Create AI-powered tutorials effortlessly: Learn, teach, and share knowledge with our intuitive platform. (Get started for free)
The Evolution of AI-Powered Threat Detection in Cybersecurity A 2024 Perspective
The Evolution of AI-Powered Threat Detection in Cybersecurity A 2024 Perspective - AI-Powered Threat Detection Advancements in 2024
The year 2024 is witnessing a surge in the sophistication of AI-powered threat detection, driven by the ever-evolving tactics of cyber attackers. Organizations are recognizing the necessity of incorporating advanced AI solutions to stay ahead of these increasingly complex threats. This emphasis on AI in cybersecurity underscores a broader shift in how we approach online security, recognizing the need for continuous innovation.
Beyond simply employing AI, the conversation is pivoting towards responsible and ethical deployment. We're seeing a growing awareness of the potential pitfalls of relying solely on AI, necessitating thoughtful consideration of how it's applied and integrated into existing security infrastructure. At the core of these improvements are techniques like machine learning and natural language processing, allowing for faster and more accurate threat detection in real-time.
Given the crucial role AI is playing in cybersecurity, discussions are becoming more focused on crafting robust and secure frameworks. These frameworks need to not only enhance threat detection capabilities but also protect the integrity and security of the AI systems themselves, ensuring they remain reliable and trustworthy in the face of evolving cyberattacks. This highlights a crucial point—building secure AI systems is integral to the future of cybersecurity and protecting against the increasingly sophisticated threats of 2024.
The field of AI-powered threat detection is progressing rapidly in 2024. We're seeing a push towards leveraging quantum computing for faster data processing, enabling these systems to handle the increasingly complex threats we face in real-time. A notable shift is the emergence of self-learning algorithms, which potentially reduce the reliance on human experts for constant updates. These systems are becoming more adaptive, reacting to new attack patterns autonomously, which could lead to faster responses to unknown threats.
Another fascinating development is the creation of tools capable of predicting attacker behavior by analyzing past data and current actions. This predictive approach allows for a proactive defense, potentially thwarting attacks before they even occur, a significant step beyond reactive responses. Improvements in natural language processing are enabling the analysis of unstructured data, like emails and messages, helping to identify social engineering attacks, something that often escapes traditional methods.
Furthermore, advancements in anomaly detection have made a significant impact on identifying insider threats with remarkable accuracy. These systems can pinpoint subtle shifts in user behavior, revealing previously hidden threats. The use of synthetic data for training machine learning models is allowing organizations to simulate attacks in a safe environment. This provides valuable insights into the effectiveness of existing defenses and strengthens their incident response procedures without risking exposure to real-world attacks.
The collaboration between threat detection and blockchain technology is an interesting development, as it allows for enhanced data integrity and the ability to trace attacks back to their origin more effectively. Federated learning, a technique that trains models across multiple organizations without exposing sensitive data, is promising for collective cybersecurity improvement while addressing privacy concerns.
AI-driven threat intelligence reports are becoming more sophisticated, tailoring threat information to specific industries and geographic locations. This allows for more precise risk management based on location-specific vulnerabilities and industry-specific attack patterns. Finally, we're seeing the integration of behavioral biometrics into threat detection, enhancing the precision of user authentication. By creating individual behavioral profiles for users, systems can better recognize deviations and flag unauthorized access attempts, making it more difficult for malicious actors to bypass security measures. While the future of AI in cybersecurity is filled with promise, it's important to consider the potential challenges and ensure a balanced approach to its implementation.
The Evolution of AI-Powered Threat Detection in Cybersecurity A 2024 Perspective - Machine Learning Models Tackle Advanced Persistent Threats
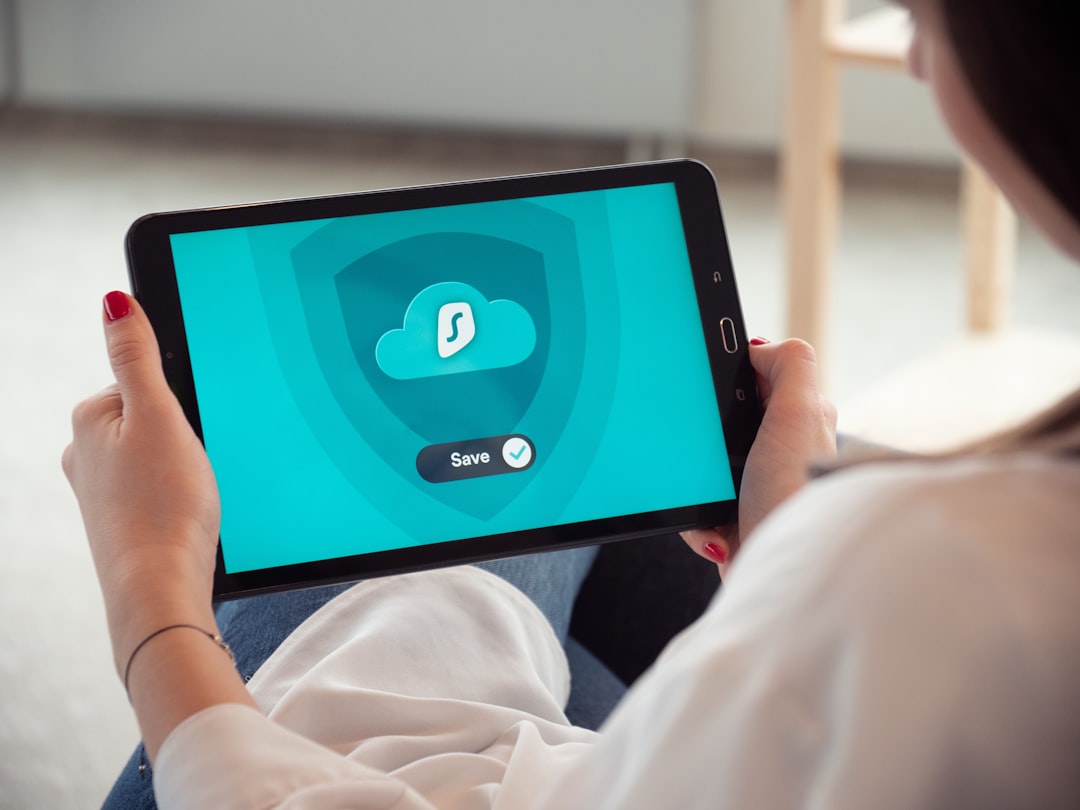
Advanced Persistent Threats (APTs) continue to pose a significant challenge to organizations due to their persistent and stealthy nature, making them difficult to detect using conventional methods. Machine learning models are increasingly being used to address this challenge, offering a more robust and adaptive approach to threat detection. These models are trained to identify subtle patterns and behaviors characteristic of APT attacks, often hidden from standard security tools.
Supervised learning methods play a key role, utilizing labeled datasets to train models in recognizing known APT techniques and behaviors. However, the dynamic nature of cyber threats and the emergence of new attack techniques, like the misuse of large language models, require constant refinement and adaptation of these machine learning models. Researchers are exploring hybrid approaches, incorporating deep learning methods, to further enhance detection capabilities and improve upon existing techniques.
The continuous evolution of cyber threats necessitates a persistent focus on innovation within machine learning to effectively counter evolving APT tactics. The efficacy of machine learning models in identifying APTs is becoming increasingly critical as organizations strive to protect themselves from increasingly sophisticated attacks and ensure the integrity of their cybersecurity defenses.
Advanced Persistent Threats (APTs) are a persistent challenge for organizations due to their subtle nature and ability to evade traditional security measures. Machine learning (ML) and artificial intelligence (AI) are emerging as powerful tools for improving APT detection, leveraging their ability to find subtle patterns and novel attack methods. For example, ML models can analyze a wide range of data sources, from network traffic and logs to endpoint activity, spotting early warning signs that might otherwise go unnoticed.
One intriguing approach is the use of supervised learning, where models are trained on labeled datasets of known APT techniques and behaviors. This can help identify familiar tactics, but it doesn't necessarily guarantee protection against novel attacks. While supervised learning helps with known threats, the ever-evolving nature of attacks means a significant portion of cyberattacks remains outside of the labeled data used to train the models. This gap underscores the ongoing need for adapting these techniques.
AI-driven cybersecurity is a game changer in the fight against cyber threats, making detection both faster and more accurate. It's becoming increasingly clear that AI will be essential as the volume and complexity of cyberattacks continues to rise. The faster the detection, the faster the chance to mitigate any potential damage, which is crucial for protecting individuals and organizations.
Researchers are evaluating a variety of ML algorithms to improve APT detection, including Random Forest, Gradient Boosting, and MLPClassifier. The field is exploring innovative techniques like hybrid deep learning approaches to enhance existing methods. However, challenges remain. For instance, new attack behaviors, such as prompt injections and the misuse of large language models, are testing the limits of current APT detection methods.
The landscape of cyberattacks is dynamic, with threat actors constantly innovating their tactics. A comprehensive review of existing APT research underscores the need for continual development and refinement of detection methodologies. There is a continued need to address novel attack techniques, like prompt injection attacks related to misuse of large language models. This underlines the ongoing research and development efforts needed to keep pace with the threats that are evolving. Overall, while there are many benefits of machine learning and AI models, there are also challenges to address in order to keep the models relevant in a constantly evolving threat environment.
The Evolution of AI-Powered Threat Detection in Cybersecurity A 2024 Perspective - Real-Time Anomaly Detection Using Neural Networks
Real-time anomaly detection using neural networks is becoming essential for navigating the complex cybersecurity landscape. These systems harness the power of machine learning to dynamically respond to evolving threats, identifying subtle deviations from expected network behavior. The use of Graph Neural Networks (GNNs) has proven particularly valuable, enhancing the ability to detect anomalies by analyzing intricate relationships within network data. While these advancements are promising, there are limitations. The need for substantial labeled datasets and the constant adaptation of detection strategies remain key challenges. As threats continue to evolve, the creation of dependable real-time anomaly detection systems will be crucial for safeguarding digital spaces.
Real-time anomaly detection using neural networks is becoming increasingly important in cybersecurity, with researchers actively exploring various approaches. The architecture of these networks, particularly convolutional and recurrent types, plays a crucial role in processing the often sequential and spatially structured data involved in network traffic and security events. However, a challenge arises in the need for diverse training data that represents both normal and anomalous behavior. Without sufficient variety, these models can become overly specialized, struggling to detect new attack methods used by cybercriminals.
The ability of neural networks to process large volumes of data in real time, often through parallel computing, is essential for timely anomaly detection. A key advantage is that these models often include automatic feature extraction, meaning they can identify important patterns without needing a lot of human input during model creation. This automation can speed up the development process.
Combining multiple neural networks, also known as ensemble methods, has shown promise in improving the overall accuracy of anomaly detection. This approach can reduce false alarms while maximizing detection rates. Furthermore, transfer learning can be valuable in situations where labeled training data is limited. By taking a model pre-trained on a different task, we can potentially jumpstart the process of building an anomaly detection system for a new cybersecurity context.
Many neural network approaches incorporate adaptive learning to continually improve their performance. This is crucial, as the cyber threat landscape is constantly evolving. However, this area of research raises the issue of model interpretability. It is often hard to explain precisely *why* a particular anomaly was flagged, which can hinder cybersecurity professionals from fully evaluating the significance of an alert and responding appropriately.
In the realm of cybersecurity, speed is paramount. Even small delays can have significant negative consequences. Therefore, the development of real-time anomaly detection systems needs to consider low latency and how the system can be integrated smoothly into existing security tools. These integration issues can create roadblocks to effective implementation, so thoughtful planning is needed to avoid such challenges.
In summary, neural networks offer exciting possibilities for improving anomaly detection in cybersecurity, especially in real-time situations. But these promising techniques must address limitations related to training data diversity, interpretability, and integration with existing systems to truly be impactful in real-world security contexts. As the landscape of online threats continues to evolve, ongoing research and development efforts are needed to adapt and enhance these neural network approaches for optimal protection.
The Evolution of AI-Powered Threat Detection in Cybersecurity A 2024 Perspective - Automated Incident Response and Remediation Systems
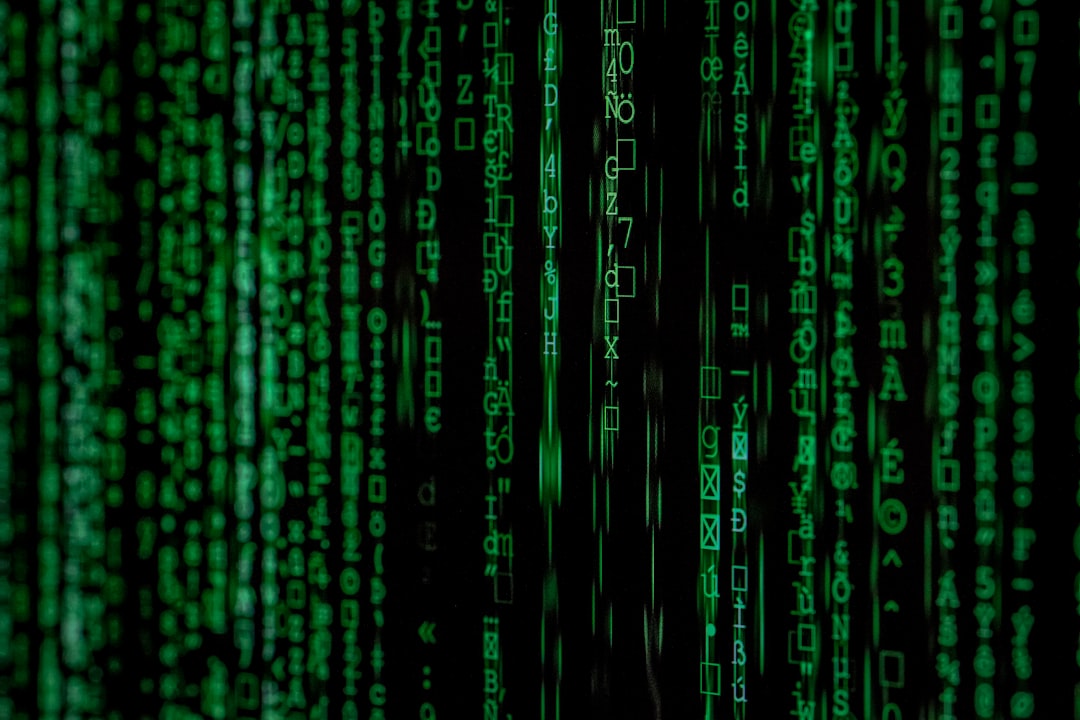
Automated Incident Response and Remediation Systems are increasingly vital in today's cybersecurity landscape. These systems use AI to significantly accelerate and improve the detection and handling of security incidents. Automation helps reduce alert fatigue, a common problem in traditional security operations, and improves the accuracy and speed of threat responses. As threats become more sophisticated and numerous, AI integration allows organizations to not only react to incidents but also to anticipate and prevent potential attacks. However, relying on automated systems brings challenges, such as the possibility of overlooking nuanced issues in complex situations where human expertise is needed. The ongoing development and refinement of these systems are crucial in achieving the delicate balance between leveraging AI's speed and efficiency while maintaining the importance of human oversight.
Automated Incident Response and Remediation Systems (AIRRS) are increasingly vital in the cybersecurity landscape of 2024, primarily due to their ability to react to threats much faster than human operators. This rapid response, measured in milliseconds, is essential to minimize the impact of cyberattacks. However, the reliance on automation in complex attack scenarios raises questions about human oversight and the potential for unintended consequences.
Many AIRRS are designed to learn from past incidents, employing reinforcement learning techniques to adapt their responses to recurring or novel attack patterns. This iterative process allows them to continually improve their ability to address evolving threats. A key feature of these systems is their integration with diverse security tools, including firewalls and endpoint detection systems. This integration provides a comprehensive view of the threat environment, enabling more effective threat management.
One challenge with widespread adoption of these systems is the scalability required for large organizations. Real-time data processing and intricate configurations pose significant hurdles in applying them across complex enterprise environments. Additionally, while these systems excel at addressing known threats, their contextual awareness can be limited, sometimes leading to misclassification or inappropriate responses to attacks requiring more nuanced human judgment. This highlights a critical area for improvement.
Interestingly, some AIRRS incorporate predictive analytics to anticipate potential security weaknesses. This allows organizations to preemptively address vulnerabilities and avoid breaches. Moreover, by automatically recording incidents and responses, they contribute to simplified audit trails and streamline compliance efforts across various regulatory frameworks.
Instead of complete automation, some implementations focus on a human-in-the-loop approach. This hybrid model allows security personnel to supervise the system's actions and ensure that complex or nuanced decisions are still guided by human expertise. However, the inherent limitation of these systems is their tendency to misinterpret unfamiliar attack vectors, relying as they do on historical data. This underscores the constant need to provide fresh threat intelligence to these systems to maintain their efficacy in the dynamic cybersecurity world. Continuous training is essential to ensure their ability to stay ahead of emerging threats and remain a valuable component in cybersecurity defenses.
The Evolution of AI-Powered Threat Detection in Cybersecurity A 2024 Perspective - Integration of Natural Language Processing in Threat Intelligence
The integration of Natural Language Processing (NLP) within threat intelligence is revolutionizing how we understand and counter cyberattacks. NLP's ability to sift through unstructured data, including emails, online conversations, and social media posts, helps uncover social engineering tactics and phishing schemes that often escape traditional security tools. This capability improves the accuracy of threat detection and, importantly, allows for quicker incident response by automating the parsing of large amounts of textual data. However, successfully implementing NLP in this arena faces obstacles. Training NLP models on sufficiently varied data is a significant hurdle, as is ensuring these models can maintain a nuanced understanding of the constantly changing cyber threat landscape. Ongoing advancements in NLP technologies are anticipated to enhance threat intelligence methodologies, ultimately strengthening our overall cyber defenses. Despite the progress, it's crucial to recognize that NLP models, like any AI tool, must be used thoughtfully and critically to ensure they are effective and reliable in their role.
Integrating Natural Language Processing (NLP) into threat intelligence has opened up exciting avenues for analyzing the vast sea of unstructured data that surrounds us, including social media and online forums. This allows cybersecurity systems to identify emerging threats that traditional methods might miss, which is pretty useful in a world where attacks are constantly evolving.
NLP helps in recognizing phishing scams and social engineering tactics by meticulously dissecting the language used in communications. It looks for subtle manipulative language that may hint at malicious intent, which often goes undetected by more basic approaches.
Furthermore, by utilizing sentiment analysis, NLP tools can gauge the emotional tone within online communications. This helps recognize malicious campaigns that try to exploit public sentiments or leverage crises for their gain. It's an interesting area of research.
These advanced NLP algorithms can automatically gather threat intelligence from many different sources. This significantly cuts down the time security analysts spend on collecting data, enabling them to focus more on the important stuff, like making strategic choices and developing future defenses.
Interestingly, researchers are creating NLP models that not only detect threats but also generate human-readable reports summarizing their findings. This can help bridge the communication gap between technical security teams and upper management, which could improve the efficacy of threat responses.
When these language models are trained specifically on the unique terminology and language found within cybersecurity, we see a marked increase in their accuracy in threat classification. Generic language models sometimes get tripped up by the specialist language within the field.
The real-time analysis capabilities of NLP systems can accelerate the time between the initial discovery of a threat and the response to it. This speed is quite important and gives organizations a leg up in thwarting cyberattacks before they spread, a crucial aspect of defensive security.
One issue with relying on NLP for threat intelligence is the possibility of false positives. Ambiguous language can lead to these false alarms, so continuously adjusting and updating the NLP models to adapt to the ever-changing language patterns used by attackers is a key ongoing challenge.
NLP also plays a role in automating the process of sorting security alerts. By categorizing them based on their urgency and what type of alert it is, security teams can focus on the biggest threats first. It's like a triage system but for cyberattacks.
As more organizations incorporate NLP tools into their security frameworks, we're seeing concerns about the ethical implications of this automated surveillance and monitoring. This brings up questions regarding user privacy and data protection laws, issues we need to think about moving forward.
The Evolution of AI-Powered Threat Detection in Cybersecurity A 2024 Perspective - Quantum Computing's Impact on AI-Based Cybersecurity Measures
Quantum computing's emergence introduces a new layer of complexity to the field of AI-based cybersecurity. The potential for quantum computers to break current encryption methods poses a significant threat to existing security protocols. This reality necessitates a shift towards developing new security measures that are resistant to these future capabilities. Post-quantum cryptography is gaining attention as a potential solution, focusing on creating encryption methods that can withstand the processing power of quantum computers.
At the same time, the role of AI in cybersecurity is evolving to counter the risks associated with quantum computing. Researchers are exploring how AI, specifically machine learning algorithms, can be integrated into cryptographic systems to improve security. Furthermore, AI is likely to be used in developing more comprehensive security frameworks designed to handle the challenges of a quantum computing era. This convergence of quantum computing and AI has the potential to fundamentally alter cybersecurity practices, creating a need for advanced defense strategies that can ensure the resilience of digital systems.
Quantum computing's potential to process information at an exponentially faster rate than traditional computers is creating both excitement and concern in the cybersecurity realm. This speed could enable AI to analyze and react to cyber threats in real-time, an area where traditional AI sometimes falls short due to processing limitations. However, this increased processing power also poses a threat to current encryption methods, as quantum computers have the potential to break them. Developing quantum-resistant algorithms becomes crucial to ensure that our fundamental online security practices remain robust.
Interestingly, quantum computers are particularly good at optimizing complex AI models. This could potentially improve the accuracy of threat detection by ensuring models are trained on the most relevant data, potentially leading to better outcomes. The unique properties of quantum physics, like entanglement, could also provide more secure communication channels, making certain information transfer theoretically impossible to intercept.
AI's current approach to cybersecurity often relies on traditional probability and pattern recognition. Quantum computing, however, provides a completely different way to represent information, with the ability to represent both deterministic and probabilistic elements. This could potentially lead to AI cybersecurity approaches that can identify threats in much more nuanced ways, potentially yielding breakthroughs in threat detection.
There are, however, significant challenges to implementing quantum computing in cybersecurity. Creating practical and error-corrected quantum algorithms remains a significant research hurdle. Until this is addressed, we won't see the full potential of quantum computing in cybersecurity.
Looking ahead, quantum computing's integration with AI might redefine how we think about cybersecurity. Quantum machine learning could revolutionize threat intelligence, providing an unprecedented level of data analysis that could lead to the ability to identify and predict cyber threats with far greater accuracy than before. But as we see with most new technological innovations, there's a flip-side. Quantum computers, if used by attackers, could potentially break through traditional cybersecurity barriers more easily.
Moreover, quantum key distribution and other quantum-based techniques could facilitate a more decentralized approach to threat detection. This could help organizations build more resilient defenses and improve the overall security posture of the internet.
It's clear that the relationship between quantum computing and AI in cybersecurity is a fundamental shift, not just an evolution. We're likely to see entirely new cybersecurity models emerge, potentially changing the way organizations manage risk and threats. It's an exciting and complex space, with many potential benefits and challenges to navigate.
Create AI-powered tutorials effortlessly: Learn, teach, and share knowledge with our intuitive platform. (Get started for free)
More Posts from aitutorialmaker.com: