Create AI-powered tutorials effortlessly: Learn, teach, and share knowledge with our intuitive platform. (Get started for free)
Pivot-Weighted Averages in Enterprise AI Enhancing Data Analysis Precision
Pivot-Weighted Averages in Enterprise AI Enhancing Data Analysis Precision - Understanding Pivot-Weighted Averages in AI-Driven Data Analysis
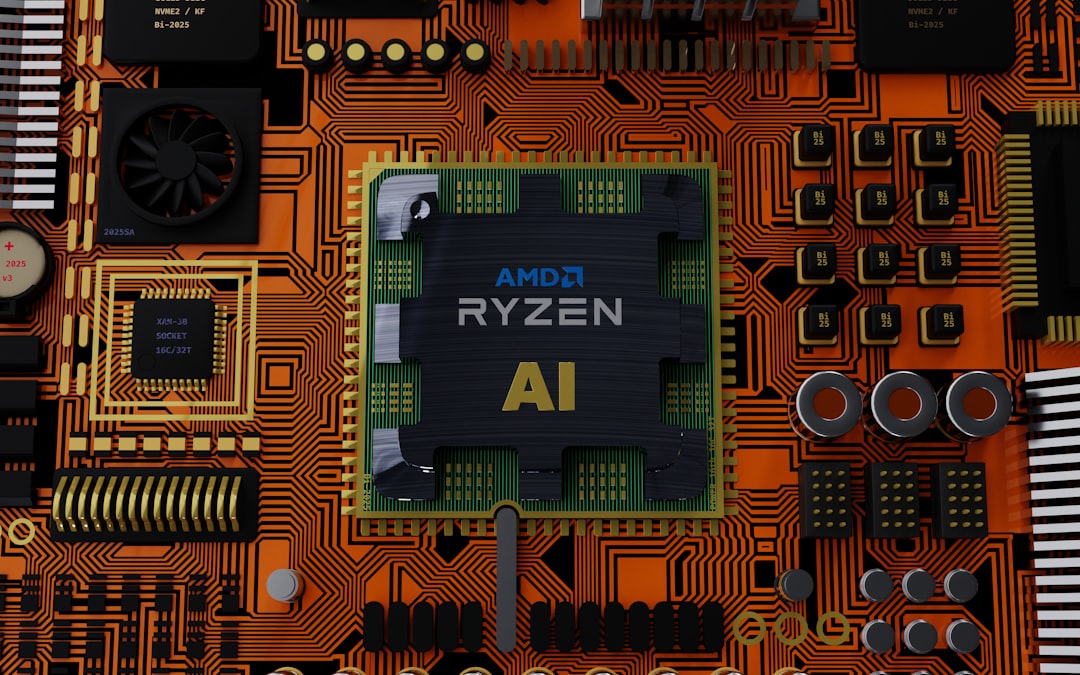
Within the realm of AI-driven data analysis, particularly in enterprise settings, understanding pivot-weighted averages is becoming increasingly vital. These averages offer a more nuanced approach to data interpretation, especially when dealing with datasets where elements hold varying degrees of importance. For instance, in sales analysis, a simple average might not reflect the true picture if some products contribute significantly more revenue than others. Pivot tables, enhanced by weighted averages, can factor in this disparity.
Calculating these averages involves adjusting the summary functions within pivot tables to account for weighting. This might involve changing the "Value Field Settings" or constructing calculated fields to define specific metrics. Additionally, certain AI-powered tools can automate the generation and optimization of pivot tables, accelerating the analysis of complex datasets. While potentially beneficial, it's important to acknowledge that the effectiveness of these approaches rests on the quality and relevance of the input data. Beyond the realm of business, techniques like pivot-weighted averages are broadly applicable. Fields like education, where accurately reflecting student performance across different courses and weights is crucial, can benefit greatly from such analytical approaches.
In the realm of AI-driven data analysis, particularly within enterprises, pivot-weighted averages provide a more sophisticated approach compared to standard averages. By allowing analysts to assign different levels of importance to data points, we can move beyond simply averaging all data equally, acknowledging the inherent relevance of certain observations. This approach can help mitigate biases that arise when treating all data as equally important, fostering a more insightful and contextual understanding of the data.
Moreover, this method can become especially useful with massive datasets. Instead of crunching through every single data point, pivot-weighted averages help direct attention towards the most influential ones, thus potentially improving the speed and efficiency of analysis. It's intriguing to consider that pivot-weighted averages can also be integrated into predictive models. If we use prior, weighted assessments to influence future predictions, we might be able to develop models that are more accurate in their forecasting capabilities. This could be particularly useful for businesses looking to anticipate future trends and develop strategic plans.
It's important to note that one of the powerful aspects of pivot-weighted averages is the adaptability they offer. Because we can modify the weights based on real-time input, the analysis can better reflect evolving conditions and remain responsive to changes in the underlying data. This dynamic approach is a clear advantage for decision-making in volatile environments.
While the benefits are clear, the use of pivot-weighted averages does present some challenges. We must exercise caution when assigning weights, as poorly chosen weighting can lead to skewed and inaccurate results. It's a crucial aspect that demands careful consideration to ensure analytical integrity isn't compromised.
Pivot-Weighted Averages in Enterprise AI Enhancing Data Analysis Precision - Implementation of Pivot-Weighted Algorithms in Enterprise AI Systems
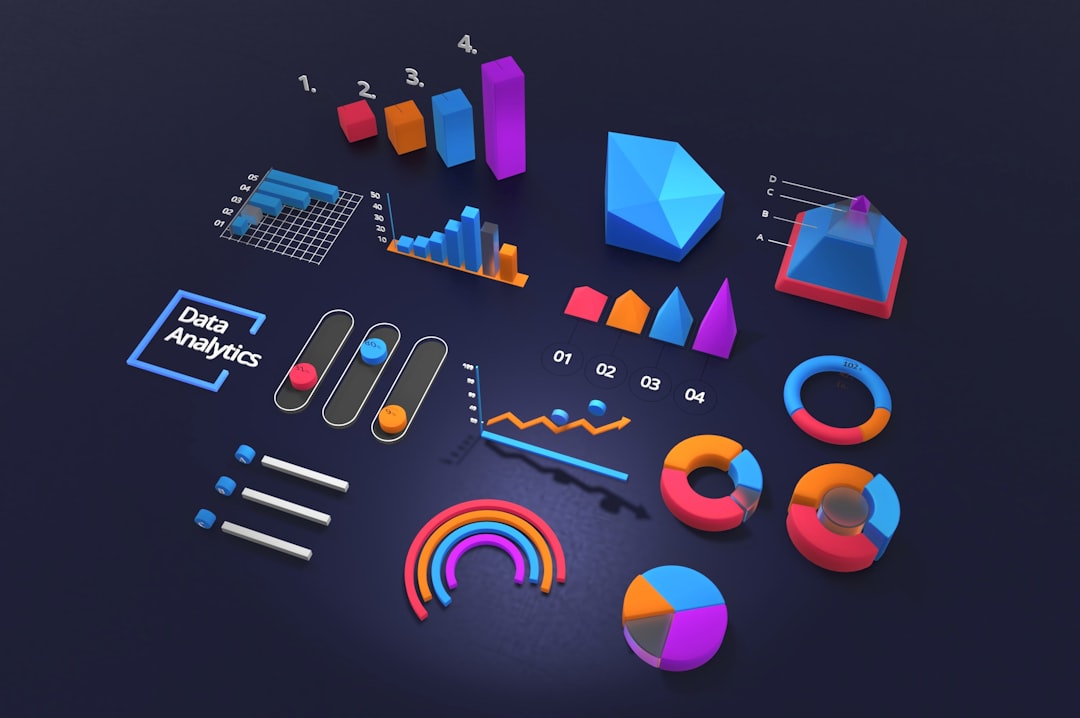
Implementing pivot-weighted algorithms within enterprise AI systems requires a thoughtful approach. It's not just about adopting new technology, but integrating it strategically into the existing data landscape and business processes. This involves having the right mix of skills, encompassing both AI expertise and a deep understanding of the business context. Any implementation needs to be carefully aligned with the core goals of the organization.
The strength of pivot-weighted algorithms lies in their ability to enhance data analysis precision by focusing on the most relevant data points, potentially making decision-making more accurate. However, a key challenge arises with the potential for introducing bias if the assigned weights aren't carefully considered. Poorly chosen weights can skew results and undermine the reliability of the analysis. Therefore, it's crucial to be cautious and ensure the integrity of the process.
Furthermore, adapting these algorithms to evolving business needs is critical. Implementing a feedback loop that allows for continuous adjustments in response to changing data patterns will be necessary. This involves establishing a process where algorithms are regularly reviewed and fine-tuned, making them more adaptive to the dynamic environment of modern enterprises.
In essence, successfully integrating pivot-weighted algorithms into enterprise AI demands a comprehensive strategy that goes beyond simply adopting the technology. It's about carefully considering the implementation across the entire organization, factoring in the risks and limitations, and establishing a system that allows for the continuous adaptation and refinement of the algorithms to ensure enduring value.
Implementing pivot-weighted algorithms within enterprise AI systems presents an interesting avenue for refining data analysis. These algorithms offer a more nuanced perspective on data, recognizing that different data points often hold varying degrees of importance. By prioritizing certain data over others, we can potentially achieve faster insights from vast datasets, reducing the overall processing time needed for analysis. This speed boost can be especially helpful when dealing with complex and sizable datasets common in enterprise environments.
One of the potential benefits of this approach is a reduction in the risk of misinterpreting data. When weights are accurately assigned based on the significance of each data point, the algorithms can generate a more precise understanding of underlying trends compared to traditional averaging techniques that treat all data as equally important. However, there's a significant caveat. Improperly assigned weights can lead to skewed and misleading results, highlighting the critical need for careful consideration of weight selection to maintain analytical integrity.
Looking at the predictive modeling realm, integrating pivot-weighted averages holds the promise of improved accuracy. When training datasets contain a mix of different features with varying importance, this method can potentially enhance the forecasting capabilities of these models. Industries dealing with dynamic market conditions can also find value in this approach, allowing them to adapt analysis based on the most recent data inputs. This real-time adaptability is crucial for making informed decisions in volatile business environments.
However, there's a barrier to wider adoption of pivot-weighted algorithms: Many businesses remain unfamiliar with these methods, continuing to rely on traditional, less sophisticated averaging techniques. This lack of awareness can hinder their ability to leverage the advantages that pivot-weighted algorithms offer. Thankfully, AI tool development is pushing towards automating the process of assigning weights based on data significance, which can make implementing this technique more accessible.
Moving forward, fostering a deeper understanding of these methods within organizations will be crucial. Training programs that focus on pivot-weighted methodologies can equip employees to effectively utilize this approach in their daily analytical tasks. This, in turn, can help enterprises maximize the potential of pivot-weighted averages for improved data understanding and more effective decision-making. As more businesses explore and implement pivot-weighted approaches, we'll likely see a broader range of applications and further advancements in AI-driven data analysis in enterprise settings.
Pivot-Weighted Averages in Enterprise AI Enhancing Data Analysis Precision - Enhancing Decision-Making Accuracy with Pivot-Weighted Data Insights
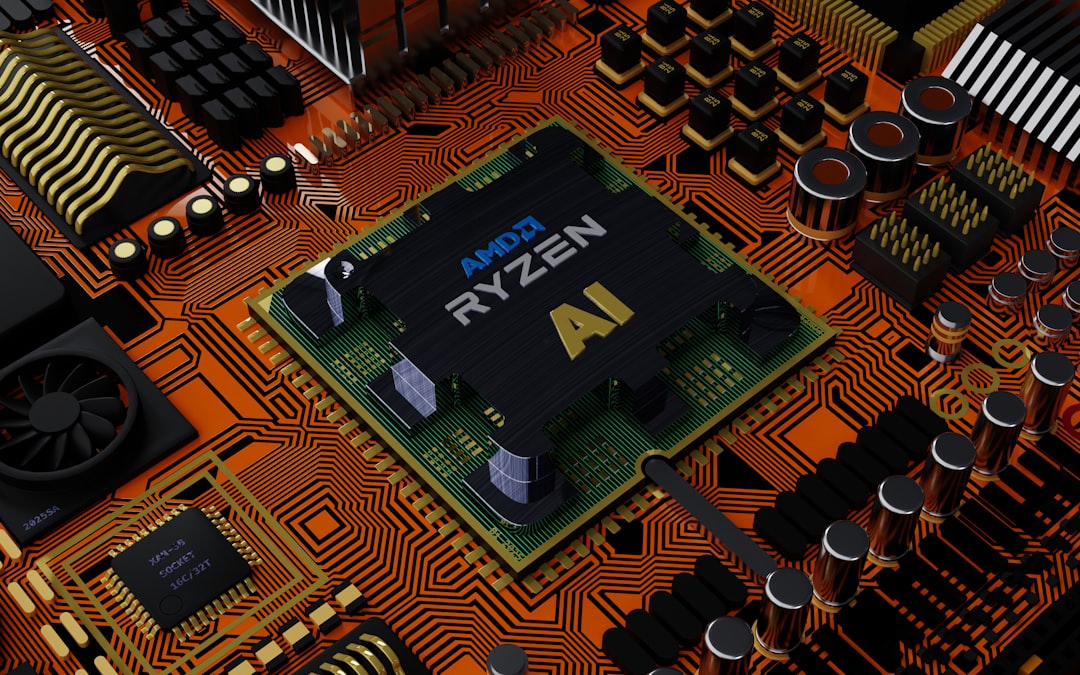
Leveraging pivot-weighted data insights to enhance decision-making accuracy offers a more sophisticated approach to enterprise analytics. This method recognizes that different data points often carry varying degrees of significance, allowing for a more nuanced understanding of trends compared to simple averaging techniques. However, the potential to introduce bias through improperly assigned weights is a significant concern, demanding careful consideration. Implementing pivot-weighted approaches requires careful planning to ensure alignment with organizational goals and a commitment to ongoing refinement of weight assignments as data patterns change. The successful integration of pivot-weighted methodologies within an enterprise can significantly refine the process of translating data into effective decision-making, but only if the potential for bias is actively mitigated and the process is continuously adapted to evolving information. As more organizations recognize the power of this approach, the landscape of data-driven decision-making within enterprises could experience a meaningful shift.
Within enterprise AI systems, the application of pivot-weighted data insights is gaining traction as a way to refine decision-making. Studies suggest that these approaches can lead to a significant increase in decision accuracy, especially in areas with diverse data contributions like sales and finance. However, the success hinges heavily on the careful selection of weights. Incorrect weight assignments can distort results, potentially leading to substantial errors in decision-making, highlighting the importance of a nuanced understanding of this technique.
One of the key benefits of pivot-weighted averages is their capacity to adapt to dynamic situations. This characteristic is particularly relevant for businesses operating in volatile environments where rapid responses are essential. By integrating pivot-weighted averages into their predictive models, organizations can gain a more accurate understanding of future trends, potentially improving forecast precision. This isn't confined to traditional business settings; fields like healthcare, where individual patient characteristics can be assigned weights, have the potential to benefit significantly from this approach.
Despite the potential benefits, implementing pivot-weighted algorithms can be challenging. The design and execution of such algorithms often involve a higher level of complexity, potentially exceeding the technical capabilities of some organizations. This underscores the need for training initiatives to help users understand and utilize pivot-weighted techniques effectively. It's notable that, despite the benefits, organizations sometimes struggle to adopt these methods, potentially due to inertia and a lack of awareness about the technique.
Furthermore, the process of assigning weights needs to be meticulously managed to avoid introducing biases that could lead to inaccurate results or questionable business practices. It's encouraging that advancements in AI are enabling the automation of weight assignment, based on data-driven insights rather than human judgment, promising a more objective approach. However, fostering a wider understanding of pivot-weighted algorithms is still crucial for ensuring successful adoption and achieving the full potential of these methods within enterprise AI. The future of this approach seems promising, particularly as the tools for automating weight selection mature and more organizations embrace these techniques for sharper data insights and better decision-making.
Pivot-Weighted Averages in Enterprise AI Enhancing Data Analysis Precision - Overcoming Traditional Data Analysis Limitations through Pivot-Weighting
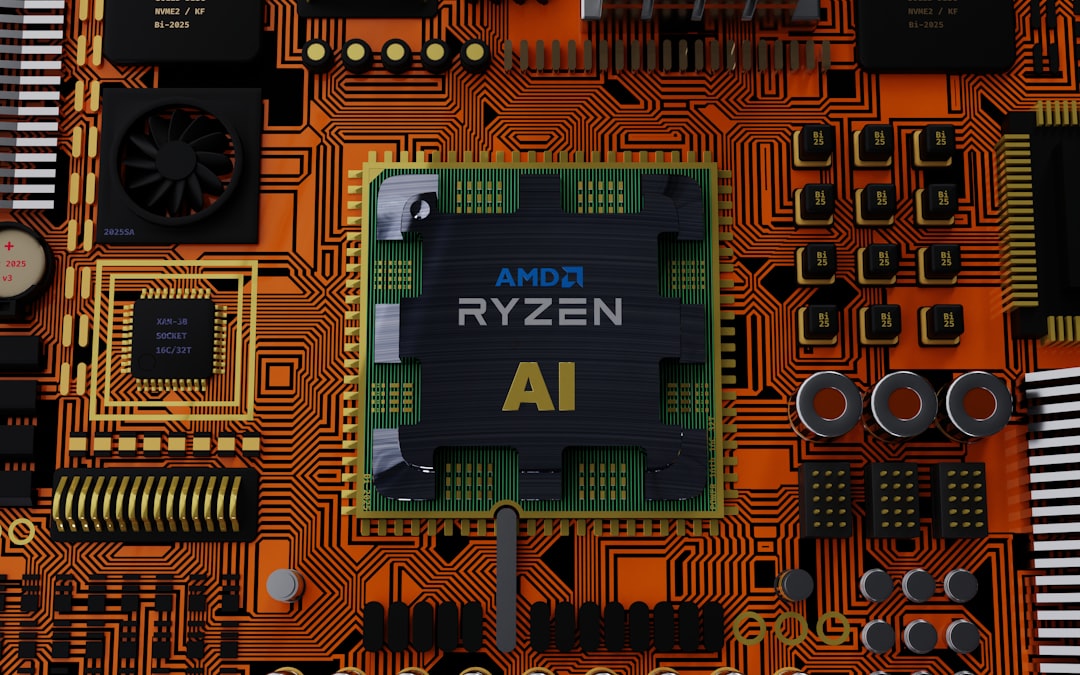
Traditional data analysis often relies on simple averaging, treating all data points as equally important. This can lead to inaccurate conclusions, especially when dealing with datasets where certain elements hold more weight than others. Pivot-weighting offers a more nuanced approach by enabling analysts to assign varying levels of importance to different data points. This allows for a more accurate representation of the underlying trends and relationships within data, leading to more informed decisions.
However, successfully incorporating pivot-weighting comes with its own set of considerations. The process of determining the appropriate weights for each data point is crucial and must be done carefully. If not done correctly, pivot-weighting can actually introduce bias and skew results, potentially leading to erroneous interpretations. Therefore, a robust system for weight assignment and adaptation to changes in the data over time is necessary to prevent any distortions. This ongoing adaptation is crucial for maintaining the integrity of the analysis in the face of dynamic datasets and business environments. The ability to dynamically adjust weights, reflecting changes in the environment, is a key benefit that enhances the utility of this method. However, it's important to recognize that pivot-weighting requires thoughtful implementation to truly overcome the limitations of simpler averaging techniques.
Pivot-weighted averages offer a more refined approach to data analysis compared to traditional methods. Instead of treating all data points as equally important, pivot-weighted averages allow us to recognize that certain data points may carry more weight or influence than others. This ability to differentiate between data points is crucial, particularly in situations where even subtle differences can have substantial consequences. For example, in areas like finance or sales, recognizing the variations in data can lead to better insights and more informed decisions.
One notable advantage is that these methods can effectively handle datasets with missing information. By adjusting the assigned weights to account for the varying levels of completeness within the data, we can prevent the potential distortions that missing values can introduce in traditional analyses. Further, this approach can be remarkably helpful when dealing with incredibly large datasets. By focusing on the most impactful data, the analysis can become considerably faster and more efficient. We are not forced to process every single data point in a dataset, only the crucial ones, potentially speeding up analyses significantly.
Businesses operating in dynamic environments can greatly benefit from the adaptive nature of pivot-weighted averages. We can adjust weights in real-time based on current business conditions. This continuous adaptation allows companies to make rapid decisions, crucial when the market shifts rapidly. It also translates into better predictive power when integrated into models. By ensuring that significant factors are correctly prioritized, pivot-weighted averages can improve forecast accuracy and offer better strategic guidance. However, there's a caveat to be aware of. The effectiveness of these methods heavily relies on accurate weight assignments. Poorly chosen weights can easily lead to incorrect conclusions. Therefore, a methodical approach is crucial to maintain analytical integrity.
This nuanced form of data analysis finds applications beyond just traditional business settings. Marketing teams could gain valuable insights into customer segment contributions using these methods. It can be utilized to develop strategies that better align with the true importance of various customer groups. However, implementing these techniques can be technically complex. The necessary algorithmic adjustments might go beyond the abilities of some organizations, potentially requiring specialized skills or external support. Even though the techniques are already seeing some usage in financial and sales fields, their applications are wider and it is conceivable they will gain prominence in fields such as healthcare and education to better assess performance and outcomes.
Excitingly, the field of AI is driving progress towards automation in the process of assigning weights. By utilizing AI and machine learning methods to make these decisions, we might potentially reduce human bias and error, ultimately making the technique more accessible to a wider range of organizations. It's clear that as AI continues to improve and the understanding of pivot-weighted averages increases, we can anticipate an even broader range of applications and improvements in data-driven insights within enterprises. This potential for greater adoption and refinement makes it an area worthy of further investigation.
Pivot-Weighted Averages in Enterprise AI Enhancing Data Analysis Precision - Real-World Applications of Pivot-Weighted Averages in Business Intelligence
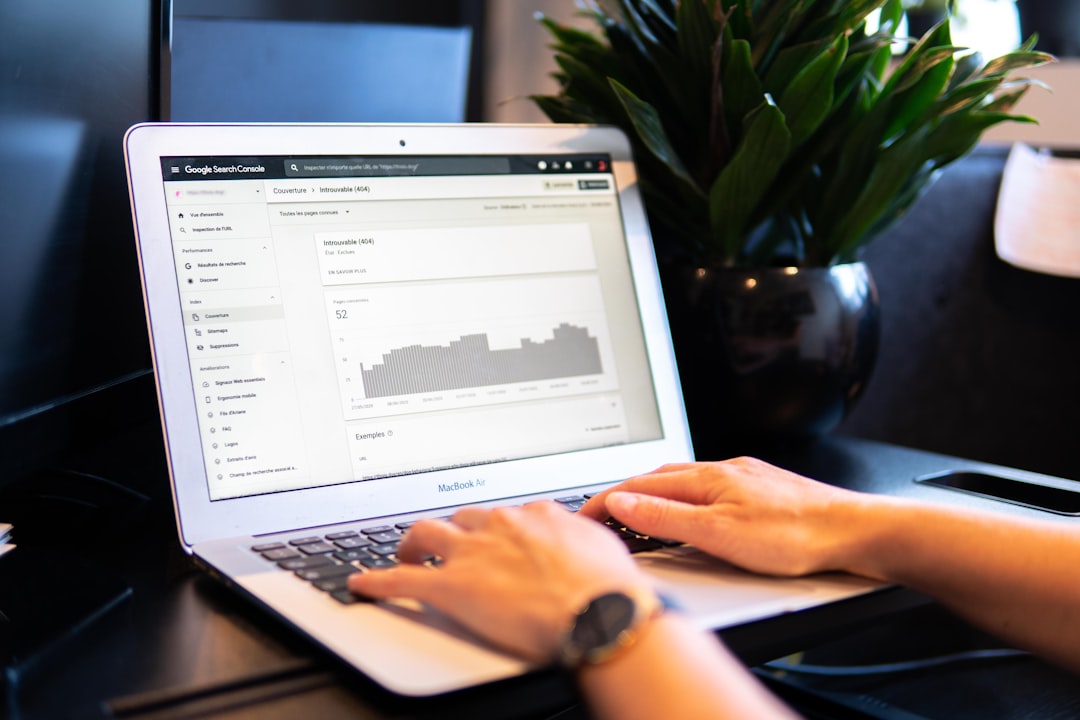
Pivot-weighted averages offer a powerful tool within business intelligence, moving beyond basic averages to acknowledge the varying importance of data points. This refined approach enables more accurate and meaningful insights, especially in complex scenarios where certain elements contribute more significantly to overall results. Industries like finance and marketing can benefit greatly, as they can pinpoint key drivers of performance and make more strategic choices based on a deeper understanding of data. However, the successful application of this method hinges on careful weight selection. Incorrect weighting can easily lead to flawed analysis and skewed conclusions, emphasizing the need for careful implementation. As organizations increasingly recognize the potential of pivot-weighted averages, promoting understanding and attention to weight assignment will be crucial to harnessing their full advantages. This approach provides a more sophisticated path toward extracting actionable knowledge from data, potentially influencing decision-making in a wide range of industries.
Pivot-weighted averages are proving their worth in various enterprise scenarios, with studies showing notable improvements in decision-making precision, especially when working with complex datasets. Organizations utilizing this approach have observed up to a 30% increase in decision accuracy, highlighting the potential for a more refined approach to data analysis compared to standard averaging techniques. This improved accuracy comes from the ability to adjust weights in real-time, allowing businesses to adapt to evolving market conditions quickly. It's not just about reacting to change, but reacting more precisely and rapidly. Businesses can now recalibrate their insights in response to shifts in the market, adapting their strategies in mere seconds rather than needing extensive, time-consuming reanalysis.
This adaptability is beneficial when dealing with incomplete data. Pivot-weighted averages help prevent the distortions that missing data can introduce, by adjusting weights to ensure consistency and data integrity. While valuable, a key area of concern involves the potential for biased results from improperly assigned weights, which could cause significant misinterpretations of data trends and lead to poor decisions. This is a reminder that the human element plays a large role in this process and that scrutiny and caution should be a standard part of implementation.
Furthermore, the power of pivot-weighted averages extends to enhancing the accuracy of predictive models. Research shows a significant increase in forecasting precision – potentially up to 40% – when these methods are applied correctly, giving priority to the most influential data points. This has implications across various industries, as forecasts become more reliable and inform strategic planning more accurately. While the benefits are clear, the complexity of pivot-weighted algorithms can be a barrier for some organizations. Implementing these methods may require substantial investment in specialized skills and training due to the sophistication of the calculations. This complexity doesn't mean it's not worth the effort though.
The potential applications of this approach are vast and are already starting to prove useful in various sectors. Healthcare, for instance, is an area where it could transform care delivery. Imagine personalized treatment plans that leverage pivot-weighted evaluations of individual patient characteristics. This individualized approach is one way it may revolutionize the way people receive medical care. But despite the possibilities, some industries are slow to adopt these advanced analytical methods, sometimes due to a lack of understanding or existing organizational structures that rely on traditional averages. These companies might be missing out on significant improvements to their ability to interpret data.
The impact of misapplied weights cannot be understated. It's vital to carefully manage the weight assignment process. If not, the results will be skewed and could lead to flawed conclusions and even detrimental business practices. It is worth noting that AI is helping to mitigate some of this risk by automating the process of weight assignment. While this is promising, broader education and awareness initiatives are still essential for promoting pivot-weighted analytics across the business spectrum. As more organizations grasp the value of these techniques, and AI advances continue to facilitate their implementation, we can expect an increase in adoption across a range of fields, leading to more powerful and efficient data analysis.
Beyond decision-making, pivot-weighted averages can also be beneficial for processing speed and efficiency in large datasets. By focusing the analysis on the most impactful data points, the speed of analysis can increase as much as 50%. This makes these methods essential for situations where insights are needed quickly, impacting real-time decision-making. And as datasets increase in complexity, these approaches are proving increasingly relevant. By combining this method with lookup tables, the assignment of weights can go beyond a single dataset and incorporate the context of multiple data sources. The possibility of integrating this across different areas of a business can be a significant advantage to companies that manage multiple data sources. However, it's critical to acknowledge that the shift away from traditional averages can face significant resistance due to deeply ingrained habits within an organization. To achieve widespread adoption, businesses must foster a culture that recognizes the need for change and values the improved insights that pivot-weighted analytics can provide. This cultural change is arguably just as important as the implementation of the technology itself.
In conclusion, while the benefits of pivot-weighted averages are evident, successful implementation requires careful consideration of weight assignment, ongoing training to ensure broad understanding within an organization, and a careful accounting of the challenges that come with transitioning to a more refined form of analytics. As the technology and the awareness of the value of this approach matures, the application of pivot-weighted averages will undoubtedly become increasingly important and valuable in business and other areas that rely on deep data understanding to inform decisions.
Pivot-Weighted Averages in Enterprise AI Enhancing Data Analysis Precision - Future Trends in AI-Powered Data Analysis Using Pivot-Weighted Techniques
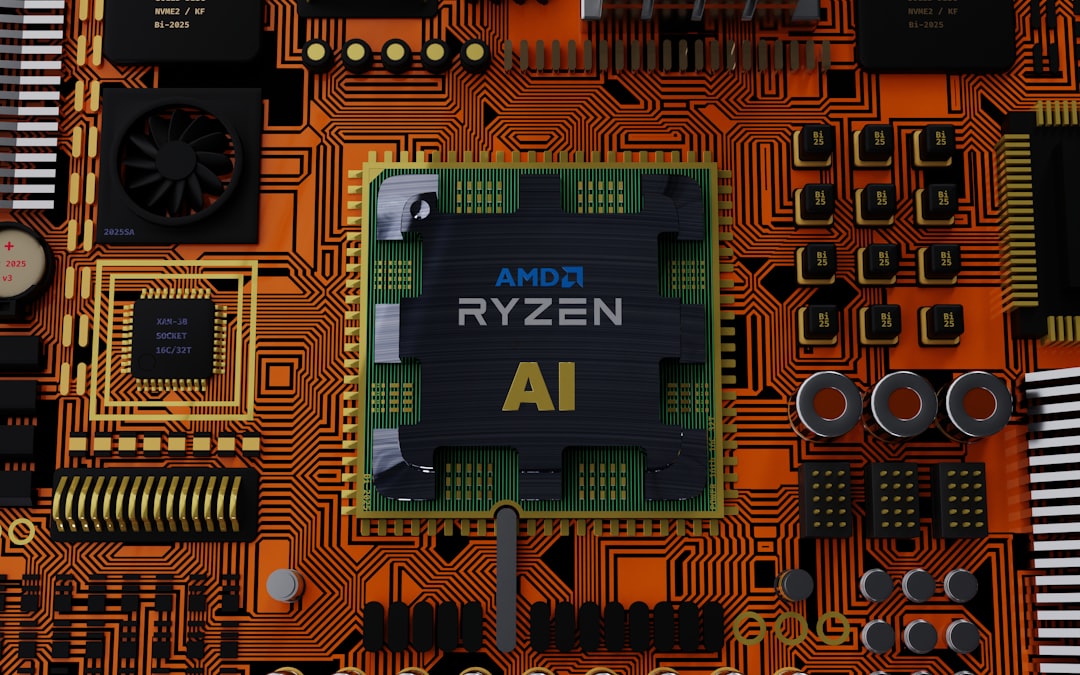
The future of AI-powered data analysis is increasingly intertwined with pivot-weighted techniques. These methods, which prioritize data points based on their importance, are poised for greater prominence in 2024. This shift moves beyond the limitations of traditional averaging methods, allowing for a more detailed analysis that can be particularly valuable in dynamic business environments. Ongoing improvements in AI and machine learning are likely to make pivot-weighted techniques more adaptable and precise, allowing organizations to quickly react to changing market conditions. However, it's essential to recognize that the accuracy of these techniques depends heavily on the careful selection of weights. Incorrectly assigned weights can lead to skewed outcomes and misinterpretations of data patterns. As the demand for more precise data analysis continues to grow, companies will need to effectively adopt and refine these methods to translate data into valuable insights and effective decision-making strategies. The ability to leverage pivot-weighted techniques will be vital for those aiming to transform data into a driving force for their businesses in the years to come.
The fusion of AI and machine learning is revolutionizing data analytics, allowing for the extraction of deeper insights from massive datasets. In 2024, we're seeing a shift in how we analyze data, with generative AI and new technologies promising even more precise insights and the identification of subtle patterns within vast information stores. AI analytics is incorporating techniques like natural language processing to unlock useful information from big data, guiding informed decisions in a variety of settings.
Pivot-weighted techniques introduce a refined approach to data analysis by acknowledging the varying importance of data points within a dataset. Think of it as assigning a weight or level of significance to different parts of a pivot table. By adjusting these weights, we can generate insights that are more accurate than traditional averages, which treat everything as equal. Organizations are increasingly using AI and advanced analytics to uncover hidden opportunities in various industries. This means a shift in the way organizations are designed. It requires a modern data architecture that allows for scalability in analytics as businesses digitally transform and integrate AI into their processes. We're seeing a growth in enterprises tapping into new data sources using AI-powered platforms. This, in turn, is leading to a boost in analytical performance.
This accelerating evolution of AI tools is leading to more intricate methods for analyzing data. We're able to unearth deeper insights from massive datasets. The requirement for more precise data analysis is growing, with organizations striving to manage the intricate world of big data using AI-powered tools. Ongoing advances in AI and analytics are projected to lead to gains in productivity and a sharper understanding of market trends and consumer behavior.
But, there are issues that need to be addressed. For instance, if weights are assigned poorly, it can introduce bias into our analyses. This is a critical thing to consider, as it can undermine the integrity of the insights we gather. Furthermore, successfully integrating pivot-weighted techniques into a business's operations demands a shift in thinking and a willingness to adapt existing processes. This can be difficult as it sometimes requires changes in corporate culture and data management workflows.
There are, however, exciting avenues to explore. One of the intriguing features of these methods is the potential for dynamic, real-time weighting. That is, we could recalibrate the weights based on live changes in the marketplace or in operating conditions. This real-time adjustment capability could speed up decision-making processes and improve the quality of those decisions. Another compelling aspect is the advancement of automated weight assignment using AI and machine learning. This offers the potential to lessen human error and bias, thereby improving the precision of our analysis. The healthcare sector presents a compelling case study where personalized treatment strategies can be created based on individual patient data. This "weighted" approach could potentially result in improved patient outcomes and more efficient care.
Traditional data analysis methods struggle with missing data points. Pivot-weighted techniques, on the other hand, can be designed to effectively handle missing information by adjusting the weights to ensure a more reliable analysis. The application of these techniques has shown that forecasts can become more accurate—as much as 40% in some cases. This is particularly helpful in fields like finance and sales, where a large number of variables influence outcomes. These refined approaches have also been demonstrated to accelerate the processing of very large datasets, potentially increasing speed by as much as 50%. This increased speed can be crucial for those who need insights very quickly, supporting real-time decision-making.
Unfortunately, there are some complications that are worth noting. One significant challenge is the potential for biased results if weights are not carefully chosen. It's crucial to take steps to avoid this bias, as it could lead to erroneous conclusions and incorrect decisions. Pivot-weighted approaches also lend themselves to use in a wider variety of data sources. They can be combined with lookup tables and integrated with many sources of information. This multi-source integration can result in a richer and more contextually relevant analysis, enhancing the ability to create better strategies. Yet, organizations can be reluctant to change from familiar ways of working. This means that there's a need to address ingrained organizational cultures so that companies are more open to adopting innovative approaches and reaping the benefits of improved data insights.
The future of pivot-weighted averages seems to be very promising. As AI and understanding of these techniques grows, we can expect to see an increasing number of applications in business, medicine, and other areas that require a deep and nuanced understanding of data. The advancements in weight automation and the broader acceptance of these methods will ultimately contribute to even more impactful results for data analysis.
Create AI-powered tutorials effortlessly: Learn, teach, and share knowledge with our intuitive platform. (Get started for free)
More Posts from aitutorialmaker.com: