Create AI-powered tutorials effortlessly: Learn, teach, and share knowledge with our intuitive platform. (Get started for free)
UBC's AI Research 7 Key Projects Shaping the Future of Machine Learning
UBC's AI Research 7 Key Projects Shaping the Future of Machine Learning - AIMSI Cluster Recruits Five AI Professors for Cutting-Edge Research
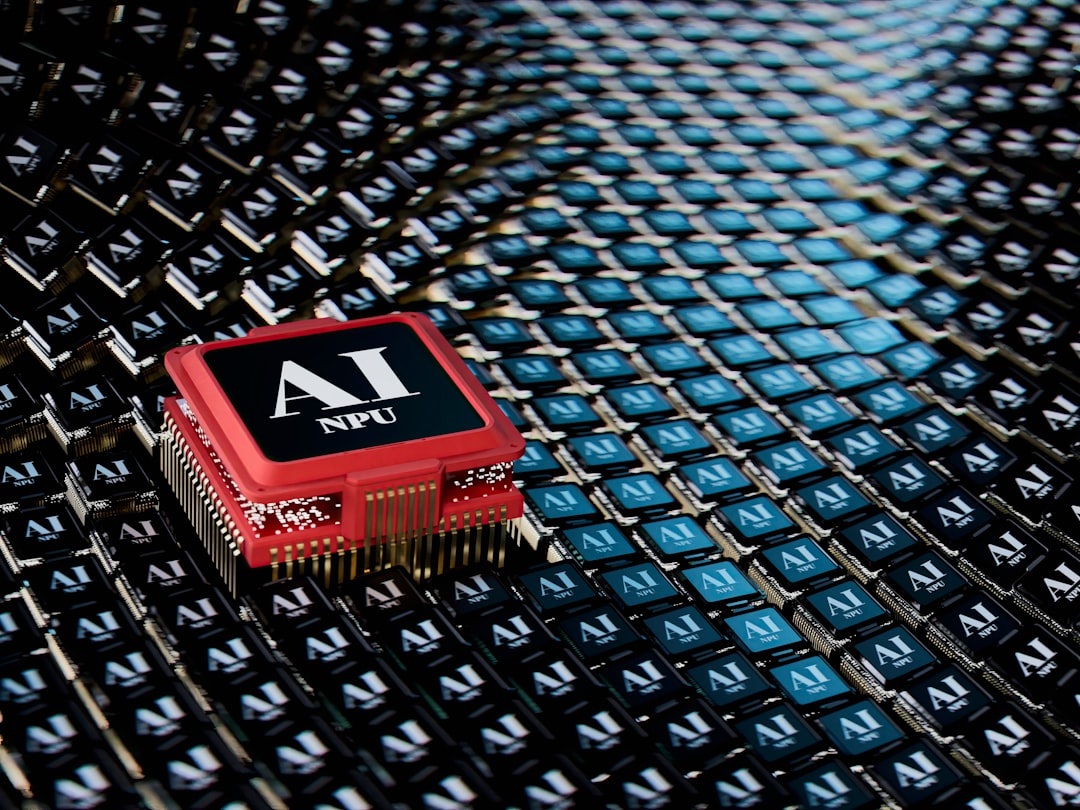
The AIMSI cluster at UBC has brought on board five new AI professors to significantly enhance their research capabilities. This recruitment drive is a clear indication of the university's commitment to advancing artificial intelligence and establishing itself as a leader in machine learning research. The move reflects the growing importance of AI as a transformative technology with the potential to revolutionize various fields. It suggests that UBC recognizes the crucial role AI will play in the future, particularly as it promises to have a major economic impact. This strategic expansion of AI expertise underscores the growing importance of collaboration in the field, emphasizing the synergy between AI and other scientific disciplines. This commitment to building a strong AI research community positions UBC to play a leading role in shaping the future of machine learning.
The AIMSI cluster's recruitment of five AI professors signifies a substantial commitment to bolstering UBC's position in AI research. It seems to be a reflection of a broader trend among universities – a competitive scramble for top talent to drive ambitious research agendas. These new faculty members bring specialized knowledge in crafting machine learning algorithms that could potentially enhance the transparency and understandability of AI models, which is a major hurdle in the field.
Their appointments appear to be well-founded, backed by secured competitive grants that align with UBC's focus on technological progress. Notably, their collective experience includes significant work in federated learning, a technique that enables training models on scattered data sources while prioritizing data privacy. This collaborative environment across different disciplines at UBC may spark unforeseen breakthroughs in implementing AI across a diverse range of fields, such as healthcare, the financial industry, and robotics.
Furthermore, this recruitment effort underscores a commitment to inclusivity in the tech sector, with one of the professors having a history of mentoring underrepresented groups in STEM. Their ambitious goals include publishing high-impact research within the next two years, helping UBC gain more prominence in the global AI landscape. Another key focus will be on improving the efficiency of training extensive neural networks, an increasingly crucial concern given the escalating computational demands of modern AI. By leveraging UBC's current connections with industry players, it is hoped this initiative will result in a synergistic exchange of ideas and foster the development of tangible applications for their research discoveries. Ultimately, this strategic shift within UBC showcases a clear focus on AI research methodologies that aim to address practical problems rather than focusing solely on theoretical advancements. This emphasizes the university's desire to contribute to real-world solutions.
UBC's AI Research 7 Key Projects Shaping the Future of Machine Learning - Machine Learning in Robotics Advances at UBC Labs
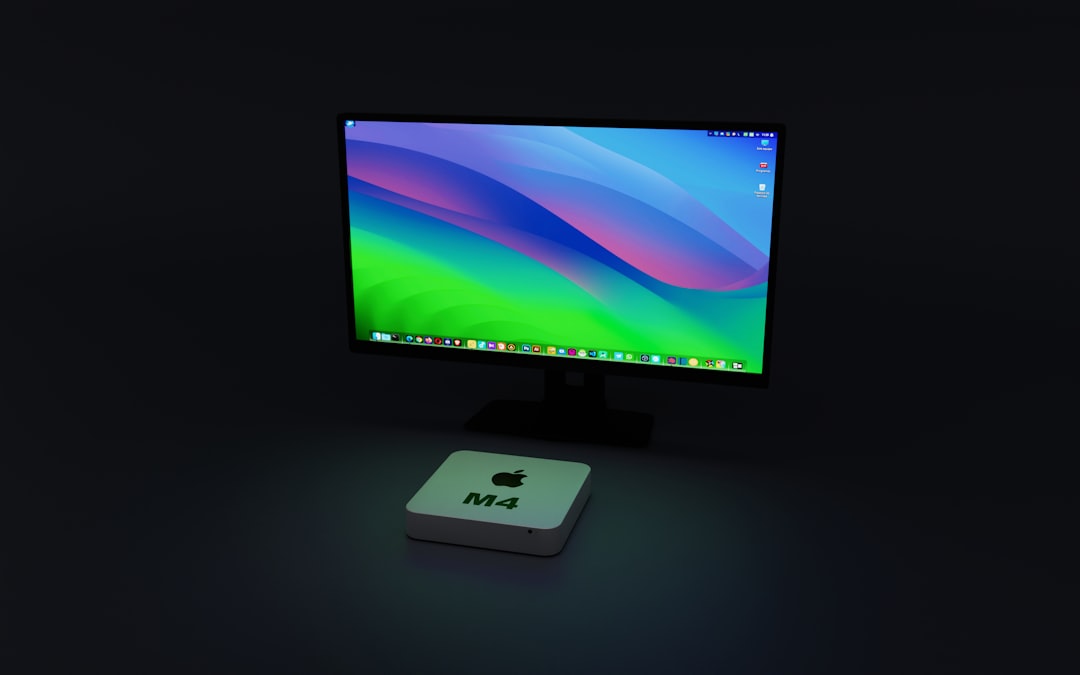
UBC's labs are making strides in integrating machine learning into robotics, driven by a collaborative effort across departments like Computer Science, Statistics, and Mathematics. Researchers are tackling practical challenges in various fields, including energy and manufacturing, by combining machine learning with established principles of control and optimization, particularly evident in the work of the Algorithms Optimization and Control Lab (AOCL). Their research utilizes machine learning, game theory, and control optimization to find solutions for complex real-world scenarios.
Additionally, UBC researchers are delving into human-robot collaboration (HRC) and using machine learning to enhance the design and cognitive aspects of robots working alongside humans. This research focuses on how machine learning can facilitate better human-robot interactions and task execution. While pursuing innovation in robotics, researchers at UBC are also acutely aware of ethical implications, with a specific focus on developing machine learning algorithms that protect the privacy of user data.
In essence, UBC’s robotics research showcases a broader commitment to advancing machine learning in ways that are both technologically innovative and mindful of the social implications of AI. This dual focus positions UBC as a leader in exploring how robotic systems can be developed to be both powerful and responsible tools.
UBC's research groups, spanning Computer Science, Statistics, and Mathematics, are deeply engaged in the intersection of machine learning and robotics. The Pan-Canadian AI Strategy, with its over 100 CIFAR AI Chairs established since 2017, has undeniably fueled this research drive across Canada. Notably, Dr. Park, an expert in privacy-preserving machine learning algorithms, joined UBC in 2021, bringing international expertise to the team.
It's becoming increasingly evident that AI, including machine learning and deep learning, is the driving force behind advanced robotic systems. UBC has launched a training initiative to cultivate a new generation of researchers equipped with the latest machine learning techniques for future AI advancements. One particular lab, the Algorithms Optimization and Control Lab (AOCL), is at the heart of many of these projects. They are pushing boundaries in areas like control theory, optimization, and computing, with specific applications in energy, manufacturing, and robotics. Their current work focuses on real-world challenges, leveraging optimization techniques, game theory, and machine learning to find solutions.
Machine learning is proving to be a critical component for progress in numerous fields, and robotics is no exception. There's a growing interest in human-robot collaboration (HRC) where machine learning techniques play a key role in designing efficient collaborative tasks and enhancing robots' cognitive abilities. It's becoming clear that a deeper understanding of the relationship between machine learning methods and task design is crucial for the future of human-robot collaboration, as indicated by systematic reviews in the field.
While there's undeniable progress, there are still many hurdles. It remains a challenge to create robots that can truly adapt to novel and unpredictable circumstances. The field of soft robotics, which relies on machine learning to regulate stiffness and flexibility, holds great promise but needs further refinement. Similarly, transfer learning holds the potential to significantly enhance robots' ability to generalize and learn new skills. However, much more research is needed before it can become widely applied.
Ultimately, the pursuit of more adaptable robots able to work safely alongside humans and navigate complex environments requires a multidisciplinary approach. While UBC's current efforts are quite promising, many obstacles remain in achieving truly autonomous robots capable of handling unpredictable scenarios. The field is full of exciting possibilities, but it’s important to acknowledge that the path forward requires constant development and improvement of current machine learning techniques to achieve full robotic potential.
UBC's AI Research 7 Key Projects Shaping the Future of Machine Learning - Biological Applications of AI Explored in University Projects
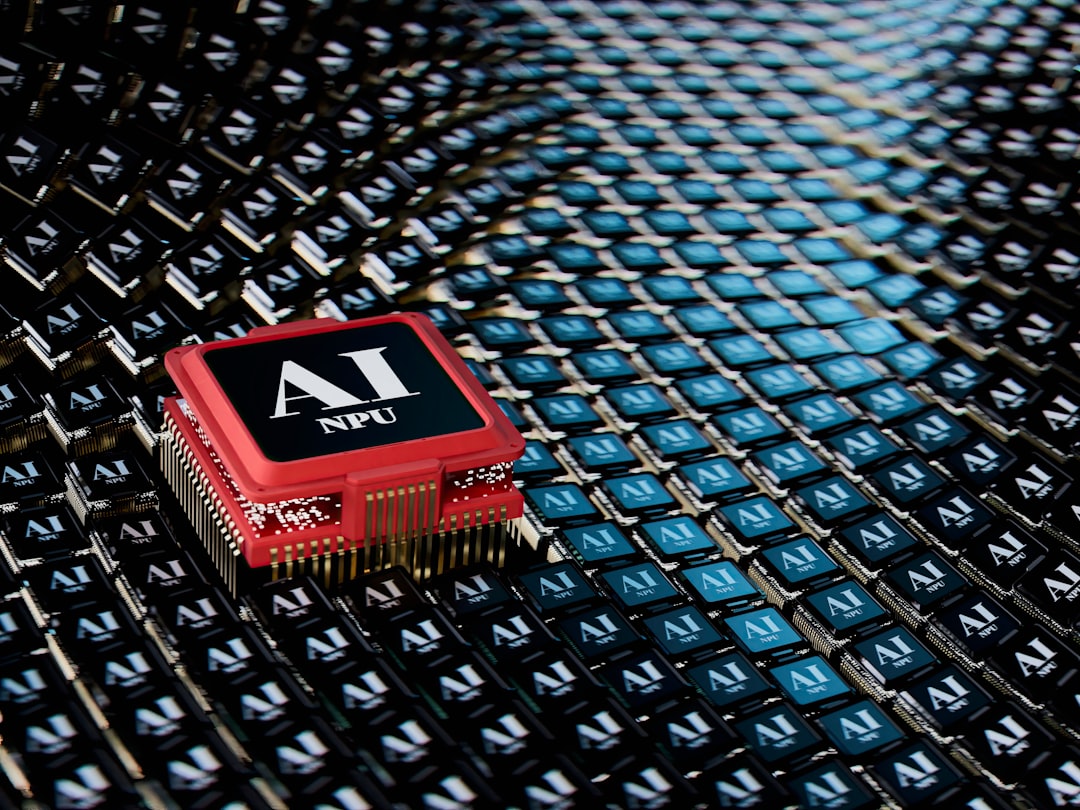
University projects are increasingly exploring the use of AI in biology, fueled by the explosion of biological data. Machine learning methods are being applied to solve complex problems in areas like predicting diseases, particularly in fields like mental health and gut microbiome research. Researchers are also delving into the fundamental building blocks of life, using AI for tasks like RNA structure prediction, a vital aspect of RNA stability and drug discovery efforts. This trend reflects a growing integration of AI across various biological disciplines, with the potential to accelerate advancements in both diagnostics and treatment.
Despite this promise, there are hurdles to overcome. AI's success in biology relies heavily on effectively managing and interpreting vast datasets. Finding ways to ensure AI models produce clear and reliable insights is crucial. Successfully harnessing the power of AI in biological research will likely depend on continued collaboration between computer scientists and biologists, along with the development of more rigorous analytical frameworks to tackle both technical and ethical questions that arise. The future of AI applications in biology appears bright, but depends on thoughtfully navigating the complex challenges inherent in this rapidly evolving field.
Researchers at UBC are pushing the boundaries of how we understand and interact with biological systems using AI. They're exploring how AI can enhance protein folding simulations, potentially revolutionizing drug discovery by predicting protein structures more accurately and quickly than traditional methods. This is a fascinating area, as the 3D structure of a protein is crucial for its function, and understanding this structure can be a major bottleneck in drug development.
Another interesting line of research involves using AI to analyze RNA sequencing data. This can lead to more accurate identification of gene expression, potentially unlocking new insights into genetic diseases and paving the way for more personalized medicine approaches. It's exciting to think about how this could help us understand the complex interplay between genes and disease.
Deep learning is also being used to decipher intricate biochemical pathways, essentially allowing scientists to visualize and predict cellular behaviors in ways that were previously difficult. This is a crucial step in bridging the gap between theoretical models and experimental validation, which can often be a challenge in computational biology.
The collaboration between AI researchers and neuroscientists is also noteworthy. They're employing machine learning to analyze large brain imaging datasets, hoping to gain a deeper understanding of cognitive functions and neurodegenerative diseases. This has the potential to greatly enhance our understanding of complex brain processes and lead to new diagnostic and treatment approaches.
One of the more innovative aspects of these projects is the creation of "digital twins" of biological systems. These AI models can simulate living organisms, providing a safer and more efficient way to test new drugs without extensive live animal testing. This has ethical implications and could drastically change how pharmaceutical companies develop and test new medications.
The university’s work on federated learning is especially intriguing within healthcare. This approach allows hospitals to collaborate on AI models without sharing sensitive patient data. This holds great promise for preserving patient privacy while also improving predictive capabilities in medical diagnoses and treatments.
The field of microbiome research is also benefitting from the integration of AI. It’s allowing for more in-depth analyses of gut health and could even lead to personalized dietary recommendations based on an individual's unique microbiome profile. This could have huge implications for public health and personalized nutrition.
Researchers are also investigating the use of reinforcement learning in synthetic biology. Here, algorithms are being used to teach engineered microorganisms to optimize their metabolic processes, which could lead to more efficient biofuel production and better waste management techniques. This could address some major environmental concerns and could potentially change our approach to resource management.
A crucial challenge that’s being addressed is the need for AI models in biology to be more transparent and understandable. The goal is to develop more interpretable algorithms that can explain their predictions, enhancing trust and facilitating the adoption of AI in clinical settings. This is vital if AI is to be truly integrated into healthcare and other applications.
UBC researchers are also making strides in using AI to predict evolutionary trends. They’re analyzing genomic data across various species to potentially reshape our understanding of how evolution works, which could have a significant impact on conservation efforts. Understanding the evolutionary history of different species is critical for protecting them, and AI may provide new tools for conservation biologists.
Overall, it's clear that the convergence of AI and biology has the potential to create a new era of scientific discovery, with significant implications for human health, the environment, and our understanding of the natural world. The research efforts at UBC highlight the immense potential of AI to address some of the most pressing challenges we face today. However, it's crucial to continue exploring the ethical and societal implications of these technologies to ensure that AI is developed and deployed in a responsible and beneficial manner.
UBC's AI Research 7 Key Projects Shaping the Future of Machine Learning - Quantum Computing and AI Integration Efforts Underway
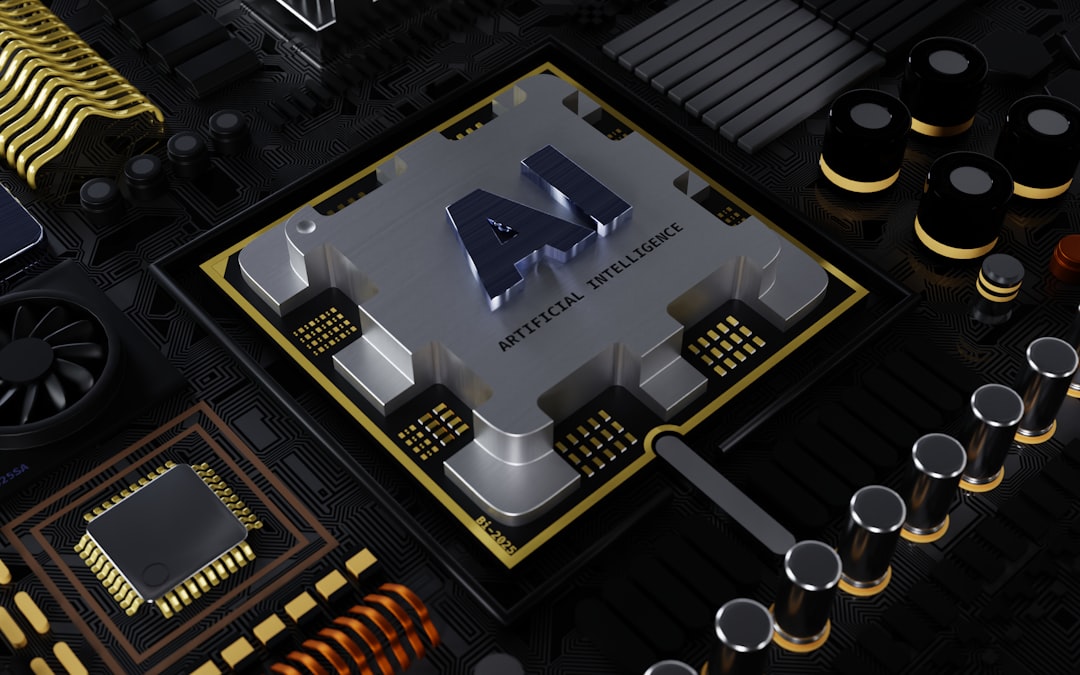
The intersection of quantum computing and artificial intelligence is a burgeoning field, often referred to as "Quantum AI," with the potential to revolutionize various industries. This integration aims to leverage the unique capabilities of quantum computing, particularly its speed and processing power, to enhance artificial intelligence applications, particularly within the context of Industry 4.0. This convergence is driving innovations and necessitating changes in how we manage these innovations. Scientists are delving into using quantum computing hardware like quantum chips for machine learning tasks, seeking to surpass the limitations of classical computing approaches and tackle more complex issues. This has the potential to accelerate progress in fields like drug discovery, where the ability to optimize processes could lead to solutions for significant global health problems. While promising, this merging of technologies also necessitates careful consideration of the ethical implications involved in developing and employing these powerful tools. The journey forward requires a balance of technological advancement and responsible development.
The potential of quantum computing to accelerate specific types of AI tasks, like optimization and large-scale data handling, is sparking a lot of interest. Quantum algorithms, like Quantum Support Vector Machines, are being explored for potentially speeding up tasks such as image and speech recognition, which traditionally rely on complex classification processes. There's also exploration of blending quantum neural networks with established AI models to improve their ability to generalize, potentially reducing the amount of data needed for training and enhancing predictive accuracy.
However, current quantum hardware faces limitations, including short coherence times for qubits and high error rates. These limitations currently hinder the broader adoption of quantum computers in practical AI applications. Nevertheless, researchers at UBC are investigating the use of quantum simulations to gain a better understanding of the internal workings of complex AI models. This could lead to the development of novel AI architectures that exploit the unique characteristics of quantum states for more efficient learning.
The quest for "quantum advantage" in AI, where quantum computers definitively outperform their classical counterparts, continues to drive this research area. Establishing concrete benchmarks and identifying practical use cases for near-term quantum applications is still a major hurdle. One interesting approach is the development of hybrid quantum-classical algorithms. These strategies aim to combine the strengths of both classical and quantum computing, allowing researchers to leverage existing AI systems while gradually incorporating quantum methods.
Currently, many quantum machine learning algorithms still heavily depend on classical techniques for data preparation, like noise reduction and transformations. This indicates that traditional methods will likely remain important in the development of future quantum-enhanced AI systems. The field is also exploring using quantum principles to improve federated learning frameworks. The goal is to securely distribute model updates in a way that protects the privacy of sensitive data, a critical issue in traditional machine learning setups.
While the potential benefits of quantum AI are compelling, the ethical and practical implications of its integration require careful consideration. Questions around accessibility and potential power imbalances arise as only those with access to advanced quantum resources may benefit from these technologies. These considerations will need to be central to the future development and deployment of these advanced systems.
UBC's AI Research 7 Key Projects Shaping the Future of Machine Learning - Healthcare Innovations Through Machine Learning at UBC
UBC is at the forefront of using machine learning to improve healthcare, moving beyond just developing algorithms to focusing on practical applications. Their research strives to bridge the gap between theory and practice, with projects like predicting tuberculosis risk in patients showcasing how machine learning can combine big data with clinical decision-making. This drive to implement AI solutions is evident in the collaboration across different departments at UBC, including the formation of the Centre for AI Decision-making and Action (CAIDA). The university's goal is clear: to improve healthcare within British Columbia and contribute to global advancements in this field through AI. However, challenges remain in understanding and interpreting AI predictions, a crucial aspect for widespread use in healthcare. While the potential is immense, there's a need to ensure that machine learning applications in medicine are transparent and easily understood by medical professionals to facilitate their adoption and ensure trust. UBC's initiatives demonstrate a wider trend within AI research, a focus on using it to solve real-world problems while carefully considering ethical implications.
UBC's researchers are pushing the boundaries of how machine learning can be used to improve healthcare, moving beyond the initial development stages and focusing on practical applications within the clinical setting. One notable research area involves leveraging machine learning to predict the risk of tuberculosis in patients, demonstrating how big data can be integrated into clinical management. This work exemplifies how AI can enhance decision-making in complex health scenarios.
The efforts at UBC are not confined to individual labs. Instead, there's a collaborative ecosystem across faculties, research groups, and external partnerships, all working to advance AI applications in healthcare. This collaborative approach is particularly evident in the Centre for AI Decision-making and Action (CAIDA), which is dedicated to investigating the broader implications of artificial intelligence in health-related contexts.
A central theme throughout UBC's AI healthcare research is improving the health and well-being of British Columbians, and contributing to global health advancements more broadly. Machine learning, with its ability to analyze vast quantities of data, offers new pathways for understanding disease progression, enhancing diagnostics, and optimizing treatment approaches.
A key challenge, and focus, is understanding causality within machine learning models in healthcare. If successful, these models could theoretically be used to answer complex questions about the potential impact of medical interventions. Researchers are grappling with how to build AI systems that can effectively answer the "what if" questions that are so crucial to evidence-based medicine.
Furthermore, UBC's projects are exploring the use of synthetic data to overcome limitations associated with using real patient data for training AI models. These efforts aim to both improve the capabilities of AI in healthcare while mitigating privacy concerns.
AI's influence in healthcare is clearly growing, driving shifts in clinical practices and how medical knowledge is generated and utilized. This leads to questions about how human medical intuition and insight needs to adapt alongside these AI powered advancements. UBC's AI research includes many different projects focused on applying machine learning to enhance the efficiency and coordination of healthcare delivery, particularly spanning different medical specialties. However, one has to wonder if the field can effectively move forward without careful consideration of how best to integrate these AI technologies into the human experience of healthcare in a way that is beneficial and ethically sound.
UBC's AI Research 7 Key Projects Shaping the Future of Machine Learning - Interdisciplinary AI Collaboration Accelerates Technology Development
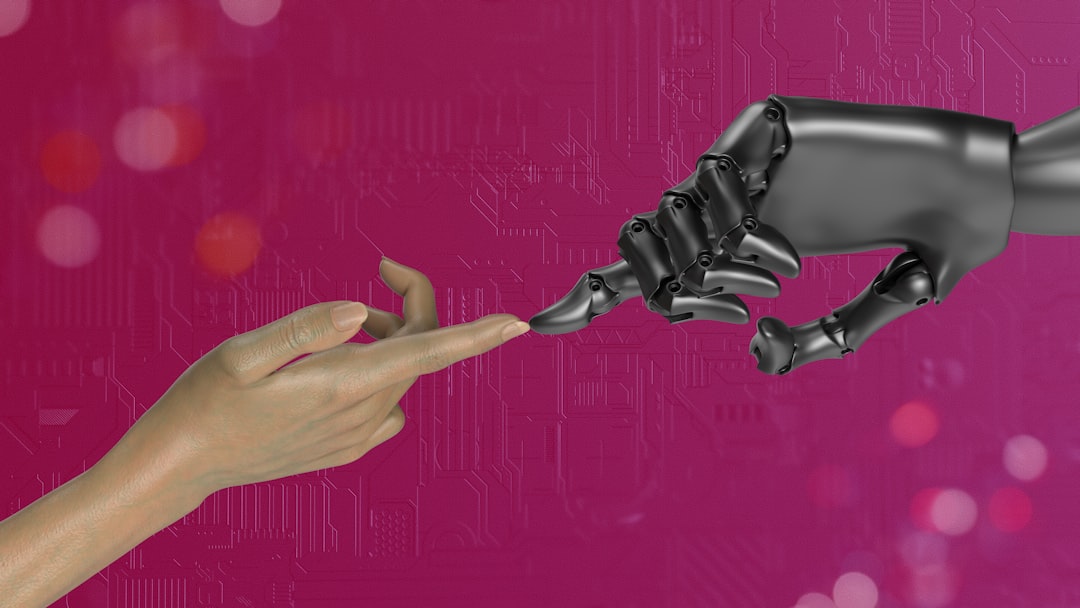
The convergence of diverse fields within artificial intelligence research is rapidly accelerating technological progress, exemplified by initiatives at universities like UBC. By bringing together experts from disciplines like computer science, mathematics, and biology, researchers can address complex problems more effectively. This interdisciplinary approach is crucial for creating AI systems that not only deliver powerful results but also operate in a way that is understandable and transparent. Understanding how AI systems arrive at their conclusions – known as decision explainability – is increasingly important for building trust and ensuring that these powerful tools are used responsibly. This collaborative spirit is vital to navigate the societal impacts and ethical considerations that come with advancements in AI. It allows researchers to anticipate and mitigate potential issues, paving the way for the development of AI systems that truly benefit society. As we move forward, fostering interdisciplinary collaboration will be key to unlocking the full potential of AI and ensuring its deployment is both innovative and beneficial.
The convergence of diverse academic disciplines within UBC's AI research is proving remarkably fruitful for accelerating technological development. We're seeing a fascinating synergy emerge as engineers, psychologists, and other experts pool their knowledge. This collaborative approach allows for innovative solutions that would be hard to achieve within isolated research silos, giving us a much richer landscape for problem-solving.
The competition for top AI talent is fierce, and UBC's recruitment strategy is a testament to this trend. Universities are increasingly recognizing that interdisciplinary collaborations are crucial for attracting the best researchers, and this push for collaboration may be a critical factor in driving long-term progress.
UBC's commitment to federated learning is a strong example of how these interdisciplinary efforts are translating into real-world applications. By enabling AI models to learn from decentralized datasets, we can gain the benefits of diverse data without compromising privacy, opening up possibilities that were previously unimaginable.
These collaborative research efforts frequently extend into multi-year projects that tackle complex technological hurdles. What's particularly exciting is that these partnerships often bridge academia and industry, leading to promising new technologies with strong potential for real-world impact.
Researchers across different faculties are increasingly using a rapid prototyping approach to AI development. This fast-paced experimentation and evaluation process creates an environment where concepts can be iterated on rapidly, allowing for adjustments and revisions based on direct feedback in a short timeframe.
Integrating insights from cognitive science, psychology, and neuroscience is enriching UBC's AI projects. By examining how humans think and make decisions, AI systems can be designed to better understand and interact with our world.
The emphasis on addressing real-world challenges is a core principle for UBC's AI research. It's encouraging to see a focus on developing AI solutions that aren't just theoretically sound, but also practically useful. This approach is crucial for bridging the gap between the lab and the actual needs of industry and society.
UBC's projects in healthcare often involve global partnerships, where AI is used to address worldwide health problems. This international lens fosters valuable exchange and allows research to focus on global needs, pushing the envelope in global health technology advancements.
The researchers at UBC understand that alongside technological advances, ethical considerations are paramount. They are actively involved in creating frameworks that help to ensure AI is developed responsibly, considering its social and ethical implications.
The interdisciplinary nature of UBC's research extends into the exciting world of quantum computing. They are exploring how quantum principles can enhance machine learning algorithms, addressing current limitations in processing and data handling. This effort positions UBC as a key player in the fascinating frontier of technology convergence, showcasing their drive to continually refine existing AI tools while looking to the future of computing.
UBC's AI Research 7 Key Projects Shaping the Future of Machine Learning - Industry Partnerships Enhance Real-World AI Applications
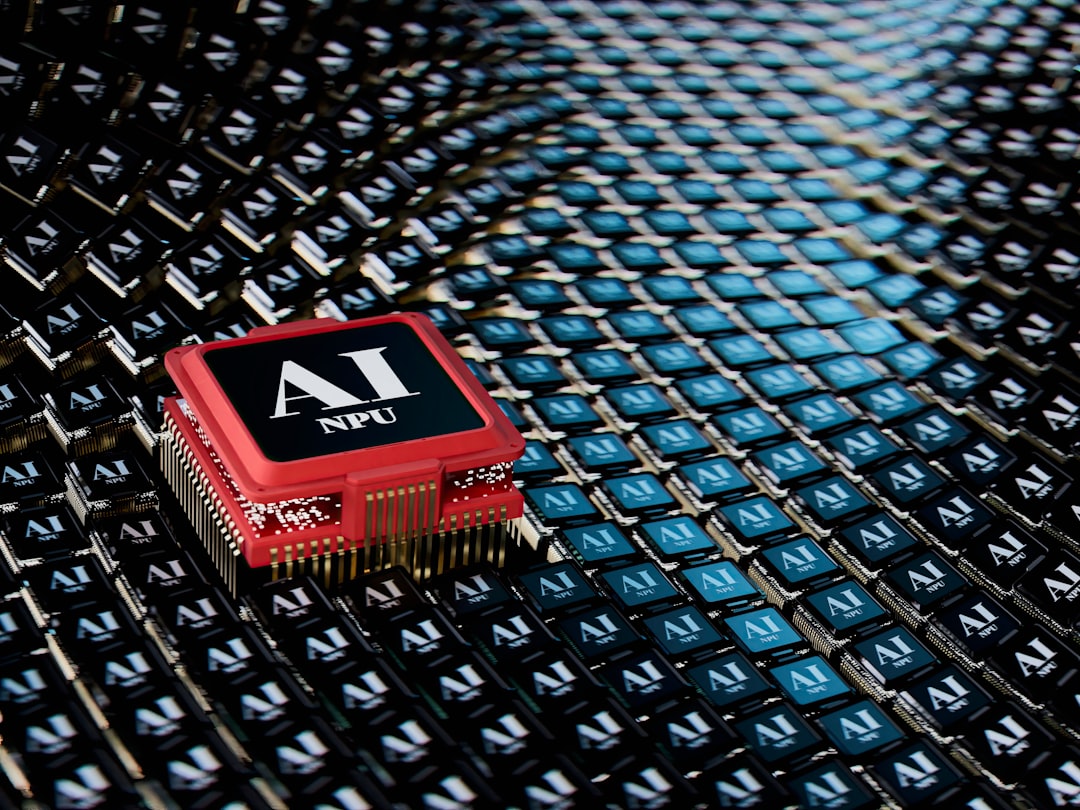
The integration of industry partnerships is a growing trend within UBC's AI research, proving crucial for translating theoretical advancements into tangible, real-world applications. By collaborating with businesses across various fields, researchers gain access to practical challenges and datasets that can inform and refine their AI models. This collaboration provides a valuable pathway to bridge the gap between theoretical AI concepts and the demands of specific industries, driving innovation in areas such as efficiency and productivity. These partnerships are not simply about resource sharing, but also crucial for navigating the complex ethical considerations surrounding AI implementation, ensuring that these powerful technologies are deployed responsibly and for the benefit of society. Moving forward, UBC's emphasis on combining strong AI research with industry needs, coupled with a commitment to tackling real-world problems, positions them to make significant contributions to both local and global communities. This approach highlights the importance of interdisciplinary work in developing AI, ultimately demonstrating its potential to become a transformative force in our world.
UBC's AI research benefits significantly from strong ties with various industries. By working closely with companies and organizations, researchers gain a deeper understanding of real-world problems that AI can help solve. This collaboration is particularly noticeable in the healthcare sector, where partnerships with local providers have allowed researchers to adapt and improve machine learning models within clinical settings, pushing the boundaries of predictive healthcare.
We're also seeing a growing trend of AI systems being developed with practical applications in mind, as shown by collaborations with tech companies in finance and logistics. This ensures that AI research remains relevant to ongoing business operations. Interestingly, UBC's partnerships aren't limited to for-profit ventures; they also extend to non-profits, highlighting a commitment to using AI for societal benefit, especially in areas like public health and community services.
These collaborations frequently involve joint grant applications between UBC researchers and industry partners. This shared-funding model allows for greater research with potential commercial applications and fosters a dynamic environment of shared risk and innovation. The diverse makeup of UBC’s AI research groups encourages a productive exchange of ideas. Insights from fields like psychology and biology are helping to shape AI applications, resulting in technologies that are more attuned to human needs and behaviors.
One of the most impactful aspects of these collaborations is the constant exchange between academics and industry professionals. This two-way feedback loop doesn't just validate research hypotheses, it also helps guide the direction of future AI research. Additionally, UBC has developed a system where graduate students work directly with industry partners, providing invaluable hands-on experience while also ensuring that research stays focused on real-world problems.
These collaborations have fostered an environment ripe for rapid prototyping. Researchers can develop and evaluate AI solutions in real-time, making the innovation process more efficient. Moreover, UBC’s commitment to developing AI in a transparent and responsible way is strengthened by these partnerships, where ethical considerations are prioritized. This fosters a shared understanding of responsible AI frameworks that consider both societal well-being and business goals.
It's clear that fostering relationships with different industries is a crucial element of UBC's AI research strategy. It keeps their work relevant and ensures that AI solutions developed at UBC have a tangible impact on the real world, across various sectors. While these partnerships bring many benefits, it's important to continuously assess the potential implications and ensure the ethical development and use of AI technologies remain central to their application.
Create AI-powered tutorials effortlessly: Learn, teach, and share knowledge with our intuitive platform. (Get started for free)
More Posts from aitutorialmaker.com: