Create AI-powered tutorials effortlessly: Learn, teach, and share knowledge with our intuitive platform. (Get started for free)
How AI-Powered Angle Detection Algorithms Are Revolutionizing Computer Vision in Manufacturing Quality Control
How AI-Powered Angle Detection Algorithms Are Revolutionizing Computer Vision in Manufacturing Quality Control - Neural Networks Transform Samsung Display Panel Inspection with 9% Accuracy
Samsung Display has integrated neural networks into its display panel inspection process, leading to a reported 9% improvement in defect detection. This involves employing a type of neural network called conditionally paired generative networks, which generates artificial images of potential flaws. These synthetic images are useful for improving the system's ability to spot defects in different lighting situations. The move signifies a transition away from traditional manual inspection to a more automated, AI-driven approach. This shift not only accelerates the quality control process but also enhances its accuracy. It reflects a broader trend in manufacturing where businesses are seeking to leverage AI for better quality and efficiency. Essentially, Samsung is not only enhancing its defect detection but also demonstrating its commitment to advanced manufacturing processes, solidifying its position as a leader in innovative quality assurance.
Samsung Display's adoption of neural networks for display panel inspection offers a compelling example of how AI is transforming manufacturing quality control. Historically, human inspection or rudimentary algorithms often struggled with consistently identifying defects, leading to a significant number of errors. This, in turn, could negatively affect product quality and even consumer safety. However, through integrating neural networks, particularly those capable of generating synthetic defect images, Samsung has witnessed a 9% increase in accuracy. This leap in precision isn't simply due to a larger dataset; rather, it highlights the networks' ability to discern minute anomalies often invisible to the human eye or traditional image processing methods.
Beyond higher accuracy, neural networks also accelerate inspection, which translates to faster production cycles. This speed and accuracy stem from a sophisticated understanding of the data within the images. By identifying unique patterns and features, the algorithms provide valuable insight into different defect types. This deep understanding is a boon for engineers, who can now better tackle the root causes of problems. Further, these systems are self-improving. As more data is gathered from inspections, the neural network dynamically adjusts its model, better preparing itself to tackle new, unforeseen issues.
While Samsung is making waves in this area, they are not alone. Competitors are beginning to explore comparable AI-driven solutions, which could potentially push the industry towards even faster evolution and changes in standard practices. This progress, however, also presents challenges. Such sophisticated neural networks require significant processing power, demanding robust hardware infrastructure. Furthermore, integrating these systems can be an initial investment. But, as the neural networks mature, the reduced cost of ongoing quality assurance helps offset these expenditures. The success seen in display inspection could potentially spark similar AI-driven quality control improvements in other manufacturing sectors, marking a wider shift towards AI-powered computer vision.
How AI-Powered Angle Detection Algorithms Are Revolutionizing Computer Vision in Manufacturing Quality Control - Smart Cameras with Edge Computing Detect 1mm Defects in Tesla Body Panels
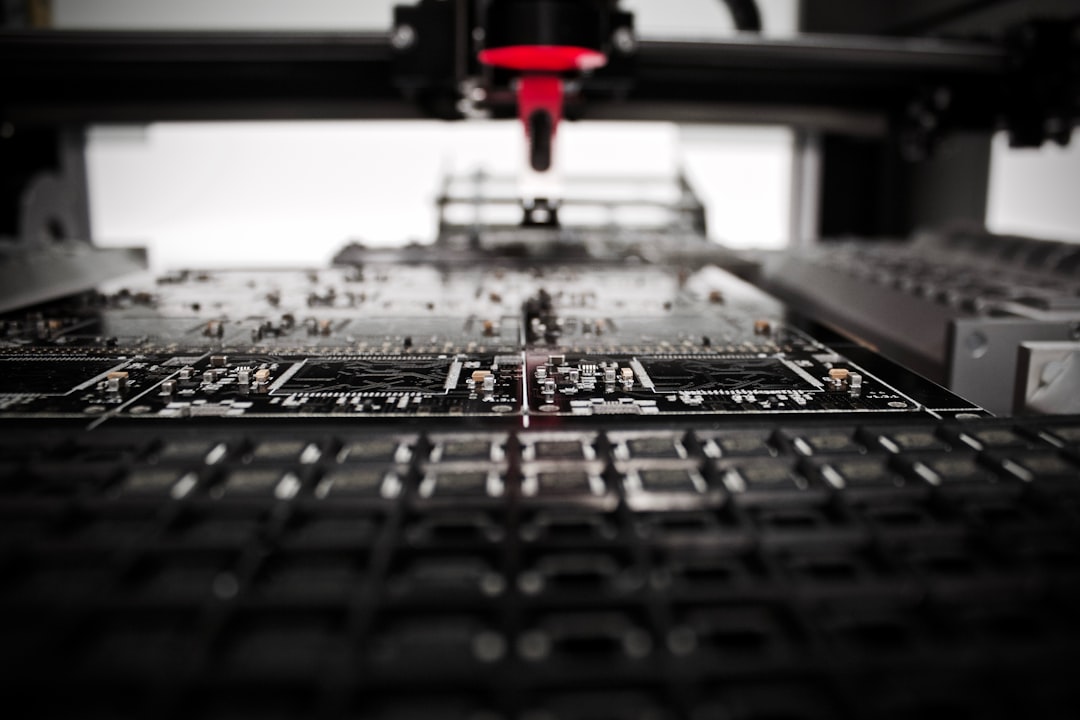
Tesla's adoption of smart cameras, combined with edge computing, signifies a significant step forward in automotive manufacturing quality control. These systems are capable of pinpointing defects as small as 1 millimeter in the body panels of their vehicles. This level of precision is achieved through the use of ultra-high resolution imaging and sophisticated algorithms that analyze crucial aspects of the panels, such as paint thickness, surface textures, and overall uniformity. The incorporation of edge computing is key because it enables real-time analysis of the massive amount of image data generated, leading to immediate defect detection. This is crucial for preventing defective products from leaving the factory and ensuring a higher level of quality.
This application of smart cameras highlights the evolving landscape of manufacturing quality control. It demonstrates a move away from relying solely on human inspectors or simpler algorithms. While these AI-driven systems may involve significant initial investment and complex integration, the potential gains in quality assurance and efficiency appear to be substantial. It is plausible that the success seen in Tesla's application will encourage other manufacturers to implement similar technologies, potentially revolutionizing quality control practices across various sectors beyond automotive. However, concerns remain around the resources needed to support such complex systems and the potential for unexpected issues to emerge. Nevertheless, it's clear that AI-powered visual inspection systems have the potential to significantly reshape quality control in manufacturing, pushing for a higher standard of product integrity.
Tesla's adoption of smart cameras with edge computing for body panel inspection showcases how advancements in computer vision are revolutionizing manufacturing quality control. These cameras can identify defects as small as 1 millimeter, a level of precision that surpasses traditional visual inspection methods, which often miss such subtle flaws. The integration of edge computing is crucial here, allowing for real-time analysis of image data directly at the camera. This eliminates the latency associated with sending data to a central server, resulting in faster feedback to the manufacturing process and potentially improving overall efficiency on the production line.
The algorithms powering these smart cameras are finely tuned to recognize specific defect patterns, which leads to more accurate quality control assessments and reduces the chance of misidentifying defects (false positives). However, a persistent challenge lies in fine-tuning these algorithms to account for varying lighting conditions and surface textures. Changes in these factors can impact the cameras' ability to reliably detect defects, highlighting the need for ongoing refinement in algorithm development.
The data these smart cameras collect is a valuable asset, forming part of a closed-loop quality management system. This system provides insights into the manufacturing process, helping manufacturers optimize production methods based on objective data. This shift away from manual inspection, which is prone to human errors and fatigue, marks a significant step towards more reliable quality control.
It's worth noting that using edge computing also has positive implications for operational sustainability. By processing data locally, these cameras minimize the amount of data transferred to cloud servers, reducing bandwidth usage and associated energy consumption. Tesla's approach is influencing the broader automotive industry, pushing other manufacturers to adopt similar cutting-edge quality control technologies to remain competitive. As smart camera technology continues to evolve, it is expanding beyond just defect detection to include simultaneous process monitoring. This means cameras can now track operational parameters like temperature and humidity, providing a more holistic understanding of the manufacturing environment.
The 1mm defect detection capability achieved by Tesla's smart camera system represents a significant leap forward. It raises the bar for quality assurance across numerous industries, not just automotive. This level of precision not only drives higher quality products but also pushes the boundaries of what's possible in manufacturing quality control. While the field is still evolving, the successes in areas like Tesla's body panel inspection indicate that AI-powered computer vision will continue to play an increasingly important role in the future of manufacturing.
How AI-Powered Angle Detection Algorithms Are Revolutionizing Computer Vision in Manufacturing Quality Control - Machine Learning Spots Welding Flaws in Real Time at BMW Leipzig Plant
BMW's Leipzig facility has integrated machine learning into its production line to spot welding defects in real-time. This is part of a wider push towards improving manufacturing quality control. Partnerships with companies specializing in AI, like IBM and AWS, have allowed BMW to develop advanced audio and visual systems capable of capturing and analyzing welding data. These systems offer near-instantaneous feedback on potential weld flaws, making it possible to inspect nearly every weld. This rapid detection and analysis allows for faster identification and correction of defects, which is crucial for maintaining high manufacturing standards.
This move reflects a broader trend in manufacturing, where the concept of Industry 4.0 is driving a transition towards digital, automated quality control processes. The use of deep learning algorithms for real-time non-destructive evaluation of welds is a significant step in this direction. Although highly beneficial, implementing such complex AI systems requires careful planning and resource allocation. The success of this approach will depend on successfully integrating these new technologies and understanding the associated costs and potential challenges.
At BMW's Leipzig plant, they've integrated machine learning to spot weld defects in real-time during the production process. This approach offers a significant improvement over traditional methods, as it allows for nearly instantaneous identification of flaws. It's quite remarkable how quickly the system can process images, pinpointing defects within milliseconds, a level of speed that's hard to match with manual inspection.
Interestingly, the AI algorithms show impressive sensitivity, capable of detecting very small imperfections, perhaps as tiny as 0.5 millimeters. Human eyes and even standard inspection methods often miss such small defects. This highlights the potential of using AI to achieve a higher level of quality than what's traditionally been possible.
What's more, this is not a static inspection system. It leverages machine learning, which means the algorithms continually adapt and learn from the data they encounter. As more welding instances are inspected, the system refines its understanding of defects, becoming increasingly effective at spotting even previously unseen types of flaws.
Integrating these AI-driven systems into existing production lines seems to be relatively straightforward. It doesn't necessitate massive changes to the production process, making it an easier change to adopt across a factory. This efficient adoption aspect is a major practical advantage, and one that helps encourage its spread across plants.
Another interesting aspect is the wealth of data these systems generate. Each inspection provides insights not only into the presence of flaws but also into the broader welding process. By analyzing these data, BMW can get a deeper understanding of trends within the process and potentially identify areas for improvement.
Interestingly, this has reportedly translated to considerable reductions in rework costs. By identifying defective parts early in the process, they've reduced the number of flawed components moving further down the assembly line. This has significant implications for the factory's profitability.
One of the major benefits is achieving greater consistency in the quality of welds. Human inspectors can be prone to fatigue and inconsistencies in their judgement. By relying on the AI, BMW can be more confident that weld quality meets a high standard across every part, reducing variability in the finished product.
It's intriguing to think that the success seen here in welding could pave the way for similar applications in other manufacturing processes. For instance, techniques for adhesive bonding or complex assembly steps might benefit from this type of AI-powered quality control. This offers an exciting prospect for broader quality control improvements in manufacturing.
The immediate feedback loop provided by the system is an important feature. Workers are alerted to defects in real-time, allowing for prompt corrective measures. This prevents the problem from impacting downstream processes, minimizing production delays.
Lastly, using the AI system significantly reduces the need for extensive manual testing of weld joints. Engineers can spend less time on these more routine checks and focus on other, more critical aspects of the manufacturing process and product design. This efficient allocation of engineering talent could lead to further improvements and innovation.
While this application of AI in manufacturing is still relatively new, its early success at BMW Leipzig suggests a promising future for AI in manufacturing quality control. It's exciting to consider how these developments will continue to evolve and shape the future of manufacturing.
How AI-Powered Angle Detection Algorithms Are Revolutionizing Computer Vision in Manufacturing Quality Control - Computer Vision Reduces Quality Control Labor Costs by 47% at Toyota Assembly Lines
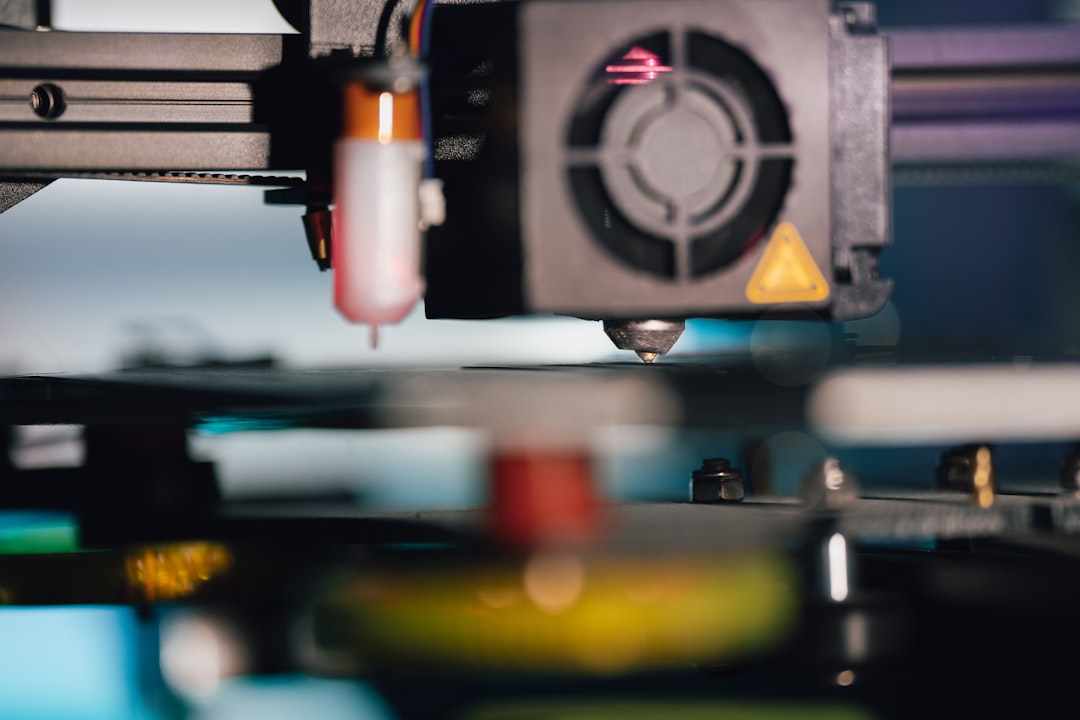
Computer vision has brought about a significant change in Toyota's manufacturing process, leading to a 47% decrease in labor costs related to quality control. This improvement is largely due to AI-powered angle detection algorithms that augment existing quality control methods. It's interesting to note that despite the potential for automation in car assembly, robots still play a minimal role at Toyota, with less than 8% of tasks handled by machines. This suggests that a balance between human expertise and technology remains important in manufacturing. Moving forward, AI's use in quality control is likely to bring more cost savings and enhanced product quality. But it's worth considering the impact of increased automation on employment within the manufacturing field, especially given Toyota's current reliance on human workers. There is a question about the extent of the use of AI in manufacturing and its future potential.
Computer vision systems have brought about a substantial 47% reduction in quality control labor costs at Toyota's assembly lines. This shift from human inspectors to automated systems isn't just about cost savings, it also leads to a more efficient production process. It's quite fascinating how quickly the changeover seems to have been implemented.
This cost reduction is closely tied to an increase in inspection speed. Toyota is now able to inspect parts much more rapidly, speeding up the entire manufacturing cycle. While that's positive, one does wonder if that speed increase has resulted in a reduction in the number of people needed on the factory floor to handle unexpected issues. It will be interesting to see if there are longer-term implications.
A key aspect of Toyota's system is its ability to analyze huge numbers of images per second with incredible accuracy, far surpassing human capabilities. This accuracy means that very tiny defects that might escape a human eye can be caught and dealt with promptly. It makes you wonder if the threshold of 'defect' was redefined with the advent of this new technology. Is it identifying anomalies that historically wouldn't have been seen as quality issues, potentially leading to unnecessary rejects and material waste?
Beyond simply finding defects, the system collects data, which helps Toyota's engineers uncover recurring defect patterns. This capability allows them to get ahead of the problem, proactively making adjustments in their processes to reduce future flaws. It's encouraging to see proactive improvement based on data, however, what kind of problems can be anticipated? Are we heading towards a future where the AI is driving production changes without necessarily understanding the engineering implications?
This computer vision setup doesn't remain static. It's a dynamic system that learns and adapts based on past inspections. As more data is collected, its accuracy improves, and it becomes increasingly adept at recognizing novel or evolving types of defects. It seems likely this will get to a point where the AI itself is suggesting design and manufacturing process alterations. The question is, will it be able to justify those suggestions in a way that engineers will accept? Are there potential issues of 'black box' optimization here that could cause unforeseen problems?
One notable effect is on the human workforce. Instead of performing repetitive inspections, human workers are retrained for more complex quality control roles that need a more sophisticated human judgment call. It would be useful to know how easily factory workers are able to adapt to these new roles and if the retraining initiatives have been successful. Are there people who are unable to adjust and if so, how is the company supporting their transition?
It's also remarkable how robust the system is. It doesn't matter the lighting conditions, the system seems to consistently provide high-quality results. This adaptability is crucial, ensuring quality control isn't impacted by changing factory environments or shifts. While that's encouraging, is the robustness of the technology fully tested? Is it still vulnerable to unforeseen issues related to changes in the manufacturing process or external factors?
The changes at Toyota align with broader industry trends toward smart manufacturing, where technology plays an increasingly critical role in optimizing production and ensuring product quality. But, it's certainly a shift in perspective. Previously, human inspection was integral to the process. Now, are we creating a 'human-out-of-the-loop' system? Are there checks and balances or redundancies built-in in case of unexpected outcomes?
While the shift to automation reduces the immediate need for humans in these specific inspection tasks, it raises questions about the future of the factory workforce. If the trend of reducing reliance on human inspections continues, what jobs will these workers be taking on? Furthermore, the new workforce must be prepared to manage and operate these AI-driven systems. What training and educational changes are being made to the workforce and education system?
Ultimately, while the initial investment in these computer vision technologies is substantial, the long-term labor cost savings and improvements in defect detection could result in a more efficient and ultimately more profitable operation. However, the success of this strategy is very much reliant on its ability to continuously adapt and the company's ability to manage the shifting workforce in the long term. It remains to be seen how this technology will shape the future of manufacturing and the role of humans in these processes.
How AI-Powered Angle Detection Algorithms Are Revolutionizing Computer Vision in Manufacturing Quality Control - Deep Learning Models Achieve 24/7 Surface Defect Detection in Steel Manufacturing
Deep learning is increasingly being used to identify surface defects in steel production, allowing for continuous, 24/7 monitoring. These models incorporate techniques like multiscale feature extraction to better understand the complex nature of surface flaws and categorize defects with greater accuracy. Advanced algorithms, such as YOLOv8TLC, have been developed to specifically improve the detection of larger defects. Further refinements, such as incorporating more scale detection layers, aim to reduce instances of missed defects, a common challenge with previous methods.
While progress is being made in automating these inspections, many steel mills still rely on traditional manual inspection methods. One of the major obstacles is the training time associated with these deep learning models, coupled with a reluctance to fully transition away from well-established, albeit less efficient, procedures. Nonetheless, the success of deep learning models in this domain hints at a possible future where automated visual inspection (AVI) becomes the norm. This transition holds the promise of substantial improvements in quality control, which in turn could help reduce risks associated with defective steel materials. It remains to be seen how quickly the industry as a whole adopts these AI-powered systems.
Steel production is increasingly relying on deep learning models for round-the-clock surface defect detection. This shift is driven by the limitations of human inspectors, who can experience fatigue and inconsistencies in their observations, especially during long shifts. Deep learning offers a potential solution by enabling continuous monitoring and analysis.
The integration of deep learning with image processing techniques allows for the detection of extremely small surface defects, potentially down to 0.1 millimeters. This level of precision surpasses human visual capacity and traditional inspection methods, opening the door to enhanced quality control. Deep learning systems typically leverage convolutional neural networks (CNNs) to automatically learn and extract crucial features from images. This automated feature extraction allows the system to more effectively isolate and classify defects, even under complex visual conditions.
The performance of these deep learning models tends to improve as the volume of data they process grows. This means the systems can adapt to new types of defects, continually refining their detection algorithms. This continuous learning and adaptation are significant advantages over static inspection methods. One of the practical benefits of using these deep learning models in steel manufacturing has been a noticeable reduction in false positives, the identification of defects where none actually exist. Reducing false positives helps prevent unnecessary interruptions and the costly downtime associated with spurious quality checks.
Many sophisticated deep learning systems for defect detection use a two-model approach. One model handles general defect detection, while the other specializes in recognizing specific defect types. This dual-model architecture often leads to a more flexible and robust quality control system. This approach is well-suited to environments with a variety of potential defects and variations in raw materials. Ultimately, using these deep learning models has led to demonstrable improvements in yield rates within steel manufacturing. This increased efficiency translates to a decrease in the amount of wasted material and potentially significant cost savings, as defects can be identified and addressed earlier in the production process.
However, the implementation of such advanced systems poses some challenges. Deep learning models demand specialized hardware and a significant amount of computational power, leading to high initial investment costs. This capital expenditure can be a barrier for many manufacturers looking to adopt these technologies. Beyond the immediate cost of setup, the complex nature of the AI systems raises issues related to ongoing oversight and validation of the decisions made by the algorithms.
Beyond simply finding defects, these AI models analyze the data they gather over time. This provides valuable insight into the production process itself, enabling manufacturers to optimize processes and proactively mitigate potential quality problems. While this ability to anticipate and address issues is attractive, it's also crucial to consider how human experts will integrate these insights and what level of oversight is needed.
The promise of significantly improved defect detection with deep learning is exciting, but the increasing reliance on these autonomous systems raises some questions regarding the appropriate level of human oversight and validation. As these systems learn and evolve independently, it becomes important to consider the processes needed to ensure the ongoing quality of their judgments and decision-making. This aspect of AI integration is a key area for future research and development within the manufacturing industry.
How AI-Powered Angle Detection Algorithms Are Revolutionizing Computer Vision in Manufacturing Quality Control - Automated Visual Inspection Systems Process 1200 Parts Per Minute at Foxconn
Foxconn's implementation of automated visual inspection systems showcases a significant leap in manufacturing speed and quality. These systems can process a staggering 1200 parts per minute, a feat that relies heavily on AI-powered angle detection algorithms. This sophisticated approach allows for the identification of minute flaws that might escape the human eye or traditional inspection methods, thereby improving the overall quality of manufactured products and reducing wasted materials. The integration of deep learning and advanced technologies like RGBD further enhances the capabilities of these systems, allowing for a more nuanced analysis of images and, in turn, more effective quality control. This surge in automated visual inspection represents a substantial shift in the manufacturing landscape, with a clear emphasis on using AI to establish and maintain higher production standards. While it signifies progress, it's crucial to keep in mind the broader implications of this technology on the manufacturing workforce and the potential for unforeseen complications.
Foxconn's automated visual inspection systems can process an impressive 1200 parts per minute, showcasing the potential of computer vision in high-volume manufacturing. These systems aren't just looking at simple features; they perform a comprehensive analysis of each part, checking dimensions, surface condition, and other factors – potentially exceeding what a human inspector could achieve. It's interesting to see how they handle the varied lighting conditions commonly found in factories. These systems are designed to automatically adapt, making the inspection process less susceptible to issues caused by changing light.
This automated approach has also shown a reduction in false rejects – a situation where a human inspector, maybe due to fatigue or inconsistencies, misjudges a perfectly good part. Having the inspection integrated into the production process allows for instant feedback. If a flaw is detected, operators can immediately address it, avoiding more significant waste and improving overall efficiency. Beyond just identifying defects, these systems collect data. This creates an opportunity to gain insight into the entire production process, enabling Foxconn to optimize and potentially predict quality issues more efficiently than with manual methods.
Interestingly, these systems are built with machine learning, meaning they continually learn and adapt as they analyze more data. This means the accuracy of these systems should improve with time and should be increasingly capable of identifying new and previously unseen defects. It's a compelling argument that a major benefit of these automated systems is reduced reliance on human inspectors. This can free up personnel for more complex and nuanced tasks, while also leading to cost savings. The sheer volume of production at Foxconn makes maintaining consistent quality across millions of units crucial, and the automated systems seem designed to tackle this need for standardized quality.
It seems like a key design feature is scalability. The modularity of these automated systems means that if Foxconn's production needs change, they should be able to adjust the inspection capacity relatively easily and without significant disruption to production. It will be interesting to see how this adoption of high-speed automated systems influences the wider industry and the evolving role of humans in high-volume manufacturing.
Create AI-powered tutorials effortlessly: Learn, teach, and share knowledge with our intuitive platform. (Get started for free)
More Posts from aitutorialmaker.com: