Analyzing 7 Key Metrics in Random Football Career Generators for AI-Driven Simulations
Analyzing 7 Key Metrics in Random Football Career Generators for AI-Driven Simulations - Player Performance Tracking Using AI-Powered Wearables
Artificial intelligence integrated into wearable technology has significantly altered how we track athlete performance. By meticulously analyzing specific sports metrics and individual data, these wearables enable personalized training plans. In football, for example, key performance indicators like the rate of successful passes and the percentage of won duels are meticulously tracked. This data allows coaches and players to precisely identify areas demanding improvement. The ability to analyze data in real-time not only refines training methods but also aids in preventing injuries and facilitating rehabilitation, offering actionable recommendations for athlete management. However, it's important to acknowledge a potential downside: over-reliance on the data provided by these devices. This could lead to a diminished emphasis on intuitive coaching methods and traditional insights. The ongoing development of AI in sports performance tracking undoubtedly brings exciting possibilities, yet it's crucial to carefully consider the associated challenges.
AI-powered wearables are becoming increasingly sophisticated in their ability to monitor player performance. They go beyond simple metrics by tracking a vast amount of data, sometimes exceeding 2,000 data points per second, enabling a detailed analysis of a player's movement mechanics. This level of granularity allows coaches and trainers to understand how efficiently athletes are moving and identify potential injury risks in real-time. It's not just about speed and distance covered; these devices can also provide insights into physiological factors such as heart rate variability, giving a clearer picture of an athlete's readiness for training and how quickly they are recovering.
This wealth of data, processed by advanced algorithms, can generate predictive analytics. This capability is fascinating, as it offers a glimpse into future performance trends. Coaches can potentially foresee dips in performance and tailor strategies accordingly. There's also a push towards machine learning in these systems. The idea is that the more data these algorithms are fed, the better they become at adapting to individual athlete behavior and identifying performance patterns. This customization could lead to even more targeted and effective training programs over time.
The potential impact on game strategy is substantial. Real-time data during a match could be used to inform tactical adjustments. Coaches could make decisions based on live performance indicators instead of waiting for post-game analysis. This could offer a significant advantage in competitive situations. Beyond performance enhancements, wearables are dramatically reshaping how player health is monitored. Constant data tracking enables early identification of fatigue or stress – early warnings that are crucial for injury prevention.
Some of the advanced analytics techniques employed include kinematic analysis. This focuses on the way energy is transferred throughout a player's body during movement, aiming to optimize both performance and the mechanics of their technique. The data collected isn't just for coaches and team management either. The information can be shared with medical staff, promoting collaboration across disciplines to improve an athlete's overall wellbeing.
Recent developments in wearables are exploring the use of augmented reality to visualize data during training sessions. It's interesting to think of players experiencing real-time performance feedback visually. This could have a huge impact on how athletes learn and improve their skills. It's also notable that these technologies are not just for professional athletes. Wearables are becoming more affordable and accessible, giving amateur players access to data and analytics that were previously out of reach. This could have a positive impact on grassroots football development, making advanced performance analysis more democratic. While there are still questions about the long-term implications of this kind of technology and its potential biases, it is certainly changing the landscape of football and athlete development.
Analyzing 7 Key Metrics in Random Football Career Generators for AI-Driven Simulations - Duels Won Percentage as a Key Performance Indicator
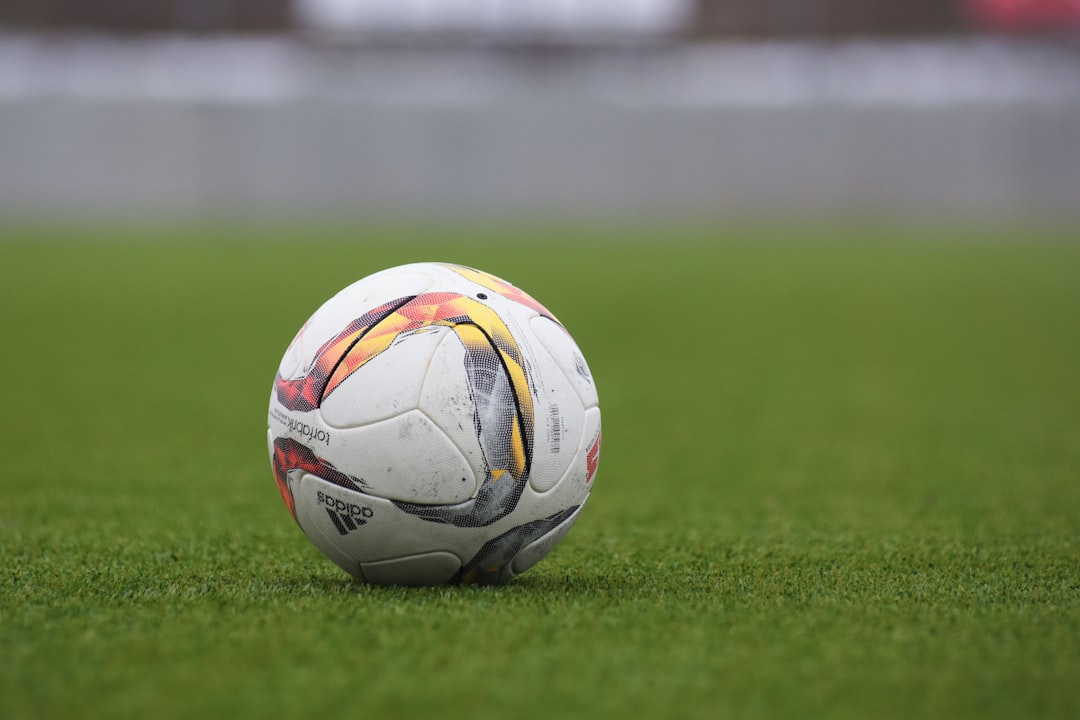
Within the realm of football analytics, duels won percentage emerges as a crucial key performance indicator (KPI) for gauging a player's effectiveness. It offers a direct window into a player's ability to succeed in individual battles, which can significantly impact the course of a match and the overall performance of their team. While metrics like pass completion rate are commonly used, duels won percentage emphasizes the value of a player's determination and skill under pressure. In an environment where teams are increasingly reliant on data-driven strategies, recognizing the intricacies of duels won can be a key differentiator in designing tailored player development plans and enhancing team performance.
It's important to note that relying solely on statistical indicators like duels won percentage can potentially overshadow the more qualitative aspects of the sport. While data offers valuable insights, it shouldn't entirely replace a nuanced understanding of how the game unfolds and the interplay of tactical and strategic decisions on the field. Coaches and analysts should strive for a balanced approach that integrates quantitative data analysis with their own observations and experiences. Ultimately, the success of any performance indicator depends on its careful interpretation and application within a broader framework of understanding how football is actually played.
1. The percentage of duels won, often overlooked, can be surprisingly insightful when evaluating a player's overall impact on the game. Research suggests that players who frequently win duels contribute significantly to maintaining possession and bolstering the team's defensive strength, making this a valuable indicator for judging both attacking and defending players.
2. Intriguingly, the importance of duels won seems to vary depending on a player's position. For example, defenders may excel in aerial duels, while midfielders might be more successful in ground duels, suggesting that the role a player fulfills within the team influences how we should interpret their duel win rates.
3. Data analysis hints at a connection between a player's duels won percentage and their career trajectory. It appears that players with a duels won percentage above 60% tend to have higher contract values and enjoy longer careers, indicating that individual battles contribute not just to the tactics of the game, but also to a player's marketability and potential earning power.
4. The context in which a duel occurs can be just as significant as the win itself. Winning a duel in a crucial area of the field, like the final third or the defensive third, can have very different strategic implications. Success in the final third might be more directly linked to scoring chances, while winning duels in the defensive third is more likely tied to preventing the opposition from scoring.
5. One interesting finding is that players with a high duels won percentage often display mental toughness and physical resilience, characteristics often linked to long and successful careers in sports. Studies have indicated that the ability to bounce back after losing a duel can positively impact overall performance.
6. Traditional scouting approaches might not adequately capture the nuanced information that a duels won percentage can provide. Advanced tracking technologies reveal that a high duels won percentage can be misleading if a player is not engaging in many duels. This highlights the importance of considering the frequency of duels alongside the percentage won.
7. A player's ability to win duels can be significantly affected by injuries. Data suggests that players recovering from injury often show lower duel win percentages, raising questions about relying solely on this metric to determine a player's potential.
8. The duels won percentage can potentially offer clues about a team's overall success. Historical data shows that teams with higher collective win rates in duels tend to perform better in the league, suggesting that coaching staff could benefit from making duel-winning a key part of their training and strategy.
9. Some have voiced concerns that an overemphasis on duels won percentage could lead to a more aggressive and perhaps overly physical style of play, potentially increasing the risk of fouls and impacting the flow of the game. Finding a balance between achieving positive performance metrics and maintaining a fair and enjoyable game is important.
10. The emergence of AI-driven simulations has started to influence the way we think about duels won percentages. By analyzing game footage and considering the decision-making processes that lead to duel outcomes, these simulations offer a more complete picture of player performance than simply looking at win rates. These deeper insights could enhance our understanding of player analytics.
Analyzing 7 Key Metrics in Random Football Career Generators for AI-Driven Simulations - AI-Driven Tactical Decision Making in Virtual Football Scenarios
Within the realm of virtual football, AI is increasingly shaping tactical decision-making, pushing the boundaries of sports analytics. AI systems, like those under development, are leveraging techniques like geometric deep learning to analyze game patterns and provide real-time insights to coaches. This can range from predicting player movements during set pieces to identifying tactical trends in opponents' play. The collaboration between developers and clubs like Liverpool FC emphasizes the importance of blending expert knowledge with cutting-edge AI capabilities to make these tools effective. While these AI systems can enhance tactical awareness and potentially provide a significant edge in game planning, the complexity of football tactics presents a continuous challenge for AI development. We must remember that even the most sophisticated algorithms can't fully encapsulate the intricate strategic thought processes and instinctual decisions that human coaches bring to the sport. The field is still developing, and there is a need for continuous refinement to improve the models' accuracy and ability to adapt to diverse playing styles and scenarios. Though potentially transformative, we must remain mindful of the limitations and ensure that these tools augment rather than replace the insightful expertise of human coaches.
AI systems are increasingly being used to help football coaches make tactical decisions in virtual environments. These systems, often using a technique called geometric deep learning, can analyze game situations and provide insights that are both comprehensive and timely. For example, some AI systems can predict which player will first touch the ball after a corner kick, highlighting the potential for AI to identify tactical patterns that might otherwise go unnoticed.
Interestingly, some of these AI systems have been developed in collaboration with professional clubs, such as Liverpool FC, which emphasizes the importance of real-world expertise in developing effective tools. The goal is to give coaches an edge by helping them understand the tactics used by opponents. This is a challenging problem because it requires finding algorithmic solutions to recognizing complex tactical patterns within the game.
These systems are not just producing raw data; they're designed to produce actionable suggestions. In some instances, the suggestions provided by AI have been judged by human experts to be indistinguishable from the tactics employed by professionals in real-world practice. Furthermore, these suggestions can be quite accurate. Some research indicates a 90% accuracy in selecting appropriate corner kick setups based on AI-driven analysis.
This development of AI-driven tactical decision-making represents a major shift in how football might be analyzed and coached. It's essentially applying data-driven insights to empower coaches, with the potential to improve their decision-making during both matches and training sessions. It's part of a broader trend we're seeing in sports analytics, where AI technologies are being incorporated into coaching and strategic planning.
While promising, it's important to remember that there are still challenges in modeling complex football tactics using algorithms. This highlights the need for continuing research and innovation to improve the capabilities of AI within the sport. The complexity of the game – with its tactical variations, player interactions, and unpredictable elements – requires ongoing development to ensure these tools can capture the nuances of the sport.
Analyzing 7 Key Metrics in Random Football Career Generators for AI-Driven Simulations - Personalized Training Programs Based on AI-Generated Metrics
AI-generated metrics are increasingly used to create personalized training programs for athletes, potentially transforming how they develop. These programs analyze an athlete's performance data and pinpoint areas where improvement is needed, leading to individually tailored training plans. This tailored approach allows for real-time adjustments to training, making learning more efficient and effective. AI also helps simplify complex training materials into more manageable chunks, making it easier for athletes to grasp new skills and concepts. Moreover, AI can analyze past performance to suggest specific training interventions, moving beyond the standard coaching methods. However, while AI offers significant benefits, maintaining a balanced approach that integrates technology with the expertise and instincts of coaches is crucial. Relying too heavily on AI-driven training could neglect valuable aspects of a coach's intuition and knowledge. The aim is to leverage these technologies to enhance athlete development, not to replace the fundamental roles of human coaching and understanding of the game.
AI can analyze a player's progress and adjust training plans on the fly, based on metrics it generates in real time. This adaptive approach potentially speeds up skill development by allowing for immediate adjustments between training sessions.
By crunching biomechanical data, sophisticated algorithms can pinpoint inefficiencies in movement and suggest modifications to enhance stability and performance, ultimately aiming to decrease the odds of injuries.
It's interesting that these AI training tools aren't just for the pros anymore. They're making their way into amateur sports, meaning that a wider range of athletes can benefit from performance analysis once exclusive to elite levels.
One of the promising applications of AI-generated metrics is injury risk prediction. By processing historical data on injuries and their connection to training, these systems can suggest athlete-specific preventive measures.
The combination of personalized training plans and wearables might lead to increased engagement from athletes. If they see improvements and receive prompt feedback, it could make them more motivated to follow their training schedules.
Not only can AI systems dissect physical performance, but some can also gauge psychological factors affecting an athlete's ability, such as fatigue and stress levels. This wider perspective on performance could make for more comprehensive player development programs.
There's a potential drawback though: over-reliance on the data generated by AI. While data provides valuable specifics, it might overshadow the insights of a human coach—experience, instincts, and interpersonal skills. A balance between AI and traditional coaching is probably key.
Machine learning lets AI evolve alongside athletes. By continuously refining training programs based on fresh data, these AI systems potentially become even more effective over time.
The scope of AI-driven personalized training goes beyond just exercise intensity; it can factor in recovery metrics, hydration, and dietary requirements. It's a more comprehensive approach, considering the whole picture.
While AI-driven metrics offer a lot of insights, they might not be equally effective across all sports and athletes. Customizability remains essential; using the same approach across the board might not align with the unique demands of various athletes and positions.
Analyzing 7 Key Metrics in Random Football Career Generators for AI-Driven Simulations - Geometric Deep Learning Applications in Football Strategy Simulation
Geometric deep learning is emerging as a powerful tool in football strategy simulation, particularly for refining tactical decision-making. Systems like TacticAI, a joint project of Google DeepMind and Liverpool FC, are leveraging this approach to analyze historical game data, focusing on aspects like corner kick strategies. By examining player positioning and likely outcomes, these AI systems aim to provide coaches with valuable insights. A key benefit of geometric deep learning in this context is its ability to incorporate the symmetries of the football field, thereby simplifying the analysis and reducing the need for excessive data manipulation. This increased data efficiency allows AI to efficiently identify crucial patterns in opposing teams' tactics, which can be invaluable for coaches looking to gain a competitive edge. While promising, it's important to recognize the limits of current AI applications in football. These models, while increasingly sophisticated, still struggle to capture the nuanced human intuition and experience that coaches bring to the game. Nevertheless, the potential for AI to augment decision-making during games and training sessions is significant, with implications potentially extending to areas beyond sport, such as military simulations where movement patterns and tactical maneuvers are crucial. As this technology advances, its capacity to analyze strategic patterns and predict outcomes will likely play a growing role in both virtual and real-world football scenarios.
Geometric deep learning presents a fresh perspective on analyzing the spatial dynamics of a football pitch, enabling AI models to grasp player interactions in a way that traditional data methods often miss. This includes understanding how the positioning of players impacts match outcomes, potentially leading to more nuanced tactical analysis.
One interesting application is in forecasting formations and the subsequent shifts in player actions during a game. By reviewing past data, AI can simulate possible formations that maximize team effectiveness against specific opponents, potentially giving teams an advantage when planning for matches.
Research has shown that relationship-based analysis using geometric deep learning can significantly boost player recruitment strategies. Teams are now utilizing these insights to assess not just individual abilities, but also how well a prospective player might integrate into the team's structure tactically.
The inclusion of geometric deep learning allows for real-time tactical adjustments during games. Coaches can utilize algorithms to analyze player movement as it unfolds, helping them make informed choices, such as changing formations or swapping out players based on live data instead of historical trends.
Geometry-based models can highlight "hot zones" on the field, pinpointing areas where teams could capitalize on weaknesses in their opponents' defenses. This spatial analytical approach can aid coaches in creating plays that focus on high-value areas, boosting the chance of scoring.
Sophisticated simulations, powered by these techniques, can produce training scenarios specifically designed for particular opponents. This allows players to practice in game-like situations, focusing on learning how to counter opposing tactics and potentially enhancing their in-game decision-making abilities.
A key challenge lies in interpreting the model's output. The intricate nature of player behavior can lead to overfitting in simulations, resulting in insights that might not necessarily translate effectively into actual game situations. A careful balance between data-driven insights and human intuition is crucial.
The feedback loop generated by geometric deep learning enables continual improvement of tactical strategies. As more match data fuels the model, it can evolve, continually refining its ability to predict successful tactics based on previously observed plays.
Teams that use these sophisticated analytical approaches often report an improvement in player comprehension of their roles within various tactical frameworks. This heightened awareness could lead to more unified team performances, as players get better at anticipating each other's movements.
There's a growing concern about overly relying on AI models to dictate tactical choices. While informative, these systems can't fully capture the emotional and psychological aspects of player performance, highlighting the need for a balanced approach that combines analytics with human judgment on the field.
More Posts from aitutorialmaker.com: