming increasingly important as it enhances both their security and functionality. Through the analysis of large datasets and user behavior, AI algorithms can improve the accuracy of interest rate adjustments, leading to more responsive and efficient lending and borrowing processes within DeFi. However, it is important to acknowledge that the increasing reliance on AI and automated systems in finance introduces new vulnerabilities and raises questions about the potential for unforeseen systemic risks. Balancing the potential benefits of AI with the need for a stable and secure financial environment is a crucial ongoing challenge. The way DeFi protocols manage and forecast interest rates may be irrevocably changed through AI and will need robust oversight to manage and navigate the potential consequences.
Decentralized finance (DeFi) protocols, particularly those focused on loanable funds, rely on smart contracts to create decentralized markets for borrowing and lending. These protocols use interest rate mechanisms to balance supply and demand for funds, adjusting rates based on the prevailing market conditions. Researchers have noted AI's potential to bolster the dependability and security of these DeFi systems, perhaps reshaping how they operate.
DeFi presents a new financial landscape that leverages blockchain technology to deliver financial services without traditional intermediaries. The way interest rates are set varies across DeFi protocols, with non-linear models offering greater flexibility in adjusting rates compared to simpler, linear models. Integrating AI into interest rate forecasting holds the potential to improve the accuracy of predictions in DeFi, which could significantly influence borrowing and lending strategies. However, the growth of DeFi has also brought new risks and challenges, especially those tied to the inherent nature of new technologies and decentralized systems.
The transparency offered by DeFi's smart contract-based, automated transactions is beneficial, but it also exposes users to unique risks compared to traditional finance. Combining AI with DeFi opens up promising possibilities for innovation, especially in areas like managing liquidity and optimizing interest rates. For instance, AI's capacity for real-time data processing allows DeFi protocols to dynamically adjust interest rates in response to constantly changing market situations, providing a quicker response than the often slower, periodic adjustments of traditional financial systems.
Additionally, DeFi protocols could potentially leverage AI to improve the smart contract risk assessment process. This could lead to potentially lower borrowing costs and more optimized collateral requirements by enabling a more nuanced risk evaluation for each borrower rather than relying on a one-size-fits-all approach. Furthermore, AI can help analyze social media sentiments to better understand market behavior shifts, giving DeFi platforms a head-start in predicting borrower demand and preemptively adjusting interest rates to mitigate risks related to fast-changing market sentiment.
Advanced machine learning can also be used to identify patterns in borrower behavior that are predictive of defaults with increased precision. This could enable lending platforms to adjust interest rates or tighten loan criteria before substantial losses occur. It's conceivable that AI could facilitate algorithmic lending strategies in DeFi, where interest rates are automatically adjusted based on borrower creditworthiness and broader market information, ultimately promoting more efficient allocation of funds.
AI can also influence decentralized autonomous organizations (DAOs) that govern DeFi protocols. By incorporating AI into their decision-making processes based on predictive data analysis, DAOs might become more resilient and able to respond to changing market dynamics. Furthermore, optimizing liquidity mining strategies by using AI to pinpoint the ideal incentive structures to attract liquidity in real-time could lead to more competitive interest rates for both lenders and borrowers.
While the use of AI in DeFi offers opportunities, it also carries some potential concerns. For example, the application of AI to rapidly identify arbitrage opportunities across various DeFi protocols can enable users to profit from differences in interest rates. However, this could introduce greater volatility and unpredictability in interest rate settings across the DeFi landscape. AI's ability to analyze historical data and recognize economic patterns that influence interest rates could provide valuable insight to DeFi protocols for developing strategies to protect against economic downturns. These patterns might not be readily apparent to human analysts.
Interestingly, AI can also potentially customize the borrowing experience within DeFi by tailoring interest rates based on an individual's behavior and past interactions with the platform. This might pave the way for fairer and more appealing financial offerings. Though, we need to approach this with caution, as it can also raise ethical and privacy concerns. These are just a few of the potential ways that AI is shaping the future of DeFi, and this is an area that requires ongoing research and exploration.
AI's Impact on Interest Rate Forecasting A Market for Loanable Funds Perspective - AI's role in forecasting international capital flows and equilibrium rates
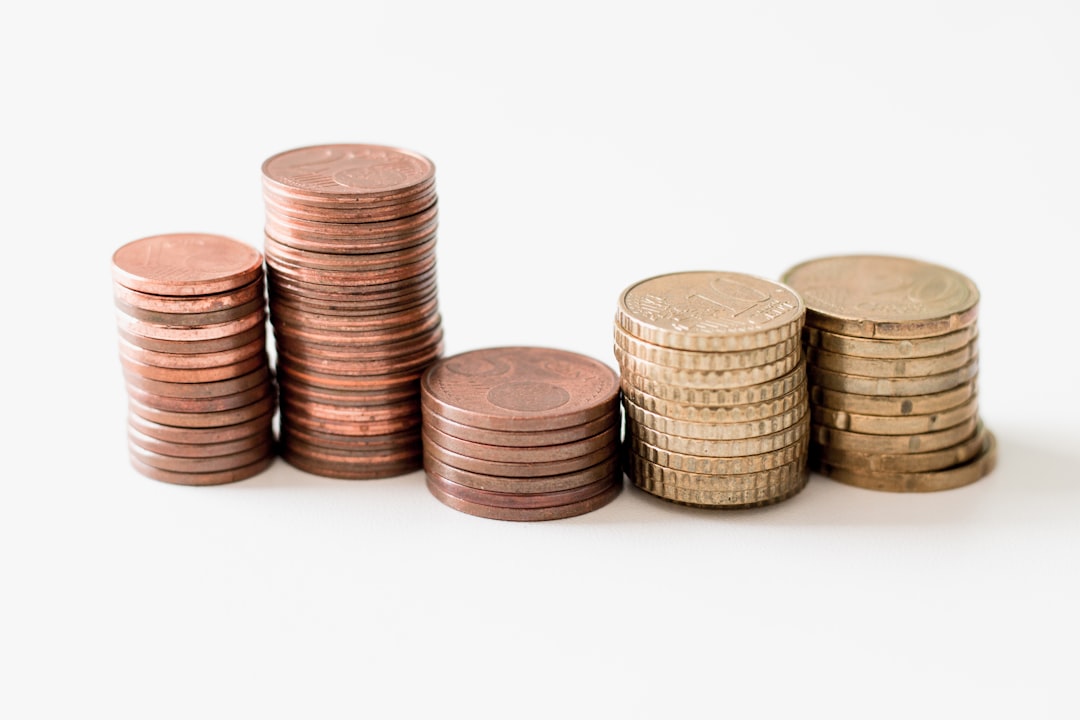
AI is becoming increasingly important in predicting international capital flows and the equilibrium interest rates that emerge in the global financial system. AI algorithms can analyze extensive data on variations in real interest rates across different countries, helping to pinpoint how these differences drive investment decisions. This capacity for real-time analysis of both inflows (foreign investment in domestic assets) and outflows (domestic investment in foreign assets) is especially valuable in understanding economic stability, especially in developing economies where foreign capital can reduce overall risk and promote growth. AI can also track how international capital flows influence domestic interest rates, providing a better understanding of how global finance impacts local economies and helping to inform policy decisions and investment strategies. It is crucial to remember that AI, despite its advanced capabilities, is still a tool and cannot fully grasp the intricate and often unpredictable nature of human economic decision-making that can drive markets. While AI enhances forecasting, it can't eliminate the inherent uncertainties associated with the complexity of economic interactions.
AI's potential in forecasting international capital flows and equilibrium exchange rates is gaining attention. AI models can combine traditional macroeconomic data with less conventional sources, such as online activity and search patterns, to create a more comprehensive picture of how capital moves internationally. This broader view may reveal correlations between public sentiment and capital flow shifts that were previously harder to detect.
Machine learning algorithms can adapt rapidly to changes in real-time data streams, allowing financial institutions to anticipate potential market disruptions related to unforeseen events, like geopolitical shifts or regulatory adjustments. These shifts, in turn, can influence equilibrium exchange rates.
However, AI models haven't fully cracked the code on predicting human behavior in financial markets. People often act irrationally, leading to a disconnect between predicted and actual capital flow patterns.
Research suggests that AI could increase the efficiency of international capital markets. By pinpointing areas where capital is allocated inefficiently across the globe, AI might help optimize equilibrium rates and ensure funds are deployed effectively.
AI also has the potential to manage risks associated with volatile capital flows. Its ability to uncover hidden patterns in financial data can help identify early warning signs of capital flight or inflows, giving institutions time to adjust strategies and maintain stability.
Machine learning methods have shown promise in modelling the complex relationships between various international economic indicators, such as exchange rates and interest rates. This holistic perspective offers a significant improvement over more isolated approaches to forecasting capital flows.
Through stress testing, AI can help analysts simulate the impact of interest rate fluctuations on capital flows. This ability to anticipate various risk scenarios equips decision-makers with crucial insights to navigate uncertain economic environments.
There's an increasing trend towards visualizing capital flows with more detail, thanks to AI. We can see not only the overall trends, but also more specific regional and industry-based influences on equilibrium rates.
Interestingly, AI models can even anticipate the reactions of global investors to policy shifts. By understanding how capital flows are likely to respond to upcoming monetary or fiscal policy changes, financial institutions can improve their planning.
While the future of AI in forecasting capital flows is bright, it's vital to acknowledge the limitations of AI's transparency. The inner workings of these models often aren't easily understood, raising valid concerns about how these forecasts are used in crucial financial decisions.
AI's Impact on Interest Rate Forecasting A Market for Loanable Funds Perspective - Algorithmic detection of market shifts during economic downturns
During economic downturns, the capacity of algorithms to detect shifts in market dynamics is becoming increasingly significant. Businesses are eager to harness AI's capabilities for more accurate economic forecasting, particularly during periods of volatility. By utilizing advanced machine learning techniques, financial institutions can delve deeper into understanding patterns in borrowers' behaviors, including loan default rates and overall market sentiment. This increased awareness can be invaluable during economically challenging times.
AI's power to analyze vast datasets in real-time allows for a quicker response to potential changes in market conditions. This heightened responsiveness supports more timely intervention strategies to reduce risks associated with market shifts. Furthermore, integrating AI can offer insights into how financial crises might unfold, allowing for the identification of early warning signs and proactive measures to potentially mitigate their impact.
It's crucial, however, to approach the reliance on AI with prudence. The intricate nature of human behavior and the subtle nuances of economic interactions remain complex challenges that algorithms might struggle to fully encompass. While AI's analytical capabilities are undoubtedly promising, its limitations must be acknowledged and considered in the context of practical applications within the financial landscape.
During economic downturns, the ability to detect market shifts early on becomes crucial. Algorithms are increasingly being used to analyze massive datasets in real-time, hoping to pick up on trends that might be missed by humans. They can sift through mountains of financial transactions, economic indicators, and even social media chatter to identify subtle changes in consumer behavior, especially when it comes to borrowing and loan repayment. This capability is particularly interesting for researchers because it might help signal an impending downturn.
Advanced machine learning methods can go further. They can adapt their predictions as new information becomes available, making them more responsive to the rapid changes that can occur during economic contractions. These algorithms can also help to reveal how changes in interest rates, for example, feed back into borrower behaviors and possibly create cycles of instability. It's fascinating how these feedback loops can be uncovered.
One particularly interesting aspect is the ability of these models to blend traditional economic data with less conventional information, like social media trends and e-commerce patterns. This can provide a more complete picture of how consumers and markets are shifting, something that traditional methods might miss. And, because they can recognize non-linear relationships in economic data, the models can potentially gain a more accurate understanding of how various factors interact, leading to better interest rate predictions and market shift forecasts.
Despite the promise of AI in this area, there are hurdles to overcome. One significant issue is the unpredictable nature of human behavior, especially in moments of crisis when fear and uncertainty might dominate financial decision-making. It's a challenge for algorithms to capture these emotional aspects.
Still, it's also possible that these algorithms could act as early warning systems for systemic risks in the economy. By continuously monitoring a variety of economic data, they might pick up on signs of emerging dangers, potentially allowing for proactive policies to help stabilize things. They can also help identify local economic differences and variations in interest rate sensitivity, making it possible to design targeted interventions that are more effective.
However, a major concern remains: the lack of transparency in how these algorithmic models make their predictions. This can be a problem for policymakers and investors who need to understand the reasoning behind these forecasts before making critical decisions. It's an interesting question whether we can trust these 'black boxes' during periods of economic turmoil. There's still much to learn about how best to leverage AI to predict market shifts, especially when the economic landscape is rapidly changing.
AI's Impact on Interest Rate Forecasting A Market for Loanable Funds Perspective - AI-enhanced modeling of government demand for loanable funds
AI's integration into modeling the government's demand for loanable funds offers a powerful new tool for interest rate forecasting. By leveraging massive datasets including economic indicators, fiscal policy actions, and even less traditional data such as social media trends, AI can significantly improve our understanding of how government borrowing influences equilibrium interest rates. These advanced models allow for more dynamic adjustments to forecasts, giving policymakers a real-time view of how government spending and fiscal policy choices might affect the loanable funds market. This can provide valuable information to make informed decisions about government spending and related policies.
While the potential benefits of AI are undeniable, it's important to acknowledge that the loanable funds market is still susceptible to human behavior, which can be difficult to predict. The complex interplay of motivations, perceptions, and economic conditions can sometimes lead to unpredictable outcomes that AI models may not fully capture. Therefore, though AI offers an enhanced understanding of government demand for loanable funds, it should be used alongside human judgment and experience when interpreting results and informing policy decisions. The future of interest rate forecasting is likely to be significantly impacted by these AI-powered models, but a critical and nuanced approach will ensure that these tools are employed responsibly and effectively.
The application of AI to model government demand for loanable funds opens up new avenues for understanding how government borrowing impacts the overall economy. AI models can leverage a wider range of data sources compared to traditional methods. This includes online activity, public opinion expressed on social media, and macroeconomic news, allowing for a richer context when evaluating government borrowing decisions.
One of AI's strengths is its ability to adapt quickly to real-time information. This allows for a more agile and responsive model compared to older methods which often lag behind current market conditions. This is especially important when considering how borrower and lender behavior changes during economic swings.
However, despite their power, AI models struggle with predicting the unpredictable nature of human behavior, particularly in moments of crisis when fear can drive unusual borrowing patterns. It's this human element of decision-making that poses a challenge for AI's forecasting accuracy.
Additionally, AI techniques can provide a granular view of regional economies by identifying variations in loan demand. This insight is beneficial for policymakers who might develop more targeted economic policies or pinpoint areas requiring specific financial support. Similarly, AI's capability to recognize early signs of potential loan defaults offers an opportunity for governments to implement preventative measures to maintain financial stability.
International capital flows, as analyzed by AI, can significantly affect a nation's equilibrium interest rates and, consequently, government borrowing costs. This creates complexities in government economic planning that need careful consideration.
Machine learning, a key aspect of AI, has the potential to expose hidden, non-linear relationships within economic data. This could lead to a more accurate understanding of the diverse factors influencing government borrowing. Moreover, AI models can uncover intricate feedback loops within the loanable funds market. These loops highlight how changes in interest rates can influence borrower behavior, which in turn can affect government borrowing demands, making forecasting more intricate.
A concern arising from the use of AI in these models is the lack of transparency in their decision-making processes. This "black box" aspect can create skepticism among policymakers who might be hesitant to fully trust forecasts when designing financial strategies.
Ultimately, AI-powered insights could enable governments to develop more refined and targeted loan programs. By creating a more accurate assessment of various borrower risk profiles, policymakers can develop solutions that effectively address specific economic needs. This could enhance efficiency in how government funds are allocated.
While AI holds immense potential in this area, continued research and critical evaluation are essential for navigating the complexities and ensuring responsible application of these new modeling approaches within the loanable funds market.
More Posts from aitutorialmaker.com: