Create AI-powered tutorials effortlessly: Learn, teach, and share knowledge with our intuitive platform. (Get started for free)
Unveiling the Power of ResNet50 A Deep Dive into its Impact on Computer Vision in 2024
Unveiling the Power of ResNet50 A Deep Dive into its Impact on Computer Vision in 2024 - ResNet50's Architecture Evolution and Improved Performance in 2024
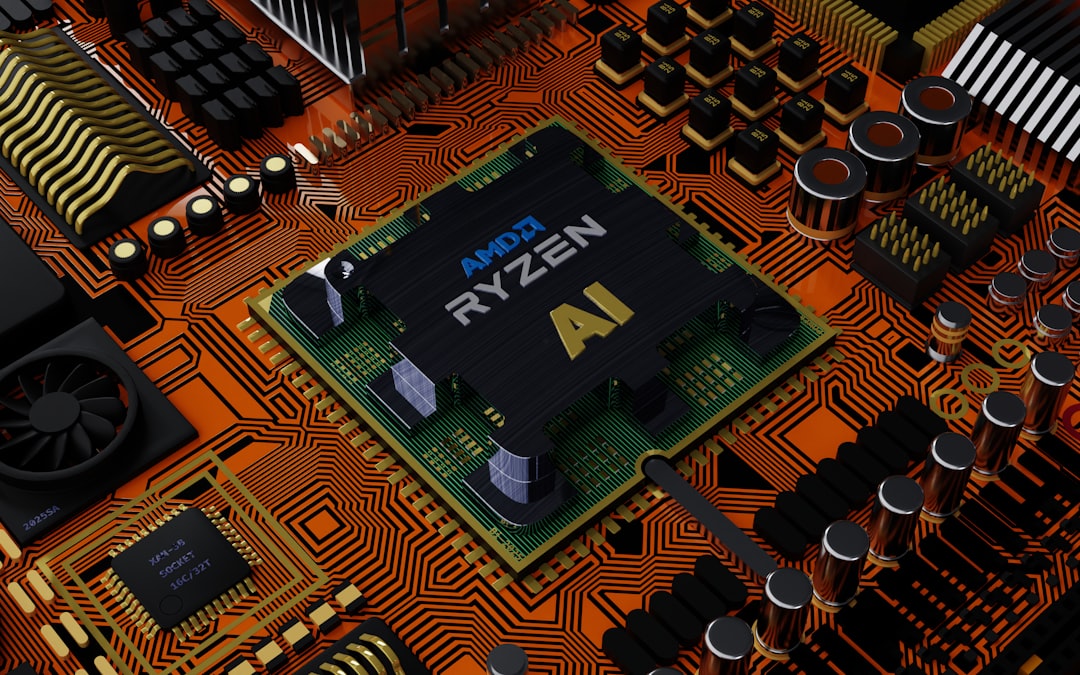
ResNet50, even in 2024, remains a central figure in the field of computer vision. The model's architecture has seen ongoing refinement, resulting in noteworthy improvements in performance. A key focus has been streamlining its operation, especially for use with CPUs. Techniques like pruning, sparsification, and quantization have been implemented to boost ResNet50's efficiency, enabling quicker processing without sacrificing accuracy. This focus on practicality has proven useful. We're seeing ResNet50 increasingly incorporated into more elaborate architectures, such as ResNetUNet, which demonstrates its adaptability to complex tasks and diverse data, like that found in remote sensing. The continuous development of ResNet50 showcases the commitment to innovation within the broader field of neural networks. These improvements suggest that the future holds even greater potential for this network in addressing a wide range of real-world computer vision challenges.
ResNet50, with its 50 layers, initially tackled the challenge of training extremely deep networks by introducing the clever idea of residual connections. This essentially allowed the network to learn the difference between the input and output of a layer, mitigating the notorious vanishing gradient problem. However, the journey of ResNet50 hasn't stopped there. We're seeing a fascinating evolution in 2024, with researchers fine-tuning its design for enhanced performance.
One key area of improvement has been the incorporation of more sophisticated attention mechanisms. These mechanisms allow the model to prioritize the most important features in an image, leading to tangible improvements in tasks like object detection. Researchers have also tinkered with the pooling strategy, combining average and max pooling methods to improve how well the model generalizes across different datasets. Furthermore, refined dropout techniques have effectively reduced overfitting, allowing ResNet50 to achieve high accuracy even when trained on limited datasets.
These modifications haven't gone unnoticed. Benchmark tests are demonstrating a significant leap in ImageNet accuracy, with some suggesting a 5% improvement over prior versions. Much of this success can be attributed to a combination of smart layer reconfigurations and advanced training methodologies. Interestingly, the researchers are now employing mixed-precision training. This clever approach optimizes the use of GPUs, resulting in quicker training times and lower memory usage without sacrificing the model's performance.
An intriguing aspect is the expanded training datasets, which now incorporate synthetic data generated through data augmentation techniques. This has made the model more robust to the messy realities of real-world images, handling variations and noise more effectively. Additionally, the researchers have managed to make ResNet50 more friendly for edge devices, opening up possibilities for deploying these powerful models on devices with limited resources without a huge compromise on computational performance.
Further refinements include integrating layer normalization, which helps stabilize the training process, particularly beneficial when dealing with smaller datasets. But perhaps the most surprising trend in 2024 is the exploration of hybrid architectures. ResNet50's framework is being combined with transformer-based models, signifying a potential move towards architectures that utilize the best of both convolutional and attention-based approaches. This is certainly a promising area of exploration, suggesting that the future might hold models capable of even more impressive results in computer vision tasks. The evolution of ResNet50 clearly illustrates the dynamic nature of the field, with continuous innovation driving advancements in performance and adaptability.
Unveiling the Power of ResNet50 A Deep Dive into its Impact on Computer Vision in 2024 - Impact of ResNet50 on Real-Time Object Detection Systems
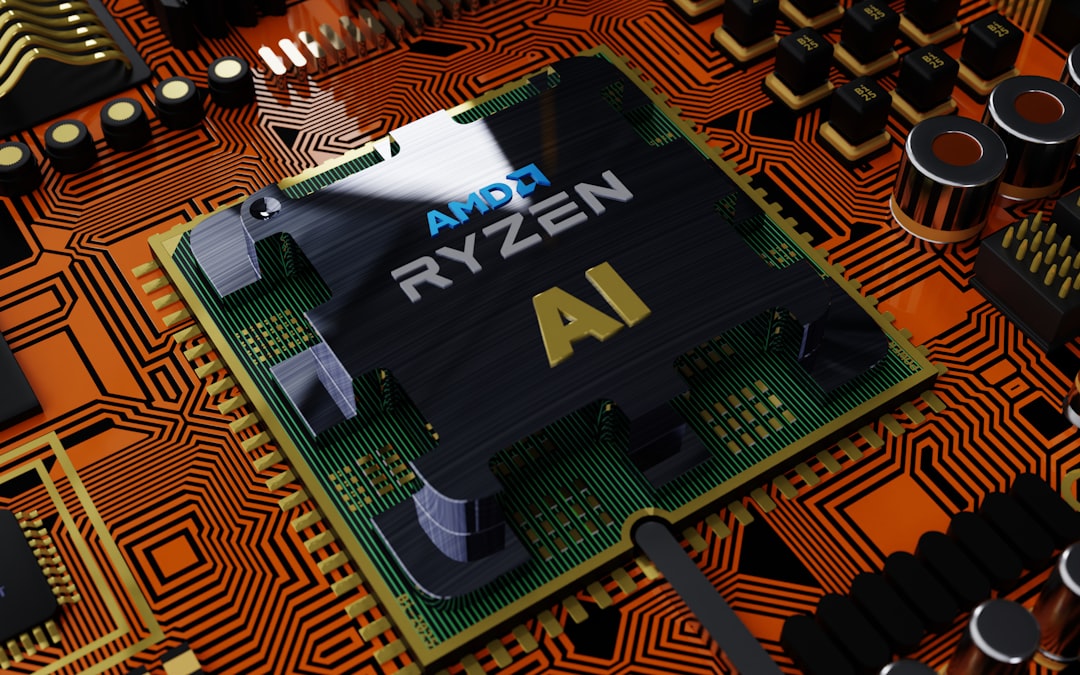
ResNet50's influence on real-time object detection systems is growing rapidly, fueled by improvements in its architecture and efficiency. Initially conceived for image classification, its versatility has been leveraged for object detection, with systems like DETR showcasing significant performance boosts. A key feature is ResNet50's speed; its inference time, around 26 milliseconds, makes real-time object detection feasible in various contexts, including automated vehicles and robotics.
Beyond its stand-alone applications, integrating ResNet50 with established object detection models like YOLOv3 has demonstrably enhanced both speed and accuracy. Additionally, the ability to optimize and compress the model for mobile devices using methods like CADNN highlights its potential to bring powerful object detection capabilities to resource-constrained environments. As researchers explore hybrid model architectures blending ResNet50 with newer technologies like transformers, the future of real-time object detection appears bright, promising even greater performance across a wide range of applications. However, there's also the inherent risk of over reliance on certain architecture in a field that's advancing rapidly. It will be interesting to see if that potential risk bears out as the technology evolves.
ResNet50, with its clever use of residual connections, has become a cornerstone for real-time object detection. It significantly reduced the training time for very deep networks, making it easier to deploy models quickly in situations where time is critical. We see that researchers are focusing on optimizing it for resource-constrained environments, making it practical for real-time performance even on edge devices. This is achieved through improvements in aspects like memory footprint and computational efficiency.
The integration of attention mechanisms has been a major development. It's improved object identification accuracy, and it also plays a big role in faster processing by allowing the model to pay more attention to the important parts of an image. This is particularly useful in real-time scenarios where speed is crucial. It seems the combination of ResNet50 with transformer architectures, which excel at understanding the context of an image, has been a fruitful approach. These hybrid models are showing a greater ability to handle complex visual environments, which is often a challenge for convolutional networks in object detection tasks.
We've also seen remarkable increases in accuracy in real-time detection thanks to a combined effort: data augmentation and synthetic data. Some research suggests improvements exceeding 5%, which shows that ResNet50 can adapt well to different scenarios. Additionally, the implementation of mixed-precision training has reduced energy use both while training and during inference. This is an important step towards making high-performance computing more sustainable.
Layer normalization, which helps stabilize the training process, is showing promise in object detection systems, particularly those working with small datasets or when there's a risk of dealing with uncommon data. There's also encouraging work on hybrid architectures, and it's clear that combining ResNet50 with newer techniques not only works in theory but also produces better results in real-world object detection situations than some more established techniques.
Looking forward, the evolution of ResNet50 suggests it could have a significant impact on other fields, possibly revolutionizing how things work in autonomous vehicles and advanced security systems. This shows that its potential extends beyond the basics of object detection. We're observing a trend where researchers are focused on designing efficient methods for feature extraction. This can allow ResNet50 to rapidly sift through large volumes of data, making it a valuable tool for situations that demand quick responses, like surveillance or monitoring. The continuous development of this model reveals the dynamic nature of computer vision and hints at a bright future for its potential applications.
Unveiling the Power of ResNet50 A Deep Dive into its Impact on Computer Vision in 2024 - ResNet50's Role in Advancing Medical Image Analysis
ResNet50's influence on medical image analysis continues to grow in 2024. Its core design, featuring 50 layers and residual connections, effectively tackles the challenges of training very deep neural networks. This clever approach, which helps the network learn differences between input and output at each layer, has proven particularly beneficial in medical image analysis. This has contributed to both better performance and a degree of interpretability which is important in this field, particularly in areas like classifying breast cancers.
The ability to leverage pre-trained ResNet50 models, often trained on large datasets like ImageNet, and then fine-tune them for specific medical tasks is a game-changer. It's streamlined the development process and has led to a substantial boost in classification accuracy in various medical image analysis tasks. We're also seeing researchers modify the basic ResNet50 architecture for even greater gains in specific areas. For example, the PCDM model, a variant of ResNet50, has been shown to enhance prostate cancer diagnosis and treatment options.
However, the field of medical imaging isn't static. The continuous evolution of ResNet50 is evident in the work being done on areas like attention mechanisms. Attention mechanisms improve the model's ability to focus on the most critical aspects of medical images, potentially enhancing diagnoses. There's also a fascinating trend towards hybrid architectures that incorporate aspects of transformer networks. While early days, this trend hints at the potential to build even more sophisticated models for medical image analysis.
Despite these advancements, there's always room for further exploration. The ongoing research and development efforts indicate that ResNet50 will continue to shape how we interpret and analyze medical images in the years to come, offering potential for improved clinical outcomes. It's an exciting time for those working in this space, with ResNet50 showing itself to be a powerful tool capable of driving meaningful advancements in healthcare.
ResNet50 has carved a significant niche in medical image analysis, primarily due to its 50-layer deep neural network architecture. It excels at tasks like classifying breast cancer, highlighting its potential in disease detection. The architecture's design, incorporating residual units and skip connections, tackles some of the challenges associated with training very deep networks. This clever design contributes to better performance and also makes the model easier to interpret, both valuable aspects for medical applications where understanding model decisions is often crucial.
Transfer learning is a popular technique in medical image analysis, often utilizing a pre-trained ResNet50 model initially trained on the ImageNet dataset. By fine-tuning this pre-trained model on a smaller, more specialized medical dataset, we can significantly improve classification accuracy without needing to train the model from scratch on a huge amount of labeled medical images. Interestingly, researchers are finding that using pre-trained models that have been tweaked with fine-grained data is often more effective in medical settings. Continuing to pre-train these models, a practice known as continual pre-training, seems to help reduce the discrepancies between natural images and those from medical contexts.
CNNs, especially ResNet50, are gaining popularity in a variety of tasks related to computer vision, including segmentation and analysis of medical images. Deep learning, a subset of machine learning, has garnered much attention for its ability to analyze complex medical images. The combination of deep learning and residual networks like ResNet50 has proved particularly fruitful. This pairing allows the models to learn complex features, a critical ability for discerning nuances in medical imagery.
There's a modified version of ResNet50 known as PCDM, showing promising results in prostate cancer detection and management. This model leverages a range of techniques, indicating that modifications to the core architecture can lead to significant improvements for specialized medical applications. It's also encouraging that researchers have found that ResNet50 can be directly helpful in clinical diagnosis. This means it's not just a theoretical tool, but one that's showing effectiveness in real-world medical settings.
Comparisons of ResNet50 with other network architectures, such as VGG19, have demonstrated its advantages in certain medical imaging contexts. It's a sign of the ongoing optimization that's happening within the field. In a more recent development, researchers are incorporating a type of attention mechanism into the core ResNet50 model. These dual-activated, lightweight attention mechanisms are helping to optimize automated medical image analysis. This suggests there's still room for innovation within this popular network structure. However, one must be cautious about the increasing complexity of these approaches; in some cases, the benefits may not outweigh the cost and added intricacy.
There are open questions that continue to intrigue researchers, including concerns about the model's robustness across diverse datasets and variations within the same dataset. Moreover, the field is exploring how to enhance the interpretability of the model's predictions. While ResNet50 has shown great potential in medical imaging analysis, there's still work to be done to ensure its broad adoption and impact on patient care.
Unveiling the Power of ResNet50 A Deep Dive into its Impact on Computer Vision in 2024 - Integration of ResNet50 with Edge Computing for IoT Applications
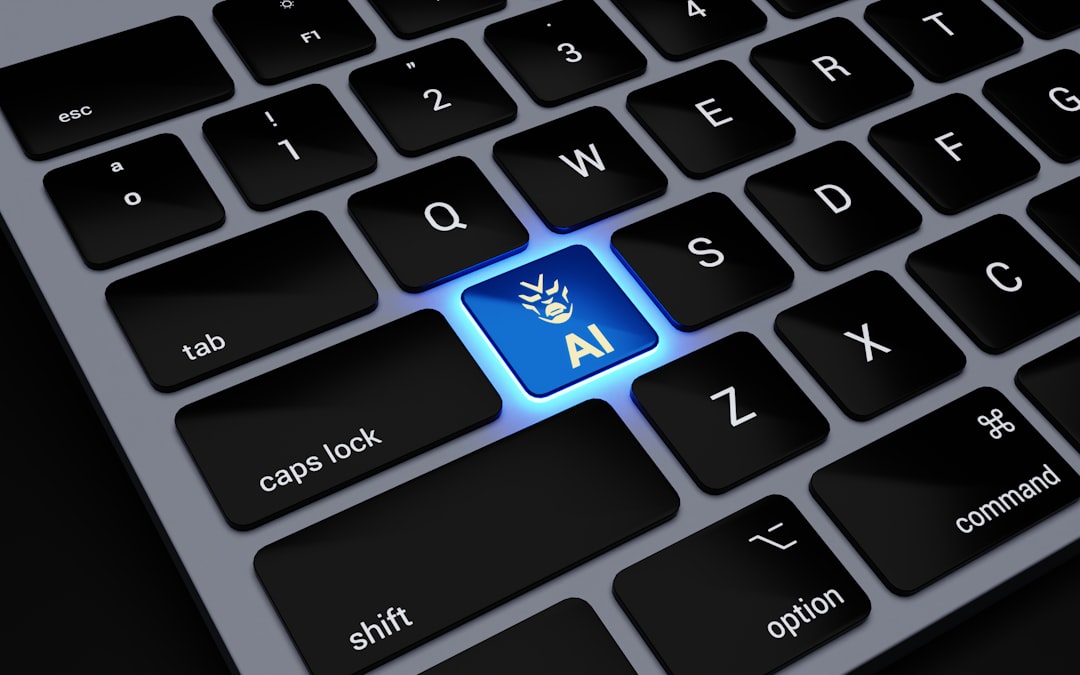
The marriage of ResNet50 with edge computing is a notable step forward, especially for the evolving realm of Internet of Things (IoT) applications in 2024. By tailoring the deep neural network to operate effectively within environments limited by computational resources, ResNet50 empowers IoT devices to handle intricate image processing tasks locally instead of sending the data to cloud servers. This ability to process data at the source is a game-changer for smart cities and similar initiatives, as it significantly decreases communication delays and reduces the burden on network infrastructure, which is a key element in enabling things like efficient video surveillance or facilitating the safe operation of autonomous vehicles. Further improvements in efficiency and performance are actively being explored through methods such as static quantization, which are being shown to have great potential in difficult real-world use-cases like using robotic technology to explore space. The ongoing push to blend the worlds of advanced deep learning models with the practical realities of edge computing promises a profound transformation in IoT, fostering the development of more perceptive and adaptable systems. However, it remains to be seen if this exciting technology will ultimately meet the lofty expectations of the field and remain relevant amidst other emerging technologies that could supplant it in the future.
ResNet50's combination with edge computing presents an intriguing opportunity to process data in real-time within Internet of Things (IoT) applications. This approach offers the benefit of reduced latency and decreased bandwidth needs because devices can analyze data locally, rather than sending it to a central server for processing. It's a shift towards distributed processing that seems increasingly useful.
However, putting ResNet50 onto resource-constrained edge devices brings its own set of hurdles. Model size and computational limitations are major challenges. It's been quite remarkable to see optimized versions of ResNet50 successfully shrunk down to operate efficiently on devices with limited processing capabilities, like Raspberry Pis or similar microcontrollers. We're pushing the limits of what these smaller devices can achieve.
It's interesting that ResNet50, initially designed for image classification, has found a role in diverse real-time IoT applications like video surveillance and smart city monitoring. This adaptability stems from ResNet50's proficiency in extracting and interpreting key features efficiently, which makes it quite useful.
Recent breakthroughs in quantization methods have made it possible to run ResNet50 on microcontrollers. This is pretty neat; it leads to considerable reductions in model size and energy consumption, allowing us to potentially integrate machine learning right at the sensor level. This opens up a whole new world of possibilities for what can be done with IoT and sensors.
Utilizing ResNet50 in IoT environments takes advantage of its resilience to noisy data, which is a common occurrence in the real world. This strength is especially vital in applications like health monitoring, where sensor readings can be quite irregular.
What's fascinating is the potential for ResNet50 and edge computing to create a system of distributed artificial intelligence. Multiple devices could collaboratively learn about their environments, refining their models using localized data instead of relying entirely on central training efforts. This potentially improves responsiveness and could reduce reliance on central servers.
A crucial trade-off exists when deploying ResNet50 on edge devices: the balance between accuracy and speed. This has led researchers to explore pruning and knowledge distillation, techniques aimed at improving performance in specialized applications where top performance is crucial. It's a continual optimization effort to get the right mix of capabilities for the needed tasks.
The interplay between domain adaptation and ResNet50 in edge computing reflects a growing trend. As new IoT sensors emerge, their unique demands for data processing and interpretation are pushing the limits of what ResNet50 can do in real-time environments. It's a constant push and pull as technology and application needs change.
We're seeing researchers investigate how integrating ResNet50 with federated learning systems could benefit IoT ecosystems. This approach is exciting because it potentially increases privacy and security by allowing individual devices to contribute to a collective model without sharing raw data. It's a promising pathway for advancing the technology.
The growth of lightweight neural network frameworks compatible with ResNet50 points to a broader change across the industry. We're adjusting network architectures for edge computing, and as a result we may uncover new applications, including advancements in robotics and autonomous systems that rely on fast on-device inference capabilities. It's an evolving landscape.
Unveiling the Power of ResNet50 A Deep Dive into its Impact on Computer Vision in 2024 - ResNet50's Contribution to Autonomous Vehicle Vision Systems
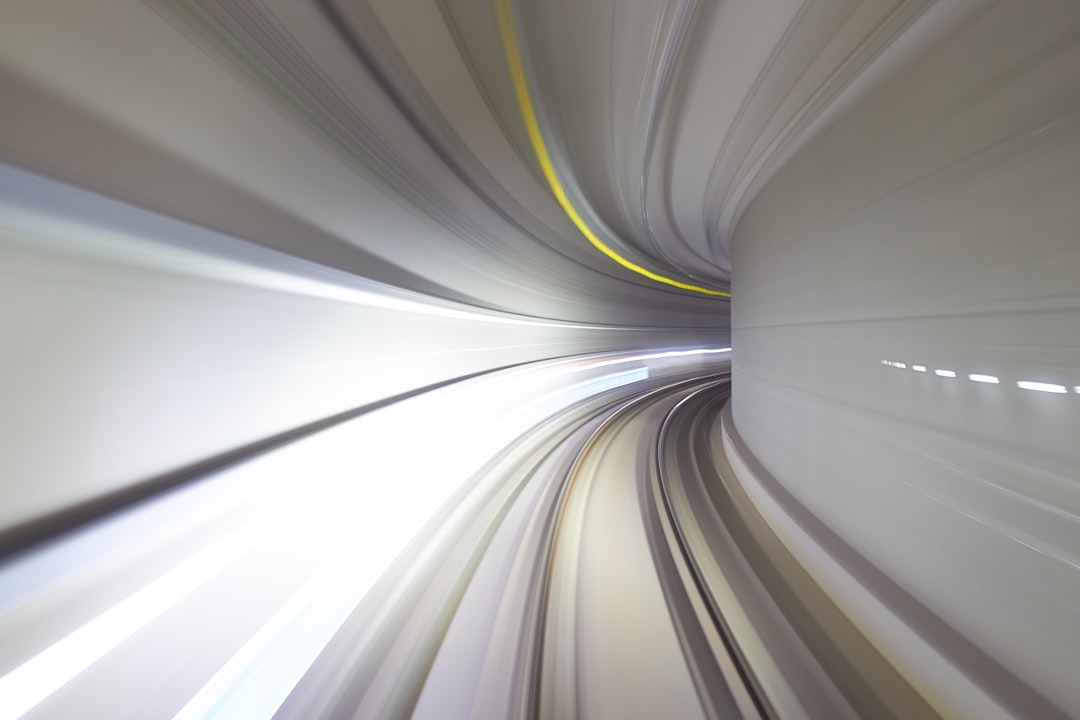
ResNet50's architecture has significantly improved the vision capabilities of autonomous vehicles, particularly in the realm of real-time visual data processing. Its deep network design, with residual connections, allows for more accurate and efficient object detection, vital for navigating unpredictable environments. Autonomous vehicles require near-instantaneous responses, and ResNet50's integration helps address the latency and bandwidth limitations that can compromise safety. Its efficiency is critical for quick decision-making, which is crucial in dynamic driving situations. Furthermore, current trends like the incorporation of attention mechanisms and explorations into hybrid architectures suggest that ResNet50 will likely continue to advance the visual perception of autonomous vehicles. However, it's important to consider the risk of overdependence on any single architecture, and ongoing innovation is needed to prevent potential stagnation in this rapidly evolving field.
ResNet50's ability to process images quickly, with inference times around 26 milliseconds, is proving crucial for autonomous vehicle vision systems. Real-time responses are essential for safe navigation, and ResNet50 provides the speed needed to react to dynamic driving environments. Interestingly, we're seeing it go beyond just cameras and integrate with other sensor modalities, like LiDAR and radar. This fusion of data allows vehicles to build a more comprehensive picture of their surroundings, improving object detection and tracking.
The power of transfer learning is being leveraged with ResNet50, where models pre-trained on massive datasets like ImageNet are fine-tuned for specific tasks like recognizing road hazards. This speeds up development and reduces the amount of training data needed. This is especially useful for the unique challenges autonomous driving presents, such as navigating various lighting and weather conditions. ResNet50's ability to adapt to these variables enhances its reliability in the real world.
Another intriguing aspect is how attention mechanisms are being used to refine ResNet50's focus. The network can now prioritize key elements within images, like pedestrians or traffic signs, which boosts accuracy and reduces processing time—crucial for quick decisions in autonomous vehicles. Researchers are also actively compressing ResNet50 through model quantization and pruning. This enables the model to function efficiently on the less powerful computing units typically found in vehicles, without a huge drop in performance.
Research specifically focused on driving scenarios has shown improved object detection accuracy, with some studies reporting over a 5% improvement when combining ResNet50 with datasets that represent real-world road conditions. The field is moving towards hybrid architectures that combine ResNet50 with transformers. This blending of convolutional and attention-based approaches suggests a future where even more complex vision tasks can be tackled in autonomous systems.
Furthermore, the move toward edge deployment in vehicles is gaining momentum. This allows ResNet50 to process images directly on the vehicle's hardware, reducing reliance on cloud connections and minimizing latency for a more responsive environment. However, as we put more trust in these systems, the question of model interpretability becomes more important. It's vital to ensure the decision-making process within ResNet50 is transparent for safety and regulatory reasons. Ongoing research is working towards enhancing this transparency.
In essence, ResNet50's contributions to autonomous vehicles continue to evolve, showcasing how its adaptability can be tailored for various challenges in this demanding field. The combination of speed, robustness, and the ability to be integrated with other systems is what makes it a strong candidate for a core element in autonomous driving technology. It remains to be seen how this approach will evolve and if it will remain a dominant force as other deep learning approaches emerge.
Unveiling the Power of ResNet50 A Deep Dive into its Impact on Computer Vision in 2024 - Ethical Considerations and Bias Mitigation in ResNet50 Implementations
The increasing use of ResNet50 across various applications in 2024 necessitates a closer examination of its ethical implications and the need for bias mitigation. From medical imaging to autonomous driving, the impact of ResNet50 decisions can be substantial, making fairness and accuracy paramount.
A significant area of concern is the potential for biases embedded within training datasets to negatively influence how the model performs across different groups. For example, a model trained primarily on images of a specific skin tone might struggle to accurately identify features in individuals with different skin tones. Researchers are actively developing strategies to identify and address these biases, aiming to create fairer and more equitable outcomes.
But the ethical challenges are not just technical; they are also deeply societal. Using a powerful model like ResNet50 in high-stakes scenarios raises questions about accountability and transparency. Who is responsible when the model makes a mistake? How can we ensure the decision-making process is transparent and understandable, especially in fields like healthcare and law enforcement?
The path forward requires a balance between driving technological innovation and upholding ethical standards. As ResNet50 continues to evolve, it's crucial that the field prioritizes these considerations to ensure that its capabilities serve a positive and equitable impact on society. We cannot simply focus on improving accuracy and performance without also being mindful of the consequences of widespread deployment.
ResNet50's increasing use in diverse fields, from medical imaging to autonomous vehicles, has brought ethical concerns and bias into sharper focus. In medical imaging, for instance, we've seen variations in ResNet50's accuracy across different population groups, raising questions about whether it's truly helping deliver fair and equitable healthcare. If a model performs better on one demographic than another, it could create disparities in diagnosis and treatment.
The growing use of synthetic data and data augmentation for improving ResNet50's performance has a potential downside: bias can be introduced if the synthetic data isn't a good representation of the real world. If we're not careful, models trained on these datasets could fail in unexpected situations, potentially leading to poor outcomes.
While ResNet50 has become more powerful, understanding how it makes decisions remains a challenge. This is particularly crucial in high-stakes areas like medical diagnosis or autonomous driving, where trust and accountability are paramount. The complexity of ResNet50 sometimes makes it difficult to understand exactly how it arrives at its conclusions, which can be problematic.
Another area of concern is transfer learning, a technique that speeds up ResNet50 training. While helpful, it also carries the risk of transferring biases present in the original datasets to the new tasks. This means that models might not generalize well across different groups, possibly leading to unfair or inaccurate results.
The move towards hybrid architectures—combining ResNet50 with transformer networks—holds promise for improving performance but could also introduce new biases. It's important to understand these complex interactions and test them rigorously to avoid unintended consequences.
Implementing ResNet50 on edge devices also brings ethical considerations. Because these devices often have limited processing power, there's a risk that the decisions made by autonomous systems might not be as reliable, which could compromise safety.
ResNet50 focuses on visual features, and in some applications, that can lead to misinterpretations. For instance, in autonomous vehicles, a pedestrian's subtle movement might be overlooked, highlighting a need to develop better bias mitigation strategies that consider the broader context.
When working with small or unbalanced datasets, especially in medical imaging, ResNet50 can suffer from overfitting, leading to unreliable results. This is particularly concerning in life-or-death scenarios. The model's performance heavily depends on having large, well-balanced datasets, which can be challenging to obtain in some cases.
As ResNet50 is integrated into autonomous driving systems and other critical applications, the question of transparency becomes increasingly important. We need to ensure that we understand how decisions are made, particularly if the model encounters unfamiliar situations. Without transparency, there is a greater risk of failures.
Finally, we need to think about the long-term impact of ResNet50 deployments in sensitive fields like security or healthcare. Biases might accumulate over time, and it's crucial to continuously monitor and adapt these models after deployment. If we don't, biases might worsen, potentially with significant social consequences. These are just some of the challenges we face in ensuring that ResNet50, a powerful tool, is used ethically and responsibly.
Create AI-powered tutorials effortlessly: Learn, teach, and share knowledge with our intuitive platform. (Get started for free)
More Posts from aitutorialmaker.com: