Create AI-powered tutorials effortlessly: Learn, teach, and share knowledge with our intuitive platform. (Get started for free)
Analyzing the Evolution of Generative AI Curricula From Basics to Advanced Applications in 2024
Analyzing the Evolution of Generative AI Curricula From Basics to Advanced Applications in 2024 - Foundational Concepts in Generative AI Curricula 2024
Generative AI curricula in 2024 are experiencing a shift towards incorporating more sophisticated techniques and applications. Curricula now commonly feature advanced models like GPT-4 and multimodal approaches, demanding a deeper understanding of underlying principles such as autoregressive modeling. This evolution necessitates a move beyond simply technical skills to encompass a wider range of disciplines. Integrating generative AI concepts into subjects like computer science, ethics, and the social sciences is becoming increasingly vital, fostering a more holistic understanding and promoting crucial skills like critical thinking and creativity. Recognizing the potential impact of generative AI, academic institutions are actively planning and allocating substantial resources to AI-driven educational initiatives over the coming years. The continuous development of generative AI necessitates the creation of robust educational frameworks to ensure students are well-prepared to navigate the multifaceted implications of this technology, encompassing both its potential and associated challenges.
The foundations of generative AI education in 2024 are undergoing a subtle yet significant shift. We're seeing a growing push to bridge the gap between artificial and human intelligence by incorporating principles from cognitive science. The idea seems to be to make AI interactions feel more natural and intuitive, a fascinating challenge.
Furthermore, there's a noticeable emphasis on the ethical implications of training these models. This suggests a move away from a purely technical approach towards a more responsible deployment of AI. It's encouraging to see this focus, though whether it will effectively address the potential downsides remains to be seen.
Advanced neural networks, particularly transformer models, have become mainstream in these curricula. This makes sense given their ability to handle complex language and ambiguity. However, I wonder if this emphasis risks neglecting other valuable architectures, potentially limiting the creativity and exploration of students.
Another trend we're observing is the increased use of unsupervised learning in introductory courses. This approach makes generative AI more accessible by removing the dependence on extensive labeled datasets. However, it's important to ensure students also get a strong foundation in supervised methods, as many real-world applications rely on this type of training.
There's also a push for more user-centric model development, where students learn how community feedback is used to fine-tune generative models. This is a welcome change, encouraging a more interactive and collaborative approach to AI design. But it also raises questions about potential biases introduced through community feedback and how those can be mitigated.
We're seeing more cross-disciplinary connections, drawing insights from fields like linguistics and psychology to better understand the semantic nuances of AI-generated content. This is encouraging, as a richer understanding of language and communication is critical to creating truly useful generative models.
Interestingly, many programs are adopting open-source tools and frameworks. This provides a great opportunity for hands-on learning and encourages collaboration within the field. However, the reliability and quality of open-source tools can be a concern, so careful curation and evaluation are necessary.
Simulations of generative AI in various industries are becoming a common part of education. This provides a good bridge between theoretical knowledge and practical applications, helping students understand real-world limitations and challenges. Yet, I also question if these simplified simulations accurately reflect the complexity of real-world scenarios.
The concept of adversarial training is being integrated to build models resistant to manipulation and capable of producing reliable outputs. This is a vital development for building trustworthy AI systems. However, it's important to note that adversarial training is a complex area and can introduce new complexities to model design and training.
Finally, we're witnessing an increasing focus on the hardware considerations of generative models. Students are now learning about computational constraints and the resources needed for deploying these models at scale. This is important for practical applications, but it's also crucial that curricula don't lose sight of the broader implications and societal impact of this technology.
Analyzing the Evolution of Generative AI Curricula From Basics to Advanced Applications in 2024 - Advanced Natural Language Processing Techniques in Educational AI
Advanced Natural Language Processing (NLP) techniques are significantly altering the educational landscape. Large Language Models (LLMs), particularly recent iterations like GPT-4, demonstrate a major leap forward in understanding and producing human-like text. This has profound implications for educational AI, particularly in automating tasks like question generation and evaluating student responses. We are witnessing a shift towards integrating more sophisticated NLP capabilities into educational applications. This includes leveraging LLMs to enhance human-machine communication and the incorporation of multimodal features, allowing for richer and more diverse learning experiences through text, images, and audio.
However, the rapid advancement and widespread adoption of these advanced NLP techniques in education necessitate a careful consideration of ethical implications. Issues such as potential biases embedded within these models, data privacy concerns, and the broader responsibility for their implementation need to be addressed. Future educational AI curricula must integrate a deeper understanding of the cognitive aspects of NLP, encouraging critical thinking around the design, deployment, and societal impact of these tools. Furthermore, a more interdisciplinary approach encompassing areas like psychology, linguistics, and even philosophy, will be critical in ensuring that NLP technologies are used thoughtfully and responsibly within educational settings. This will facilitate a more nuanced understanding of how advanced NLP impacts both the learning process and the larger educational ecosystem.
The field of natural language processing (NLP) has seen remarkable progress, with models now demonstrating impressive abilities in comprehending context and subtle meaning. This development opens up exciting possibilities for enhancing teaching methods, as AI can generate responses that mimic human conversation and offer more tailored learning experiences. However, the sophisticated transformer architectures driving these advances often demand substantial computing power, raising questions about the feasibility of implementing them in educational settings with limited resources.
NLP techniques are increasingly being used to analyze sentiment in student interactions, allowing generative AI to tailor its feedback to match the student's emotional tone. This presents a potentially transformative opportunity to revolutionize how educators provide feedback, but it also requires careful consideration of how AI should handle sensitive emotional information.
Furthermore, there's a growing emphasis on designing NLP models with multilingual capabilities. This is a crucial development for broadening access to education globally, but it also presents challenges in ensuring the accuracy and preservation of linguistic nuances across various languages. Bridging this gap and preventing loss of cultural richness in AI-driven educational tools is vital.
Integrating concepts from cognitive science into NLP algorithms aims to make AI interactions more intuitive and engaging for learners. This approach, while promising, highlights the ongoing challenge of translating complex human cognitive processes into the structured logic of AI algorithms. Successfully replicating the rich tapestry of human thought within a computational framework remains a major hurdle.
While NLP has made strides in generating high-quality text, it raises important concerns about the authenticity of student work. With the increasing accessibility of tools capable of producing convincing responses, ensuring the integrity of educational assessments becomes more challenging. Maintaining academic honesty in this evolving landscape is an issue educators need to address carefully.
Reinforcement learning-based NLP models are gaining traction in education, enabling systems to adapt more dynamically to student interactions. However, these models can introduce a level of unpredictability into responses, making it difficult to fully control the learning experience. Balancing adaptability with reliability will be a critical area for future development.
The ethical implications of using NLP in education are paramount. Biases present in the data used to train these models can inadvertently perpetuate existing inequalities in educational outcomes. Recognizing and mitigating these biases is essential for ensuring fairness and promoting equity in AI-assisted learning.
It's encouraging to see that some educational frameworks are now emphasizing the importance of teaching students about the limitations of NLP models. Highlighting potential failures and inaccuracies in AI-generated content strengthens critical thinking skills and promotes healthy skepticism about technology's role in learning.
Current research focusing on common sense reasoning holds great promise for advancing NLP capabilities in educational settings. If AI systems can not only generate text but also understand the underlying principles of logic and reason, it could profoundly transform the way assessments are designed and conducted.
In essence, the evolution of advanced NLP techniques within educational AI presents both tremendous opportunities and complex challenges. It's clear that educators, researchers, and developers must work collaboratively to navigate these complexities and ensure that AI serves as a powerful tool for fostering equitable and engaging learning experiences while preserving the core values of education.
Analyzing the Evolution of Generative AI Curricula From Basics to Advanced Applications in 2024 - Multimodal Generative Models for Interdisciplinary Learning
Multimodal generative models are becoming increasingly important for creating learning experiences that cross traditional subject boundaries. These models handle various types of information, such as text, images, and audio, which allows for richer educational interactions and encourages students to explore applications across fields like the sciences, humanities, and social studies. While these models offer significant potential, they also introduce complex ethical questions about their use and the biases they might unknowingly carry. As schools and universities integrate these advanced models, it's crucial to carefully consider their broader impact on society while taking advantage of their strengths in promoting collaboration and integration within learning. The field of multimodal generative AI in education is constantly changing as people recognize their capacity to transform learning.
Multimodal generative models, including those that handle combinations of text, images, audio, and video, are becoming increasingly important in both research and industry. These models, such as GPT-4V and Sora, have the ability to understand and create content across multiple formats, which opens up new possibilities in education and beyond. The idea is that a richer, multi-sensory experience could enhance learning, especially for more complex subjects that are difficult to convey through a single modality.
Some studies suggest that using multimodal approaches can lead to better retention of information in educational settings. This makes intuitive sense, since it's more stimulating to engage with multiple senses during learning. Whether this consistently translates into better learning outcomes still needs more exploration. However, this potential benefit is exciting, especially when it comes to diverse learning styles.
While the benefits are intriguing, these models are also computationally intensive. The larger and more complex models require robust hardware, which could create a digital divide if institutions and students don't have access to this type of technology. Ensuring equal access to the resources needed for multimodal AI will be a challenge that needs careful consideration going forward.
Interestingly, the use of diverse data inputs can be a way to identify and reduce biases in AI systems. The assumption is that by looking at various data types, models can build a more balanced and inclusive understanding of the world. This capability is important for avoiding perpetuation of existing stereotypes and creating a more equitable AI learning environment. However, this also depends on the training data itself being diverse and unbiased, which is always a crucial consideration.
These models can be tailored to each learner, offering more personalized learning experiences. For example, AI systems could modify the way they present information based on text or voice input from a student. This personalized approach has the potential to increase learner engagement and motivation, though it's not clear how broadly effective this might be in different educational contexts.
Researchers are exploring how cognitive science can improve the user-friendliness of multimodal models. The goal is to make the interaction with AI feel more natural and intuitive. However, human cognition is extremely complex, and fully translating it into a computational model is a monumental challenge. This remains a big area of active research, and it will be interesting to see how effective these techniques become in future generations of models.
One of the intriguing applications of these models in education is the ability to create simulations or virtual environments. This approach could be particularly helpful for subjects that require hands-on experience, like engineering or medicine. Students could practice in safe, controlled environments that mimic real-world conditions. It will be important to assess how effectively these simulations reflect real-world complexity.
The introduction of multimodal learning necessitates a reevaluation of how we assess student learning. The traditional methods might not be as suitable when students are interacting with various formats of information. Developing new ways to evaluate a student's understanding in this dynamic context is a challenge that needs creative solutions.
These models have the potential to improve engagement in distance learning, which has become more prevalent in recent years. By incorporating video and audio, multimodal tools can create more dynamic and engaging learning experiences compared to just relying on text-based interactions. This is a vital area to focus on as educational paradigms continue to evolve.
Though the potential of multimodal generative models is considerable, we still need to carefully consider their limitations. The models are complex and can be prone to misinterpretation or biases if not properly understood and used. It's important for educators to have training in how to effectively leverage these tools while being mindful of their strengths and weaknesses. Ensuring they are used responsibly and don't inadvertently amplify existing inequities in educational outcomes will require thoughtful discussion and ongoing research.
Analyzing the Evolution of Generative AI Curricula From Basics to Advanced Applications in 2024 - Ethical Considerations and Data Privacy in AI-Enhanced Education
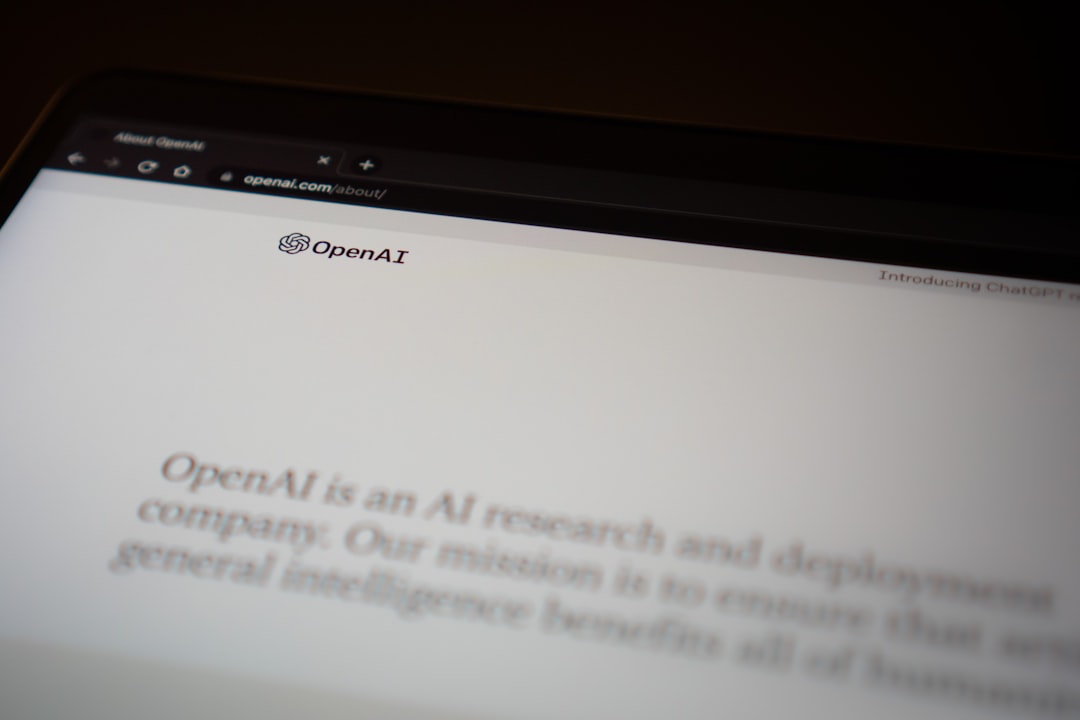
The use of AI in education presents a complex ethical landscape, particularly regarding student data privacy. The collection and analysis of vast amounts of student information raise concerns about how this data is handled and used. Issues like obtaining proper informed consent, the potential for bias embedded in AI algorithms, and the risk of data misuse demand a heightened awareness. While AI offers potential benefits like personalized learning, it's vital to ensure these advancements don't undermine students' privacy or worsen existing educational inequities. A collaborative effort between educators, AI developers, and ethicists is crucial to establish and implement clear ethical guidelines for AI in education. Moreover, continuous professional development focusing on AI ethics and data protection is essential for navigating the complexities introduced by AI in teaching and learning. Moving forward, a careful balancing act is necessary to promote innovation while maintaining ethical integrity in the educational process.
The incorporation of AI into education, while promising, presents a complex web of ethical considerations, particularly around data privacy and security. As AI systems in education gather and analyze vast quantities of student data, concerns arise about ownership and control of this information. Students might unknowingly surrender their data rights, highlighting the necessity for clear and transparent consent mechanisms within educational settings.
Despite advancements in AI, the development of robust ethical frameworks for its responsible use in educational contexts lags behind. This raises worries regarding the potential for unintended consequences, like surveillance-based learning environments. While AI-powered tools aim to enhance student engagement, they also risk fostering an atmosphere of constant observation, possibly stifling creativity and genuine engagement with the material.
AI's ability to personalize learning and automate assessments offers significant benefits. However, the very systems designed for enhancement can amplify biases embedded in the training data. This could inadvertently exacerbate existing inequalities in educational outcomes, emphasizing the need for rigorous scrutiny of bias in the design and implementation of AI educational tools.
The rapid proliferation of AI in education also necessitates a careful balancing act with evolving privacy regulations like GDPR and FERPA. Staying compliant with these regulations is becoming increasingly complex as the technologies continue to evolve. It's a delicate dance between protecting sensitive student data while reaping the benefits of AI-driven insights.
Many students and parents may not have a complete understanding of how AI technologies impact their data and privacy. Therefore, fostering genuinely informed consent becomes crucial. Without a clear understanding of the implications, there's a greater risk of ethical breaches and erosion of trust.
Furthermore, the "black box" nature of generative AI algorithms can create accountability dilemmas. When an AI system makes incorrect judgments about student performance or capabilities, it can be challenging to identify responsibility for the error. This muddies the educational landscape and makes it difficult to rectify mistakes or improve the systems.
Tools that use AI for emotion recognition, while potentially beneficial in delivering tailored feedback, tread on delicate ethical ground. Utilizing emotional data, especially from students, requires careful consideration and ethical boundaries to prevent exploitation or misuse of students' emotional states.
Ironically, while AI aims to reduce cognitive load by automating tasks, it can also create new complexities that can be burdensome for learners. Students could face overwhelming amounts of AI-driven interventions, potentially interfering with effective learning processes.
The availability of AI tools that can mimic human outputs introduces new challenges to academic integrity. Plagiarism and authentication of student work become more challenging in an environment where AI-generated content is increasingly sophisticated. Institutions need to engage in a robust dialogue surrounding AI's role in assessments to preserve the core values of academic honesty.
In conclusion, the changing landscape of education requires a shift in the role of educators. Teachers must now navigate the ethical dilemmas of AI integration and guide students in the responsible usage of these powerful tools. Cultivating critical thinking skills, alongside subject-matter knowledge, is more important than ever in an educational environment where AI plays an increasingly significant role. This ongoing evolution requires a continuous and collaborative dialogue between educators, researchers, and students to ensure that AI truly serves the core purpose of education – fostering knowledge, understanding, and a commitment to lifelong learning.
Analyzing the Evolution of Generative AI Curricula From Basics to Advanced Applications in 2024 - Practical Applications of Generative AI in Academic Research
Generative AI is increasingly impacting academic research, offering new tools for analyzing both qualitative and quantitative data. The emergence of advanced language models and computer vision systems has expanded the possibilities for generative AI applications in research. While these advancements promise to improve research efficiency and scope, they also raise concerns about academic integrity and potential misuse. Discussions around AI-generated content and plagiarism have become more prominent as these technologies become more sophisticated.
Furthermore, applications like generating knowledge graphs demonstrate the potential of generative AI to synthesize large datasets. However, researchers need to critically assess the potential biases inherent in these models and carefully evaluate the results produced to ensure the integrity of their findings. Maintaining research standards while employing generative AI requires a thoughtful approach that balances innovation with established principles of academic practice. Researchers must navigate this evolving landscape, understanding the powerful capabilities of generative AI while simultaneously mitigating potential risks to ensure the integrity of academic research.
Generative AI's capabilities are proving quite useful in various research areas, especially within education. It's fostering a new level of collaboration across fields like linguistics, psychology, and education. Researchers are pooling their knowledge to refine AI models that tackle complex learning challenges.
Furthermore, the way we design and develop academic programs is being transformed by generative AI. Educational institutions can now use data-driven insights to dynamically tailor curricula, creating a more responsive learning experience that potentially boosts student success.
Generative AI applications have also advanced text and speech synthesis capabilities. This allows for the creation of personalized learning environments that adapt to students' preferred learning styles, which is potentially improving understanding and recall of information.
We see its use in design and engineering, where students can leverage generative AI for quick prototyping. They can experiment with design concepts faster compared to conventional techniques. This accelerated learning process encourages a more innovative mindset in students.
AI-generated content has the potential to be customized to learners with various attention spans and learning preferences. It can cater to visual, auditory, and kinesthetic styles, leading to improved engagement for a wider range of students.
The development of real-time simulations powered by generative AI is quite interesting. These simulations can adapt based on user input, providing students with interactive experiences that replicate scenarios in fields like engineering and healthcare. This experiential learning approach is promising.
Generative AI excels at processing large volumes of research literature and summarizing key points and emerging trends. This allows researchers to stay current on advancements without getting bogged down in an overwhelming amount of information.
We're also seeing applications that focus on identifying and minimizing biases in datasets. This is promising for promoting fairness and enhancing the overall quality of research.
AI tools are beginning to be used in the peer-review process for academic publications. It potentially streamlines the evaluation process and cuts down on revision time, potentially accelerating the dissemination of research findings. Whether this improves the quality of published research remains to be seen.
There's a growing trend toward automating various administrative tasks at academic institutions. Generative AI can assist with everything from class scheduling to student enrollment, freeing up researchers and educators to concentrate on more important tasks like teaching and innovation.
It's an exciting time for generative AI within academic research, and we are only beginning to explore its full potential. However, it's important to critically assess its implications, including any unforeseen biases or consequences.
Analyzing the Evolution of Generative AI Curricula From Basics to Advanced Applications in 2024 - Future Trends and Challenges in AI-Driven Educational Technology
The future of education is increasingly intertwined with AI-driven technologies, bringing about a wave of both exciting prospects and complex challenges. The rise of generative AI, particularly through large language models, is rapidly changing how learning takes place. Educators are now faced with the task of helping students develop a strong understanding of AI, promoting critical thinking skills that are essential in this changing landscape. Personalized learning experiences are becoming more commonplace with the growing adoption of AI-powered tools, but this progress also introduces significant concerns about protecting student data and addressing potential biases within AI systems. Developing proficiency in areas like prompt engineering and understanding the ethical dimensions of AI usage are becoming vital skills for both students and educators. Moving forward, educational communities will need to create comprehensive frameworks for managing the use of AI in education. Striking a careful balance between innovation and responsible AI practices will be central to ensuring a future where AI enhances, rather than undermines, the core values of education.
The integration of AI in education is accelerating, with generative AI models like LLMs rapidly becoming a mainstay in learning environments. This shift, spurred by tools like ChatGPT, has led to a surge in initiatives focused on utilizing AI for text generation and learning applications. The Education 40 Alliance's focus on AI's potential to transform learning underscores its promise, but also highlights the necessity for the field to meticulously address the complexities of its application.
Discussions are centered on the critical need for AI literacy, prompt engineering abilities, and strong critical thinking skills – essential competencies for both students and teachers as they navigate this new educational landscape. AI has the capacity to help overcome learning gaps stemming from recent global events by providing adaptable educational resources that cater to individual student needs and strengths. This potential for personalization could lead to a shift in how we teach, potentially creating more tailored learning pathways for students with diverse learning profiles.
Examining the influence of generative AI on education necessitates exploring areas like changes in knowledge and skill assessments, the emergence of new educational use cases, and the effects on student creativity and critical thinking. Educators are facing immediate challenges in integrating these AI tools effectively into their classrooms. Initiatives like MIT Open Learning's "Generative AI in Education" symposium emphasize the imperative for educators to anticipate and understand the implications of AI for the future of education.
AI's capability to leverage real-time student data to personalize curricula is becoming increasingly refined. Understanding the intricacies of human cognition, like how cognitive load impacts learning, is now a crucial consideration when designing AI tools that support the learning process. There is an ongoing concern whether current AI models can accurately simulate various facets of cognitive function.
Integrating adversarial training into the development of AI models is becoming common practice to improve their resilience against manipulation. While promising, adversarial training can also bring added complexities and unpredictability, particularly in the educational context.
The burgeoning field of AI-powered educational applications necessitates careful consideration of ethical implications around student data use. Developing clear policies and frameworks regarding informed consent, data ownership, and data protection is paramount to ensuring responsible AI adoption.
AI models are now being developed with the capability to assess and respond to student emotional cues. This presents both opportunities to provide tailored feedback and considerable ethical questions about the privacy and appropriateness of emotional monitoring.
The proliferation of multimodal AI models, while promising, raises questions about the accuracy of these models in representing complex human learning processes. Different modalities can lead to different interpretations of content, potentially hindering the effectiveness of the technology.
The development of more sophisticated AI models demands significant computational resources, raising concerns about accessibility and equity. Institutions with limited resources could be at a disadvantage, leading to the widening of existing educational gaps.
The integration of generative AI across disciplines, while potentially fostering innovative solutions, is also challenging traditional educational structures. Curricula and assessment methods need to adapt to incorporate these interdisciplinary approaches.
The capacity of AI to generate content that is nearly indistinguishable from human writing poses challenges for educational integrity and the authentication of student work. The need for new methods of assessment and maintaining academic honesty is essential.
Simulations powered by AI offer exciting potential for hands-on learning in fields like healthcare and engineering, but the question remains if these simulations can adequately capture the unpredictable nature of real-world scenarios, and if they prepare students for those complexities.
In conclusion, the future of AI-powered education presents a complex mix of opportunities and challenges that will require continued exploration, collaboration, and critical reflection. Understanding and addressing the evolving ethical and technical complexities will be essential in realizing the full potential of AI while upholding the core values of education.
Create AI-powered tutorials effortlessly: Learn, teach, and share knowledge with our intuitive platform. (Get started for free)
More Posts from aitutorialmaker.com: