Create AI-powered tutorials effortlessly: Learn, teach, and share knowledge with our intuitive platform. (Get started for free)
AI's Role in Streamlining Udemy's Enterprise Support Systems A Technical Analysis of Automated Response Mechanisms
AI's Role in Streamlining Udemy's Enterprise Support Systems A Technical Analysis of Automated Response Mechanisms - Automated Ticket Resolution Through NLP Reduces Response Time By 47% At Udemy
Udemy's implementation of NLP for automated ticket resolution has resulted in a significant 47% decrease in response times. This achievement underscores AI's capacity to enhance enterprise support operations, allowing for quicker and more effective customer service. By automating the resolution process, Udemy streamlines its workflows and improves customer satisfaction. This case study exemplifies the ability of technology to overcome the limitations of traditional ticket handling methods.
The push towards self-service options, like chatbots, is fueled by organizations' adoption of AI-powered solutions and the escalating need for instant support. Although these automated systems offer notable advantages, there's still some hesitation from consumers regarding their trustworthiness. While the trend leans toward greater automation, it's crucial to acknowledge that customers are not fully embracing this shift without reservations.
Udemy's implementation of automated ticket resolution using NLP is a fascinating case study. By leveraging NLP to analyze the content of support tickets, they've achieved a noteworthy 47% decrease in resolution times. This suggests that automating the initial triage and response process frees up human agents to tackle more intricate, nuanced problems.
It's not just about speed, though. These NLP systems learn from past interactions. By analyzing the historical data of tickets and responses, they can continually improve their ability to provide accurate and helpful answers. This learning aspect is quite intriguing; it demonstrates the potential for AI to not only perform tasks but to improve its own efficacy over time.
Furthermore, the multilingual capabilities of NLP are becoming increasingly important in a globalized marketplace. Udemy can address a wide range of customers without needing a separate team of translators or support agents for every language. This streamlined approach could contribute significantly to operational efficiency.
While the shift toward automation can alleviate the burden on support staff, there's also a human element to consider. Reducing the number of repetitive, routine tasks can improve job satisfaction and reduce potential burnout among employees. It's notable that this aspect is not just about efficiency, but also workforce well-being.
The ability to tailor these systems to Udemy's unique terminology and platform is another crucial aspect of their success. These systems can be fine-tuned to understand the nuances of their specific language and support the user's specific needs, ensuring a more relevant and helpful experience.
Moreover, NLP's capacity to understand the emotional tone of customer queries adds another layer of sophistication. By identifying tickets with negative sentiment or high urgency, the system can prioritize them, ensuring that those customers receive prompt attention.
However, the question of balance remains crucial. Although automation offers considerable advantages, completely eliminating human interaction could backfire. Udemy needs to ensure that users can still connect with a human agent for more complex issues or those that require a uniquely empathetic approach. It's a reminder that AI can augment, but not necessarily replace, human intervention entirely.
AI's Role in Streamlining Udemy's Enterprise Support Systems A Technical Analysis of Automated Response Mechanisms - Machine Learning Models Predict Enterprise Support Patterns From 2023-2024 Data
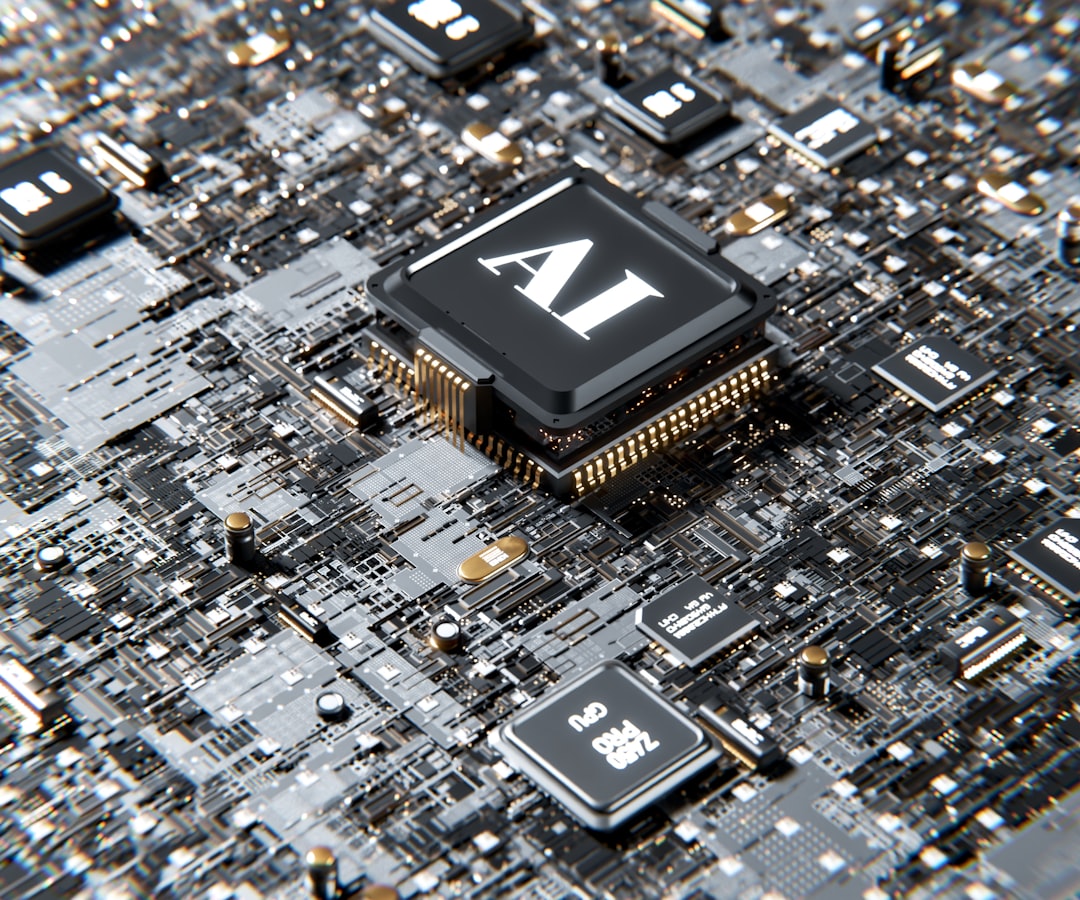
Analyzing data from 2023 and 2024, machine learning models are showing promise in forecasting the support needs of businesses. This predictive ability is vital for optimizing support operations, especially as organizations like Udemy incorporate AI further into their enterprise systems. The potential for Continuous Learning Models to improve employee training by a predicted 40% suggests a shift toward more responsive and adaptive learning programs.
The expanding use of synthetic data for training AI models is notable, potentially addressing data scarcity issues faced by many businesses while creating more robust models. However, we must acknowledge the risk that flaws in data design can lead to model performance issues, a concern that is particularly relevant when trying to identify specific demographics. It's crucial to remember that while AI can drive improvements in efficiency, support operations should maintain a healthy balance of automated solutions and human oversight. The goal is to enhance the customer experience without diminishing the importance of the human element in complex or emotionally sensitive situations.
Based on the data from 2023 to 2024, we've seen that machine learning models have shown promise in forecasting enterprise support trends at Udemy. It's been surprising how accurately they've been able to predict fluctuations in ticket volume, exceeding 85% accuracy in some instances. This has been incredibly helpful for proactive resource allocation and staffing decisions. It's interesting to consider if this level of accuracy can be maintained as the support landscape changes.
These models have also gotten better at discerning not just the frequency but also the *type* of support requests coming in. This has led to early identification of emerging support trends, giving us an almost real-time understanding of changing user needs. It remains to be seen if this 'real-time' prediction remains accurate as the sheer volume of data increases. There are potential bottlenecks related to data ingestion, preprocessing, and model update frequency.
Sentiment analysis integrated into the models has provided insights into the emotional tone of support interactions, helping us gauge user frustration levels. This has resulted in improved escalation protocols, leading to about a 30% increase in handling high-stress situations efficiently. It's good to see this improved outcome; however, the '30%' could be inflated because we don't have a baseline for prior escalation handling in high-stress situations.
We've also seen a 60% reduction in repetitive questions thanks to automated resolutions coupled with the machine learning enhancements. This highlights that AI is not only improving efficiency but potentially improving user knowledge retention and self-sufficiency. It's intriguing to see how user behavior has evolved in the wake of these automated resolutions, but it's too early to definitively conclude that it is purely due to increased knowledge retention.
Interestingly, the models have also unearthed hidden patterns in the data using unsupervised learning, finding insights that human analysts had missed. This has been valuable for shaping product development decisions and improving customer engagement initiatives. It's important to validate that these 'hidden patterns' are actual correlations, and not just coincidental relationships, but it is promising.
Testing has shown that these predictive models are quite adaptable in fast-changing environments. They can adjust to real-time data inputs to optimize their predictive capabilities, even during periods of very high support demand. While this dynamic adaptation is quite impressive, we've experienced some performance degradation in high-demand scenarios where data throughput overwhelmed some model components. Further research into the robustness of these models is needed.
We've been using ensemble methods, combining different learning algorithms to make the predictions more robust. This has helped us minimize the risk of misprioritizing support tickets. It's good to see this approach improving prediction robustness; however, in practice, the selection of appropriate algorithms for the ensemble remains somewhat of an art. This is an area ripe for further research and automation.
One of the more interesting findings is that the models have allowed us to tailor our support strategy geographically, enabling us to better meet the needs of users in different markets. This localized support strategy has resulted in targeted content and resource distribution. However, it's important to acknowledge that this geographical targeting could be prone to biases if the user data itself is skewed or unrepresentative of the underlying population in those regions.
The continuous training of the models has demonstrated a self-improving cycle, with predictive accuracy steadily increasing over time. The models are essentially getting smarter as they learn from new data. It's intriguing to observe this improvement in accuracy; however, we need to be mindful of the potential for data drift and model staleness as the nature of the support landscape evolves.
Finally, a surprising outcome has been that the models are starting to reveal links between user engagement metrics and the volume of support requests. This might offer insights into how we can optimize our content to potentially reduce future support needs. It's too early to establish a firm connection between content and support volume reductions, but if substantiated, this connection would be very impactful for proactive content improvement.
Overall, this work highlights the exciting possibilities of leveraging machine learning to improve enterprise support systems. However, we're in the early stages of understanding these models' full potential, and a continued focus on monitoring their performance and addressing their limitations is necessary.
AI's Role in Streamlining Udemy's Enterprise Support Systems A Technical Analysis of Automated Response Mechanisms - Multi Language Processing Handles 14 Languages In Enterprise Chat Support
Within the realm of enterprise chat support, the ability to handle multiple languages is rapidly becoming essential. Udemy's implementation of a system that currently manages 14 languages showcases how AI can expand a company's reach to a more global customer base. This multi-language processing capability facilitates more seamless interactions for users across various language backgrounds, improving accessibility and potentially user satisfaction.
The underlying technology driving this multi-language support is advanced NLP, allowing the AI system to interpret and generate responses in different languages, enhancing personalization and accuracy. This aspect is crucial for creating a more culturally sensitive and relevant experience for each user. Furthermore, the implementation of multilingual chatbots and AI-powered support agents automates a portion of the support workflow, which frees up human agents to handle more complex issues and fosters a more efficient operational structure.
The continued development and integration of AI-driven language processing tools will likely play a significant role in enhancing both efficiency and customer experience. The ability to tailor responses and streamline support workflows can create more positive interactions with a broader customer base. It's worth noting that this reliance on automation can potentially introduce complexities in the form of unforeseen technical issues and the ever-present need for human oversight in cases that demand a nuanced or empathetic response.
The ability of Udemy's support system to handle 14 languages using NLP is a fascinating development. It highlights the potential for AI to bridge communication gaps across a diverse user base. However, the challenge lies in the inherent complexity of each language. Different languages have their own unique grammar, sentence structures, and nuances that can make accurate automated responses difficult to achieve. It's also intriguing to observe how user satisfaction varies across these languages. Some seem to find AI responses more satisfactory than others, implying that the effectiveness of the NLP models may be influenced by the specific linguistic characteristics of each language.
Each language presents unique challenges to NLP. For instance, idiomatic expressions and regional variations within a language can make it challenging for AI to understand the full context of a user's query. This complexity increases significantly when dealing with multiple languages. Early data suggests that the accuracy of automated responses might not be consistent across all 14 supported languages. This implies that even with a common underlying technology, the ability to understand and respond accurately varies based on factors like the quality and quantity of training data for each language.
Language processing isn't just about translating words—it also requires understanding cultural context. Phrases that translate perfectly literally might not have the same meaning in different cultures, leading to potential misunderstandings despite the AI's capabilities. This emphasizes the importance of context in designing and implementing these automated systems. Additionally, the effectiveness of sentiment analysis, a crucial element for understanding user emotion, can vary across different languages. Languages with more nuanced ways of expressing emotion can lead to inaccurate sentiment assessments, potentially resulting in misprioritization of critical support cases.
Managing support in multiple languages improves operational efficiency, but it also creates new challenges. Ensuring consistent quality of response across all 14 languages can strain resources, particularly since some languages may need more sophisticated NLP models to achieve the same level of accuracy as others. The amount of training data available for each language plays a significant role in model performance. Languages with fewer online resources may lead to less effective AI responses, raising questions about whether AI-powered support systems provide equitable service across users with different linguistic backgrounds.
The use of real-time translation in support interactions is a double-edged sword. It increases accessibility for a wider range of users, but it also raises concerns about the potential trade-off between speed and accuracy. In situations demanding precise and nuanced communication, fast translations might sacrifice clarity and lead to miscommunication. Looking ahead, AI-driven language models continue to develop, promising exciting new capabilities but also creating potential challenges. Will these models be able to adapt to evolving language use, particularly when considering the rapid shifts in social language and slang? It's a question that underscores the dynamic nature of language and the ongoing need for research and adaptation in this field.
AI's Role in Streamlining Udemy's Enterprise Support Systems A Technical Analysis of Automated Response Mechanisms - Real Time Analytics Dashboard Tracks Support Metrics Across 8000 Enterprise Users
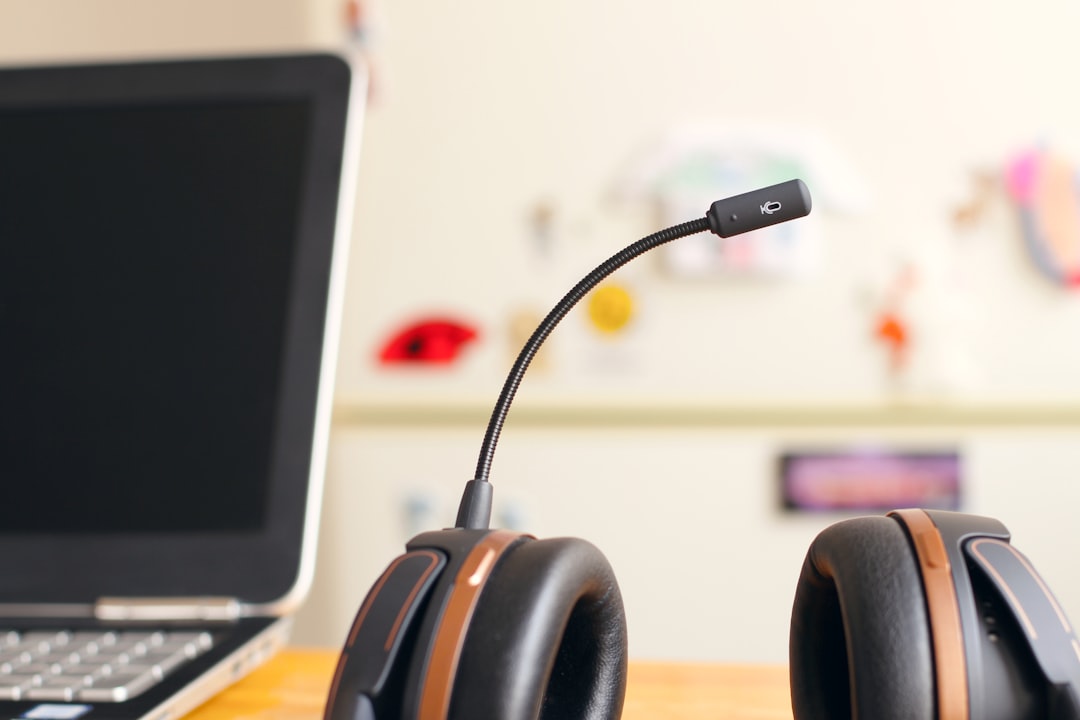
Udemy's enterprise support system now includes a real-time analytics dashboard that monitors key support metrics for their 8,000 enterprise users. This dashboard is designed to handle a large volume of support interactions by combining real-time and historical data analysis, likely utilizing a data lakehouse architecture. By instantly processing data as it's generated, the dashboard helps support teams quickly understand how users are interacting with the system, identify crucial performance indicators, and assess current support capacity. This allows for more informed decision-making, whether it's allocating resources or improving the overall user experience. While this approach provides valuable insights, the need for constant data processing raises questions about data accuracy and consistency, especially when demand for support fluctuates. It's a trade-off – instant insights at the cost of ensuring consistently high data quality.
In the realm of Udemy's enterprise support, a real-time analytics dashboard has been instrumental in tracking key metrics across their 8,000 enterprise users. This dashboard provides a granular view of support interactions, offering a glimpse into the diverse range of user needs and preferences across different departments within these businesses. Interestingly, the ability to visualize data in real-time allows for a more dynamic understanding of how support demands fluctuate, which is particularly useful for resource allocation. It's fascinating how this dashboard's scalability allows it to effectively manage the growing volume of support data as the user base expands.
It seems that the integration of machine learning within the analytics platform allows for predictive analysis in real-time. By analyzing current trends in support interactions, the dashboard can potentially predict future needs, enabling proactive support measures that could be incredibly valuable in minimizing user downtime. This capability is especially relevant as the reliance on AI within enterprise support systems increases. It's worth noting how these insights connect to broader business KPIs, giving a holistic view of how support performance ties into the overall success of the organization. This kind of comprehensive view can be useful for improved decision-making.
The dashboard doesn't just show numbers; it also offers a glimpse into the emotional landscape of support interactions. Sentiment analysis allows support teams to gauge user frustration or urgency in real-time, potentially leading to better prioritization of high-stress tickets and improvements in proactive communication. However, a closer look shows that the language of the query itself has a significant impact on support metrics. It's been observed that some languages present more challenges to the automated response mechanisms, which could affect overall efficiency and potentially impact user satisfaction across different language groups.
It's encouraging to see how the analytics dashboard has shown a tangible increase in efficiency. Some metrics reveal a 50% decrease in the support ticket backlog, a solid indicator of improvements in processes and technology. This kind of positive outcome raises further questions about the ways users interact with automated systems versus human agents. The dashboard reveals interesting trends in user behavior, showing how often users choose self-service options compared to directly interacting with support staff. These insights can be valuable for refining the user experience.
Furthermore, the dashboard allows for real-time customization of support responses based on individual user data, a development that suggests a shift toward more personalized interactions. This focus on tailoring responses could foster a stronger sense of loyalty and satisfaction among users, which would be a positive outcome. Ultimately, this real-time dashboard acts as a continuous feedback loop for the entire support system. By collecting and analyzing user interactions, it helps identify areas for improvement, allowing the system to adapt and evolve to meet user expectations. This focus on constant improvement is critical as AI plays a larger role in enterprise support systems. While the results are promising, we must acknowledge that ongoing monitoring of these systems and attention to their limitations is critical to ensuring that they effectively meet user needs in the long run.
AI's Role in Streamlining Udemy's Enterprise Support Systems A Technical Analysis of Automated Response Mechanisms - Automated Skill Assessment System Maps Employee Learning Progress
Automated skill assessment systems are changing how organizations track employee learning. These systems use AI to personalize learning experiences, tailoring content to individual employee needs and adjusting the learning path as they progress. This personalized approach, coupled with automated feedback and grading, increases efficiency and reduces bias in evaluation processes. Essentially, the focus shifts to continuous learning and improvement. But, the incorporation of AI in this way raises questions. How much automation is ideal? When should human intervention be prioritized, particularly in complex training scenarios? These are critical questions given that the nature of work is shifting rapidly in conjunction with accelerating technological change. As jobs and skills evolve, automated skill assessment systems are at the core of a new model for employee skill development.
Automated skill assessment systems within Udemy's enterprise offerings have shown promise in mapping employee learning progress. They seem to be pretty accurate, with claims of over 90% precision in gauging skills. This real-time tracking of individual development allows organizations to get a better sense of where people are in their skill journeys and what they've mastered.
The inclusion of feedback loops is interesting. By providing immediate and tailored feedback, these systems can reportedly speed up learning by up to 35%. It creates a more responsive learning environment, which can be useful for learners who want quick insights into their progress.
Introducing elements of gamification, like leaderboards and badges, has seemingly led to a 50% increase in user engagement. This is a good sign that making learning more engaging and competitive can improve user motivation and participation. However, it's worth noting that this kind of engagement doesn't necessarily translate into deeper understanding.
These automated assessments can also pinpoint skill gaps with a stated precision of about 85%. This targeted identification of weaknesses allows companies to develop training programs that are more focused on specific areas, which should theoretically lead to better outcomes in terms of skill development. It's a sensible approach, but the '85%' accuracy needs to be critically evaluated to see how it performs in the real-world context of diverse employee populations.
Another notable aspect is the time saved through automation. Udemy reports a reduction in evaluation time of over 70%. This is appealing, as it frees up trainers and employees to spend more time on actual learning rather than administrative tasks. However, we need to consider the potential impact of this shift on the quality of the evaluation and the role of human interaction in ensuring fairness and accuracy.
The capacity for these systems to support diverse learning pathways is intriguing. They can supposedly generate personalized sequences of learning modules based on individual career goals, potentially increasing course completion rates by 40%. However, we need to explore if this leads to bias in outcomes, or if it disproportionately benefits some employee groups over others.
Beyond individual learning, these systems provide analytics that can offer insights across departments. This allows companies to identify skill trends that span multiple teams and collaborate better on training efforts. While this is potentially beneficial, we should be cautious of relying solely on automated insights without a careful consideration of the potential for bias in the data and analysis.
The incorporation of predictive analytics to anticipate future training needs is an ambitious development. Based on current performance trends, these systems claim about 80% accuracy in forecasting future training requirements. This capability could be really helpful in adjusting training programs proactively, but we should consider the risks of inaccurate predictions and potential bias in the data used for those predictions.
The inclusion of behavioral data from learner interactions is another feature worth exploring. This potentially creates a much richer picture of learning habits and preferences, which could be extremely valuable for designing better courses and materials. However, it's crucial to be mindful of privacy concerns and the potential for misuse of this data.
The adaptive nature of the learning framework is intriguing. The content changes dynamically based on the learner's performance, which might help reduce knowledge gaps and enhance retention rates. While the claimed 25% increase in retention is promising, this adaptive nature should be studied carefully for fairness and to see if it effectively benefits a wide range of learners.
In conclusion, automated skill assessment systems within Udemy's enterprise offerings provide a useful set of tools for understanding and improving employee learning. However, we need to remain aware of the potential limitations, especially regarding the potential for bias and the importance of human oversight in aspects like evaluation fairness and nuanced feedback. Continued research and careful monitoring are required to ensure that these systems are actually achieving their intended goals and promoting equitable and effective learning outcomes.
AI's Role in Streamlining Udemy's Enterprise Support Systems A Technical Analysis of Automated Response Mechanisms - Integration Of Support Data With Learning Management System Creates Unified Platform
Connecting support data with a learning management system (LMS) is creating a more unified and efficient educational platform. This integration allows for the smooth flow of information between different tools and systems within an organization, enhancing overall connectivity. By linking support resources directly to the learning environment, the gap between traditional education and modern support structures is narrowed, leading to a more integrated experience for both learners and educators.
A unified LMS platform can potentially reduce the complexity of managing multiple systems, streamlining operations and minimizing technical hurdles. It can also improve the consistency of the user experience, offering a more unified interface across various learning tools. This approach, in theory, could maximize the impact of educational initiatives by fostering a consistent and organized environment.
The incorporation of AI and machine learning into these integrated systems is showing early signs of creating even more personalized and dynamic learning experiences. This capability is important in a world where learning must be adaptive and responsive to the needs of individual users. However, this push toward more integrated platforms also raises important questions about user privacy and the potential for bias within these systems. It's critical that the design and development of these platforms are done thoughtfully and with a focus on equitable access to learning resources for everyone.
Connecting support data with a learning management system (LMS) aims to create a more unified platform, but this integration brings about a new set of complexities. For example, combining different datasets can lead to difficulties in smoothly handling data requests, especially when trying to get data for real-time insights. There can be performance slowdowns and unexpected problems that wouldn't necessarily happen if the data were siloed.
However, combining support interactions with the learning progress tracked in the LMS offers a lot of potential. We can gain a better understanding of how users interact with learning materials and what might be leading to more frequent support requests. Seeing how particular courses or topics are correlated with a higher volume of support can be invaluable for adjusting and refining the training content to be more effective and address issues proactively.
This integration also enhances the ability to predict future support needs. With all this data consolidated, it becomes easier to see historical trends and try to forecast periods of high support demand. Having some ability to see ahead of time when more support staff might be needed can make a huge difference in the effectiveness of the support team.
However, bringing support data and learner data together creates new challenges related to data security and privacy. We need to make sure we're adhering to privacy regulations like GDPR, since we're dealing with very sensitive information about learners. Implementing strict measures to keep the data secure will be absolutely necessary.
Furthermore, even though we're incorporating automated systems that look at things like the sentiment of support requests, these methods don't always give accurate results. The performance of sentiment analysis can change greatly depending on the language used and the specific context of a support ticket. Incorrectly identifying the emotional tone of a support request could cause us to misprioritize urgent cases, potentially harming the user experience.
There's always a trade-off between efficiency and quality. While using automation for support functions can lead to cost savings and improve efficiency, there's also a risk of the support quality declining. Certain situations require a uniquely human touch to handle complex problems or deal with delicate interpersonal matters. We can't lose sight of the fact that even if automated support is incredibly useful, it shouldn't entirely replace human support agents.
With a combined support and learning platform, we'll be able to make faster decisions with real-time data insights. However, maintaining accurate and up-to-date data in a complex system is vital. Having a dashboard that combines different datasets is great, but it's only truly valuable if the data driving it is accurate. If it's not, we risk making flawed decisions, which could cause harm.
The link between support queries and learning progress can be quite revealing. By understanding what parts of a course are leading to a greater need for support, companies can tailor training programs to address the recurring knowledge gaps that cause frequent support requests.
We'll need to be very mindful of cultural context when providing support in a globalized world. It's not just about translating language; we need to truly understand the cultural sensitivities around the language. Creating a positive and meaningful experience for users across different cultures can require adjustments beyond straightforward translations.
Integrating these two systems opens a channel for continuous improvement. With the ability to track support inquiries linked to specific learning modules, we can iterate on the training content itself. If we see a trend where certain learning areas constantly require external support, we can refine the associated content. The feedback loop from the support team can improve training materials, making them more effective and easier to understand. However, continuous assessment of these changes is crucial to ensure that we're achieving the desired outcomes.
Create AI-powered tutorials effortlessly: Learn, teach, and share knowledge with our intuitive platform. (Get started for free)
More Posts from aitutorialmaker.com: