Decoding Slope in AP Stats Computer Output Key Insights for 2024
Decoding Slope in AP Stats Computer Output Key Insights for 2024 - Interpreting the Coefficient of the Explanatory Variable
Understanding the coefficient tied to the explanatory variable is fundamental in linear regression. The slope (b) quantifies the predicted change in the response variable for every single-unit alteration in the explanatory variable. This gives us insight into the nature of their relationship – whether it's a positive or negative association. While the intercept provides the predicted response when all explanatory variables are zero, its real-world meaning can be questionable and needs to be evaluated in context. It's vital to not only interpret the slope's implications but also take into account the scatter or variability present within the data. A high degree of variability signifies that the regression model might not be closely aligned with the actual observations, complicating our interpretation. In essence, interpreting linear regression requires analyzing both the slope's message and the data's spread within the particular scenario under examination.
1. The coefficient associated with an explanatory variable in a regression model reveals the predicted change in the response variable for every single unit increase in that explanatory variable, while assuming all other factors remain constant. This provides a way to pinpoint the influence of specific elements within intricate systems, which is invaluable for research efforts.
2. A positive coefficient suggests a direct relationship; as the explanatory variable rises, so does the response variable. Conversely, a negative coefficient signals an inverse relationship, prompting us to thoroughly examine the underlying mechanisms driving the observed trend.
3. The magnitude of the coefficient is not just about direction but also the strength of the association. This is particularly critical in a research context where minor changes can have major downstream consequences on the results of a study.
4. Interpreting coefficients can become tricky when explanatory variables are related to each other (multicollinearity). This can distort the actual influence of each variable, potentially leading to inaccurate conclusions based solely on the statistical output. This poses a concern when aiming for dependable results.
5. Things get more complex when working with non-linear models. Researchers need to account for how shifts in the explanatory variable impact the response variable differently throughout its range. This may necessitate further investigation beyond just focusing on the coefficient alone.
6. Coefficient significance can fluctuate based on the quantity of data points and the spread of the data. Researchers should examine each coefficient's statistical significance. Small datasets might produce imprecise estimates that may not accurately reflect larger trends.
7. The units used for both the explanatory and response variables can considerably influence the coefficient's interpretation. Researchers should either standardize the units or convert them to facilitate meaningful comparisons, especially when dealing with variables using varying scales.
8. Interaction terms can be added to the model to explore how multiple explanatory variables collectively affect the response variable. The coefficients for these interactions reveal intricate connections which can be insightful when engineering or optimizing complex systems.
9. When utilizing dummy variables, which represent categories, coefficients unveil the relative impact of one category compared to a baseline. This is crucial when researchers are trying to understand the influence of categorical predictors in their models.
10. It's vital to acknowledge that statistical coefficients, while informative, don't inherently prove cause-and-effect relationships. Researchers must blend this statistical information with subject-matter expertise and supplementary data to make well-informed decisions during the research process.
Decoding Slope in AP Stats Computer Output Key Insights for 2024 - Understanding the Expected Change in Response Variable
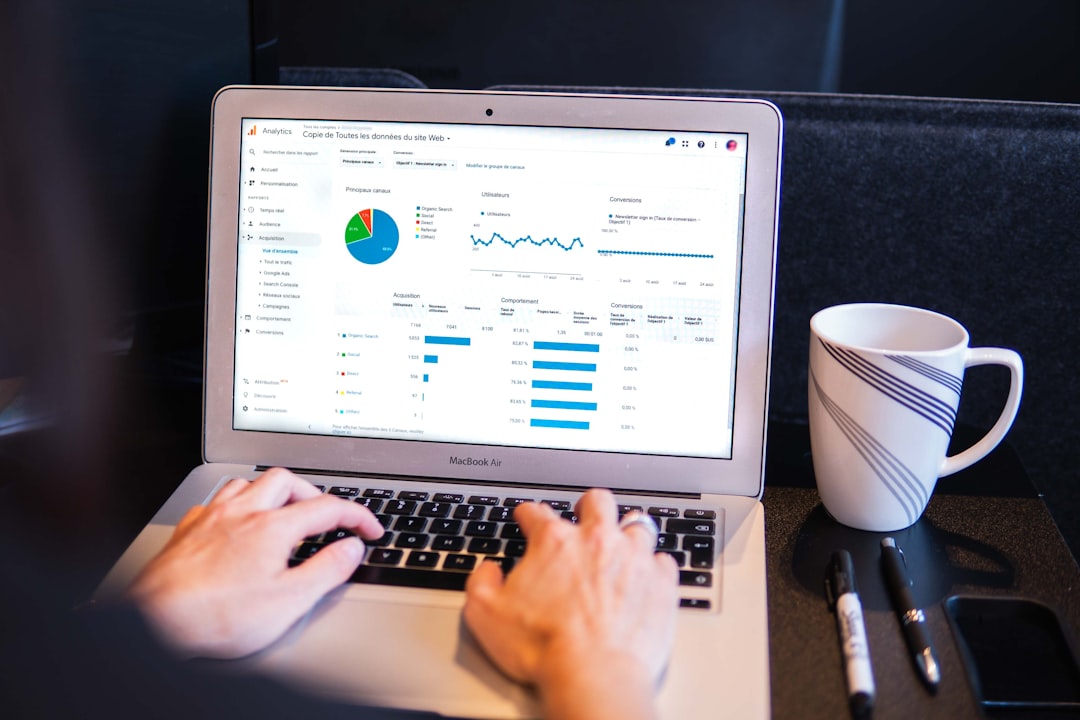
Understanding the expected change in the response variable is fundamental when interpreting the results of a linear regression. The slope, a key component of the regression line, represents the predicted change in the response variable for every one-unit change in the explanatory variable, assuming all other variables remain constant. This provides valuable insights into the relationship between the two variables, revealing whether it's positive (as one increases, so does the other) or negative (as one increases, the other decreases).
However, interpreting this anticipated change isn't always straightforward. The presence of influential data points, such as outliers, can skew the results. Furthermore, if explanatory variables are interconnected (multicollinearity), it can become challenging to isolate the true impact of each individual variable. The inherent variability or spread within the data also plays a significant role in the reliability of these predicted changes. Therefore, researchers must exercise caution when interpreting the slope and the associated expected changes in the response variable, considering not just the statistical output but also the larger context of their research and the limitations of the data. A balanced approach, encompassing both the statistical analysis and a deeper understanding of the subject matter, is needed for drawing valid conclusions from regression models.
1. The anticipated shift in the response variable is fundamentally linked to the assumption of a linear relationship in regression analysis. Any departure from this linearity can substantially distort predictions, thus, engineers implementing these models must diligently check for linearity to ensure reliable outcomes.
2. When interpreting the expected change, it's vital to consider both the statistical and practical implications of the coefficients. A statistically significant result may not necessarily translate into a meaningful real-world change, emphasizing the need for contextualized interpretations.
3. The confidence intervals surrounding the coefficient estimates provide key insights into the inherent uncertainty of predictions. Wider confidence intervals signify greater uncertainty, suggesting the predicted change might not be as reliable as a more precise estimate.
4. In certain instances, the anticipated change can be impacted by outliers, which can disproportionately influence the slope of the regression line. Recognizing and addressing these outliers is essential to avoid skewed interpretations.
5. When comparing multiple regression models, the predicted changes in the response variable can illuminate which explanatory variables play significant roles. This comparative analysis can assist engineers in prioritizing actions that produce the most significant improvements.
6. The predicted change relies on the assumption of constant error variance (homoscedasticity), meaning the variability of residuals is consistent across all levels of the explanatory variable. Violations of this assumption can result in inefficient estimates and misleading insights into the anticipated change.
7. In complex systems, interaction effects often necessitate more advanced modeling techniques. The predicted change in the response variable can vary substantially when considering these interactions, requiring a comprehensive perspective on the involved variables.
8. The expected change is not fixed; it can vary depending on the specific context of the model's application. Different datasets or operational circumstances may lead to varying interpretations of the same coefficients, making it essential to adapt predictions accordingly.
9. Non-linear transformations of explanatory variables can also influence the expected change in the response variable, demanding a thorough understanding of the underlying relationship to prevent misinterpretations.
10. Ultimately, while the expected change offers predictive capabilities, it should not be viewed in isolation. Ongoing feedback loops using real-world data can help refine models, ensuring that the expected changes remain relevant and accurate over time.
Decoding Slope in AP Stats Computer Output Key Insights for 2024 - Locating the Y-Intercept in Computer Output
When examining computer output from a linear regression analysis, understanding the y-intercept is vital. The y-intercept, often identified as "CONSTANT" in the output, represents the predicted value of the dependent variable when all independent variables are zero. It's an essential part of the prediction equation (y = a + bx), where 'a' is the y-intercept and 'b' is the slope. However, its practical meaning can be unclear, particularly if having all independent variables at zero doesn't make sense in the real world. It's important to interpret the y-intercept in conjunction with the slope to gain a complete understanding of the relationship between variables. Interpreting the y-intercept needs a careful approach because the variability of the data and the underlying assumptions of the model can affect its relevance. By critically examining the y-intercept within the broader context, we can derive more meaningful conclusions from the regression results.
1. The y-intercept, often labeled "CONSTANT" in software outputs, represents the predicted value of the response variable when all explanatory variables are zero. While sometimes insightful, its practical meaning can be unclear if zero is outside the observed data range. For example, in a model predicting crop yield based on fertilizer application, the y-intercept would be the predicted yield with zero fertilizer—a scenario potentially irrelevant in practice.
2. In many real-world cases, the y-intercept may not have a meaningful interpretation. This can be the case when zero values for the explanatory variables don't align with the system under study. If the independent variables are always positive, such as in studies involving time or temperature, interpreting the intercept at zero may not be useful. It becomes essential to critically evaluate the context of the regression model rather than blindly assuming a real-world application of the y-intercept.
3. The y-intercept can be influential in predictions, especially when working with smaller datasets. A subtle shift in the intercept can substantially alter predicted values of the response variable, emphasizing the need to carefully weigh its impact when making conclusions about the model's predictive ability. This is something to think about, especially when you're extrapolating beyond the range of your collected data.
4. When using transformations on the data, such as taking logarithms or fitting polynomials, interpreting the y-intercept becomes more complex. While the intercept might have a clear meaning in the transformed space, converting it back to the original scale requires careful consideration of how the transformation affects the baseline. It's not always a simple matter of reverse-engineering the transform.
5. In multiple regression, if some explanatory variables aren't included in the model, the y-intercept absorbs their influence. This "absorption" can skew the interpretation if you don't consider how omitted variables might affect the predicted response. We need to think about the effects of things we didn't measure, which can impact our conclusions.
6. Depending on the chosen modeling technique, especially in cases with poor data fit or diverse data conditions, the estimated y-intercept can differ. This variability in estimates reminds us that the methodological approach used influences the y-intercept and, subsequently, the overall model. One method may fit data better than another, and this will alter the estimated intercept.
7. While the y-intercept's statistical significance can be tested like other coefficients, it's not always inherently meaningful. Sometimes, a statistically significant y-intercept is just a statistical fluke, with little practical value in the context of the research. This is an issue that makes interpreting results less straightforward.
8. With categorical explanatory variables, the y-intercept often serves as a reference point for comparison against a baseline category. While this provides context, it adds layers of complexity to the interpretation, particularly when the baseline category itself has variability. It's essential to understand what each category represents to understand the intercept's role in comparisons.
9. In time series data, seasonality or time-dependent factors can cloud the interpretation of the y-intercept. Fluctuations over time can lead to a y-intercept that doesn't represent the average behavior of the system well. This can complicate the analysis and requires adjusting the model to better account for these dynamic patterns in the data.
10. The y-intercept is a critical component of the regression equation, but overemphasizing its interpretation can distract from understanding the whole model. We should be careful to balance its interpretation with the slope and other model diagnostics to draw sound conclusions about the data. This is important when understanding how the model's various parts relate to each other and their combined significance in the overall model.
Decoding Slope in AP Stats Computer Output Key Insights for 2024 - Formulating the Regression Equation from Output
Formulating the regression equation from statistical software output is a crucial step in deciphering the relationship between variables in linear regression. The equation typically follows the familiar form \( y = a + bx \), where \( a \) represents the y-intercept and \( b \) stands for the slope. The slope, or coefficient, communicates the anticipated change in the response variable \( y \) for every one-unit shift in the explanatory variable \( x \), providing a quantitative understanding of their link. The statistical output usually also provides metrics like \( R^2 \) and adjusted \( R^2 \), which offer a measure of how well the regression model captures the relationship between the variables. However, it's important to acknowledge that interpreting the equation and associated statistics needs careful consideration of the surrounding context. For example, the presence of unusual data points (outliers) or intertwined explanatory variables (multicollinearity) can influence the accuracy and trustworthiness of the regression equation and its related measures of fit.
1. The foundation of any regression equation lies within the constant term, which provides the predicted value of the response when all explanatory variables are zero. However, this interpretation becomes suspect when zero falls outside the observed data range, leading to a less meaningful interpretation. We need to always critically evaluate whether this "zero" point has relevance to the study.
2. The coefficients found in a regression equation might not always capture the most crucial factors governing the response variable. Careful validation of chosen factors is essential to ensure they truly represent the underlying system dynamics. If we're studying a complex system, simply plugging in variables without careful thought might not lead to useful predictions.
3. The magnitude of coefficients can be significantly impacted by alterations in dataset size or characteristics. This emphasizes the importance of constant data evaluation, even in early stages, as the representativeness of the sample can profoundly alter regression outcomes. This reminds us that our models are only as good as the data they're trained on.
4. The presence of interactions among variables introduces complexity to the regression model. When variables influence each other, relying solely on linear terms can obscure important information. Further investigation into interaction terms is crucial for a more complete understanding of the system. This indicates that, if variables interact, there can be non-linear dynamics that simple linear models can miss.
5. The assumptions underpinning linear regression models, such as consistent error variance (homoscedasticity) and independence of errors, are crucial for accurate results. If we violate these assumptions, we may get misleading coefficients that may lead us to faulty predictions. In some cases, this might mean needing to transform variables to satisfy model assumptions.
6. Uncertainty or error in response variable measurements can distort the regression equation. When the response is unreliable, the estimated coefficients might not reflect the true relationships. This ultimately affects the model's accuracy in predicting future outcomes. When working with experimental data, carefully considering the accuracy and error associated with measurements is paramount.
7. The standard error associated with each coefficient provides a measure of its precision. Lower standard errors indicate more reliable estimates. This allows for more confident decision-making based on the model's predictions. We gain confidence in our estimates when they have smaller associated error.
8. The scale of the explanatory variables can influence the interpretation of coefficients. Normalizing or standardizing variables can improve comparisons and interpretations, especially when working with variables on vastly different scales. This can be an important pre-processing step when the variables being studied have differing units of measure.
9. When explanatory variables are interconnected (multicollinearity), standard errors tend to become inflated. This makes it challenging to isolate the unique contribution of each variable. Addressing multicollinearity through methods such as variable selection or dimensionality reduction can provide clearer insights. Understanding this can help in making sure we select the right set of variables in our models.
10. While regression equations offer a powerful framework for understanding systems, it’s critical to recognize their limitations. Engineers need to maintain a critical perspective and constantly evaluate the model's relevance to the real-world system. Models are useful approximations of reality, and it's crucial to remember that they don't perfectly represent the real-world complexity.
Decoding Slope in AP Stats Computer Output Key Insights for 2024 - Assessing Data Variability and Its Impact on Predictions
When it comes to making predictions, particularly within the realm of regression analysis, understanding data variability is essential. The level of variability essentially reflects how spread out the data points are. High variability suggests that predictions made using a model may be less reliable or precise. Conversely, low variability indicates a closer alignment between predictions and actual observations, implying greater confidence in the model. We have several ways to measure and quantify this spread—including the range, interquartile range, variance, and standard deviation—each offering a distinct perspective on the data's distribution. In the context of predictive modeling, notably in areas like slope estimation, higher variability can impact the necessary sample size to attain reliable predictions. This highlights the significance of thoroughly assessing both data quality and its overall distribution. Ultimately, appreciating data variability isn't just a matter of statistical practice; it directly influences the precision and how well we can interpret our predictions within intricate systems.
1. The extent to which data points scatter around a central tendency, known as variability, significantly influences the trustworthiness of predictions. When data shows a wide spread, the confidence in the estimated coefficients diminishes, potentially leading to less reliable model outcomes. Engineers should regularly assess this variability to ensure they're building robust prediction models.
2. While the average (mean) of data is informative, it's equally important to consider the spread of the data, often measured by the range or standard deviation. If the standard deviation is substantial relative to the mean, it indicates a high level of dispersion. This wide spread can considerably harm the validity of predictions, particularly when fitting linear models to the data.
3. In regression models, understanding the presence of heteroscedasticity—a situation where the variation of the residuals is not consistent across the different levels of the explanatory variable—is crucial. Heteroscedasticity is a red flag that the model might not be fitting the data well, possibly producing imprecise coefficient estimates.
4. Analyzing the residuals, which are the differences between the predicted and observed values, provides further insight into data variability. Ideally, residuals should exhibit randomness, suggesting that the model has captured the underlying patterns in the data effectively. However, if we see consistent patterns in the residuals, it signals that the model might need further refinement.
5. Outliers, which are data points that deviate significantly from the rest of the data, can exert a disproportionate influence on coefficient estimates, underscoring the importance of understanding data variability. Identifying and, if appropriate, adjusting for outliers can contribute to more reliable predictions.
6. The size of the dataset used to build a model interacts with variability in a meaningful way. Using a larger dataset often leads to more reliable coefficient estimates because the standard error associated with the estimates decreases. This highlights the significance of ensuring that the dataset used for model building is appropriately sized and representative.
7. When working with categorical predictors, variability within the categories can make it difficult to clearly see the relationships in the data. If categories have significantly different sizes or variances, it can lead to skewed coefficient estimates. Therefore, careful scrutiny of the data is crucial for robust interpretations of models involving categorical variables.
8. A common oversight in predictive modeling is the effect of multicollinearity (when predictor variables are highly correlated) on data variability. Multicollinearity can inflate the standard errors of the estimated coefficients, diminishing the statistical power to detect meaningful relationships. Employing regularization techniques can help mitigate multicollinearity, leading to more interpretable models.
9. Many regression models assume that the residuals follow a normal distribution. When this assumption is not met, it might suggest that the model doesn't fully capture the variability in the data or that there are other issues with model specification. Conducting formal normality tests can help determine whether the model adequately describes the data.
10. Ultimately, the implications of variability extend beyond mere statistical considerations. By digging deeper into the underlying reasons for data variability—whether it's measurement errors, external influences, or intrinsic aspects of the system being studied— engineers can refine their models to enhance prediction accuracy and improve overall operational effectiveness.
Decoding Slope in AP Stats Computer Output Key Insights for 2024 - Evaluating Model Fit Using the Correlation Coefficient
When assessing how well a regression model fits the data, the correlation coefficient plays a key role. It quantifies the strength and direction of the linear relationship between the independent and dependent variables. A strong correlation, reflected in a coefficient closer to 1 or -1, suggests that the model's predictions are likely to be more accurate and closely aligned with the actual observations. However, just using the correlation coefficient can be deceptive. It fails to address other crucial aspects, like the existence of unusual data points (outliers) or interdependencies among predictor variables (multicollinearity). These factors can distort the real nature of the relationships we're trying to uncover. For a complete picture of how well the model fits, it's essential to also conduct a broader set of diagnostics. This involves examining the residuals (differences between predicted and actual values), considering measures of overall model fit like R-squared, and verifying if fundamental assumptions of the model are being met (like constant variance or homoscedasticity). Through this comprehensive approach, researchers can navigate potential hidden complexities and arrive at more reliable interpretations of the regression model's results.
### Evaluating Model Fit Using the Correlation Coefficient - Surprising Facts
The correlation coefficient, usually represented as \( r \), serves as a gauge of both the strength and direction of a linear relationship between two variables in regression analysis. It ranges from -1 to 1, with values close to 0 implying a weak connection. One surprising thing is that even a seemingly moderate correlation can result in decent predictive capability, contingent on the specifics of the variables.
However, the correlation coefficient has its limitations. It's unable to capture nonlinear relationships, which can lead to mistaken interpretations. For instance, a strong, yet nonlinear, connection might still show a low \( r \) value, potentially hiding valuable patterns.
Furthermore, the correlation coefficient's magnitude is significantly affected by the number of data points. A small dataset can lead to artificially inflated or deflated values of \( r \), highlighting the necessity of having enough data for a trustworthy correlation estimate. It's crucial to remember, as is often stated, that correlation doesn't demonstrate causation. Factors like lurking variables can create false correlations, making interpretation more complex.
The presence of outliers can significantly distort the correlation coefficient, leading to skewed results and possibly incorrect conclusions about the relationship between variables. Therefore, a thorough examination of the data is important before relying on the correlation value. Also, applying transformations to variables, such as logarithms, can notably alter the correlation coefficient. This is particularly crucial when working with non-normally distributed data, signifying the need for careful data preprocessing in regression analysis.
In situations where you're examining the connection between multiple variables, a correlation matrix can offer insights into their relationships. However, high correlations among explanatory variables (also known as multicollinearity) can make interpretation difficult and potentially inflate standard errors in regression models.
While the sign of \( r \) shows the direction of a relationship, it cannot reveal the extent to which one variable impacts another. This indicates that incorporating coefficient values from regression output is important for a complete understanding. When dealing with data collected over time, correlation can be deceptive due to autocorrelation, where current values influence future values. This complexity makes interpreting correlation coefficients in time-series data challenging and requires specialized time-series analyses.
The squared correlation coefficient (\( r^2 \)) offers a measure of the shared variance between the two variables. Values closer to 1 indicate a stronger connection. Yet, using this measure alone isn't enough to determine the importance or usefulness of this relationship in the context of the phenomenon under study. This emphasizes that a variety of approaches are usually needed for a comprehensive understanding of data.
More Posts from aitutorialmaker.com: